ABSTRACT
Calcium phosphates (CaPs) are extensively used as biomaterials for bone repair and regeneration and are considered an interesting synthetic alternative for natural bone grafts. However, the bone regenerative potential of many CaP bone graft substitutes requires further improvements. The bioactivity of CaPs is regulated by a variety of properties, including their chemical composition and (surface) structural properties. In this study, we performed a comprehensive characterization of six CaP ceramics that had been previously tested in several in vitro and in vivo studies, allowing us to in silico distinguish osteoinductive and non-osteoinductive CaP ceramics. The numerical parameters obtained from this characterization were further correlated with osteogenesis-associated gene expression and pathways of osteoblastic MG63 cells cultured on these six CaP ceramics. The results showed, among others, the influence of both chemical and structural parameters on cytoskeleton- and extracellular matrix-related gene expression and osteogenesis-associated pathways such as MAPK and BMP. The bioinformatics approach presented here allows exploring the transcriptional landscape induced by CaP ceramics and gaining fundamental knowledge about cell-biomaterial interactions.
1. Introduction
Calcium phosphate (CaP)-based biomaterials are widely used in orthopedic, craniomaxillofacial, and dental surgery for the repair and regeneration of bone defects. Despite significant improvements over the past decades and examples of CaP bone graft substitutes with an in vivo performance comparable to that of autologous bone (Yuan et al., Citation2010), the gold standard in bone regeneration, synthetic bone graft substitutes are in general still considered inferior to the natural bone grafts. The advantages of their use are, however, evident: they are relatively inexpensive, easy to produce in virtually unlimited amounts, and can be stored for long periods. Moreover, with bone graft substitutes, complications associated with autograft harvesting and transplantation can be avoided. In view of an increasing demand for successful treatment options for challenging bone defects (Gillman & Jayasuriya, Citation2021; Govoni et al., Citation2021), it is important to further improve the clinical performance of synthetic bone graft substitutes.
The bioactivity of CaPs, i.e., their bone regenerative potential, is influenced by a large and complex set of physico-chemical and structural properties. These properties include the chemical composition, specifically calcium, phosphorus, and other bioinorganics (Habibovic & Barralet, Citation2011), the phase composition (Danoux, Pereira, et al., Citation2016), crystallinity (Lee, Zavgorodniy, Loo, & Rohanizadeh, Citation2012), microstructure (Deligianni, Katsala, Koutsoukos, & Missirlis, Citation2000), grain size (Lapczyna et al., Citation2014), and porosity, among others. The most direct way to assess the contribution of an individual material property to a biological response would be to produce batches of CaPs differing in just one property at a time, and then evaluate their performance either in vitro or in vivo. However, this is difficult to achieve, as the properties of CaPs are largely intertwined, i.e., a change in one of them causes changes in others, particularly when conventional synthesis and processing techniques are used (Galvan-Chacon & Habibovic, Citation2017). For example, doping a CaP with an additive, such as strontium, results in the replacement of some or all calcium ions by the strontium ion in the crystal lattice, which in turn alters the crystallinity, microstructure or solubility of the material (Birgani, Malhotra, van Blitterswijk, & Habibovic, Citation2016). It is therefore difficult to distinguish between the direct chemical effect of the added ion and the changes its addition causes in other material properties (Birgani, Citation2016).
The knowledge of how each individual material property affects the interaction of the material with a biological environment is, however, crucial for developing more efficient bone graft substitutes. A number of studies addressed this issue by developing novel methods to decouple the importance of chemistry and surface structure, such as coating methods to isolate the effect of surface (micro)structure (dos Santos, Farina, Soares, & Anselme, Citation2009), methods to replicate the surface structure of a CaP into a polymer that does not contain the ceramic (Danoux, Sun, et al., Citation2016; Groen et al., Citation2017), the controlled modification of the surface structure (Deligianni et al., Citation2000; Mazón, García-Bernal, Meseguer-Olmo, Cragnolini, & De Aza, Citation2015), or the use of composite materials containing inorganic constituents of the ceramic rather than the ceramic itself (Danoux et al., Citation2015). Although these studies have made an important contribution to the current understanding of the property-function relationships of CaPs, the methods they used are not sufficient to understand the role of all properties and neglect the relevant relationships between them (Engel et al., Citation2008).
A different approach consists of applying bioinformatics tools based on big data analysis to establish correlations between material properties and biological responses. For example, gene expression microarray technologies allow measuring thousands of protein-encoding genes at a time, providing very large and comprehensive datasets. This approach has been successfully applied on ceramic and composite materials to understand how physico-chemical stimuli influence cell behavior (Baker et al., Citation2014; Eyckmans et al., Citation2013). For example, Groen et al. linked the transcriptional profile of MG63 cells, an osteogenic cell line frequently used to assess the osteogenic potential of ceramics (Lincks et al., Citation1998), to the CaP material properties by culturing the cells on osteoinductive and non-osteoinductive ceramics, as well as replicas of the structure of those materials fabricated with different chemical compositions (Groen et al., Citation2017). The results identified genes responding to microstructure, calcium, and phosphate ion levels.
In this study, we used a big data approach to link the properties of CaP ceramics with the gene expression levels of cells cultured on them. To complement previous studies, we performed a highly comprehensive material characterization, which, combined with a machine learning approach, allowed us to study the role of individual material properties in the biological response to the materials. The six CaPs selected, comprised pairs of materials with similar starting chemical composition but sintered at different temperatures, allowing to study the effect of similar chemical properties but different microstructures. These ceramics have been used in a large number of previous in vitro and in vivo studies (Barrère-de Groot, Yuan, & de Bruijn, Citation2018; Davison, Yuan, de Bruijn, & Barrere-de Groot, Citation2012; Davison et al., Citation2014; Duan et al., Citation2017; Groen et al., Citation2017; Habibovic et al., Citation2006; Li, De Wijn, Li, Layrolle, & De Groot, Citation2003; Othman et al., Citation2019; Wang et al., Citation2015; Yuan, Yang, De Bruij, De Groot, & Zhang, Citation2001; Yuan, van Blitterswijk, de Groot, & de Bruijn, Citation2006; Yuan et al., Citation2010), facilitating additional interpretation of the results by comparing their performance with previous data.
The comprehensive characterization of the physico-chemical and microstructural properties of the six materials resulted in a total of 131 numerical parameters. The characterization techniques included X-ray diffraction analysis (XRD) with Rietveld analysis, inductively-coupled plasma mass spectrometry (ICP-MS) ion quantification to determine both the materials composition and its ions exchange dynamics with cell culture medium, scanning-electron microscopy (SEM), mercury intrusion, porosimetry, confocal laser profilometry and micro-computed tomography (microCT). Further clustering and correlation analyses were then performed using a transcriptomics dataset of MG63 cells cultured on the six materials obtained in a previous study (Groen et al., Citation2017) to identify links between materials parameters and osteogenesis-associated gene expression and pathways.
The approach presented here allows exploring the transcriptional landscape induced by ceramics, identifying which material properties are linked with particular genes or gene pathways, and represents a valuable tool for gaining fundamental knowledge about cell-biomaterial interactions in the context of bone regeneration.
2. Materials and methods
2.1. Materials characterization
2.1.1. Ceramic particles
The six CaP ceramics used in this study were supplied by Kuros Biosciences BV in the shape of particles with a size of 1–2 mm. The materials were produced with three different chemical compositions: HA, TCP, and BCP. For each chemistry, two materials were produced with different microstructure: small/large grain size, low/high microporosity, and low/high specific surface area.
2.1.2. Chemical characterization
The ceramic particles were ground in an agate mortar, reduced to fine powder, loaded into a plastic container, and analyzed using X-ray diffraction (XRD, Bruker D2 Phaser) with a step size of 2θ 0.01°, one second per step and one rotation per second, in the interval 2θ 5–80°. The data was analyzed using Profex v.4.1.0 to perform a Rietveld refinement of the crystal structure (Doebelin & Kleeberg, Citation2015). The powders were also analyzed by Fourier Transform Infrared Spectroscopy (FTIR) in the attenuated total reflection mode (ATR). The spectra were analyzed using SpectraGryph 1.2 (Spectroscopy Ninja).
For the elemental composition analysis, 20 milligrams of particles of each material were dissolved in 2 mL of 60% ultrapure nitric acid (HNO3, 60% ultrapure for trace analysis, Supelco, Merck) overnight, followed by the addition of 8 mL of 1% HNO3. Afterwards, a further 20x dilution was performed in a matrix of 1% HNO3 and gallium standard (Ga standard for ICP-MS, VWR) to achieve a final Ga concentration of 100 ppb, which was used as an internal standard for the quantification. A standard curve was prepared with 7 points using a multi-elements standard (Thermo Fisher Scientific) and Ca and P standards (standard solution, 1000 mg/L, Aristar), in order to detect concentrations of Ca and P between 0.1 ppm and 200 ppm, and the other elements contained in the Multi elements solution (Na, Mg, S, K, Fe, Ga, and Sr) in the range 0.1 to 5 ppm.
To analyse the ion exchange between the ceramics and the cell culture medium, an identical experimental approach was applied as for the transcriptomics data experiments (Groen et al., Citation2017). In brief, 2–3 particles (20 mg) were placed in triplicate per condition and time point in 48 well-plates and 0.4 ml cell culture medium (α-MEM, 10% fetal bovine serum, 0.2 mM ascorbic acid, 2 mM L-glutamine, 100 U/ml penicillin, 100 µg/ml streptomycin) was added to each well. The plates were incubated in a cell culture incubator (5% CO2, humidity control), the medium was refreshed every two days, and collected and frozen on day 2, 4, 7, and 14. Then, the samples were prepared for the analysis by dissolving 100 µL of cell culture medium in 0.9 mL 60% nitric acid (HNO3, 60% ultrapure for trace analysis, Supelco, Merck) overnight, followed by the addition of 9 mL 1% HNO3 containing Ga standard (Ga standard for ICP-MS, VWR) to obtain a final Ga concentration of 100 ppb, which was used as an internal standard in all samples. The standard 6-point curve was prepared in the same matrix as the samples and used to quantify Ca, P and Mg in the range of 0.1 ppm to 2 ppm.
2.1.3. Structural characterization
The surface roughness parameters of the particles were characterized with a confocal laser scanning optical profilometer (Keyence VKX-200, Keyence, Japan) using a 150x objective. For each material, three different areas of 6897.4 µm2 were imaged on three different particles (n = 3). The profilometry data was processed using the Keyence Multifile Analyzer software, obtaining multiple parameters recommended in ISO 25178 to quantify standard roughness (description in Supplementary Table 1). As reported previously by Groen et al. (Groen et al., Citation2017), the microstructure was also observed by an SEM (XL30, Philips) in the secondary electron mode. The grain size of the ceramics was determined by measuring the lateral distance of 60 different grains from three independent SEM images using Image J. Additionally, high-resolution SEM images were acquired (Teneo, FEI) at 5 kV voltage and 2000, 10,000, and 30,000 times magnification, using an Everhart Thornley Detector to collect secondary electrons and a Trinity Detector to collect backscattered electrons.
The ceramic particles were further analyzed by µCT (SkyScan 1272 11Mp scanner, Bruker Corporation, USA) with cone-beam geometry and a 4032x2688 CCD detector. The images were acquired using a 0.25 mm aluminum filter and software-correcting for alignment, thermal drift, beam hardening, and ring artifacts, using a voxel size of 2.253 µm3. The image dataset was reconstructed using the NRecon v.1.6.10.4 software and analyzed using CTAn (both Bruker Corporation). The VOI was carefully selected to exclude the container in which the particles were imaged. Using the 3 D analysis tool of CTAn, multiple structural properties were quantified, including the total porosity, percentage of closed pores, and trabecular thickness.
The porosity of the particles was also analyzed by mercury intrusion porosimetry (Groen et al., Citation2017). Briefly, the pore size distribution, microporosity (percentage of pores smaller than 10 μm), and specific surface area were determined using mercury intrusion porosimetry (Quantachrome Instruments, Pore Master). Additional parameters concerning the shape of the pore distribution curve were quantified, and a fit to a pseudo-Voigt curve (convolution of a Lorentzian distribution and a Gaussian distribution) was calculated. Density measurements were performed to determine the true (skeletal) density of the materials using a helium pyconometer (Accu Pyc II 1340 gas pyconometer, Micrometrics) and analyzed with Pore Master software.
2.2. Cell culture and transcriptomics analysis
The dataset describing the transcriptomic profile of MG63 cells cultured on the different ceramics was obtained by Groen et al. in a previous study (Groen et al., Citation2017). Briefly, human osteosarcoma MG63 cells were seeded at a density of 200,000 cells in wells containing 150 µL particles, and cultured for 48 hours, using cells cultured on tissue culture polystyrene as a control. After cell culture, the total RNA was isolated, cDNA was synthesized, and hybridized on a microarray using Illumina HT-12 v4 expression Beadchips.Streptavidin Cy-3 was added to develop a fluorescent signal. The arrays were scanned using an Illumina microarray reader, and the raw intensity values were corrected for background using BeadStudio (Illumina).
2.3. Materials properties dataset processing and PCA analysis
The original dataset containing all materials parameters obtained from the materials characterization had dimensions of 6x161, where 6 is the number of different materials and 161 is the number of material parameters. Statistically significant differences between material parameters were established using a one-way ANOVA. An overview of statistical differences between material parameters can be found in the open access data repository. Material parameters that represented technical variations were excluded from further processing. Parameters that showed a strong correlation (>0.99) with another parameter were further excluded from the dataset. Incomplete material parameters (e.g., missing values) were also removed from the dataset. The final dataset obtained had dimensions of 6x76. PCA analysis was performed using R version 4.03. The hierarchical cluster plot was calculated in R version 4.03 by the complete linkage method and a Euclidian distance metric on the scaled data. Data visualization was achieved through Graphpad Prism version 9.3.1.
2.4. Gene dataset processing and material-gene correlation analysis
Heat map clustering of gene expression levels across the different materials was accomplished by selecting the top 500 genes demonstrating the highest variability across the materials. Variability is defined as the difference between a given value and the mean value. Processing and visualization were accomplished in R version 4.03. Visualization of the heat map was further processed using Graphpad Prism version 9.3.1.
For the gene-material correlation analysis, we used the same final dataset used for the PCA analysis. We further included genes belonging to the top 20% with the highest variability across the materials. Spearman correlation between genes and material properties were computed in R version 4.03 and visualized through R package ggplot version 3.1.1. Genes that demonstrated a correlation higher than 0.7 or lower than −0.7 and a p-value lower than 0.05 were considered significant. Gene ontology (GO) pathway analysis of each significant gene was performed in R version 4.03.
3. Results and discussion
3.1. Materials characterization
The main objective of the comprehensive materials characterization was to obtain a large set of numerical parameters describing the six CaP ceramics (HA1150, HA1250, TCP1050, TCP1100, BCP1150, BCP1300). The ceramics can be grouped into three categories based on their chemical composition (HAs, TCP1050, and BCPs) and per chemical composition category into two groups with different structural and topographical properties, resulting from differences in sintering conditions. Finally, the ceramics can be grouped into an osteoinductive (HA1150, TCP1050, BCP1150) and non-osteoinductive (HA1250, TCP1100, BCP1300) category, as shown in previous studies (Davison et al., Citation2014; Maazouz, Chizzola, Dobelin, & Bohner, Citation2021; Yuan et al., Citation2006; Citation2010). The complete list of parameters and a description of the least known ones is provided in Supplementary Table 1.
3.1.1. Chemical characterization
XRD analysis was performed to describe the crystalline composition and structure of the CaP ceramics. shows the XRD patterns of the ceramic powders, indicating the peaks corresponding to each of the phases present in the CaP materials, namely HA, β-TCP, and periclase. The Rietveld analysis details are provided in Supplementary Figure 1. For all conditions, the χ2 parameter, which quantifies the difference between the experimental data and data predicted by the model, was below the acceptance limit defined as χ2 < 1.5 (the closer the χ2 value is to 1, the better the fit). The results of the analysis are shown in and represented in . During the data processing, we observed that the refinement did not give results below the acceptance limit, i.e., the model could not adequately describe the pattern, unless the possibility of Ca substitution by magnesium (Mg) was introduced into the code for refinement. The percentage of Mg substitution was calculated as the average for both CaP phases, i.e., over the total number of atoms present in the molecule without impurities. Mg is commonly introduced in the preparation of CaP, as it stabilizes the β-TCP phase and avoids the formation of α-TCP (Gibson & Bonfield, Citation2002; Kannan, Ventura, & Ferreira, Citation2007).
Figure 1. XRD analysis and Rietveld refinement. a) XRD patterns of the six CaP ceramics, with the peaks corresponding to the three main phases (HA, β-TCP, Periclase) indicated by arrows of different colors. b) Phase composition quantified by Rietveld refinement demonstrates the chemical composition differences between HA, TCP and BCP ceramics. c) Magnesium substitution percentage in the crystal lattice, obtained by Rietveld analysis. The error bars represent the estimated standard deviation in the quantification given by the model.
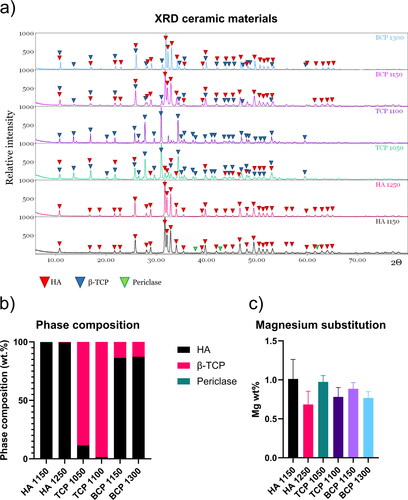
Table 1. Phase composition of the different materials.
As expected, the two HA ceramics were largely phase-pure, with small percentages of periclase (Mg oxide) due to the presence of Mg and a small percentage of β-TCP in HA1250. TCP1050 contained 11.5 wt.% HA, whereas in TCP1100, the HA percentage was about 1.5 wt.%, due to the higher sintering temperature. Both biphasic ceramics contained about 87 wt.% HA, 13 wt.% TCP, and a negligible amount of periclase (Sheikh et al., Citation2015; Tamimi et al., Citation2012).
Other parameters obtained from the refinement and included in the model were the lattice parameters, the crystallite size, and the microstrain.
ICP-MS analysis was applied to quantify the proportion of the different elements (Ca, P and Mg) in the ceramic particles upon complete dissolution. Moreover, ICP-MS analysis of the cell culture medium was performed upon immersion of the ceramic particles for 2, 4, 7, and 14 days in the cell culture medium. The quantification of the Ca and P content in the particles () and the resulting Ca/P and (Ca + Mg)/P atomic ratio () showed that the materials were not completely stoichiometric. Our results for the (Ca + Mg)/P ratio are in accordance with those obtained by Maazouz et al. in a recent study in which the same materials were analyzed (Maazouz et al., Citation2021). The ICP-MS results confirmed the presence of Mg in the CaPs in similar amounts as suggested by the XRD analysis ().
Figure 2. ICP-MS analysis on CaP ceramics and culture medium. a–e) Ca, P, and Mg ion content of the six CaP ceramics. f–h) Ca, P, and Mg ion content of the cell culture medium. The error bars represent the standard deviation of 3 independent measurements.
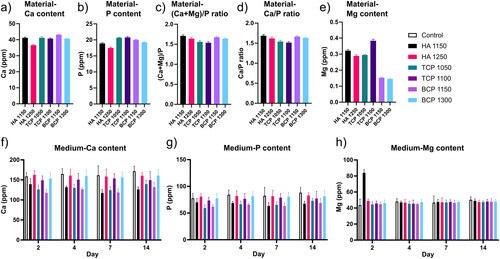
The elemental analysis of the cell culture medium upon ceramic immersion () showed significant differences between the control, i.e., cell culture medium not containing a material, and the different materials, as well as among the different ceramics. Specifically, within the category of the ceramics with similar chemistry, those sintered at lower temperature had lower Ca levels in the culture media than the chemically similar material sintered at higher temperatures and even lower than in the control well (). A similar trend was observed for P, although in this case, the effect was less profound (). It was observed that the uptake of Ca and P was higher in the materials sintered at lower temperatures than in chemically similar materials sintered at higher temperatures. This uptake of Ca and P was probably due to a higher porosity/smaller grain size obtained at lower sintering temperatures, which translated into a higher specific surface area.
Various CaP ceramics have been reported to decrease the Ca content of the cell culture medium, which itself is saturated towards HA (Birgani et al., Citation2016; Othman et al., Citation2019). The observation was hypothesized to be either due to the precipitation of a new CaP layer on the surface of the ceramic, or due to the formation of calcium carbonate or calcium hydroxide (Maazouz et al., Citation2020; Citation2021).
Regarding the Mg content of the cell culture medium, the results suggested a burst release of Mg from HA1150, with a concentration at day 2 being about twice as high as that of the control and other ceramics (). HA1150 was the ceramic with the highest percentage of periclase among the six tested materials. No significant differences between the control and the other five ceramics were observed in terms of Mg content of the medium at any time point.
3.1.2. Structural characterization
Besides chemical composition, structural properties, including surface topography and properties of porous structure, have been shown to affect the biological response to CaP ceramics. First, SEM imaging was used to obtain qualitative information about the CaP surface topography and structure, including pores, grains, and other features. The SEM micrographs () exhibited differences in surface microstructure among the different ceramics. The materials sintered at lower temperatures (left column) contained more micropores and were comprised of smaller grain, while the materials sintered at higher temperatures (right column) had scarce microporosity and larger grain size.
Figure 3. Structural analysis of CaP ceramics. a) High-resolution SEM micrographs illustrating the difference between osteoinductive (left column) and non-osteoinductive ceramics (right column). b) Pore size distribution obtained using mercury intrusion porosimetry analysis. Osteoinductive materials demonstrate to contain pores of multiple sizes. Pores were not detected for the non-osteoinductive materials except for TCP1100, which contains pores of a larger size. c) Grain size quantification demostrates low values for osteoinductive and high values for non-osteoinductive ceramics. d) Microporosity across the ceramics. e–j) Selected profilometric parameters demonstrate a larger diversity across the ceramics. k–m) Selected microCT parameters allowing a description of the 3D structures across the ceramics. The error bars represent the standard deviation of 3 independent measurements.
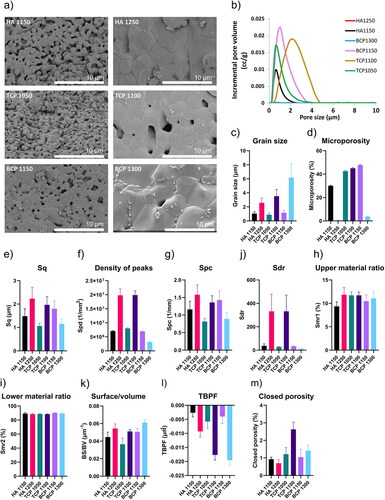
We confirmed these visual observations through mercury intrusion porosimetry, which allowed quantifying the pore size distribution (). The grain size quantification of the materials further confirmed the observations and showed that BCP1300 had the largest grain size among the six ceramics, with the lowest values found for HA1150, TCP1050, and BCP1150 (). The pore size distribution curves () obtained from mercury intrusion porosimetry were also distinct for the different ceramics. It is important to emphasize that the mercury intrusion porosimetry quantification only included pores with sizes below 15 µm, and therefore the total porosity of the ceramics is plausibly higher than the microporosity values (). Taken together, these results showed different degrees of porosity among different ceramics, with HA1250 almost devoid of pores and BCP1300 having very low porosity. It is also important to note that the difference in porosity between TCP1050 and TCP1100, although statistically significant, was smaller than was observed for the HA and BCP pairs. Importantly, the ceramics with lower porosity are the non-osteoinductive ones, and porosity has been reported to play an essential role in the osteoinductive potential of CaP ceramics (Bohner & Miron, Citation2019).
Next, we performed confocal profilometry to quantify the ceramic surface properties. The 3D height maps again exhibited differences in microstructural features depending predominantly on the sintering temperature (Supplementary Figure 3). The root mean square height (Sq) (), a parameter frequently used to quantify surface roughness (Semnani, Citation2017; Webb et al., Citation2012), was higher for the non-osteoinductive ceramics, expect for the BCP ceramic, with Sq being highest for BCP1150. This is an interesting observation considering that roughness is generally considered to improve an osteogenic phenotype (Faia-Torres et al., Citation2014). A similar trend was observed for the peak density parameter (Spd), a parameter that represents the number of peak features per mm2 (). The arithmetic mean curvature (Spc) is a feature that represents the arithmetic mean of the principal curvature of the peaks of the surface, i.e., a low Spc indicates that the points of contact of the surface have rounded shapes, while higher values mean that they have pointed shapes. Spc values also followed a similar trend as the Sq parameter (). Also, the developed interfacial area ratio (Sdr) was significant different between TCP1150 and BCP1300, and BCP1150 and BCP1300, with large yet non-significant higher levels detected for HA1250 and TCP1100 (). The upper material ratio (Smr1), which physically represents the areal material ratio that divides the areas that would be removed by initial abrasion with another surface from the core surface of the material (Supplementary Figure 4), was only significantly different between HA1150 and the two TCP ceramics (). The lower material ratio (Smr2), which represents the percentage of the measurement area that contains valley structures able to hold liquid on the surface and improve lubricity (Supplementary Figure 4), was significantly different between BCP1150 and the two TCP ceramics ().
MicroCT images also provided information about the surface topography and structure. In addition, since microCT is a 3D imaging technique, it allows the quantification of parameters such as the pore interconnectivity and the number of closed pores. Due to the resolution of the acquisition, 2.253 µm3 voxel size, the microCT results are complementary to those from the mercury porosimetry, as they predominantly cover larger pores and 3D macrostructure. The surface-to-volume ratio of the non-osteoinductive ceramics was higher than their osteoinductive counterparts (). While this result may be considered counterintuitive, as it is to be expected that materials with higher porosity have a higher surface-to-volume ratio, this is probably due to a relatively low resolution of the technique, not taking into account the pores smaller than 2.253 µm3. Of interest, the trabecular bone pattern factor (TBPF), a measure of bone microarchitecture, also allowed distinguishing osteoinductive and non-osteoinductive ceramics and was highest for HA1150 and lowest for BCP1300 (). The percentage of closed porosity, i.e., pores without connection to the exterior of the ceramic particles, was relatively low for all ceramics (). It is noticeable that the least osteoinductive material of the set, TCP1100, exhibited the highest percentage of closed porosity. This is relevant because the presence of surface pores has been recently suggested to be a key parameter for CaP-induced osteoinduction in vivo (Bohner & Miron, Citation2019). To conclude, these methods provided a detailed material properties characterization of each ceramic.
3.2. Correlations among material properties
After establishing an extensive dataset describing different properties of the six ceramics, we first aimed to investigate which of the material parameters are correlated. For this, Spearman correlations analysis was performed and plotted in a heatmap ().
Figure 4. Heat map showing Spearman correlations among material properties of the CaP ceramics. Positive correlations between material parameters are marked in blue, while negative correlations are marked in red.
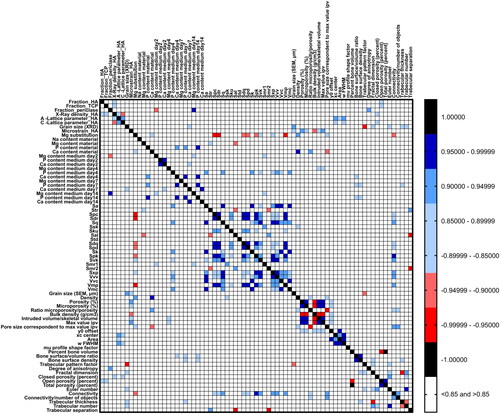
The Mg substitution percentage showed correlations with seven surface topographical parameters, namely the arithmetic mean peak curvature, the developed interfacial area ratio, the root mean square gradient, the density of peaks, the reduced peak and valley height, and the peak material volume. This is an interesting finding, as it is well known that adding bioinorganics to the CaP lattice can significantly affect the ceramic microstructure (Birgani et al., Citation2016; Farzadi, Bakhshi, Solati-Hashjin, Asadi-Eydivand, & Osman, Citation2014; Tahmasebi Birgani et al., Citation2016). Specifically, Mg substitution has been shown to change the size and morphology of CaP nanoparticles (Batra, Kapoor, & Sharma, Citation2013; Nabiyouni, Ren, & Bhaduri, Citation2015) and the crystallinity of amorphous CaP powders (Mehrjoo et al., Citation2015). Here, Mg substitution percentage showed a strong negative correlation with Spc and Sdr (r = −0.95), indicating that a higher Mg substitution was associated with more pointed shapes and a smaller surface area. The specific correlation graphs are depicted in Supplementary Figure 5A. Of interest, the addition of Mg to CaPs can also lead to the formation of periclase, which, based on our analysis, correlated with the Mg release from the ceramic, negatively at day 2 and positively at day 14. Furthermore, it was observed that the fraction of periclase positively correlated with the degree of anisotropy of the material, suggesting that the presence of periclase leads to a more anisotropic material, perhaps due to an anisotropic spatial distribution of the phases.
We also observed a strong correlation between the levels of Ca and P in the medium (Supplementary Figure 5B), which was already evident in , showing a similar trend for the content of both elements, depending on the ceramic type tested.
The grain size was shown to be inversely correlated with the trabecular pattern factor, which describes the concavity and convexity of the material, and is related to the connectivity of the material (Supplementary Figure 5C) (Hahn, Vogel, Pompesius-Kempa, & Delling, Citation1992).
A number of other correlations was observed between porosity, profilometry, and microCT parameters. It is plausible that due to the definitions of these parameters and their scale of quantification (i.e. submicrometer-scale in porosimetry and profilometry, micrometer and milimiter-scale in microCT), they actually may describe the same structural features.
3.3. PCA and hierarchical cluster analysis of material properties
We further wanted to establish if the extensive material characterization is sufficient for distinguishing the ceramic materials from each other. For this, we employed a principal component analysis (PCA). To reduce the dimensionality of the data and improve the accuracy of the algorithm, we removed material parameters that represented technical variations and those that showed a strong correlation (>0.99) with other parameters. The final dataset included 76 material properties. Of interest, the PCA plot shows grouping of the materials, with on the one side the osteoinductive ceramics HA1150, BCP1100, and TCP1050, and on the other side the non-osteoinductive ceramics BCP1300, HA1250, and TCP1100 (). Here, the non-osteoinductive ceramics had more distinct properties than the osteoinductive ceramics as demonstrated by their larger distance between them. The data demonstrates that the physico-chemical set of properties we investigated allows separating ceramics that are osteoinductive and non-osteoinductive.
Figure 5. Characterization of material parameters allows identifying common characteristics in the ceramic materials. a) PCA plot of the six CaP ceramics based on the quantified materials parameters. The osteoinductive materials BCP1150, HA1150, and TCP1050 cluster together on the left side of the plot. The non-osteoinductive ceramics appear to be more distinct between each other. b) Hierarchical cluster analysis of the material properties illustrates common and distinct material characteristics across the materials. Cluster four, containing parameters of grain size and Ca/P/Mg content in the medium, allows for distinguishing osteoinductive and non-osteoinductive ceramics.
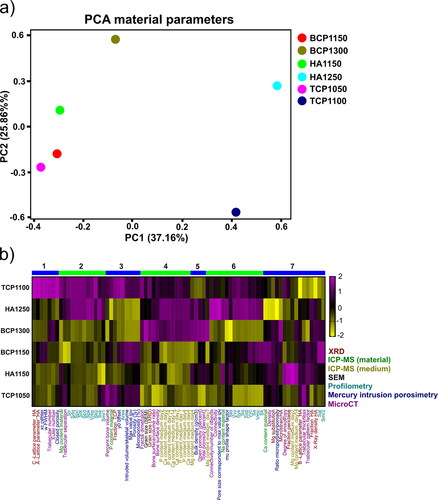
Next, we wanted to investigate which properties are responsible for this separation by employing a hierarchical cluster analysis (). Through this visualization, it becomes clear that cluster 4 allows distinguishing the osteoinductive and non-osteoinductive ceramics. This cluster contains the grain size, bone surface/volume ratio, and the Ca/P/Mg concentration in the medium, confirming the observations shown in and . Of interest, also in cluster 2 a separation between the osteoinductive and non-osteoinductive ceramics is possible except for BCP1300, which followed a similar pattern as BCP1150, HA1150, and TCP1050 concerning the surface roughness parameters.
Among the three osteoinductive materials, HA1150 appears to be the most distinct regarding its physico-chemical properties, as seen from the PCA plot. The difference between BCP1150 and TCP1050 is derived from both the structural and chemical parameters. HA1150 possesses a lower porosity (30%) than TCP1050 (44.6%) and BCP1150 (48.4%) as shown in and cluster 3. The release of Mg was also higher for HA1150, as shown in , especially on day 2, and contained more periclase (cluster 7). Looking at its performance in vivo, HA 1150 induced less de novo bone formation upon implantation in the femoral muscle of dogs, and the onset of osteoinduction occurred later relative to BCP1150 (Yuan et al., Citation2006). These results further support the proposition that the properties of the ceramics indeed impact their osteoinductive potential.
BCP1150 and BCP1300, despite having a similar chemical composition (), appeared on the opposite sides of the PCA plot. The properties that make them different are both structural and chemical concerning the ion exchange with the culture media (cluster 4). Their porosity and microporosity differ strongly, with over a 10-fold difference, as is shown in e and cluster 3, and is noticeable in the SEM images (). Moreover, the two ceramics differed in their grain sizes () and bulk densities. In an earlier in vivo study, BCP1150 had superior bone formation properties compared to BCP1050 (Habibovic et al., Citation2006), which the authors suggested to be due to the microstructure difference between the two ceramics, possibly coupled to the ions exchange dynamics differences on the surface.
3.4. Correlations between material properties and gene expression
We further aimed to correlate material properties with gene expression levels of MG63 cells cultured on these ceramics. This will allow us to investigate which genes or pathways associated with osteogenesis are activated due to individual material parameters. To demonstrate that the ceramics induce unique gene expression profiles, we performed a hierarchical cluster analysis of the 500 most variable genes between the ceramics (). Four main clusters allowed separating the gene expression profiles across the ceramics. The largest differences were observed between BCP1150 and HA1250. Of interest, TCP1050 and TCP1100, despite being different in their properties and differing significantly in their osteoinductive potential, appeared close in the clustering analysis, suggesting that their influence is reflected by the differential expression of a relatively small number of genes. The two HAs, HA1150 and HA1250, are close in the cluster hierarchy, although in this case, the number of genes with similar expression levels are smaller than TCP1050 and TCP1100. BCP1150 and BCP1300 induced the largest difference in gene expression of the ceramics with similar chemical composition.
Figure 6. CaP ceramics induce distinct gene expression profiles in MG63 cells and allow a correlation analysis with material properties. a) Hierarchical cluster analysis of the top 500 genes with strongest gene expression variation across the six CaP ceramics. Ceramics with identical chemical compositions cluster together except for the BCP ceramics. b) Heatmap showing the frequency of significant correlations between CaP ceramic properties and pathways associated with osteogenesis.
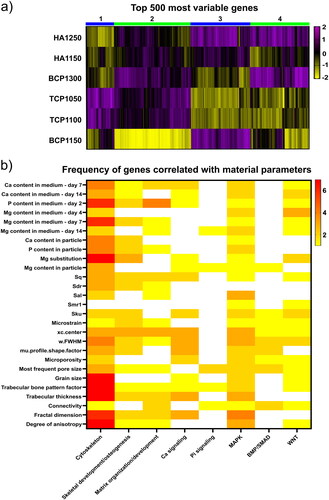
Next, we correlated the material properties with gene expression of each ceramic through Spearman correlations. The genes with a p-value lower than 0.05 and a correlation value higher than 0.7 or lower than −0.7 were grouped into eight categories based on Gene Ontology (GO) terms associated with osteogenic signaling pathways (Vermeulen, Tahmasebi Birgani, & Habibovic, Citation2022). Such an approach was previously employed to elucidate associations between surface topographical structures and gene expression levels in hMSCs (Vasilevich et al., Citation2020). The GO categories included the cytoskeleton, skeletal development and osteogenesis, matrix organization and development, calcium signaling, phosphate signaling, the mitogen-activated protein kinase (MAPK) pathway, the BMP pathway, and the WNT pathway. Next, we plotted in a heatmap the number of genes from each category correlated to an individual material property of which the variability between the ceramics was sufficient to allow correlations with gene expression levels ().
The cytoskeleton-related genes had numerous correlations with structural parameters identified using the microCT analysis. These parameters include the degree of anisotropy of the material, the fractal dimension, the trabecular thickness, and the trabecular bone pattern factor. The degree of anisotropy accounts for the existence of preferential alignment along a spatial axis in the material and is negatively correlated with the expression of genes related to the cytoskeleton (Supplementary Figure 6a). This suggests that cells on isotropic surfaces have higher cytoskeleton-related gene expression levels compared to anisotropic surfaces. Previous research with micro-topographical surfaces that induced cell morphologies with a high level of anisotropy similarly associated this observation with a decreased expression of cytoskeleton-related genes (Vermeulen et al., Citation2020). Also the fractal dimension, a quantification of an object’s surface complexity through surface features that are repeated over different spatial scales, had negative correlation coefficients with cytoskeleton gene expression (Supplementary Figure 6b).
The correlations with the trabecular pattern factor and the trabecular thickness (Supplementary Figure 6c, d) indicated that cytoskeleton-related gene expression was enhanced on structures with thicker and more interconnected trabeculae. In addition, the ceramic grain size appeared to be correlated to the cytoskeleton-related genes (Supplementary Figure 6e), although both positive and negative correlations were observed. Previous literature has shown that smaller CaP grain sizes led to a better attachment of fibroblasts and osteoblasts to the materials surfaces (Dasgupta, Tarafder, Bandyopadhyay, & Bose, Citation2013; Veljović et al., Citation2012). The porosity parameters also showed numerous correlations with cytoskeleton genes (Supplementary Figure 6f–i), which is not surprising since porosity, pore size, and pore distribution influence cell attachment and morphology (Deligianni et al., Citation2000; Guadarrama Bello, Fouillen, Badia, & Nanci, Citation2017). Several roughness parameters, the root mean square height, the developed interfacial area ratio, the autocorrelation length, and the kurtosis, exhibited correlations with the cytoskeleton genes (Supplementary Figure 6k–n), which was expected as roughness can affect cytoskeleton formation (Anselme & Bigerelle, Citation2011; Faia-Torres et al., Citation2014; Linez-Bataillon, Monchau, Bigerelle, & Hildebrand, Citation2002). Finally, Ca, P, and Mg ion ceramic content and levels in the cell culture medium also influenced cytoskeleton formation, a relationship that has been widely reported (Bennett & Weeds, Citation1986; Murakami et al., Citation2010; Rosado & Sage, Citation2000).
The genes related to skeletal development and osteogenesis showed correlations with both chemical and structural parameters (Supplementary Figure 7). The composition of the ceramic and the inorganic content of the cell culture medium have been reported to influence osteogenesis processes (Habibovic & Barralet, Citation2011; Landi et al., Citation2008; Otsuka et al., Citation2008). Related to this is also the microstrain, influenced by the Mg substitution, which determines the stability of the crystalline phase, and therefore indirectly influences the inorganic content of the medium. Besides these chemical parameters, correlations for genes related to osteogenesis were found for microstructure parameters such as root mean square height (Sq) and pore size distribution parameters, which was also reported before (Dalby et al., Citation2007; Wilkinson et al., Citation2011).
The set of genes related to matrix organization and development was predominantly correlated with structural parameters (Supplementary Figure 8). To the best of our knowledge, the effects of trabecular thickness, fractal dimension connections, and connectivity on the expression of matrix-related genes have not been studied directly yet. However, it is plausible that these structural dimensions change cellular morphology, influencing the expression of matrix-related genes (Le et al., Citation2017). The correlation with Sq was expected as surface roughness is known to influence matrix development (Cai et al., Citation2020).
The Ca signaling gene set had a small number of correlations with the Ca levels in the cell culture medium (Supplementary Figure 9). In contrast, the Ca signaling was correlated mainly with structural parameters. As discussed, this may be an indirect effect, as (surface) structural properties largely determine the available surface area for interaction with the biological environment, which in turn affect the ions exchange dynamics with the environment, including the exchange of Ca. Furthermore, since cell morphology can influence Ca signaling (Ribeiro, Reece, & Putney, Citation1997), also here, a connection can be made with structural parameters that influence cell shape.
Only a few genes were found to correlate with the phosphate signaling, mainly affected again by the pore size (Supplementary Figure 10). For example, both Mg levels and pore connectivity showed correlations with the xenotropic and polytropic virus receptor 1 (XPR1), which encodes a protein involved in phosphate homeostasis by mediating the phosphate export from cells (Chande & Bergwitz, Citation2018; Mailer et al., Citation2021).
The MAPK pathway affects genes related to a variety of cellular processes, such as proliferation, differentiation, stress response and apoptosis, which can be modulated by the materials properties. It is further related to the phosphorylation and activation of RUNX2 through various signaling pathways such as collagen-integrin binding or BMP signaling (Franceschi & Xiao, Citation2003). According to our results, MAPK showed abundant correlations with structural parameters (Supplementary Figure 11a–i). Similarly, correlations with Ca, P, and Mg levels in the medium were observed (Supplementary Figure 11j–s), which confirms the findings from previous studies (Barradas et al., Citation2012).
The BMP pathway, an important contributor to osteogenic processes (Wang, Wang, et al., Citation2019), was mainly influenced by structural parameters (Supplementary Figure 12). Previous studies where a chemistry-topography decoupling strategy was used, showed a mild effect of topography on BMP-2 expression (Danoux, Sun, et al., Citation2016; Sun et al., Citation2016).
Finally, we observed correlations between WNT pathway genes and both chemical and topographical parameters (Supplementary Figure 13). Examples include the WNT5b ligand, a crucial component of the WNT pathway (Perkins, Suthon, Miranda-Carboni, & Krum, Citation2021), with a positive correlation existing with Smr1 and the P levels in the cell culture medium. Next to WNT5b, we also found a positive correlation between the Mg content of the cell culture media and receptor tyrosine kinases Ryk, WNT1-Inducible-Signaling Pathway Protein 1 (WISP1), and Zinc Finger RANBP2-Type Containing 1 (ZRANB1), all proteins related to WNT signaling (Green, Nusse, & van Amerongen, Citation2014; Tran, Hamada, Schwarz-Romond, & Bienz, Citation2008; Xu, Corcoran, Welsh, Pennica, & Levine, Citation2000). Why this correlation exist needs further investigation. Considering the importance of WNT signaling for osteogenesis, it is not surprising that CaP ceramics can influence this pathway (Wang, Pan, et al., Citation2019).
The bioinformatics method used here proved capable of identifying numerous correlations between material properties and gene pathways, most of which are supported by the literature. This allows to select a certain material property and analyze its influence at a single gene level. For example, several strong correlations were observed between the microporosity and a range of genes belonging to various categories as described before (). Previous research associated microporosity with cell adhesion (Hoai & Nga, Citation2018), osteogenic differentiation, and in vivo bone formation (Duan et al., Citation2017). In addition, the presence of micropores has been related to changes in cytoskeleton (Hoai & Nga, Citation2018), ECM production (Lien, Ko, & Huang, Citation2009), and migration towards/through the pores (Hoai & Nga, Citation2018; Li et al., Citation2015; Mandal & Kundu, Citation2009; Pamula, Filova, Bacakova, Lisa, & Adamczyk, Citation2009). Finally, cell proliferation also seems to be affected by the microporosity of the substrate (Hoai & Nga, Citation2018; Hornez et al., Citation2007; Li et al., Citation2015).
Table 2. Genes correlated with the microporosity parameter with known relevant functions.
This study also showed some unexpected results, which require further investigation. For example, based on the previous literature, one would expect stronger correlations between the Ca signaling pathway, the Ca content of the ceramics and the cell culture medium (Barradas et al., Citation2012). Similarly, no correlations were found between phosphate signaling and the P content of the medium. This might be because we only looked at a 48-hour time point in the transriptomics study and may have missed some processes, such as a strong upregulation of BMP-2 at earlier time points. Similarly, we obtained correlations for STAT1, which is known to upregulate RUNX2, but not for RUNX2 itself, which may be expressed at a later time point. Furthermore, some other parts of the experimental setup need to be considered for future studies, such as the fact that the cell culture medium used for the analysis of the inorganic content was collected in the absence of cells, which may be different from the setting where cells are present.
Taken together, the main achievement of this study is that we have shown the potential of the bioinformatics approach to explore correlations between materials properties and transcriptomics results, thereby taking into consideration many more parameters than would be possible without such a tool. The results pointed to some interesting correlations, many of which were supported by the available literature. Nevertheless, a follow-up validation phase is essential to verify the results concerning the correlations found among materials properties and between material properties and transcriptomics. In the future, we also foresee that other ceramic or composite designs, and cell types, such as primary osteoblasts or osteoclasts, will be included in our bioinformatics approach to further elucidate material parameters relevant for bone regeneration.
4. Conclusions
In this study, we used a bioinformatics approach to explore the correlations between properties of six CaP ceramics with known osteoinductive and bone regenerative potential and gene expression of MG63 cells cultured on these materials. A comprehensive material characterization and data analysis were performed to obtain a large set of numerical parameters describing both the chemistry and the microstructure of the ceramics. The results showed that the ceramics could be divided into an osteoinductive and a non-osteoinductive group based on their material parameters. A Spearman analysis of the material properties revealed a number of correlations between chemical and structural parameters, confirming that the properties of CaPs are largely intertwined. The Pearson correlation analysis of the materials properties dataset with the transcriptomics gene expression dataset identified a large number of correlations, most of which were supported by the literature. The approach here proves the value of detailed (bio)materials characterization in combination with statistical analysis to identify the genes and gene pathways regulated by the individual material properties.
Acknowledgments
The authors acknowledge Dr. Arno Wilbers (DSM Resolve) for the help with the acquisition and reconstruction of the microCT datasets, and Dr. Nicola Döbelin (RMS Foundation) for the assistance help with the Rietveld analysis.
Disclosure statement
No potential conflict of interest was reported by the authors.
Data availability statement
The raw/processed data to reproduce these findings is available in the following repository: https://doi.org/10.34894/VWMT6T
Additional information
Funding
References
- Anselme, K., & Bigerelle, M. (2011). Role of materials surface topography on mammalian cell response. International Materials Reviews, 56(4), 243–266. doi:10.1179/1743280411Y.0000000001
- Arimochi, H., Sasaki, Y., Kitamura, A., & Yasutomo, K. (2016). Differentiation of preadipocytes and mature adipocytes requires PSMB8. Scientific Reports, 6, 26791. doi:10.1038/srep26791
- Bai, J., Wioland, H., Advedissian, T., Cuvelier, F., Romet-Lemonne, G., & Echard, A. (2020). Actin reduction by MsrB2 is a key component of the cytokinetic abscission checkpoint and prevents tetraploidy. Proceedings of the National Academy of Sciences of the United States of America, 117(8), 4169–4179. doi:10.1073/pnas.1911629117
- Baker, B. A., Pine, P. S., Chatterjee, K., Kumar, G., Lin, N. J., McDaniel, J. H., … Simon, C. G. (2014). Ontology analysis of global gene expression differences of human bone marrow stromal cells cultured on 3D scaffolds or 2D films. Biomaterials, 35(25), 6716–6726. doi:10.1016/j.biomaterials.2014.04.075
- Barradas, A. M. C., Fernandes, H. A. M., Groen, N., Chai, Y. C., Schrooten, J., van de Peppel, J., … de Boer, J. (2012). A calcium-induced signaling cascade leading to osteogenic differentiation of human bone marrow-derived mesenchymal stromal cells. Biomaterials, 33(11), 3205–3215. doi:10.1016/j.biomaterials.2012.01.020
- Barrère-de Groot, F., Yuan, H., & de Bruijn, J. D. (2018). Clinical relevance of sub-micron textured calcium phosphates for bone tissue regeneration. Materials Today: Proceedings, 5(7), 15501–15505.
- Batra, U., Kapoor, S., & Sharma, S. (2013). Influence of Magnesium Ion Substitution on Structural and Thermal Behavior of Nanodimensional Hydroxyapatite. Journal of Materials Engineering and Performance, 22(6), 1798–1806. doi:10.1007/s11665-012-0462-2
- Bennett, J., & Weeds, A. (1986). Calcium and the cytoskeleton. British Medical Bulletin, 42(4), 385–390. doi:10.1093/oxfordjournals.bmb.a072156
- Birgani, Z. T. (2016). Synthetic growth factors for bone regeneration. Enschede, the Netherlands: University of Twente.
- Birgani, Z. T., Malhotra, A., van Blitterswijk, C. A., & Habibovic, P. (2016). Human mesenchymal stromal cells response to biomimetic octacalcium phosphate containing strontium. Journal of Biomedical Materials Research. Part A, 104(8), 1946–1960. doi:10.1002/jbm.a.35725
- Bohner, M., & Miron, R. J. (2019). A proposed mechanism for material-induced heterotopic ossification. Materials Today, 22, 132–141. doi:10.1016/j.mattod.2018.10.036
- Bu, W., & Su, L.-K. (2001). Regulation of microtubule assembly by human EB1 family proteins. Oncogene, 20(25), 3185–3192. doi:10.1038/sj.onc.1204429
- Cai, S., Wu, C., Yang, W., Liang, W., Yu, H., & Liu, L. (2020). Recent advance in surface modification for regulating cell adhesion and behaviors. Nanotechnology Reviews, 9(1), 971–989. doi:10.1515/ntrev-2020-0076
- Chande, S., & Bergwitz, C. (2018). Role of phosphate sensing in bone and mineral metabolism. Nature Reviews. Endocrinology, 14(11), 637–655. doi:10.1038/s41574-018-0076-3
- Dalby, M. J., Gadegaard, N., Tare, R., Andar, A., Riehle, M. O., Herzyk, P., … Oreffo, R. O. C. (2007). The control of human mesenchymal cell differentiation using nanoscale symmetry and disorder. Nature Materials, 6(12), 997–1003. doi:10.1038/nmat2013
- Danoux, C. B. S. S., Bassett, D. C., Othman, Z., Rodrigues, A. I., Reis, R. L., Barralet, J. E., … Habibovic, P. (2015). Elucidating the individual effects of calcium and phosphate ions on hMSCs by using composite materials. Acta Biomaterialia, 17, 1–15. doi:10.1016/j.actbio.2015.02.003
- Danoux, C., Pereira, D., Döbelin, N., Stähli, C., Barralet, J., van Blitterswijk, C., & Habibovic, P. (2016). The effects of crystal phase and particle morphology of calcium phosphates on proliferation and differentiation of human mesenchymal stromal cells. Advanced Healthcare Materials, 5(14), 1775–1785. doi:10.1002/adhm.201600184
- Danoux, C., Sun, L., Koçer, G., Birgani, Z. T., Barata, D., Barralet, J., … Habibovic, P. (2016). Development of highly functional biomaterials by decoupling and recombining material properties. Advanced Materials (Deerfield Beach, Fla.), 28(9), 1803–1808. doi:10.1002/adma.201504589
- Dasgupta, S., Tarafder, S., Bandyopadhyay, A., & Bose, S. (2013). Effect of grain size on mechanical, surface and biological properties of microwave sintered hydroxyapatite. Materials Science & Engineering. C, Materials for Biological Applications, 33(5), 2846–2854. doi:10.1016/j.msec.2013.03.004
- Davison, N. L., Luo, X., Schoenmaker, T., Everts, V., Yuan, H., Barrère-de Groot, F., & de Bruijn, J. D. (2014). Submicron-scale surface architecture of tricalcium phosphate directs osteogenesis in vitro and in vivo. European Cells & Materials, 27, 281–297; discussion 296–297. doi:10.22203/ecm.v027a20
- Davison, N., Yuan, H., de Bruijn, J. D., & Barrere-de Groot, F. (2012). In vivo performance of microstructured calcium phosphate formulated in novel water-free carriers. Acta Biomaterialia, 8(7), 2759–2769. doi:10.1016/j.actbio.2012.04.007
- Deligianni, D. D., Katsala, N. D., Koutsoukos, P. G., & Missirlis, Y. F. (2000). Efect of surface roughness of hydroxyapatite on human bone marrowcell adhesion, proliferation, differentiation and detachment strength. Biomaterials, 22(1), 87–96. doi:10.1016/S0142-9612(00)00174-5
- Doebelin, N., & Kleeberg, R. (2015). Profex: A graphical user interface for the Rietveld refinement program BGMN. Journal of Applied Crystallography, 48(Pt 5), 1573–1580. doi:10.1107/S1600576715014685
- dos Santos, E. A., Farina, M., Soares, G. A., & Anselme, K. (2009). Chemical and topographical influence of hydroxyapatite and beta-tricalcium phosphate surfaces on human osteoblastic cell behavior. Journal of Biomedical Materials Research. Part A, 89(2), 510–520. doi:10.1002/jbm.a.31991
- Duan, R., Barbieri, D., Luo, X., Weng, J., Bao, C., de Bruijn, J. D., & Yuan, H. (2017). Variation of the bone forming ability with the physicochemical properties of calcium phosphate bone substitutes. Biomaterials Science, 6(1), 136–145. doi:10.1039/c7bm00717e
- Engel, E., Del Valle, S., Aparicio, C., Altankov, G., Asin, L., Planell, J. A., & Ginebra, M.-P. (2008). Discerning the role of topography and ion exchange in cell response of bioactive tissue engineering scaffolds. Tissue Engineering. Part A, 14(8), 1341–1351. doi:10.1089/ten.tea.2007.0287
- Eyckmans, J., Roberts, S. J., Bolander, J., Schrooten, J., Chen, C. S., & Luyten, F. P. (2013). Mapping calcium phosphate activated gene networks as a strategy for targeted osteoinduction of human progenitors. Biomaterials, 34(19), 4612–4621. doi:10.1016/j.biomaterials.2013.03.011
- Faia-Torres, A. B., Guimond-Lischer, S., Rottmar, M., Charnley, M., Goren, T., Maniura-Weber, K., … Neves, N. M. (2014). Differential regulation of osteogenic differentiation of stem cells on surface roughness gradients. Biomaterials, 35(33), 9023–9032. doi:10.1016/j.biomaterials.2014.07.015
- Fang, Y., Zekiy, A. O., Ghaedrahmati, F., Timoshin, A., Farzaneh, M., Anbiyaiee, A., & Khoshnam, S. E. (2021). Tribbles homolog 2 (Trib2), a pseudo serine/threonine kinase in tumorigenesis and stem cell fate decisions. Cell Communication and Signaling: CCS, 19(1), 41. doi:10.1186/s12964-021-00725-y
- Farzadi, A., Bakhshi, F., Solati-Hashjin, M., Asadi-Eydivand, M., & Osman, NAa. (2014). Magnesium incorporated hydroxyapatite: Synthesis and structural properties characterization. Ceramics International, 40(4), 6021–6029. doi:10.1016/j.ceramint.2013.11.051
- Franceschi, R. T., & Xiao, G. (2003). Regulation of the osteoblast-specific transcription factor, Runx2: Responsiveness to multiple signal transduction pathways. Journal of Cellular Biochemistry, 88(3), 446–454. doi:10.1002/jcb.10369
- Galvan-Chacon, V. P., & Habibovic, P. (2017). Deconvoluting the bioactivity of calcium phosphate-based bone graft substitutes: Strategies to understand the role of individual material properties. Advanced Healthcare Materials, 6(13), 1478.
- Gibson, I. R., & Bonfield, W. (2002). Preparation and characterization of magnesium/carbonate co-substituted hydroxyapatites. Journal of Materials Science. Materials in Medicine, 13(7), 685–693.
- Gillman, C. E., & Jayasuriya, A. C. (2021). FDA-approved bone grafts and bone graft substitute devices in bone regeneration. Materials Science & Engineering. C, Materials for Biological Applications, 130, 112466. doi:10.1016/j.msec.2021.112466
- Govoni, M., Vivarelli, L., Mazzotta, A., Stagni, C., Maso, A., & Dallari, D. (2021). Commercial bone grafts claimed as an alternative to autografts: Current trends for clinical applications in orthopaedics. Materials (Basel), 14(12), 3290. doi:10.3390/ma14123290
- Green, J., Nusse, R., & van Amerongen, R. (2014). The role of Ryk and Ror receptor tyrosine kinases in Wnt signal transduction. Cold Spring Harbor Perspectives in Biology, 6(2), a009175.
- Groen, N., Yuan, H., Hebels, D. G., Kocer, G., Mbuyi, F., & LaPointe, V. (2017). Linking the transcriptional landscape of bone induction to biomaterial design parameters. Advanced Materials, 29(10), 3259.
- Guadarrama Bello, D., Fouillen, A., Badia, A., & Nanci, A. (2017). A nanoporous titanium surface promotes the maturation of focal adhesions and formation of filopodia with distinctive nanoscale protrusions by osteogenic cells. Acta Biomaterialia, 60, 339–349. doi:10.1016/j.actbio.2017.07.022
- Habibovic, P., & Barralet, J. E. (2011). Bioinorganics and biomaterials: Bone repair. Acta Biomaterialia, 7(8), 3013–3026. doi:10.1016/j.actbio.2011.03.027
- Habibovic, P., Yuan, H., van den Doel, M., Sees, T. M., van Blitterswijk, C. A., & de Groot, K. (2006). Relevance of osteoinductive biomaterials in critical-sized orthotopic defect. Journal of Orthopaedic Research, 24(5), 867–876. doi:10.1002/jor.20115
- Hahn, M., Vogel, M., Pompesius-Kempa, M., & Delling, G. (1992). Trabecular bone pattern factor – a new parameter for simple quantification of bone microarchitecture. Bone, 13(4), 327–330. doi:10.1016/8756-3282(92)90078-b
- He, D., Liu, F., Cui, S., Jiang, N., Yu, H., Zhou, Y., … Kou, X. (2020). Mechanical load-induced H2S production by periodontal ligament stem cells activates M1 macrophages to promote bone remodeling and tooth movement via STAT1. Stem Cell Research & Therapy, 11(1), 112. doi:10.1186/s13287-020-01607-9
- Hoai, T. T., & Nga, N. K. (2018). Effect of pore architecture on osteoblast adhesion and proliferation on hydroxyapatite/poly(D,L) lactic acid-based bone scaffolds. Journal of the Iranian Chemical Society, 15(7), 1663–1671. doi:10.1007/s13738-018-1365-4
- Hornez, J. C., Chai, F., Monchau, F., Blanchemain, N., Descamps, M., & Hildebrand, H. F. (2007). Biological and physico-chemical assessment of hydroxyapatite (HA) with different porosity. Biomolecular Engineering, 24(5), 505–509. doi:10.1016/j.bioeng.2007.08.015
- Kannan, S., Ventura, J. M., & Ferreira, J. M. F. (2007). Aqueous precipitation method for the formation of Mg-stabilized β-tricalcium phosphate: An X-ray diffraction study. Ceramics International, 33(4), 637–641. doi:10.1016/j.ceramint.2005.11.014
- Kano, S., Miyajima, N., Fukuda, S., & Hatakeyama, S. (2008). Tripartite motif protein 32 facilitates cell growth and migration via degradation of Abl-interactor 2. Cancer Research, 68(14), 5572–5580. doi:10.1158/0008-5472.CAN-07-6231
- Kean, M. J., Williams, K. C., Skalski, M., Myers, D., Burtnik, A., Foster, D., & Coppolino, M. G. (2009). VAMP3, syntaxin-13 and SNAP23 are involved in secretion of matrix metalloproteinases, degradation of the extracellular matrix and cell invasion. Journal of Cell Science, 122(Pt 22), 4089–4098. doi:10.1242/jcs.052761
- Landi, E., Logroscino, G., Proietti, L., Tampieri, A., Sandri, M., & Sprio, S. (2008). Biomimetic Mg-substituted hydroxyapatite: From synthesis to in vivo behaviour. Journal of Materials Science. Materials in Medicine, 19(1), 239–247. doi:10.1007/s10856-006-0032-y
- Lapczyna, H., Galea, L., Wüst, S., Bohner, M., Jerban, S., Sweedy, A., … von Rechenberg, B. (2014). Effect of grain size and microporosity on the in vivo behaviour of beta-tricalcium phosphate scaffolds. European Cells and Materials, 28, 299–319. doi:10.22203/eCM.v028a21
- Le, B. Q., Vasilevich, A., Vermeulen, S., Hulshof, F., Stamatialis, D. F., van Blitterswijk, C. A., & de Boer, J. (2017). Micro-Topographies Promote Late Chondrogenic Differentiation Markers in the ATDC5 Cell Line. Tissue Engineering. Part A, 23(9–10), 458–469. doi:10.1089/ten.TEA.2016.0421
- Lee, W. H., Zavgorodniy, A. V., Loo, C. Y., & Rohanizadeh, R. (2012). Synthesis and characterization of hydroxyapatite with different crystallinity: Effects on protein adsorption and release. Journal of Biomedical Materials Research. Part A, 100(6), 1539–1549. doi:10.1002/jbm.a.34093
- Li, S., De Wijn, J. R., Li, J., Layrolle, P., & De Groot, K. (2003). Macroporous biphasic calcium phosphate scaffold with high permeability/porosity ratio. Tissue Engineering, 9(3), 535–548. doi:10.1089/107632703322066714
- Li, Y.-F., Gregersen, H., Nygaard, J. V., Cheng, W., Yu, Y., Huang, Y., … Chen, M. (2015). Ultraporous nanofeatured PCL-PEO microfibrous scaffolds enhance cell infiltration, colonization and myofibroblastic differentiation. Nanoscale, 7(36), 14989–14995. doi:10.1039/c5nr04244e
- Liang, T., Gao, W., Zhu, L., Ren, J., Yao, H., & Wang, K. (2019). TIMP-1 inhibits proliferation and osteogenic differentiation of hBMSCs through Wnt/beta-catenin signaling. Bioscience Reports, 39(1), BSR20181290.
- Lien, S. M., Ko, L. Y., & Huang, T. J. (2009). Effect of pore size on ECM secretion and cell growth in gelatin scaffold for articular cartilage tissue engineering. Acta Biomaterialia, 5(2), 670–679. doi:10.1016/j.actbio.2008.09.020
- Lincks, J., Boyan, B. D., Blanchard, C. R., Lohmann, C. H., Liu, Y., & Cochran, D. L. (1998). Response of MG63 osteoblast-like cells to titanium and titanium alloy is dependent on surface roughness and composition. Biomaterials, 19(23), 2219–2232. doi:10.1016/S0142-9612(98)00144-6
- Linez-Bataillon, P., Monchau, F., Bigerelle, M., & Hildebrand, H. F. (2002). In vitro MC3T3 osteoblast adhesion with respect to surface roughness of Ti6Al4V substrates. Biomolecular Engineering, 19(2–6), 133–141. doi:10.1016/S1389-0344(02)00024-2
- Luftman, K., Hasan, N., Day, P., Hardee, D., & Hu, C. (2009). Silencing of VAMP3 inhibits cell migration and integrin-mediated adhesion. Biochemical and Biophysical Research Communications, 380(1), 65–70. doi:10.1016/j.bbrc.2009.01.036
- Maazouz, Y., Chizzola, G., Dobelin, N., & Bohner, M. (2021). Cell-free, quantitative mineralization measurements as a proxy to identify osteoinductive bone graft substitutes. Biomaterials, 275, 120912. doi:10.1016/j.biomaterials.2021.120912
- Maazouz, Y., Rentsch, I., Lu, B., Santoni, B. L. G., Doebelin, N., & Bohner, M. (2020). In vitro measurement of the chemical changes occurring within beta-tricalcium phosphate bone graft substitutes. Acta Biomaterialia, 102, 440–457. doi:10.1016/j.actbio.2019.11.035
- Mailer, R. K., Allende, M., Heestermans, M., Schweizer, M., Deppermann, C., Frye, M., … Renné, T. (2021). Xenotropic and polytropic retrovirus receptor 1 regulates procoagulant platelet polyphosphate. Blood, 137(10), 1392–1405. doi:10.1182/blood.2019004617
- Mandal, B. B., & Kundu, S. C. (2009). Cell proliferation and migration in silk fibroin 3D scaffolds. Biomaterials, 30(15), 2956–2965. doi:10.1016/j.biomaterials.2009.02.006
- Mazón, P., García-Bernal, D., Meseguer-Olmo, L., Cragnolini, F., & De Aza, P. N. (2015). Human mesenchymal stem cell viability, proliferation and differentiation potential in response to ceramic chemistry and surface roughness. Ceramics International, 41(5), 6631–6644. doi:10.1016/j.ceramint.2015.01.110
- Mehrjoo, M., Javadpour, J., Shokrgozar, M. A., Farokhi, M., Javadian, S., & Bonakdar, S. (2015). Effect of magnesium substitution on structural and biological properties of synthetic hydroxyapatite powder. Materials Express, 5(1), 41–48. doi:10.1166/mex.2015.1205
- Mizuno, N., Iwata, T., Ohsawa, R., Ouhara, K., Matsuda, S., Kajiya, M., … Kurihara, H. (2020). Optineurin regulates osteoblastogenesis through STAT1. Biochemical and Biophysical Research Communications, 525(4), 889–894. doi:10.1016/j.bbrc.2020.03.028
- Murakami, K., Yasunaga, T., Noguchi, T. Q. P., Gomibuchi, Y., Ngo, K. X., Uyeda, T. Q. P., & Wakabayashi, T. (2010). Structural basis for actin assembly, activation of ATP hydrolysis, and delayed phosphate release. Cell, 143(2), 275–287. doi:10.1016/j.cell.2010.09.034
- Nabiyouni, M., Ren, Y., & Bhaduri, S. B. (2015). Magnesium substitution in the structure of orthopedic nanoparticles: A comparison between amorphous magnesium phosphates, calcium magnesium phosphates, and hydroxyapatites. Materials Science & Engineering. C, Materials for Biological Applications, 52, 11–17. doi:10.1016/j.msec.2015.03.032
- Nehlig, A., Molina, A., Rodrigues-Ferreira, S., Honore, S., & Nahmias, C. (2017). Regulation of end-binding protein EB1 in the control of microtubule dynamics. Cellular and Molecular Life Sciences: CMLS, 74(13), 2381–2393. doi:10.1007/s00018-017-2476-2
- Othman, Z., Fernandes, H., Groot, A. J., Luider, T. M., Alcinesio, A., Pereira, D. d M., … Habibovic, P. (2019). The role of ENPP1/PC-1 in osteoinduction by calcium phosphate ceramics. Biomaterials, 210, 12–24. doi:10.1016/j.biomaterials.2019.04.021
- Otsuka, M., Oshinbe, A., LeGeros, R. Z., Tokudome, Y., Ito, A., Otsuka, K., & Higuchi, W. I. (2008). Efficacy of the injectable calcium phosphate ceramics suspensions containing magnesium, zinc and fluoride on the bone mineral deficiency in ovariectomized rats. Journal of Pharmaceutical Sciences, 97(1), 421–432. doi:10.1002/jps.21131
- Pamula, E., Filova, E., Bacakova, L., Lisa, V., & Adamczyk, D. (2009). Resorbable polymeric scaffolds for bone tissue engineering: The influence of their microstructure on the growth of human osteoblast-like MG 63 cells. Journal of Biomedical Materials Research. Part A, 89(2), 432–443. doi:10.1002/jbm.a.31977
- Perkins, R. S., Suthon, S., Miranda-Carboni, G. A., & Krum, S. A. (2021). WNT5B in cellular signaling pathways. Seminars in Cell & Developmental Biology, 125, 11–16.
- Place, D. E., Malireddi, R. K. S., Kim, J., Vogel, P., Yamamoto, M., & Kanneganti, T. D. (2021). Osteoclast fusion and bone loss are restricted by interferon inducible guanylate binding proteins. Nature Communications, 12(1), 496. doi:10.1038/s41467-020-20807-8
- Prihadi, J. C., Sugandi, S., Siregar, N. C., Soejono, G., & Harahap, A. (2018). Imbalance in extracellular matrix degradation in urethral stricture. Research and Reports in Urology, 10, 227–232. doi:10.2147/RRU.S178904
- Ribeiro, C. M., Reece, J., & Putney, J. W. Jr.(1997). Role of the cytoskeleton in calcium signaling in NIH 3T3 cells. An intact cytoskeleton is required for agonist-induced [Ca2+]i signaling, but not for capacitative calcium entry. The Journal of Biological Chemistry, 272(42), 26555–26561. doi:10.1074/jbc.272.42.26555
- Riggs, K. A., Hasan, N., Humphrey, D., Raleigh, C., Nevitt, C., Corbin, D., & Hu, C. (2012). Regulation of integrin endocytic recycling and chemotactic cell migration by syntaxin 6 and VAMP3 interaction. Journal of Cell Science, 125(Pt 16), 3827–3839.
- Rosado, J. A., & Sage, S. O. (2000). The actin cytoskeleton in storemediated calcium entry. The Journal of Physiology, 526(2), 221–229. doi:10.1111/j.1469-7793.2000.t01-2-00221.x
- Semnani, D. (2017). Geometrical characterization of electrospun nanofibers. In Electrospun nanofibers (pp. 151–180). Sawston, UK: Woodhead Publishing.
- Sheikh, Z., Abdallah, M.-N., Hanafi, A., Misbahuddin, S., Rashid, H., & Glogauer, M. (2015). Mechanisms of in Vivo Degradation and Resorption of Calcium Phosphate Based Biomaterials. Materials (Basel, Switzerland), 8(11), 7913–7925. doi:10.3390/ma8115430
- Stiburek, L., Cesnekova, J., Kostkova, O., Fornuskova, D., Vinsova, K., Wenchich, L., … Zeman, J. (2012). YME1L controls the accumulation of respiratory chain subunits and is required for apoptotic resistance, cristae morphogenesis, and cell proliferation. Molecular Biology of the Cell, 23(6), 1010–1023. doi:10.1091/mbc.E11-08-0674
- Sun, L., Danoux, C. B., Wang, Q., Pereira, D., Barata, D., Zhang, J., … Habibovic, P. (2016). Independent effects of the chemical and microstructural surface properties of polymer/ceramic composites on proliferation and osteogenic differentiation of human MSCs. Acta Biomaterialia, 42, 364–377. doi:10.1016/j.actbio.2016.06.018
- Tahmasebi Birgani, Z., Fennema, E., Gijbels, M. J., de Boer, J., van Blitterswijk, C. A., & Habibovic, P. (2016). Stimulatory effect of cobalt ions incorporated into calcium phosphate coatings on neovascularization in an in vivo intramuscular model in goats. Acta Biomaterialia, 36, 267–276. doi:10.1016/j.actbio.2016.03.031
- Tamimi, F., Le Nihouannen, D., Eimar, H., Sheikh, Z., Komarova, S., & Barralet, J. (2012). The effect of autoclaving on the physical and biological properties of dicalcium phosphate dihydrate bioceramics: Brushite vs. monetite. Acta Biomaterialia, 8(8), 3161–3169. doi:10.1016/j.actbio.2012.04.025
- Tran, H., Hamada, F., Schwarz-Romond, T., & Bienz, M. (2008). Trabid, a new positive regulator of Wnt-induced transcription with preference for binding and cleaving K63-linked ubiquitin chains. Genes & Development, 22(4), 528–542. doi:10.1101/gad.463208
- Vasilevich, A. S., Vermeulen, S., Kamphuis, M., Roumans, N., Eroumé, S., Hebels, D. G. A. J., … de Boer, J. (2020). On the correlation between material-induced cell shape and phenotypical response of human mesenchymal stem cells. Scientific Reports, 10(1), 18988. doi:10.1038/s41598-020-76019-z
- Veljović, D., Colić, M., Kojić, V., Bogdanović, G., Kojić, Z., Banjac, A., … Janaćković, D. (2012). The effect of grain size on the biocompatibility, cell-materials interface, and mechanical properties of microwave-sintered bioceramics. Journal of Biomedical Materials Research. Part A, 100(11), 3059–3070. doi:10.1002/jbm.a.34225
- Vermeulen, S., Roumans, N., Honig, F., Carlier, A., Hebels, D. G. A. J., Eren, A. D., … de Boer, J. (2020). Mechanotransduction is a context-dependent activator of TGF-beta signaling in mesenchymal stem cells. Biomaterials, 259, 120331. doi:10.1016/j.biomaterials.2020.120331
- Vermeulen, S., Tahmasebi Birgani, Z., & Habibovic, P. (2022). Biomaterial-induced pathway modulation for bone regeneration. Biomaterials, 283, 121431. doi:10.1016/j.biomaterials.2022.121431
- Wang, J., Wang, M., Chen, F., Wei, Y., Chen, X., Zhou, Y., … Zhang, X. (2019). Nano-hydroxyapatite coating promotes porous calcium phosphate ceramic-induced osteogenesis via BMP/Smad signaling pathway. International Journal of Nanomedicine, 14, 7987–8000. doi:10.2147/IJN.S216182
- Wang, L., Barbieri, D., Zhou, H., de Bruijn, J. D., Bao, C., & Yuan, H. (2015). Effect of particle size on osteoinductive potential of microstructured biphasic calcium phosphate ceramic. Journal of Biomedical Materials Research. Part A, 103(6), 1919–1929. doi:10.1002/jbm.a.35325
- Wang, Y., Pan, J., Zhang, Y., Li, X., Zhang, Z., Wang, P., … Li, J. (2019). Wnt and Notch signaling pathways in calcium phosphate-enhanced osteogenic differentiation: A pilot study. Journal of Biomedical Materials Research. Part B, Applied Biomaterials, 107(1), 149–160. doi:10.1002/jbm.b.34105
- Webb, H. K., Truong, V. K., Hasan, J., Fluke, C., Crawford, R. J., & Ivanova, E. P. (2012). Roughness parameters for standard description of surface nanoarchitecture. Scanning, 34(4), 257–263. doi:10.1002/sca.21002
- Wilkinson, A., Hewitt, R. N., McNamara, L. E., McCloy, D., Dominic Meek, R. M., & Dalby, M. J. (2011). Biomimetic microtopography to enhance osteogenesis in vitro. Acta Biomaterialia, 7(7), 2919–2925. doi:10.1016/j.actbio.2011.03.026
- Xiao, Y., Guo, Q., Jiang, T.-J., Yuan, Y., Yang, L., Wang, G.-W., & Xiao, W.-F. (2019). miR4833p regulates osteogenic differentiation of bone marrow mesenchymal stem cells by targeting STAT1. Molecular Medicine Reports, 20(5), 4558–4566.
- Xu, L., Corcoran, R. B., Welsh, J. W., Pennica, D., & Levine, A. J. (2000). WISP-1 is a Wnt-1- and β-catenin-responsive oncogene. Genes & Development, 14(5), 585–595. doi:10.1101/gad.14.5.585
- Yuan, H., Fernandes, H., Habibovic, P., de Boer, J., Barradas, A. M. C., de Ruiter, A., … de Bruijn, J. D. (2010). Osteoinductive ceramics as a synthetic alternative to autologous bone grafting. Proceedings of the National Academy of Sciences of the United States of America, 107(31), 13614–13619. doi:10.1073/pnas.1003600107
- Yuan, H., van Blitterswijk, C. A., de Groot, K., & de Bruijn, J. D. (2006). A comparison of bone formation in biphasic calcium phosphate (BCP) and hydroxyapatite (HA) implanted in muscle and bone of dogs at different time periods. Journal of Biomedical Materials Research Part A, 78A(1), 139–147. doi:10.1002/jbm.a.30707
- Yuan, H., Yang, Z., De Bruij, J. D., De Groot, K., & Zhang, X. (2001). Material-dependent bone induction by calcium phosphate ceramics: A 2.5 years study in dogs. Biomaterials, 22(19), 2617–2623. doi:10.1016/S0142-9612(00)00450-6