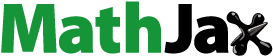
ABSTRACT
The effects of gender stereotype threat on mathematical test performance in the classroom have been extensively studied in several cultural contexts. Theory predicts that stereotype threat lowers girls’ performance on mathematics tests, while leaving boys’ math performance unaffected. We conducted a large-scale stereotype threat experiment in Dutch high schools (N = 2064) to study the generalizability of the effect. In this registered report, we set out to replicate the overall effect among female high school students and to study four core theoretical moderators, namely domain identification, gender identification, math anxiety, and test difficulty. Among the girls, we found neither an overall effect of stereotype threat on math performance, nor any moderated stereotype threat effects. Most variance in math performance was explained by gender, domain identification, and math identification. We discuss several theoretical and statistical explanations for these findings. Our results are limited to the studied population (i.e. Dutch high school students, age 13–14) and the studied domain (mathematics).
Since the first studies on the negative effect of stereotype threat on women’s math performance (Spencer, Steele, & Quinn, Citation1999), numerous studies have addressed both the generalizability of the effect and important theoretical moderators (Spencer, Logel, & Davies, Citation2016). Although several meta-analyses of published studies highlighted relatively robust effects (Nguyen & Ryan, Citation2008; Picho, Rodriguez, & Finnie, Citation2013; Walton & Spencer, Citation2009), some researchers have voiced their concern about the improper use of covariates that leads to inflated Type I error rates in stereotype threat studies (Stoet & Geary, Citation2012; Wicherts, Citation2005), and the potentially overestimated effects of stereotype threat due to publication bias and related biasing factors regarding how researchers analyze their data and present their results (Flore & Wicherts, Citation2015; Ganley et al., Citation2013). These problems can impede our understanding of psychological phenomena like the effects of stereotype threat on test performance, and raise questions about the generalizability of the effect across cultural settings and age groups. Such issues can be (partly) resolved by pre-registration (see e.g. Wagenmakers, Wetzels, Borsboom, van der Maas, & Kievit, Citation2012) of large confirmatory stereotype threat studies.
Most of the research on gender stereotype threat in the math domain concerned college students, however, it is clear that early effects of stereotype threat on high school students could potentially have a negative long-term impact on girl’s identification with mathematics and hence their later performance in this domain and related domains (viz. Science, Technology, Engineering, and Mathematics or STEM fields). Several studies have addressed stereotype threat effects among girls in diverse cultural contexts (see Flore & Wicherts, Citation2015 for a review), and the results are somewhat mixed. It is clear that studies in actual class settings (instead of lab settings) among high school populations would throw important light on the generalizability of gender stereotype threat effects to mundane settings that are relevant for pupils’ later academic careers. Moreover, a large-scale study in a new cultural context adds to knowledge about the generalizability of stereotype threat effects in classroom environments that have hitherto been studied only in a limited number of countries.
In this registered report, we aimed to obtain a reliable and unbiased estimate of the effects of negative gender stereotypes on the mathematical test performance among Dutch high school students. Additionally, we aimed to replicate the moderating effects of variables domain identification (Keller, Citation2007a), gender identification (Schmader, Citation2002), math anxiety (Delgado & Prieto, Citation2008), and test difficulty (Keller, Citation2007a) in a large sample of Dutch high school students.
Stereotype threat and underlying mechanisms
Stereotype threat theory predicts that members of a negatively stereotyped group will underperform when that stereotype is made salient or relevant for the task at hand. In their seminal paper on stereotype threat, Steele and Aronson (Citation1995) described how African Americans underperformed on cognitive ability tests when reminded of the negative stereotype stating that African Americans have lower intellectual abilities than European Americans. Similarly, when confronted with the negative stereotype concerning their in-group, women were found to underperform on mathematics tests (e.g. O’Brien & Crandall, Citation2003; Spencer et al., Citation1999) and driving tests (Yeung & von Hippel, Citation2008), elderly were found to underperform on memory tests and cognitive tests (Lamont, Swift, & Abrams, Citation2015) and students from lower socio-economic backgrounds were found to underperform on intelligence tests (Désert, Préaux, & Jund, Citation2009; Spencer & Castano, Citation2007). Based on theory, members of positively stereotyped groups (e.g. men or European Americans) are expected to remain uninfluenced by stereotype threat manipulations.
Of the many negative stereotypes that have been studied in the context of stereotype threat, the stereotype that women are not as good in mathematics as men (Spencer et al., Citation1999) is one of the most frequently studied. Multiple meta-analyses on this topic have produced similar results: the estimated averaged effect size ranges from small (d = 0.24) to medium (d = 0.48), indicating that women tend to underperform when they are exposed to explicit or implicit stereotype threats (Doyle & Voyer, Citation2016; Nguyen & Ryan, Citation2008; Picho et al., Citation2013; Walton & Cohen, Citation2003; Walton & Spencer, Citation2009). The studies included in aforementioned meta-analyses were carried out in different countries (with samples from Canada, France, Germany, Italy, the Netherlands, Spain, Uganda, United Kingdom, and United States) and the participants were usually either college students or students from primary or secondary education. The effect sizes within these meta-analyses show a considerable amount of heterogeneity, indicating that the magnitude of the effect sizes varies across studies (Nguyen & Ryan, Citation2008; Picho et al., Citation2013), possibly due to moderators.
Moderators
Spencer et al. (Citation2016) and Inzlicht and Schmader (Citation2012) reviewed the main moderators of the effects of stereotype threat. Here, we focus on the three most relevant individual characteristics of female test-takers that are thought to moderate susceptibility to stereotype threat and consider test difficulty as an important factor in determining whether tests are affected by stereotype threat.
Domain identification
Theory predicts that members of negatively stereotyped groups will only underperform on stereotype relevant tasks if they are highly identified with the construct that the task is supposed to measure (Keller, Citation2007a; Steele, Citation1997; Steele & Aronson, Citation1995). Notably, stereotype threat will only undermine mathematics test performance for women who consider the subject of mathematics to be important to them. For women who are weakly identified with mathematics, the negative stereotype will not trigger anxiety or negative thoughts during test-taking because they are probably less interested in good results in mathematics compared to women who strongly identify with mathematics. This theoretical prediction is supported by several studies showing that women with high domain identification under threat average larger performance decrements than women with low domain identification (Keller, Citation2007a; Lesko & Corpus, Citation2006; Steinberg, Okun, & Aiken, Citation2012). The meta-analytic evidence in favor of the moderating effect of domain identification is somewhat mixed. Walton and Cohen (Citation2003) found that studies with samples consisting of highly identified participants in the stereotyped domain showed larger stereotype threat effects than studies that did not select samples of highly domain-identified group members. Yet, Nguyen and Ryan (Citation2008) found that samples of moderately math-identified women were more strongly influenced by stereotype threats than highly math-identified women.
Gender identification
A second moderator that received attention in the stereotype threat literature is group identification, i.e. the degree to which the test-takers consider membership of the stereotyped group to be an important part of their self-identity (Schmader, Citation2002). The moderating effect of gender identification follows the same logic as the moderating effect of domain identification: women who do not strongly identify with their gender have little reason to feel threatened by the negative female stereotype. Several studies have shown that indeed math performance is generally less affected by stereotype threat for women who believed that gender was not an important part of their identity, compared to women for whom gender was an important part of their identity (Schmader, Citation2002; Wout, Danso, Jackson, & Spencer, Citation2008). However, other studies failed to find moderating effects of gender identification (Cadinu, Maass, Frigerio, Impagliazzo, & Latinotti, Citation2003; Eriksson & Lindholm, Citation2007), or even found women having lower levels of gender identification to be more strongly influenced by negative stereotypes compared to women who were more strongly gender identified (Kiefer & Sekaquaptewa, Citation2007).
Math anxiety
A third construct implicated as both a moderator and a mediator of stereotype threat is math anxiety. First, the gender differences in mathematical test performance could be partly mediated by state anxiety (Osborne, Citation2001) and state anxiety is sometimes (albeit not always; Schmader & Johns, Citation2003; Steele & Aronson, Citation1995) found to mediate the stereotype threat effect: under stereotype threat women not only scored lower on the mathematics tests compared to men and women in the control condition, but they also showed higher scores on physiological anxiety measures like skin conductance, blood pressure, and lower scores on skin temperature (Osborne, Citation2007). Women in threat conditions tend to link gender stereotypes to their own perception of anxiety more strongly than women in low threat conditions or men (Johns, Schmader, & Martens, Citation2005). Finally, state anxiety mediates the relationship between coping sense of humor and mathematics test performance for women (Ford, Ferguson, Brooks, & Hagadone, Citation2004). Instead of studying state anxiety as mediator, trait math anxiety can be treated as a moderator variable of the stereotype threat effect. Overall, there is a gender gap in reported math anxiety, with girls reporting a higher level of math anxiety than boys (Else-Quest, Hyde, & Linn, Citation2010). A study on Spanish high school students showed that math anxiety moderated the stereotype threat effect, in the sense that higher math anxiety scores were associated with stronger decrements under stereotype threat (Delgado & Prieto, Citation2008).
Test difficulty
Finally, studies have shown that gender stereotype threat is moderated by math test difficulty in both college samples (O’Brien & Crandall, Citation2003; Spencer et al., Citation1999) and school samples (Keller, Citation2007a; Neuville & Croizet, Citation2007). In most of these samples, stereotype threat effects were stronger for difficult tests than for easier tests (Neuville & Croizet, Citation2007; Nguyen & Ryan, Citation2008; Spencer et al., Citation1999). Use of easy tests can actually lead to improved scores for girls under stereotype threat, probably due to heightened motivation and lower threat posed by such easier tests (O’Brien & Crandall, Citation2003; Spencer et al., Citation2016). Some researchers suspected that students who work on difficult tests might experience more physiological arousal (Ben-Zeev, Fein, & Inzlicht, Citation2005; O’Brien & Crandall, Citation2003), resulting in larger performance decrements under stereotype threat. A third explanation is that more difficult tests require more controlled attention as part of working memory than easier tests. Because working memory can be occupied by suppression of negative thoughts concerning the stereotypes or other situational pressures (Beilock & Decaro, Citation2007; Beilock, Rydell, & McConnell, Citation2007; Schmader & Johns, Citation2003), test-takers under threat might experience greater difficulty solving the more difficult problems. This would result in larger performance decrements on the more difficult tests.
Stereotype threat in school aged children
Although the theory of stereotype threat has been well established based on lab studies, the critique that these studies were limited in terms of generalizability drove stereotype threat researchers into the classroom (Aronson & Dee, Citation2012; Wax, Citation2009). A first study in the United States on stereotype threat in elementary and middle schools showed that the salience of gender lowered mathematical test performance of girls (Ambady, Shih, Kim, & Pittinsky, Citation2001). However, this finding was limited to age groups of 5–7 and 11–13, and did not appear among students aged between 8 and 10. Ambady et al. argued that this might have been due to the higher degree of chauvinism regarding gender in the latter age group, but this explanation has received little attention in further studies on stereotype threat. Nonetheless, the effects of stereotype threat for girls was also found in other countries, like France (Bagès & Martinot, Citation2011), Germany (Keller, Citation2007a; Keller & Dauenheimer, Citation2003), Italy (Muzzatti & Agnoli, Citation2007), Spain (Delgado & Prieto, Citation2008), and Uganda (Picho & Stephens, Citation2012). However, in several similar experiments conducted in Italy and the United States the null hypothesis was not rejected (e.g. Agnoli, Altoè, & Muzzatti, Citationn.d.; Cherney & Campbell, Citation2011; Ganley et al., Citation2013; Stricker & Ward, Citation2004). Effects of stereotype threat on math performance among college students have been found in the Netherlands before (Marx, Stapel, & Muller, Citation2005; Wicherts, Citation2005). However, we are not aware of any published stereotype threat studies on the gender–math relationship conducted at Dutch high schools. Our study fills this gap in the literature.
As with adult samples, the results of previous stereotype threat experiments among girls are mixed; the estimated effect sizes of the simple effect (i.e. the standardized mean difference of girls in the stereotype threat condition and girls in the control condition) ranged from a large effect in the expected direction to a medium effect in the opposite direction. Combining the information of all available stereotype threat experiments for school aged girls yielded an average estimated effect size of 0.22 in the expected direction, but also substantial heterogeneity in underlying effects (Flore & Wicherts, Citation2015).
Methodological considerations
Three methodological and statistical issues in the replicability debate (Asendorpf et al., Citation2013) are particularly relevant for stereotype threat research: pre-registration, a priori power analyses and multilevel analysis. First, pre-registration has received little attention in articles on stereotype threat (for exceptions, see Finnigan & Corker, Citation2016; Gibson, Losee, & Vitiello, Citation2014; Moon & Roeder, Citation2014). There are several upsides to pre-registered studies. Notably, when a study is pre-registered it is easier to certify that statistically significant results were actually based on a priori hypotheses and pre-specified analyses thereof. This counters biases caused by hypothesizing after results are known (i.e. HARKing, Kerr, Citation1998) and ad hoc analyses of the data that are focused on finding desirable (usually significant) results (Wagenmakers et al., Citation2012; Wicherts et al., Citation2016). Moreover, pre-registration ameliorates the effects of publication bias by assuring publication of results regardless of the outcome.
Second, it is crucial to conduct proper a priori power analyses. The samples of schoolchildren gathered in stereotype threat experiments are relatively small and power analyses are not often reported (for exceptions, see Stricker & Ward, Citation2004; Titze, Jansen, & Heil, Citation2010). Because the average effect sizes in the field have consistently been shown to be small to medium, we suspect that many stereotype threat studies reported in the past were underpowered, leading to inaccurate effect size estimates without publication bias and inflated estimates of effect sizes under various scenarios with publication bias. Prior power analyses enable informed decisions regarding the sample sizes needed for studying relatively subtle effects.
Third, it is important to consider the clustered nature of data gathered in schools in the analysis of the data from stereotype threat studies. An assumption of common statistical techniques like AN(C)OVA or linear regression analysis is the independence of observations. If students from the same classroom are included in the analysis, this assumption is likely violated. Positive dependencies inflate Type I error rates if left uncorrected. Depending on the severity of the violation, the effective sample size of the study will be lower than the observed sample size (i.e. a larger intraclass correlation [ICC] coefficient will lead to a smaller effective sample size). Thus, the nested structure of the data requires a multilevel analytic approach.
In the present study, we incorporated these three improvements. Our registered experiment is not designed to “prove” or “disprove” the general existence of the stereotype threat phenomenon, but rather to study the effects of a common stereotype threat manipulation in the Dutch high school population in actual classrooms. The Dutch are fairly regular in terms of gender stereotypes (Miller, Eagly, & Linn, Citation2015) and studying stereotype threat in this context contributes to much needed information about when and among which students stereotype threat affects mathematics test performance. On top of that, we believe that the method we use (i.e. pre-registration, a priori power analysis, and multilevel analysis when observations are dependent) could solve some existing problems in the field if adopted in future stereotype threat studies.
In our registered study, we used materials and procedures that are commonly used in the stereotype threat literature. We used an experimental paradigm that involved both an explicit stereotype threat manipulation (Spencer et al., Citation1999) and a control condition in which the negative stereotype was actively nullified (Smith & White, Citation2002). We selected a sample of high-achieving students, for which the effects of stereotype threat are expected to be strongest due to higher levels of domain identification (Steele, Citation1997; Steinberg et al., Citation2012). Moreover, in our study, boys and girls worked simultaneously on the mathematics test in regular classrooms. We did so because the presence of boys has been found to yield larger decrements in girls’ mathematics test performance due to stereotype threat (Huguet & Régner, Citation2007). Our main hypothesis was to find an interaction effect between stereotype condition and gender on the number of correct questions on the math test. We expected a simple effect for girls, with higher performance for girls in the safe control condition. Based on theory, we had no specific expectation for the simple effects among boys.
Method
Participants
The participants were students attending the second year of Dutch high school (typically 13–14 year olds), which is equivalent to the eighth grade in the US school system. We selected average to high-achieving students by including classes from the second highest education level “Hoger Algemeen Voorgezet Onderwijs” (i.e. senior general secondary level or HAVO) and highest education level “Voorbereidend Wetenschappelijk Onderwijs” (i.e. pre-university secondary education or VWO) in the Dutch high school system. In our pre-registered sampling plan, we aimed to randomly select schools from a list of high schools offering mixed classes of potential HAVO and VWO students in the Dutch provinces of Noord-Brabant, Utrecht, and Zuid-Holland. However, in practice we had to deviate from this plan, because a large portion of contacted schools (83.33%) declined to participate. After consultation, the editors and we agreed to use a convenience sample at the level of schools, instead of the random sample of schools that we had hoped to select. Additionally, we included two schools outside of our target provinces. Besides these two changes, our sampling plan followed the pre-registration.
Principals of the schools were first contacted by email. In cases where we failed to receive a reply within a week, we contacted the schools by phone, followed by another email if needed. Whenever these three means of contacting were unfruitful, we contacted other schools. Additionally, some schools were contacted in a more informal manner, although we always asked for permission of the principal. Once the principals of the schools agreed to participate, both parents and students of HAVO/VWO classes in the school were asked a week in advance to object if they did not want (their child) to participate. If the student and/or the parents objected, that student was allowed to quietly work on his or her schoolwork during data collection. Participating students were asked to complete the entire set of materials during regular classes in regular classrooms. We planned to sample schools until we had at least 946 girls in our sample (see section Power for the specifics on this number). The Ethics Committee of Tilburg School of Social and Behavioral Sciences approved our study (registration no. EC-2015.53).
Procedure
To heighten the chances of finding an effect, we chose an optimal implementation of the experimental paradigm according to stereotype threat theory. Specifically, we used an explicit threat manipulation, combined with a nullified threat control condition (Steele, Citation1997). Moreover, both boys and girls were present during test-takingFootnote1 (Inzlicht & Ben-Zeev, Citation2000; Sekaquaptewa & Thompson, Citation2003) and we selected classes consisting of average to high-achieving students (Steele, Citation1997). Students received a bundle of materials in a closed envelope. The material consisted of two parts: the first part contained the mathematics test including an introduction in two versions that differed across conditions (an instruction heightening stereotype threat in the experimental condition and a nullification sentence in the control condition). The second part of the materials contained background questions such as gender and age, the manipulation check, and several psychological scales. To assign students to conditions we used a within-cluster approach, i.e. students were individually randomly assigned to either the stereotype threat condition or the control condition within their class.
A female experiment leaderFootnote2 who was blind to the experimental condition instructed students to first read the introduction carefully, to solve the math problems, and finally to fill out the questionnaire. We emphasized that it was important that students would complete all questions in the bundle, but that they could quit the experiment halfway by putting a mark on the first page. The students were allowed 20 min to finish the test, and 10 min to finish the questionnaire. The introduction started with the following piece of text [in Dutch but translated here in English]:
With this mathematics test we want to measure the ability level of high school students. This test has been used in the past. It turned out that students with good grades on this test had on average higher grades in high school and had a better chance to pass their final exam. We would like to know how well high school students in the Netherlands perform on this test.
In the stereotype threat condition, the introduction continued with “The most recent study carried out four years ago showed that boys and girls do not perform equally well on this mathematics test. There was a difference in the average grade on the test between boys and girls”. A similar explicit manipulation has been successfully implemented in past studies (e.g. Delgado & Prieto, Citation2008; Keller & Dauenheimer, Citation2003; Picho & Stephens, Citation2012). In the control condition, the introduction continued with “The most recent study carried out four years ago showed that boys and girls perform equally well on this mathematics test. There was no difference in the average grade on the test between boys and girls”. A similar nullified control condition has been successfully implemented in past studies (e.g. Keller & Dauenheimer, Citation2003; Marchand & Taasoobshirazi, Citation2013; Neuburger, Jansen, Heil, & Quaiser-Pohl, Citation2012). All instructions and materials were in Dutch and are available on the OSF (https://osf.io/yt83j/).
To check whether the students read the introduction, we asked the students to select among four options the correct year in which the mathematics test had been studied before as written in the introduction of the test. The written instruction ended with a warning that students were not allowed to use a calculator. Additionally, students were informed that wrong answers would be punished with a correction for guessing. This was done to induce a prevention focus, which has been found to yield stronger stereotype threat effects (Keller, Citation2007b; Keller & Bless, Citation2008; Ståhl, Van Laar, & Ellemers, Citation2012). Moreover, correction for guessing was (until recently) routinely implemented in high-stakes testing environments like GRE testing (Educational Testing Service, Citation2016), and as such was expected to contribute to creating an atmosphere similar to real-life high-stakes testing. After all students finished reading the introduction and answered the check question, the experiment leader gave them a sign to start working on the mathematics test. Students who finished the mathematics test early were instructed to wait for a signal from the experiment leader, after which they were allowed to continue with the second part of the study. In the second part of the study, students first filled in their age, ethnicity (based on whether both parents were born in the Netherlands or somewhere else), and gender. Subsequently, they were asked to answer the following question as a manipulation check: “Previously boys and girls performed equally on this mathematics test”, which was an item in multiple-choice format that could be answered with either “yes, boys and girls performed equally on this test”, “no, boys and girls did not perform equally on this test” or “I don’t know”. This question was followed by the item “who do you think usually performs better on mathematics tests like these? Boys or girls?”, on which the students could answer by selecting one of the following options: “boys get better grades on math tests”, “girls get better grades on math tests”, “boys and girls get equal grades on math tests”, and “I don’t know”. After answering these manipulation checks, students finished the post-test questionnaire consisting of four scales: gender identification, math anxiety, and two scales of domain identification. After finishing those questionnaires, students were asked to hand in their assessment enclosed in the envelope and to wait silently until everyone was finished.
Materials
The main dependent variable was the score on the mathematics test. We strived to construct a mathematics test with desirable psychometric properties. Specifically, we included items with desirable item properties. To this end, we constructed a mathematics test consisting of 20 items selected from the 2003 TIMSS study (Martin, Mullis, Gonzalez, & Chrostowski, Citation2004). This TIMSS study involved large samples of eighth grade students from 48 countries, including the Netherlands. We used reliably estimated item parameters based on this large international data set (Martin et al., Citation2004) to construct a test with items that varied in difficulty and had relatively high discrimination parameters. The difficulty parameters of the selected items ranged from −0.174 to 1.157 in the overall TIMSS sample. Our test consisted of 8 items in the content domain Geometry and 12 items in the content domain number. Because of the unavailability of the 2003 version (Annemiek Punter, Personal communication, 14 September 2015), we asked two Dutch mathematics teachers with excellent English proficiency to translate the items into Dutch. All items were multiple-choice items with four or five answer categories. To examine the moderating effect of test difficulty, we split the mathematics test in an easy test consisting of the 10 items with the lowest item difficulty parameters, and a difficult test consisting of the 10 items with the highest item difficulty parameters (as estimated in the TIMSS sample).
In addition to this mathematics test, participants filled out two scales assessing different dimensions of domain identification (12 items), a scale measuring gender identification (4 items), and a scale measuring math anxiety (10 items). These four constructs are considered as moderators of the stereotype threat effect among the girls. The first scale of domain identification measured the importance of mathematics according to the students (e.g. “I think mathematics will help me in my daily life”). The second scale of domain identification measured positive affect with regards to mathematics (e.g. “I enjoy learning mathematics”). Both scales were retrieved from the 2003 TIMSS study (Martin et al., Citation2004). We slightly modified the gender identification scale used by Schmader (Citation2002) to fit the population of high school students. The scale consisted of 4 items (e.g. “being a girl/boy is an important part of my self-image”). Finally, we used the Math Anxiety Scale (Prieto & Delgado, Citation2007) to measure math anxiety (e.g. “before taking a math exam I feel nausea”). Although this scale originally contained 18 items, we created a shorter version to deal with time constraints by selecting 10 items with sufficient variance in the item difficulty parameters. Answers to all scales were given on a five-point Likert scale ranging from does not apply to me to does apply to me. The scales were translated into Dutch by the first author, and those translations were checked for deviations from the original by the third author.
Pilot study
To ensure that the materials were appropriate for the targeted population, we conducted a pilot among 76 high school students from three classes of a school in the province of Zuid-Holland (21 girls, 54 boys, 1 gender unknown). With these pilot data, we checked whether floor or ceiling effects occurred, whether the items had desirable psychometric properties, whether the time allotted for the different parts of the study was sufficient, and whether instructions and manipulation checks were successful. For the pilot study, we carried out the exact procedure as described above apart from three minor details.Footnote3 Scale analyses were conducted using R packages “CTT” (Willse, Citation2014) and “Scale” (Giallousis, Citation2015).
The mean number of correct items on the math test was M = 12.41 out of 20 items (SD = 2.74), with individual scores ranging from 7 to 18. Of the 76 students, 96% answered the read check correctly and 74% answered the manipulation check correctly. Scale reliability of the four psychological scales ranged from acceptable (Cronbach’s αtest anxiety = .68 and Cronbach’s αgender identification = .67) to good (Cronbach’s αliking math = .82 and Cronbach’s αimportance math = .81). Three items of the test anxiety scale showed item–rest correlations smaller than .30, and showed confirmatory factor analysis single-factor loadings smaller than .30 (items 5, 7, and 8). We decided to replace the test anxiety scale with a Math Anxiety Scale, based on both psychometric arguments (i.e. reliability of the scale was somewhat low, some items showed low factor loadings) and theoretical arguments (i.e. the Math Anxiety Scale is more likely to moderate stereotype threat than the test anxiety scale). The item–rest correlations for gender identification items were all .30 or higher, as were the standardized factor loadings. Because the scale analyses of the latter three scales showed satisfactory results we did not alter these scales.
The times allotted for the mathematics test (20 min) and the questionnaire (10 min) were both sufficient. We experienced no problems with the instructions in the pilot.
Statistical analysis
Main analysis
In , we present an overview of our planned analyses. For our main analysis, we first used an F-test to test for differences in mathematical performance between the classes. If this F-test showed a p-value <.05, we planned to conduct a multilevel analysis with the observed individual scores as first level and the class level as the second level. Here we planned to use a random intercepts model, with fixed slopes for the main effects and the interaction effect. We also planned to include two second-level predictor variables: gender of the teacher (GT) and class composition (CC), which was defined as the percentage of girls present in the classroom. For individual i in classroom j, we defined the model as:
We assumed that the scores are mutually independent N(0, σ2). On the second level, the model was defined as:
These analyses were run with the R-package lme4. In the case that the F-test for the class effect would show a p-value >.05, we planned to ignore the nested structure, and to conduct a standard two-way ANOVA instead of a multilevel analysis. As pre-registered, all analyses were carried out thrice. First, we ran analyses with the guess corrected score on the complete math test as the dependent variable. For the second analysis, we ran the analysis with the 10 easiest questions on the math test as dependent variable, and for the third analysis we used the dependent variable consisting of the 10 most difficult questions. We used a guess correction based on formula scoring (Frary, Citation1988).
We expected a significant interaction between the stereotype threat condition and gender, with a smaller effect for the easy subtest than for the difficult subtests. If this interaction was significant at α = .05, we planned to proceed to an analysis of simple effects. We hypothesized that girls in the stereotype threat condition would score lower on the mathematics test than girls in the control condition, and planned to test this with a one-sided test at α = .05. We had no hypothesis for the simple effects analysis for boys, thus we treated this analysis as exploratory.
Additionally, we registered to test multiple competing inequality and equality constrained hypotheses using the Bayes factor (Jeffreys, Citation1961; Kass & Raftery, Citation1995). Bayes factors have the advantages that they can be straightforwardly used for simultaneously testing multiple (i.e. more than two) non-nested hypotheses and that they allow one to quantify the evidence in the data in favor of a hypothesis (e.g. the null) relative to another hypothesis. These properties are not shared by classical p-values. presents our pre-registered competing hypotheses of interest.
Table 1. Competing hypotheses Bayesian analysis.
For the no stereotype threat hypothesis H0, we placed equality constraints on the means for the conditions, while allowing the means on mathematical test scores for boys and girls to differ. This no stereotype threat hypothesis could subsequently be compared to the stereotype threat hypothesis H1, and the stereotype threat and stereotype lift hypothesis H2.Footnote4 Finally, we compared all of these hypotheses with the complement hypothesis HC. To compare these hypotheses, we used the default Bayes factor methodology of Mulder (Citation2014), Gu, Mulder, Deković, and Hoijtink (Citation2014), and Gu, Mulder, and Hoijtink (Citation2018). In this methodology, the data are implicitly split in a minimal fraction that is used for prior specification and a maximal fractional that is used for hypothesis testing (O’Hagan, Citation1995). Therefore, default Bayes factors can be used in an automatic fashion without needing to formulate prior distributions for the anticipated effects (Berger & Pericchi, Citation1996). Our pre-registered interpretation of Bayes factors follows guidelines presented in Kass and Raftery (Citation1995) and is shown in .
Table 2. Interpretation Bayes factors.
Moderators
We considered two versions of domain identification, gender identification, and math anxiety as potential moderators. The moderators were separately added to the model tested in the section Main analyses, which means we planned to test three models. The moderator variable, the three-way interaction term (i.e. Condition × Gender × Moderator) and subsequent second-order interaction terms were added as first-level predictors. All moderator variables were treated as continuous variables, and were grand-mean centered.
We pre-registered that a potential significant three-way interaction would be followed by three analyses to inspect the interaction of condition and gender on the number of correctly answered mathematics items separately for students with low scores on the moderator (one standard deviation below the mean), average scores on the moderator (the mean), and high scores on the moderator (one standard deviation above the mean). In cases of a significant Condition × Gender interaction, we planned to proceed to simple effects to inspect the effect of condition for girls and boys separately. Finally, if more than one moderator variable would show a significant three-way interaction, we planned to run a final model with all of those variables included.
Power
Because the main focus of this registered report is to replicate the stereotype threat effect, we conducted a power analysis for the interaction effect and the simple effect for girls. Moreover, we conducted a power analysis for the moderating variables. All power analyses were carried out using G*Power 3.1.3 and with the goal to obtain a power of at least .80 for all analyses.
For the interaction effect, we used the information from the largest stereotype threat study administered in high schools that we are familiar with (Stricker & Ward, Citation2004). In this sample, the effect size η2interaction was larger than .05, but smaller than .10. A power analysis with η2 = .05 indicated that we would need a total sample size of 152. Subsequently to find an effect size of d = 0.30 in the analysis of simple effects (one-sided) for girls we would need 278 participants. We selected this effect size because we took precautions to maximize the effect (e.g. select average- to high-achieving participants, have members of the other sex present, construct a difficult test), leading us to expect a somewhat larger effect than the averaged effects of the meta-analyses.
Due to the nested structure of the data, we expected the observations within classes not to be completely independent, which meant that these power analyses are too liberal. We corrected for this dependency by multiplying the needed sample size under the assumption of independent observations with the design effect. To calculate the design effect, we used the following formula in which K is the number of classes, is the number of children within class K and ρ is the ICC.
We assumed that ρ = .10 and . This will lead to a design effect of 3.4. Therefore, to obtain enough power for the simple effects analysis we multiplied the calculated sample size (i.e. 278 girls) by 3.4, leading to a required sample of 946 girls. Because we did not expect a difference in mathematics scores between the experimental and control conditions for boys, there was no need to conduct a power analysis for these simple effects. Hence, we simply sampled schools until we obtained enough girls in our sample, while also measuring boys because the theory stipulates no effect for them, and because it is crucial to have boys present during the testing of the girls.
We also calculated total required sample sizes (i.e. girls and boys together) to test the three-way interactions by means of a F-test in the context of multiple linear regression for the moderator variables domain identification and math anxiety. A power analysis for the three-way interaction of moderator variable domain identification (R2change = .05, retrieved from Steinberg et al., Citation2012) showed that 152 students were required, whereas a power analysis for the three-way interaction of moderator variable math anxiety (η2partial = .02 retrieved from Delgado & Prieto, Citation2008) showed that 387 students were required. Taking the nested data into account, we found the need for a maximum of 1316 students (i.e. 387 students times 3.4). Because we planned to sample schools until we acquired 946 girls in our sample, we expected to end up with a total sample size larger than 1316. This guaranteed adequate power for the tests of the three-way interaction for variables domain identification and math anxiety. For the variable gender identification, we could not find a useful effect size estimate of the three-way interaction in the literature, which rendered a well-informed power analysis problematic. We assumed the effect size of the three-way interaction for gender identification to not be much smaller than the three-way interactions of domain identification and math anxiety, which meant the power of this particular test would be sufficient with a sample consisting of 946 girls and a similar number of boys. Taken together, this made our registered study the largest gender stereotype threat experiment in class settings to date.
Handling missing data
As pre-registered, missing data were handled as follows. First, we removed participants list-wise who quit the experiment partway through because those missing values do not give us any information about the mathematics ability of the participants. Second, we wanted to mirror a regular testing session, thus if a participant failed to fill in a (few) item(s) on the mathematics test those items would be classified as a wrong answer for that participant. Participants who skipped more than 30% of the mathematics test were removed list-wise. If we encountered missing values on the covariates, we removed participants from the analyses of that particular moderating variable. Moreover, we anticipated three circumstances in which data from specific classes would be worthless. First, we planned to drop classes in which the students were making noise during test administration, based on an assessment that the majority of students in a class were talking for more than 2 min during test administration. Second, we planned to drop classes in which more than 50% of the students failed to complete the entire set of materials, because either the material was too difficult for this class or the students collectively failed to make a serious effort to complete the materials. Third, we planned not to take data into account of students who entered the class more than 5 min late because they then would need to rush through the material, giving them a disadvantage on the mathematics test.
Handling outliers and sensitivity analyses
We planned to carry out a set of sensitivity analyses to be included in Appendix A. First, we checked for robustness by removing outliers based on the median absolute deviation (MAD)–median rule (Wilcox, Citation2011). We subtracted the median score of all observations, to obtain the median of those new scores (MAD). The MADN was then calculated by dividing the MAD by 0.6745. An observation then was flagged as an outlier if it exceeded the following cutoff rule:
Observations flagged as outliers were removed from the data set only for the sensitivity analyses. Because all of our important variables are based on sum scores of scales, we did not anticipate many outliers (Bakker & Wicherts, Citation2014). In our second set of registered sensitivity analyses aimed at checking for robustness, we removed all participants who incorrectly answered the manipulation check and/or the read check, and reanalyzed the remaining data.
Results
Participants
Data were gathered between 30 September 2016 and 28 March 2017 at 21 Dutch high schools. The data were from 86 classes and included a total of 2126 students, typically aged either 13 or 14 (M = 13.39, SD = 0.62). Due to a low response rate at the level of schools (16.67% of the original sample of schools participated), we deviated from our registered sampling strategy and collected a convenience sample. The schools we visited were situated in the provinces of Zuid-Holland (4 schools), Noord-Brabant (12 schools), Utrecht (3 schools), Gelderland (1 school), and Overijssel (1 school). We visited 35 VWO classes (the highest level of education in the Netherlands), 41 HAVO classes, and 10 HAVO/VWO mixed classes. Gathering of the data took 6 months instead of the planned 3 months. These changes in sampling strategy were needed to obtain a sufficiently large data set. Changes were discussed and approved by the editor of CRSP. In the Discussion section, we will consider how these alterations in design could have influenced the results.
As decided a priori, we removed students having more than 30% missing data on the math test. This left us with data from N = 2067 students. Three more students were removed because they did not mark their gender, so our final data set consisted of N = 2064 students. Because students were usually quiet during test administration and classes were never late, we did not need to remove entire classes. Some classes were somewhat noisy or appeared less concentrated, and some students appeared not to take the study seriously by looks of their booklets (e.g. showing very clear aberrant answering patterns on the math test like aaaaa9aaaaaaaaaaaaaa, or making remarks in the comment section that implied they did not take the test seriously). In the section Exploratory analyses, we report results after removing data from these students and classes.
Descriptives
For boys and girls in both conditions, provides the means, standard deviations, and sample sizes for the main dependent variable guess corrected math performance, and for sum scores on the moderators math anxiety (scale ranging from 10 to 50), domain identification (scale ranging from 12 to 60) and gender identification (scale ranging from 4 to 20). Moreover, this table includes the number correct, the number of items unanswered on the math test, and accuracy score (the number correct divided by the number attempted) to give a complete overview of math test performance. Note that scores on the Math Anxiety Scale were low on average and positively skewed. Scores on the domain identification scale were below the midpoint of the scale as well However, the large-scale TIMSS 2003 survey showed that such scores below the midpoints of the relevant scales are also common for Dutch students in TIMMS (Martin, Gonzalez, & Chrostowski, Citation2003). As such, low scores on the current domain identification scale are not out of the ordinary. Table B1 in the Online Supplemental Material reports the proportions of gender stereotypes held by boys and girls, pooled over experimental conditions. For boys, the option “boys are better” was most popular, but the proportions for “girls are better” and “equally good” were selected almost as often. For girls, the most popular statement was “equally good” closely followed by “girls are better”, whereas a much smaller group of girls selected “boys are better”. Cronbach’s α for all scales and the math test are reported in , together with effect size Cohen’s d to illustrate differences between groups.
Table 3. Averages and standard deviations for math performance (scored in several ways), missing values, math anxiety scale, domain identification, and gender identification.
Table 4. Cohen’s d, Cronbach’s α, greatest lower bound, skewness, and kurtosis.
Reliabilities for the scales were acceptable (gender identification) to high (domain identification, math anxiety). The lower reliability estimate of the scale gender identification is probably due to the (short) length of the scale. Moreover, a considerable number of students indicated that they found the gender identification scale somewhat confusing, so we will be cautious with the interpretation of results with this scale. In the Appendix, we fitted a graded response model to the three psychological scales to assess the psychometric qualities of those scales in more detail. Reliability of the math test might be compromised due to the relative homogeneity of the sample (as we tried to select a group of highly identified students).Footnote5
Pre-registered analyses
Manipulation check
Overall, 91% of the students answered the read check correctly (“In what year was this mathematics test studied before?”), indicating that a large majority of the students read the introduction to the math test. Moreover, 84% of all students answered the manipulation check correctly (“Did boys and girls perform equally on the math test?”). The option “yes, there were differences between boys and girls” was selected more often by students in the ST condition (N = 834) than students in the control condition (N = 41), and the option “no, there were no differences between boys and girls” was selected more often by students in the control condition (N = 898) than students in the ST condition (N = 72, χ2(1) = 1418.4, p < .001; students who answered “Don’t know” (N = 205) or failed to answer this question (N = 14) were excluded from this analysis). In the section Sensitivity analyses, we consider the influence on our main results after removing students who incorrectly answered the read check and/or the manipulation check.
Frequentist approach
A first analysis showed that there are significant differences between classes in guess corrected math performance (F(85, 1978) = 6.847, p < .001). Because of these differences (and following our pre-registration), we used multilevel analysis instead of a standard 2 × 2 ANOVA.
We carried out a sequential multilevel regression analysis, in which we added (clusters of) variables in a stepwise fashion. The model that includes all variables equals the model we pre-registered. The results are given in . The random intercept model highlights considerable variation due to differences between classes, with a sizable ICC coefficient of . Adding gender as a predictor variable resulted in a better model compared to the random intercept model, pointing to a significant gender gap with boys outscoring girls. Adding the main effect of stereotype threat (Model 2), the interaction effect of gender and stereotype threat (Model 3), and the class-level variables gender of the present teacher and proportion of boys in the classroom (Model 4) did not result in a significant improvement in model fit. Fit criteria AIC and BIC were lowest for Model 2, thereby confirming that the model with only gender showed the best fit.
Table 5. Main analyses: fit measures, deviance, unstandardized regression coefficients, and variance components for models without moderators.
To see whether students performed differently on the difficult or easy items, we ran the same models using the (guess corrected) easiest 10 items, and the most difficult 10 items (guess corrected). We observed the same pattern of results when we solely analyzed the easy items, and when we solely analyzed the difficult items, i.e. Model 2 showed the best fit. The results of these analyses can be found in Table B2 in the Online Supplemental Material.
Bayesian approach
We calculated default Bayes factors to quantify the evidence for the four competing hypotheses in . Parameters were estimated in R package “lme4”, taking the multilevel structure of the data into account. No other variables were included in this model. The default Bayes factors were calculated using software package BaIn (Gu et al., Citation2018), and they are reported in . Note that BaIn provides Bayes factors for each of the four hypotheses against an unconstrained (reference) hypothesis, denoted by Hu. Subsequently using the transitivity property of the Bayes factor, these Bayes factors were used to compute the Bayes factors between the key hypotheses H0, H1, H2, and Hc. We found most evidence for the specified null hypothesis H0 that a stereotype threat does not exist. Comparing H0 to the competing hypotheses H1, H2, and Hc showed clear support for the former hypothesis. There is strong evidence for H0 (i.e. the null hypothesis of no threat effect) against H1 (i.e. the stereotype threat hypothesis) and very strong evidence for H0 against H2 (i.e. the stereotype threat and stereotype lift hypothesis) and for H0 against Hc (i.e. the complement hypothesis). Assuming equal prior probabilities for the hypotheses (i.e. hypotheses are equally likely a priori), we calculated posterior probabilities: P(H0|x) = .963, P(H1|x) = .034, P(H2|x) = .001, and P(Hc|x) = .002, which can be interpreted as the probabilities that a hypothesis is true after observing the data. Similarly, as with the Bayes factors, the posterior probabilities show strong evidence in favor of the null hypothesis of no stereotype threat effect in these data.
Table 6. Bayes factors for competing hypotheses.
Moderators
For all three moderators (math anxiety, domain identification, and gender identification), we carried out a series of multilevel analyses, starting with a simple random intercept model, to which we added the following terms in a stepwise fashion: (Model 1) the moderating variable, (Model 2) gender, (Model 3) experimental condition, (Model 4) two-way interaction effect ST × Gender, (Model 5) three-way interaction ST × Gender × Moderator, including all possible two-way interactions, (Model 6) gender of the teacher and proportion of girls in the classroom. provides model comparison and fit indices.
Table 7. Main analyses: fit statistics and model comparison for moderating variables and stereotype threat.
shows that adding math anxiety to the model improved fit. Subsequently adding gender to the model improved fit as well. Adding more variables such as the experimental condition or the interactions did not improve fit. In , we report regression parameters for the best fitting model per moderator variable. We still see a negative effect of gender, indicating that (controlled for math anxiety) girls performed worse on the math test than boys, and a negative linear effect of math anxiety indicating that (controlled for gender) higher scores on math anxiety were associated with lower scores on the math test. The same pattern emerged for domain identification; adding domain identification to the random intercept improved fit, and subsequently adding gender to the model improved fit as well. In this model, gender continued to be a significant predictor, indicating that (controlled for domain identification) girls performed worse on the math test than boys, and a positive linear effect of domain identification indicating that (controlled for gender) higher scores on domain identification were associated with lower scores on the math test. For the variable gender identification, the pattern was different: including gender identification did not improve fit, whereas adding gender to the model did increase model fit.
Table 8. Unstandardized regression coefficients for models with moderators estimated with ML.
Because none of the interaction effects of the moderators with the experimental condition and gender were significant, this concludes the main analyses as we described them in our pre-registration. Under the section Exploratory analyses, we present a final model in which we included math anxiety, domain identification, and gender and their interaction terms as predictor variables. To ensure valid inferences from this model, we checked and reported results on model assumptions as described by Snijders and Bosker (Citation2012) which can be found in the Online Supplemental Material.
Sensitivity analyses
In the first round of sensitivity analyses, we removed all students who either answered the read check or the manipulation check incorrectly. In total, 1596 students remained in this analysis. We re-analyzed the main analyses (i.e. fitting the four models to test the overall effect of ST with all items analyzed), and the three moderator analyses. The results of the main analysis were unchanged in this sensitivity analysis. Specifically, we still found a gender gap-favoring males, and Model 2 turned out to fit the data the best. Results of this sensitivity analysis using this adjusted data set corroborated results from the regular moderator analyses for all three moderators (tables with model comparison statistics are included in the Online Supplemental Material). For the second set of sensitivity analyses, we calculated outlying scores for all the scales we used as moderator variables (i.e. math anxiety, domain identification, and gender identification) according to the MAD–Median rule as we pre-specified in the Methods section. We repeated the moderator analyses without outlying scores on that particular moderator. Again, those analyses corroborated the results from the main analyses (tables with model comparison statistics are included in the Online Supplemental Material).
In registered reports, researchers make decisions regarding the analyses a priori, but unanticipated issues might emerge during the study. We explored the influence of several variables we did not include in our pre-registration, and provide most of these results in the Online Supplemental Material. Including these variables or altering variables (e.g. education level, type of class, presence of the teacher, different scoring of the domain identification scale, different scoring rules for the math test, linear effect of time) did not yield novel important insights. Unsurprisingly, we found that education level of the class predicted math performance. Since these analyses capitalize on chance, their results do not carry the same weight as those from the confirmatory analyses. We do believe these analyses are useful to demonstrate the robustness of the results. We shared all used scripts on OSF (https://osf.io/yt83j/).Footnote6 We included three exploratory analyses in this paper that are in our opinion a valuable complement to our main analyses.
Exploratory analyses
To create a final model, we used math anxiety, domain identification, and gender as predictor variables. To obtain the final model, we included math anxiety and domain identification (Model 1), gender (Model 2), the two-way interactions Gender × Math anxiety, Gender × Domain identification and Math anxiety × Domain identification (Model 3), and finally a three-way interaction between the three predictors (Model 4). Model 1 predicted significantly better than the null model p < .001), whereas Model 2 outperformed Model 1
p < .001) and Model 3 outperformed Model 2
p = .034). Model 4 did not predict better than Model 3. We report the regression coefficients for Model 3 in . Model 3 highlighted interaction effects of gender and domain identification, math anxiety, and domain identification. The positive effect of domain identification on math performance turned out to be stronger for girls than for boys. The positive effect of domain identification on math performance was strongest for students who scored lowly on math anxiety (e.g. −1 SD), and least strong for students who scored highly on math anxiety (e.g. +1 SD).
Table 9. Final model: unstandardized regression coefficients and variance components for final model.
In a second exploratory analysis, we reran the analyses for a subset of highly math-identified students (N = 872). Students were marked as highly math identified when they obtained a sum score higher than 36 on the domain identification scale (consisting of 12 items). Again, adding the main effect of gender to the model resulted in a significant effect p < .001), whereas adding the main effect of ST and the Gender × ST interaction did not result in a significant improvement of the model
p = .876).Footnote7
Finally, we included a third exploratory analysis in which we ran the model again for a subset of students whose parents were both born in the Netherlands (N = 1788). Rerunning the models in this subset of students gave similar results as for the main analysis with all students included: adding the main effect of gender to the model resulted in a significant effect p < .001), whereas adding the main effect of ST and the Gender × ST interaction did not result in a significant improvement of the model
p = .568). This indicates that the absence of evidence for the stereotype threat effect is unlikely to be due to negative stereotypes related to minority status.
Discussion
In this high-powered stereotype threat study, we investigated whether a common stereotype threat manipulation influenced the mathematical test performance of girls and boys in Dutch high schools. Through a series of analyses, we conclude that our data show no evidence of performance decrements due to the stereotype threat manipulation. A series of sensitivity analyses supports the robustness of our findings. Based on the default Bayes factors we conclude that there is strong evidence in favor of the null hypothesis of no stereotype threat when compared to the stereotype threat hypothesis, the stereotype threat/stereotype lift hypothesis, and the complement hypothesis. We found sizeable variation in performance between classes, partly due to the fact that we tested classes from the highest educational level (VWO), the second highest educational level (HAVO), and mixed educational levels (HAVO/VWO). Furthermore, we found that variables domain identification and math anxiety were all significant predictors of math ability. Additionally, we found a gender gap on the on math test, with boys outperforming girls. A final exploratory model described the interaction effects between the three predictors. Because we did not preregister this model, and the model was not the main focus of this paper (i.e. studying stereotype threat effects), we refrain from discussing it in more detail. Although individual differences in domain identification, math anxiety, and gender identification were expected by theory to affect susceptibility to stereotype threat, we failed to find evidence that these variables moderated stereotype threat effects in the current data.
There are several potential explanations for the lack of a stereotype threat effect in our sample. We now discuss several potential explanations for this, based on whether effects generalize over units (participants), treatment variations, outcome measures, and settings (e.g. Shadish, Cook, & Campbell, Citation2002).
First, our current sample of high school students might not be representative of the wider population of high-performing high school students in the Netherlands. Because circumstances forced us to use convenience sampling instead of random sampling, our sample might not be completely representative of the population of students we wanted to study (we defined our original population as all HAVO/VWO students from schools with mixed HAVO/VWO classes in the provinces Utrecht, Zuid-Holland, and Noord-Brabant). For instance, 11 of the schools were situated in villages, and only 10 were situated in (overall small- to medium-sized) cities. Because large cities are underrepresented in our sample, and schools situated in cities probably educate students with more diverse (ethnic) backgrounds, this might have led to selection bias. However, in gender stereotype threat studies, students from a minority background are often removed from the analyses, using the argument that the gender gap in mathematics appears only for Caucasian students (e.g. Johns et al., Citation2005). If anything, the lack of diversity should boost a stereotype threat effect instead of suppressing it. We sampled from a range of schools from different parts of the country. Given the relative homogeneity of quality and curricula across schools in the Netherlands, we used a reasonably broad sample that does attest to the generalizability of the stereotype threat effect across the Netherlands. With an exploratory analysis, we did check whether the stereotype threat effect appeared when we solely analyzed a subset of students whose parents were both born in the Netherlands. The results for this exploratory analysis were similar to the main results, so we are confident that the stereotype threat effect was not suppressed by other negative stereotypes related to country of origin.
Second, it is possible that the students in our sample lack characteristics that are needed for stereotype threat to occur, including the belief in gender stereotypes or identification with the math domain. It might be that a large share of students in our sample did not believe the stereotype that boys are typically better in mathematics than girls. When we inquired whether boys or girls usually performed better on math tasks, only a small portion of the girls answered that boys appeared to be better. However, re-analyzing the data for girls who believed that boys usually outperform girls did not change the results. Moreover, past research showed that even in the absence of explicit stereotypical beliefs amongst 13-year-old students, stereotype threat effects can be found (Muzzatti & Agnoli, Citation2007). Steele (Citation1997) remarked that students do not need to believe the stereotype themselves for stereotype threat to occur. Additionally, although we selected high-performing high school students, not all students might have been highly identified with the math domain. Yet, when we added a three-way interaction (Gender × Stereotype threat × Domain identification), we found no evidence for a stronger stereotype threat effect for students that scored higher on the domain identification scale. Moreover, re-analyzing a subset of students that were highly math identified did not result in a stereotype threat effect either.
Third, our chosen manipulation of stereotype threat could have been ineffective. However, we used a manipulation that had been commonly (and successfully) used in previous stereotype threat studies (e.g. Keller & Dauenheimer, Citation2003; Picho & Stephens, Citation2012; Spencer et al., Citation1999). Our manipulation check showed that most students read and remembered the description of the math test, and when we removed students that answered the manipulation check incorrectly the results did not change substantively. As such, we have little reason to doubt the effectiveness of the manipulation.
Fourth, there might be issues with the outcome measure used in our study. It could be that the selected math test did not elicit any threat, for instance because the wrong types of items were used or because the test was too easy. However, we selected math items from TIMSS 2003, which is a math test that has been used before in stereotype threat testing in which stereotype threat effects were found (Keller, Citation2007a; Keller & Dauenheimer, Citation2003). We carefully selected a set of geometry items on purpose because women tend to underperform in this topic. Group averages of the items answered correctly ranged between 57% (for girls in the stereotype threat condition) and 64% (for boys in the control condition), which admittedly is not the most difficult test, but does reflect a realistic testing situation. Moreover, we did not find a stereotype threat effect when we re-analyzed the data with a subtest of the 10 most difficult items. With item analysis, Item Response Theory Modeling and Differential Item Functioning analyses we could describe the influence of stereotype manipulation on an item level in more detail, but these analytic techniques are beyond the scope of this paper (see Flore (Citation2018) for an elaborate psychometric analysis on stereotype threat data). Finally, reliability of the math test was somewhat low, which might be caused by the relative homogeneity of the sample (as we tried to select a group of highly identified students). Controlling for disattenuation did not change our conclusions with regard to the stereotype threat effect (see footnote 5).
Fifth, the setting could have been insufficiently threatening for stereotype threat effects to occur, while the control condition might not have been sufficiently safe (i.e. devoid of threat) for girls to perform well. Specifically, if stereotype threat is not sufficiently removed in the control condition, no differences in math performance between the stereotype threat condition and the control condition are expected because both groups will experience threat (Spencer et al., Citation2016). To avoid this problem, we selected a control condition in which we clearly presented the mathematics test as gender fair: a safe condition that has been successfully implemented in the past (Good, Aronson, & Harder, Citation2008; Keller, Citation2007a; Keller & Dauenheimer, Citation2003). We note that our manipulation check provided reassurance that most students in the control condition recalled the test as gender fair, which should have successfully alleviated the effects of negative gender stereotypes.
Furthermore, there is a possibility that students did not feel motivated to perform well on the math test, because the stakes were not high enough for the students. Because the math test was not graded as part of the regular curriculum, students might not have tried as hard as they would on a regular math exam. Even though this explanation might sound plausible, experimental stereotype threat studies are rarely carried out in high-stakes environments because of ethical implications and practical constraints (Sackett, Citation2003). A handful of studies tried to study effects of stereotype threat in a high-stakes testing context by placing a fairly subtle manipulation before taking actual placement tests (Stricker & Ward, Citation2004), or by offering financial rewards for correctly answered items (Fryer, Levitt, & List, Citation2008). In those studies, stereotype threat effects were absent or negligible. Some authors argued that stereotype threat effects did not occur in those settings, or the effects in those settings were not as large compared to lab studies, because it is (theoretically) impossible to create a stereotype threat safe condition on high-stakes tests. This might have caused all girls to underperform, regardless of condition (Aronson & Dee, Citation2012; Spencer et al., Citation2016; Steele, Spencer, & Aronson, Citation2002). Other authors responded it is just as plausible that women in stereotype threat conditions might be less motivated to perform well on a low stakes test, whereas they are able to overcome this motivational effect on high-stakes tests (Sackett & Ryan, Citation2012). Because high-stakes tests have not shown convincing stereotype threat effects, and a substantial number of low stakes test did yield evidence for stereotype threat effects, we are not convinced that the lack of a stereotype threat effect in our current study is caused by the absence of high stakes attached to test performance.
Finally, it might be possible that the stereotype threat manipulation simply does not influence Dutch children. Even though stereotype threat effects have been found among Dutch college students (Marx et al., Citation2005; Wicherts, Dolan, & Hessen, Citation2005) and among students aged 12–16 in Italy, France, Uganda, Spain, and Germany (Delgado & Prieto, Citation2008; Huguet & Régner, Citation2007, Citation2009; Keller & Dauenheimer, Citation2003; Muzzatti & Agnoli, Citation2007; Picho & Stephens, Citation2012), there is a possibility that our studied population is not sufficiently affected by stereotype threat. For the discrepancy with past results, we can think of potential cross-cultural explanations (i.e. in Dutch society this gender stereotype has little influence on test performance), statistical explanations (i.e. a Type II error occurring), generational explanations (i.e. this generation of students is no longer sensitive to stereotype threat) or other yet unknown theoretical explanations that should be tested in later meta-analyses and randomized experiments. Post hoc, it is difficult to judge which explanation is the right one. We are convinced that we carried out a powerful and well-designed experiment. Our experiment mirrors many of the past stereotype threat studies with positive results in terms of setting, type of test, and stereotype threat manipulation, and our study is clearly superior to those earlier studies in terms of statistical power.
Our findings are not surprising given diverging results of earlier studies of stereotype threat in classroom settings. Results of past studies have been heterogeneous (see Flore & Wicherts, Citation2015 for an overview), with some studies finding large effects for specific groups (e.g. Muzzatti & Agnoli, Citation2007) and others finding no stereotype threat effect at all (e.g. Cherney & Campbell, Citation2011; Ganley et al., Citation2013). Because the divergence in earlier findings is not readily explainable in terms of theoretically driven moderators, but does match the pattern expected from publication bias in meta-analyses (Flore & Wicherts, Citation2015), several authors have suggested that publication bias and other related biases affect the literature on stereotype threat (Flore & Wicherts, Citation2015; Ganley et al., Citation2013; Stoet & Geary, Citation2012). Because of the severity of biases due to the flexibility in analyzing relatively small experiments (e.g. see Bakker, van Dijk, & Wicherts, Citation2012) and a common failure to report at least some experimental results, meta-analyses based on currently available stereotype threat studies fail to paint an accurate picture of the generalizability of stereotype threat among girls.
Now that we have a rich theoretical background of stereotype threat (Inzlicht & Schmader, Citation2012; Schmader, Johns, & Forbes, Citation2008; Spencer et al., Citation2016), it might be time to rigorously study effects of stereotype threat in future confirmatory studies. Direct replications in several contexts, with proper prior power analysis and a pre-registered methods section and analyses specified in advance, will give us a better understanding of the actual influence of stereotype threat on math performance. With registered reports and other pre-registered studies, we can systematically answer questions concerning the boundary conditions of stereotype threat: for what type of students do stereotype threat effects emerge, in which cultures, in which age groups, and on what topics do the effects occur? Once the boundary conditions in those studies are clear (e.g. if only extremely high domain identified women underperform on extremely difficult tests), we might wonder whether gender stereotype threat is as important as previously claimed, and reconsider whether we should implement general interventions to counter it (Jordan & Lovett, Citation2007; Walton, Spencer, & Erman, Citation2013). Either way, the current large-scale study does show that the effects of stereotype threat on math test performance should not be overgeneralized.
With this study, we started an effort to testing stereotype threat effects in a confirmatory fashion using a meticulous design. Other efforts to improve the replicability of stereotype threat studies, like high powered studies (Smeding, Dumas, Loose, & Régner, Citation2013; Stricker & Ward, Citation2004), additional pre-registered replication studies (Finnigan & Corker, Citation2016; Gibson et al., Citation2014; Moon & Roeder, Citation2014) are now starting to appear. We hope this trend will continue in the future, and might extend to other exciting formats like adversarial collaborations to replicate some of the original stereotype threat findings. Not only are collaborations useful to design studies with combined input of researchers with different kinds of expertise, they additionally simplify the work because multiple parties need to gather data, sharing the burden of acquiring a large sample. The advantages of large multi-lab (replication) studies are numerous: results are often more robust than results from a small study, power to find a significant stereotype threat is higher, and generalizability of stereotype threat effects across labs and cultures can be studied systematically. Such efforts shed light on the nature of stereotype threat and can help ameliorate its potential effects on women’s academic performance in fields in which they are still faced with negative stereotypes.
Supplemental Material
Download Zip (60.4 KB)Acknowledgments
We thank Robbie van Aert, Marcel van Assen, Hilde Augusteijn, Marjan Bakker, Chris Hartgerink, Michèle Nuijten, Pieter Oosterwijk, and Coosje Veldkamp for their valuable comments on an earlier draft of this paper. Additionally, we would like to thank all high school teachers and students involved in this study for their collaboration. Finally, we are indebted to Charlotte, Chris, Eda, Iris, Marion, Marloes, Maud, Myrthe, Pleun, Sofie, Tara, Tessa, and Zhané for their invaluable help in collecting the data.
Supplementary material
Supplemental data for this article can be accessed here.
Additional information
Funding
Notes
1. This was the case for the majority of classrooms. We encountered one classroom solely consisting of girls.
2. Although some studies suggest that math performance of women will deteriorate to a stronger degree when male experiment leaders run the study (Marx & Roman, Citation2002), a recent meta-analysis showed that differences in effect sizes between studies run by female experiment leaders and studies run by male experiment leaders are negligible (Doyle & Voyer, Citation2016). Based on this finding, we felt confident to have our study run by a female experiment leader.
3. First, for the manipulation in the pilot we used the sentence “The most recent study carried out in 2012 showed that boys and girls do not perform equally on this mathematics test”. To ensure the children read the manipulation carefully, we altered the manipulation for the main study to the sentence mentioned in the section Procedure. Second, we originally planned 25 min for the mathematics test, but most children were finished before 20 min were up, and started to become restless. Therefore, we changed the amount of time for the mathematics test to 20 min. Third, we used a test anxiety scale (Arvey, Strickland, Drauden, & Martin, Citation1990) in our pilot as potential moderator, but replaced it with a Math Anxiety Scale for the main study (Prieto & Delgado, Citation2007).
4. Walton and Cohen (Citation2003) observed that members of positively stereotyped groups performed slightly better on the stereotype relevant task when confronted with negative stereotypes about an out-group, a phenomenon they named stereotype lift.
5. We can calculate a disattenuated effect size taking this low reliability estimate of the test into account (Hedges & Olkin, Citation1985), comparing math performance of girls in the stereotype threat condition to performance of girls in the control condition. This would lead to a disattenuated stereotype threat effect size of . This does not change our conclusion that the stereotype threat effect in our sample is very small.
6. Because of privacy issues, we were not allowed to publish the full data. The data set is stored on DataverseNL. Researchers can request our data set through DataverseNL. We provided more information on the data-sharing procedure in the document “Data sharing” on OSF (https://osf.io/yt83j/).
7. Using higher cutoff criteria of 42 and 48 to create the subset led to similar results.
References
- Agnoli, F., Altoè, G., & Muzzatti, B. (n.d.). Unpublished study.
- Ambady, N., Shih, M., Kim, A., & Pittinsky, T. L. (2001). Stereotype susceptibility in children: Effects of identity activation on quantitative performance. Psychological Science, 12(5), 385–390.
- Aronson, J., & Dee, T. (2012). Stereotype threat in the real world. In M. Inzlicht & T. Schmader (Eds.), Stereotype threat. Theory, process, and application (pp. 264–280). New York, NY: Oxford University Press.
- Arvey, R. D., Strickland, W., Drauden, G., & Martin, C. (1990). Motivational components of test taking. Personnel Psychology, 43(4), 695–716.
- Asendorpf, J. B., Conner, M., De Fruyt, F., De Houwer, J., Denissen, J. J. A., Fiedler, K., … Wicherts, J. M. (2013). Recommendations for increasing replicability in psychology. European Journal of Personality, 27(2), 108–119.
- Bagès, C., & Martinot, D. (2011). What is the best model for girls and boys faced with a standardized mathematics evaluation situation: A hardworking role model or a gifted role model? British Journal of Social Psychology, 50(3), 536–543.
- Bakker, M., van Dijk, A., & Wicherts, J. M. (2012). The rules of the game called psychological science. Perspectives on Psychological Science, 7(6), 543–554. doi:10.1177/1745691612459060
- Bakker, M., & Wicherts, J. M. (2014). Outlier removal, sum scores, and the inflation of the type I error rate in independent samplest tests: The power of alternatives and recommendations. Psychological Methods, 19(3), 409–427.
- Beilock, S. L., & Decaro, M. S. (2007). From poor performance to success under stress: Working memory, strategy selection, and mathematical problem solving under pressure. Journal of Experimental Psychology. Learning, Memory, and Cognition, 33(6), 983–998.
- Beilock, S. L., Rydell, R. J., & McConnell, A. R. (2007). Stereotype threat and working memory: Mechanisms, alleviation, and spillover. Journal of Experimental Psychology: General, 136(2), 256–276.
- Ben-Zeev, T., Fein, S., & Inzlicht, M. (2005). Arousal and stereotype threat. Journal of Experimental Social Psychology, 41(2), 174–181.
- Berger, J. O., & Pericchi, L. R. (1996). The intrinisic Bayes factor for model selection and prediction. Journal of the American Statistical Association, 91(433), 109–122.
- Cadinu, M., Maass, A., Frigerio, S., Impagliazzo, L., & Latinotti, S. (2003). Stereotype threat: The effect of expectancy on performance. European Journal of Social Psychology, 33(2), 267–285.
- Cherney, I. D., & Campbell, K. L. (2011). A league of their own: Do single-sex schools increase girls’ participation in the physical sciences? Sex Roles, 65(9–10), 712–724.
- Delgado, A. R., & Prieto, G. (2008). Stereotype threat as validity threat: The anxiety–Sex–Threat interaction. Intelligence, 36(6), 635–640.
- Désert, M., Préaux, M., & Jund, R. (2009). So young and already victims of stereotype threat: Socio-economic status and performance of 6 to 9 years old children on Raven’ s progressive matrices. European Journal of Psychology of Education, 24(2), 207–218.
- Doyle, R. A., & Voyer, D. (2016). Stereotype manipulation effects on math and spatial test performance: A meta-analysis. Learning and Individual Differences, 47, 103–116.
- Educational Testing Service. (2016). GRE: Guide to the use of scores. Retrieved from www.ets.org/gre/guide
- Else-Quest, N. M., Hyde, J. S., & Linn, M. C. (2010). Cross-national patterns of gender differences in mathematics: A meta-analysis. Psychological Bulletin, 136(1), 103–127.
- Eriksson, K., & Lindholm, T. (2007). Making gender matter: The role of gender-based expectancies and gender identification on women’s and men’s math performance in Sweden. Scandinavian Journal of Psychology, 48(4), 329–338.
- Finnigan, K. M., & Corker, K. S. (2016). Do performance avoidance goals moderate the effect of different types of stereotype threat on women’s math performance? Journal of Research in Personality, 63, 36–43.
- Flore, P. C. (2018). The psychometrics of stereotype threat (Doctoral dissertation). Retrieved from https://pure.uvt.nl/ws/portalfiles/portal/23445144/Flore_Stereotype_7_3_2018.pdf
- Flore, P. C., & Wicherts, J. M. (2015). Does stereotype threat influence performance of girls in stereotyped domains? A meta-analysis. Journal of School Psychology, 53(1), 25–44.
- Ford, T. E., Ferguson, M. A., Brooks, J. L., & Hagadone, K. M. (2004). Coping sense of humor reduces effects of stereotype threat on women’s math performance. Personality & Social Psychology Bulletin, 30(5), 643–653.
- Frary, R. B. (1988). Formula scoring of multiple‐choice tests (correction for guessing). Educational Measurement: Issues and Practice. 7(2), 33–38.
- Fryer, R. G., Levitt, S. D., & List, J. A. (2008). Exploring the impact of financial incentives on stereotype threat : Evidence from a pilot study. American Economic Review: Papers & Proceedings, 98(2), 370–375.
- Ganley, C. M., Mingle, L. A., Ryan, A. M., Ryan, K., Vasilyeva, M., & Perry, M. (2013). An examination of stereotype threat effects on girls’ mathematics performance. Developmental Psychology, 49(10), 1886–1897.
- Giallousis, N. (2015). Scale: Likert type questionnaire item analysis. R package version 1.0.4. Retrieved from https://cran.r-project.org/package=Scale
- Gibson, C. E., Losee, J., & Vitiello, C. (2014). A replication attempt of stereotype susceptibility (Shih, Pittinsky, & Ambady, 1999): Identity salience and shifts in quantitative performance. Social Psychology, 45(3), 194–198.
- Good, C., Aronson, J., & Harder, J. A. (2008). Problems in the pipeline: Stereotype threat and women’s achievement in high-level math courses. Journal of Applied Developmental Psychology, 29(1), 17–28.
- Gu, X., Mulder, J., Deković, M., & Hoijtink, H. (2014). Bayesian evaluation of inequality constrained hypotheses. Psychological Methods, 19(4), 511–527.
- Gu, X., Mulder, J., & Hoijtink, H. (2018). Approximated adjusted fractional Bayes factors: A general method for testing informative hypotheses. British Journal of Mathematical and Statistical Psychology, 71(2), 229–261.
- Hedges, L. V., & Olkin, I. (1985). Parametric estimation of effect size from a series of experiments. In L. V. Hedges & I. Olkin (Eds.), Statistical methofs for meta-analysis (pp. 108–148). San Diego, CA: Academic Press.
- Huguet, P., & Régner, I. (2007). Stereotype threat among schoolgirls in quasi-ordinary classroom circumstances. Journal of Educational Psychology, 99(3), 545–560.
- Huguet, P., & Régner, I. (2009). Counter-stereotypic beliefs in math do not protect school girls from stereotype threat. Journal of Experimental Social Psychology, 45(4), 1024–1027.
- Inzlicht, M., & Schmader, T. (2012). Stereotype threat. (M. Inzlicht & T. Schmader, Eds.). New York, NY: Oxford University Press.
- Inzlicht, M., & Ben-Zeev, T. (2000). A threatening intellectual environment: Why females are susceptible toexperiencing problem-solving deficits in the presence of males. Psychological Science, 11(5), 365–371.
- Jeffreys, K. (1961). Theory of probability (3rd ed.). New York, NY: Oxford University Press.
- Johns, M., Schmader, T., & Martens, A. (2005). Knowing is half the battle: Teaching stereotype threat as a means of improving women’s math performance. Psychological Science, 16(3), 175–179.
- Jordan, A. H., & Lovett, B. J. (2007). Stereotype threat and test performance: A primer for school psychologists. Journal of School Psychology, 45(1), 45–59.
- Kass, R. E., & Raftery, A. E. (1995). Bayes factors. Journal of the American Statistical Association, 90(430), 773–795.
- Keller, J. (2007a). Stereotype threat in classroom settings: The interactive effect of domain identification, task difficulty and stereotype threat on female students’ maths performance. British Journal of Educational Psychology, 77(2), 323–338.
- Keller, J. (2007b). When negative stereotypic expectancies turn into challenge or threat: The moderating role of regulatory focus. Swiss Journal of Psychology, 66(3), 163–168.
- Keller, J., & Bless, H. (2008). When positive and negative expectancies disrupt performance: Regulatory focus as a catalyst. European Journal of Social Psychology, 38, 187–212.
- Keller, J., & Dauenheimer, D. (2003). Stereotype threat in the classroom: Dejection mediates the disrupting threat effect on women’s math performance. Personality & Social Psychology Bulletin, 29(3), 371–381.
- Kerr, N. L. (1998). HARKing: Hypothesizing after the results are known. Personality and Social Psychology Review, 2(3), 196–217.
- Kiefer, A. K., & Sekaquaptewa, D. (2007). Implicit stereotypes, gender identification, and math-related outcomes: A prospective study of female college students. Psychological Science, 18(1), 13–18.
- Lamont, R. A., Swift, H. J., & Abrams, D. (2015). A review and meta-analysis of age-based stereotype threat: Negative stereotypes, not facts, do the damage. Psychology and Aging, 30(1), 180–193.
- Lesko, A. C., & Corpus, J. H. (2006). Discounting the difficult: How high math-identified women respond to stereotype threat. Sex Roles, 54(1–2), 113–125.
- Marchand, G. C., & Taasoobshirazi, G. (2013). Stereotype threat and women’s performance in physics. International Journal of Science Education, 35(18), 3050–3061.
- Martin, M. O., Gonzalez, E. J., & Chrostowski, S. J. (2003). TIMSS 2003 international mathematics report. Boston: TIMSS & PIRLS International Study Center, Boston College.
- Martin, M. O., Mullis, I. V. S., Gonzalez, E. J., & Chrostowski, S. J. (2004). TIMSS 2003 international science report. Boston: TIMSS & PIRLS International Study Center, Boston College.
- Marx, D. M., & Roman, J. S. (2002). Female role models: Protecting women’s math test performance. Personality and Social Psychology Bulletin, 28(9), 1183–1193.
- Marx, D. M., Stapel, D. A., & Muller, D. (2005). We can do it: The interplay of construal orientation and social comparisons under threat. Journal of Personality and Social Psychology, 88(3), 432–446.
- Miller, D. I., Eagly, A. H., & Linn, M. C. (2015). Women’s representation in science predicts national gender-science stereotypes: Evidence from 66 nations. Journal of Educational Psychology, 107(3), 631–644.
- Moon, A., & Roeder, S. S. (2014). A secondary replication attempt of stereotype susceptibility (Shih, Pittinsky, & Ambady, 1999). Social Psychology, 45(3), 199–201.
- Mulder, J. (2014). Prior adjusted default Bayes factors for testing (in)equality constrained hypotheses. Computational Statistics & Data Analysis, 71, 448–463.
- Muzzatti, B., & Agnoli, F. (2007). Gender and mathematics: Attitudes and stereotype threat susceptibility in Italian children. Developmental Psychology, 43(3), 747–759.
- Neuburger, S., Jansen, P., Heil, M., & Quaiser-Pohl, C. (2012). A threat in the classroom. Gender stereotype activation and mental-rotation performance in elementary-school children. Zeitschrift Für Psychologie, 220(2), 61–69.
- Neuville, E., & Croizet, J. C. (2007). Can salience of gender identity impair math performance among 7–8 years old girls? The moderating role of task difficulty. European Journal of Psychology of Education, 22(3), 307–316.
- Nguyen, H. H. D., & Ryan, A. M. (2008). Does stereotype threat affect test performance of minorities and women? A meta-analysis of experimental evidence. The Journal of Applied Psychology, 93(6), 1314–1334.
- O’Brien, L. T., & Crandall, C. S. (2003). Stereotype threat and arousal: Effects on women’s math performance. Personality and Social Psychology Bulletin, 29(6), 782–789.
- O’Hagan, A. (1995). Fractional Bayes factors for model comparison. Journal of the Royal Statistical Society: Series B (Methodological), 57(1), 99–138.
- Osborne, J. W. (2001). Testing stereotype threat: Does anxiety explain race and sex differences in achievement? Contemporary Educational Psychology, 26(3), 291–310.
- Osborne, J. W. (2007). Linking stereotype threat and anxiety. Educational Psychology, 27(1), 135–154.
- Picho, K., Rodriguez, A., & Finnie, L. (2013). Exploring the moderating role of context on the mathematics performance of females under stereotype threat : A meta-analysis. The Journal of Social Psychology, 153(3), 299–333.
- Picho, K., & Stephens, J. M. (2012). Culture, context and stereotype threat: A comparative analysis of young Ugandan women in coed and single-sex schools. The Journal of Educational Research, 105(1), 52–63.
- Prieto, G., & Delgado, A. R. (2007). Measuring math anxiety (in Spanish) with the rasch rating scale model. Journal of Applied Measurement, 8(2), 149–160.
- Sackett, P. R. (2003). Stereotype threat in applied selection settings : A commentary stereotype. Human Performance, 16(3), 295–309.
- Sackett, P. R., & Ryan, A. M. (2012). Concerns about generalizing stereotype threat research findings to operational high-stakes testing. In M. Inzlicht & T. Schmader (Eds.), Stereotype threat (pp. 249–263). New York, NY: Oxford University Press.
- Schmader, T. (2002). Gender identification moderates stereotype threat effects on women’s math performance. Journal of Experimental Social Psychology, 38(2), 194–201.
- Schmader, T., & Johns, M. (2003). Converging evidence that stereotype threat reduces working memory capacity. Journal of Personality and Social Psychology, 85(3), 440–452.
- Schmader, T., Johns, M., & Forbes, C. (2008). An integrated process model of stereotype threat effects on performance. Psychological Review, 115(2), 336–356.
- Sekaquaptewa, D., & Thompson, M. (2003). Solo status, stereotype threat, and performance expectancies: Their effects on women’s performance. Journal of Experimental Social Psychology, 39(1), 68–74.
- Shadish, W. R., Cook, T. D., & Campbell, D. T. (2002). Construct validity and external validity. In Experimental and quasi-experimental designs for generalized causal inference (pp. 64–102). Boston: Houghton Mifflin Company.
- Smeding, A., Dumas, F., Loose, F., & Régner, I. (2013). Order of administration of math and verbal tests : An ecological intervention to reduce stereotype threat on girls ’ math performance. Journal of Educational Psychology, 105(3), 850–860.
- Smith, J. L., & White, P. H. (2002). An examination of implicitly activated, explicitly activated, and nullified stereotypes on mathematical performance: It’s not just a woman’s issue. Sex Roles, 47(3–4), 179–191.
- Snijders, T. A., & Bosker, R. J. (2012). Assumptions of the hierarchical linear model. In T. A. Snijders & R. J. Bosker (Eds.), Multilevel analysis. An introduction to basic and advanced multilevel modeling (pp. 152–173). London: Sage Publications.
- Spencer, B., & Castano, E. (2007). Social class is dead. Long live social class! Stereotype threat among low socioeconomic status individuals. Social Justice Research, 20(4), 418–432.
- Spencer, S. J., Logel, C., & Davies, P. G. (2016). Stereotype threat. Annual Review of Psychology, 67(1), 415–437.
- Spencer, S. J., Steele, C. M., & Quinn, D. M. (1999). Stereotype threat and women’s math performance. Journal of Experimental Social Psychology, 35(1), 4–28.
- Ståhl, T., Van Laar, C., & Ellemers, N. (2012). The role of prevention focus under stereotype threat: Initial cognitive mobilization is followed by depletion. Journal of Personality and Social Psychology, 102(6), 1239–1251.
- Steele, C. M. (1997). A threat in the air. How stereotypes shape intellectual identity and performance. American Psychologist, 52(6), 613–629.
- Steele, C. M., & Aronson, J. (1995). Stereotype threat and the intellectual test performance of African Americans. Journal of Personality and Social Psychology, 69(5), 797–811. Retrieved from http://www.ncbi.nlm.nih.gov/pubmed/7473032
- Steele, C. M., Spencer, S. J., & Aronson, J. (2002). Contending with group image: The psychology of stereotype and social identity threat. Advances in Experimental Social Psychology, 34, 379–440.
- Steinberg, J. R., Okun, M. A., & Aiken, L. S. (2012). Calculus GPA and math identification as moderators of stereotype threat in highly persistent women. Basic and Applied Social Psychology, 34(6), 534–543.
- Stoet, G., & Geary, D. C. (2012). Can stereotype threat explain the gender gap in mathematics performance and achievement? Review of General Psychology, 16(1), 93–102.
- Stricker, L. J., & Ward, W. C. (2004). Stereotype threat, inquiring about test takers’ ethnicity and gender, and standardized test performance. Journal of Applied Social Psychology, 34(4), 665–693.
- Titze, C., Jansen, P., & Heil, M. (2010). Mental rotation performance in fourth graders: No effects of gender beliefs (yet?). Learning and Individual Differences, 20(5), 459–463.
- Wagenmakers, E. J., Wetzels, R., Borsboom, D., van der Maas, H. L. J., & Kievit, R. A. (2012). An agenda for purely confirmatory research. Perspectives on Psychological Science, 7(6), 632–638.
- Walton, G. M., & Cohen, G. L. (2003). Stereotype lift. Journal of Experimental Social Psychology, 39(5), 456–467.
- Walton, G. M., & Spencer, S. J. (2009). Latent ability: Grades and test scores systematically underestimate the intellectual ability of negatively stereotyped students. Psychological Science, 20(9), 1132–1139.
- Walton, G. M., Spencer, S. J., & Erman, S. (2013). Affirmative meritocracy. Social Issues and Policy Review, 7(1), 1–35.
- Wax, A. L. (2009). Stereotype threat: A case of overclaim syndrome? In C. H. Sommers (Ed.), The science on women and science (pp. 132–169). Washington, DC.: AIE Press.
- Wicherts, J. M. (2005). Stereotype threat research and the assumptions underlying analysis of covariance. The American Psychologist, 60(3), 267–269.
- Wicherts, J. M., Dolan, C. V., & Hessen, D. J. (2005). Stereotype threat and group differences in test performance: A question of measurement invariance. Journal of Personality and Social Psychology, 89(5), 696–716.
- Wicherts, J. M., Veldkamp, C. L. S., Augusteijn, H. E. M., Bakker, M., van Aert, R. C. M., & van Assen, M. A. L. M. (2016). Degrees of freedom in planning, running, analyzing, and reporting psychological studies: A checklist to avoid p-hacking. Frontiers in Psychology, 7, 1–12.
- Wilcox, R. (2011). Modern statistics for the social and behavioral sciences: A practical introduction. Boca Raton: CRC press.
- Willse, J. T. (2014). CTT: Classical test theory functions. R package version 2.1. Retrieved from http://cran.r-project.org/package=CTT
- Wout, D., Danso, H., Jackson, J., & Spencer, S. J. (2008). The many faces of stereotype threat: Group- and self-threat. Journal of Experimental Social Psychology, 44(3), 792–799.
- Yeung, N. C. J., & von Hippel, C. (2008). Stereotype threat increases the likelihood that female drivers in a simulator run over jaywalkers. Accident Analysis & Prevention, 40(2), 667–674.