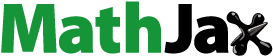
ABSTRACT
The COVID-19 pandemic and shift to remote instruction disrupted students’ learning and well-being. This study explored undergraduates’ incoming course concerns and later perceived challenges in an introductory statistics course. We explored how the frequency of concerns changed with the onset of COVID-19 (1417) and, during COVID-19, how incoming concerns compared to later perceived challenges (
524). Students were most concerned about R coding, understanding concepts, workload, prior knowledge, time management, and performance, with each of these concerns mentioned less frequently during than before COVID-19. Concerns most directly related to the pandemic—virtual learning and inaccessibility of resources—showed an increase in frequency. The frequency of concerns differed by gender and URM status. The most frequently mentioned challenges were course workload, virtual learning, R coding, and understanding concepts, with significant differences by URM status. Concerns about understanding concepts, lack of prior knowledge, performance, and time management declined from the beginning to the end of the term. Workload had the highest rate of both consistency and emergence across the term. Because students’ perceptions have an impact on their experiences and expectations, understanding and addressing concerns and challenges could help guide instructional designers and policymakers as they develop interventions.
1 Introduction
The COVID-19 pandemic sent U.S. college students home in the spring of 2020 and by the end of the term, many research teams had launched studies to better understand the impact of the shift from in-person to remote learning. In a survey of students from colleges and universities across the U.S., a number of concerns among students were identified, including balancing school, work, and home responsibilities, finding quiet study spaces, and feeling disconnected from peers (Blankstein, Frederick, and Wolff-Eisenberg Citation2020), increasing workloads, and decreasing the ability to focus on school (Barber et al. 2021). Several of these concerns were identified by a sizable proportion of the samples studied. For example, mental health concerns were present for approximately half of students (Blankstein, Frederick, and Wolff-Eisenberg Citation2020; Murphy, Eduljee, and Croteau Citation2020). Roughly a third of students reported food or housing insecurity (Blankstein, Frederick, and Wolff-Eisenberg Citation2020).
Several studies examined concerns across subgroups, with particular attention paid to students from underrepresented minority groups (URM; e.g., Black and Latinx students) and first generation (FG) students (i.e., students who are enrolled in postsecondary education whose parents did not attend college; Cataldi, Bennett, and Chen Citation2018). The gaps between them and their majority, continuing generation peers that existed before the pandemic appeared to grow. Barber and colleagues (2021) found that both URM and FG students, more than their peers, felt higher demands on their time. They were expected to help with their siblings more than their peers and increased their work hours, leaving them with, generally, less time available to participate in remote instruction and to focus on schoolwork. URM and FG students also experienced greater economic and food insecurity and were less likely to have health insurance than were their peers. Hartzell, Hagen, and Devereux (Citation2021) found that URM students experienced an increase in family duties and greater difficulty accessing effective workspaces and necessary electronic devices. That FG students were more likely than continuing-generation students to experience financial hardships during the pandemic was corroborated by Soria et al. (Citation2020), who found that that hardship was reported to be the result of lost wages from family members, lost wages from on- or off-campus employment, and increased technology expenses. As a consequence of wage loss and increased expenses, FG students were nearly twice as likely to be concerned about paying for their education than were continuing-generation students. Their survey similarly identified higher rates of food and housing insecurity and mental health disorders among FG than among continuing-generation students. Like the URM students in Hartzell, Hagen, and Devereux (Citation2021) survey, FG students reported having more difficulties in finding adequate study spaces and the technology necessary to participate in remote instruction (Soria et al. Citation2020).
Some challenges related to the pandemic period were experienced at equal rates regardless of URM status. For instance, URM and majority students were as likely to struggle with internet access and to know someone at high risk of contracting COVID-19 (Hartzell, Hagen, and Devereux Citation2021). For other challenges, rates seen among majority students exceeded those among URM students. For instance, majority students were more concerned with the impacts of pandemic-related changes on their GPA and their ability to meet deadlines as they normally would (Hartzell, Hagen, and Devereux Citation2021).
Hartzell, Hagen, and Devereux (Citation2021) study was unique in its inclusion of an open-ended question. That question enabled researchers to identify concerns they might not have expected a priori. When they asked if students were satisfied with the measures taken by their university for managing COVID-19, those who indicated that they were dissatisfied were probed about the reason for their response. Across subgroups of students, the themes that arose from their responses, in order from most frequent, were monetary concerns (e.g., refunds of tuition, fees, and housing), communication (e.g., short notice of evacuation), the learning environment (e.g., lower quality learning and unfair grading), and size/focus of the university’s response (e.g., a wish for more resources or a belief that the university’s response was excessive).
Having concerns about their academic experiences and experiencing challenges to their learning predates the COVID pandemic. Ameliorating those concerns and addressing challenges has been a focus in education, and perhaps no more so than in STEM education. As the job market increasingly demands STEM training (Xue and Larson Citation2015; Camilli and Hira Citation2018) and in an effort to close racial and gender gaps among individuals pursuing these fields (Griffith Citation2010; Hurtado et al. Citation2010; Graham et al. Citation2013; Arcidiacono, Aucejo, and Hotz Citation2016), there has been an interest in better understanding the barriers students experience. Meaders et al. (Citation2020) explored undergraduate student concerns in introductory STEM courses by condensing multiple concerns that arose from an open-ended pilot survey (knowing what to study; course difficulty; pace; being expected to do too much independent learning outside of class; lack of prior knowledge, skills, and/or background; receiving too few in-depth explanations, and being able to get help) that students rated on a scale from 0 (not concerned) to 2 (very concerned) into one score representing overall student concern. They found gender differences with female students reporting higher levels of concern than male students at the beginning of the semester. They further found that female students’ level of concern remained higher than male students during the semester even when controlling for initial concerns or course performance. Overall, students’ expectations, perceptions, and experiences play a critical role during college and can not only determine whether they persist in their chosen major, but also whether students apply statistics in their everyday lives (Kosovich, Flake, and Hulleman Citation2017; Rosenzweig et al. Citation2019).
In the present pair of studies, we were particularly interested in students’ experiences in introductory statistics courses. Introductory statistics is required across a wide variety of college majors-from physics, biology, and psychology, to sociology, economics, and political science. In the field of psychology, for example, statistical training is often considered a critical component of the curriculum (McGovern et al. Citation1991; Brewer et al. Citation1993; Garfield and Ben-Zvi Citation2007), with statistical understanding fundamental not only to writing research projects and theses but also to reading academic literature (Friedrich, Buday, and Kerr Citation2000). Understanding students’ concerns may be particularly relevant for improving instruction in complex domains like statistics, of which many students hold negative perceptions and in which many students often struggle and fail to transfer what they learn.
2 Assessing Student’s Course Appraisals
Although both the COVID-19 pandemic and the needs to increase student retention in STEM fields have heightened interest in assessing students’ perceptions of their academic experiences, a general interest in students’ perceptions is not new. Student evaluations of teaching, for example, are implemented widely and are used not only to improve the quality of teaching but also to inform retention and promotion of faculty (Zabaleta Citation2007). Although our interest in student evaluations is not limited to their thoughts on teaching, this body of work points out areas for further investigation that have informed the methodology of the present study.
Our first contribution is the timing. In most college courses, students’ opinions and course-related evaluations are solicited at the end of the course. However, there is also value in understanding how students evaluate a course or domain at its start. Prior research has shown that how people initially appraise or evaluate a situation influences their emotional reactions, the strategies they use, and how they respond to challenge and stress. For example, students who appraise a task as stressful or taxing, are more likely to experience distress related to that task (Lazarus and Folkman Citation1984). Students’ initial evaluations of the learning environment also play a role in self-regulated learning (Pintrich Citation2004; Zimmerman Citation2001; Panadero Citation2017). In the preparatory phase of learning, students analyze the task at hand, set goals, and plan their approach to learning. How students analyze the task, including what they expect will be difficult, influences the goals they set during learning, the way they approach the task, and how they assess their progress toward their goals.
Asking students to report their concerns at the start of a course offers several advantages. First, knowing what students are concerned about can assist instructors in designing instruction and creating a more student-centered learning environment. For example, knowing that students are concerned about memorizing code, formulas, or concepts could help instructors design communication about the nature of the course (e.g., they could highlight that memorizing formulas will not be required) or provide extra support to alleviate students’ concerns. Second, measuring concerns at the beginning of the course allows us to explore how they are related to challenges perceived at the end of the course as having impeded students’ success. This is important because some types of concerns may be more predictive than others. For example, concerns based on generalized assumptions about the course may not be as predictive as concerns grounded in students’ prior experiences, especially if the course violates students’ negative assumptions. Understanding how students’ appraisals at the start of the course relate to their later experiences in the course may provide insight into what aspects of statistics courses students perceive to be stressful and highlight areas for future intervention.
Another contribution is our consideration of how to measure students’ evaluations and expectations. Common methods assessing students’ appraisals and evaluations of the learning environment focus on the degree to which students evaluate a course or specific task as stressful or challenging solely relying on closed ended/multiple-choice surveys. They do not account for differences in the object of students’ evaluations. For example, two students may appraise a course as equally stressful but hold different expectations about where that stress will arise. One student may be worried about performing poorly on exams; another may be worried that they will not be able to balance the workload of the course with their other responsibilities. These categorical differences are important but are often overlooked aspects of students’ perceptions. Furthermore, most multiple-choice surveys constrain students’ responses and aren’t flexible to capture subtle changes that might be related to something unexpected like, say, a once in a century pandemic.
One way of capturing these more nuanced aspects of students’ appraisals is to supplement ratings and multiple-choice items with open-ended questions. Asking students to report their concerns at the beginning of the course (i.e., prior to any content being introduced) with the use of an open-ended question provides a way to tap into their mental models of the learning environment, including what they expect will be stressful or challenging.
3 The Current Study
This article comes at the intersection of understanding students’ expectations, perceptions, and experiences in an introductory statistics course (a STEM course required by many majors) and during the COVID pandemic, including how each of these are experienced differently by different subgroups of students. Thus, beyond exploring students’ concerns and challenges specific to a particular setting—introductory statistics—we seek to explore whether students’ concerns vary by gender and URM status. Gaining a deeper understanding of whether different subgroups of students, including by gender or URM, voice concerns/challenges differently or more frequently, will enable us to better design learning contexts and opportunity structures to support students from underrepresented or traditionally marginalized and minoritized backgrounds in STEM (e.g., female or Black and Latinx students; Gray, Hope, and Matthews Citation2018).
Our analyses focus on students’ responses to open-ended questions: “When I think about this course, I’m concerned that…” and “What, if anything, made it hard to succeed in this class?” Our desire was to allow for themes in students’ responses to arise from the responses themselves, rather than being constrained by the research team a priori. By asking these two, similar questions–one at the outset and the other at the end of the term—we hoped to gain a richer understanding of the variety of concerns students experienced and whether early concerns came to fruition. Data collection related to students’ concerns began before the COVID pandemic and continued during it, which enables us to describe concerns unencumbered by COVID as well as those affected by COVID-related challenges. Understanding those concerns could (a) help identify potential pain points among students, (b) help instructors allay potential unfounded fears, and (c) guide instructional designers and policymakers as they develop interventions. By analyzing data across different subgroups, we can assess whether concerns and challenges differed across them and whether the groups were differentially affected by the pandemic. Our research questions are thus (also see ):
Study 1a (Pre- and Mid-Pandemic Cohorts)
What concerns did students have about their introductory statistics course?
Did concerns differ by gender or URM status?
Did concerns vary depending on whether students took the course before or during the pandemic?
Were demographic subgroups (i.e., based on gender and URM status) differently affected by the onset of the pandemic?
Study 1b (Mid-Pandemic Cohort Only)
What challenges did students perceive as inhibiting their success in the course during the pandemic?
Did perceived challenges differ by gender or URM status?
To what degree did students’ initial concerns during the pandemic appear to be warranted? That is, were students’ early concerns consistent with what they later reported as having been challenges that inhibited their success in the course?
Did the degree of consistency between concerns and challenges vary by gender or URM status?
4 Method
4.1 Procedure and Study Context
Study participants were undergraduates enrolled in Introductory Statistics courses at UCLA. All sections used the same interactive statistics and data science textbook developed by CourseKata (https://coursekata.org; Son and Stigler 2017–Citation2022). The book consists of 12 chapters organized into three sections (i.e., exploring variation, modeling variation, and evaluating models) and includes over 1200 embedded formative assessments, including R programming exercises. In total, five course sections were taught in the Psychology department and three in the Political Science department. Introductory Statistics is a required course within both majors. The eight sections were taught by five instructors and together represent all of the classes at UCLA in which the Son and Stigler text was used during the 2019–2021 academic years. Data were thus collected from lecture courses held before (i.e., fall 2019 and winter 2020; 54.9%, n = 777; three course sections) and during the COVID-19 pandemic (i.e., spring 2020, winter and spring 2021; 45.1%; n = 639; four course sections). The course was not taught during the Fall 2020 term. Data were collected as part of an ongoing project, which was approved by the Institutional Review Board at the University of California, Los Angeles (IRB No: 20-001033). While the surveys were directly embedded in the online textbook and considered part of the course, they were not part of the grade nor did students receive extra credit; rather, students could always skip questions. Students knew that their de-identified responses to questions throughout the online, interactive book would be analyzed for the purpose of improving the materials themselves and at any time they were able to indicate that they wished for their data to be excluded. All data and code are available as supplementary resources.
4.2 Study 1a
4.2.1 Participants
The data used to address research questions 1–4 included undergraduate students who were enrolled in the Introductory Statistics course both before and during the COVID-19 pandemic (N 1417). This sample (see ) was 70.4% female (n = 998), 26.3% male (n = 372), 1.5% non binary (
), and 1.8% did not disclose their gender (n = 26). Overall, 33.8% self-identified as Asian or Asian American (n = 479), 26.8% as White (n = 380), 21.0% as Hispanic, Latinx, or Spanish (n = 298), 4.0% as African American or Black (
), 4.9% as Mixed (n = 69), 5.9% indicated a different race (n = 83) and 3.6% did not report their race (n = 52). For our analyses of underrepresented minorities (URM), White and Asian students were considered non-URM and African American, Black, Hispanic, Indian Subcontinent, Native American, and Greater Middle Eastern students were considered URM. Students of mixed race were included in the URM group, unless their race was a mix of White and Asian. In total, 62.2% (n = 882) students identified as either White or Asian and 34.0% (n = 482) were identified as belonging to an underrepresented minority group (URM).
Table 1 Demographic characteristics of participants in both samples.
4.2.2 Measures and Coding Procedure
Student concerns
In a required pre-course survey, students were asked the open-ended question, “When I think about this course, I’m concerned that…(If you have no concerns you may simply write ‘none’),” allowing students to express a wide range of concerns. The authors adopted this question from Gal and Ginsburg (Citation1994) and developed a preliminary coding scheme derived from the literature on student concerns as well as from students’ responses. They coded sets of 20 responses, met to discuss the codes and their application, and modified the coding scheme as necessary. They did so five times before feeling satisfied that the scheme adequately represented the universe of likely responses across the two questions and trusting that research assistants (RAs) could be trained to reliably apply the coding scheme (see ). A single student response could be coded for more than one concern.
One of the authors trained the RAs, providing them with the final coding scheme, including the overarching concept, descriptors, definitions, and examples for each code. RAs practiced applying the codes independently and reconvened to discuss questions and discrepancies. When they felt confident, RAs were assigned a subset of responses to code independently to check for inter-rater reliability. Inter-rater reliability reached over 92% for all categories and the remaining responses were divided among RAs.
Student demographics
Demographic variables that could account for differences in perceived course concerns were accounted for. These variables included self-reported gender (0 male, 1
female, 2
non binary) and underrepresented racial minority status, based on students’ self-reported race/ethnicity (0
non-URM, 1
URM).
4.2.3 Analysis
We explored students’ incoming course concerns (RQ1-RQ4) by computing frequencies of the categories of concerns mentioned. To assess differences in proportions across groups (RQ2: female vs. male; URM vs. non-URM; RQ3: pre- vs. mid-COVID cohorts; RQ4: within group differences pre- vs. mid-COVID cohorts), Pearson’s tests of independence were performed on six concerns that were mentioned by at least 10% of the full sample.Footnote1
4.3 Study 1b
4.3.1 Participants
The data used to address research questions 5–8 included students who were enrolled in the Introductory Statistics course during the COVID-19 pandemic and who were asked not only about their course concerns at the outset of the course but also, at the end of the course, about the challenges they felt might have impeded their success. While the cohort during the COVID-19 pandemic included n = 639 students, one course section (Spring 2020) was excluded since the version of the textbook they used did not include the question about challenges, reducing the sample size to n = 524 students. This study sample (see ) included 69.5% female (n = 364), 27.5% male (n = 144), 1.9% nonbinary (), and 1.1% did not disclose their gender (n = 6). Overall, 32.3% self-identified as Asian or Asian American (
), 25.4% as White (n = 133), 19.7% as Hispanic, Latinx, or Spanish (n = 103), 4.8% as African American or Black (
), 4.0% as Mixed (n = 21), 8.4% indicated a different race (n = 44) and 5.5% did not report their race (n = 29). For our analyses of underrepresented minorities (URM), White and Asian students were considered non-URM and African American, Black, Hispanic, Indian Subcontinent, Native American, and Greater Middle Eastern students were considered URM. Students of mixed race were included in the URM group, unless their race was a mix of White and Asian. In total, 58.8% (n = 308) students identified as either White or Asian and 35.7% (n = 187) were identified as belonging to an underrepresented minority group (URM).
4.3.2 Measures
Pre-Course concerns and Post-Course perceived challenges
The measures explored for this study included the same question regarding incoming course concerns as Study 1a (“When I think about this course, I’m concerned that…”) with an additional open-ended question asked post-course assessing students’ perceptions of challenges (“What, if anything, made it hard to succeed in this class?”, which was adapted from the Gal and Ginsburg Citation1994 item to be appropriate for use at the end of the course). During the development of the coding system used in Study 1a, students’ responses to the question related to challenges were taken into account. The same coding scheme was thus applied to both questions.
Student demographics
Demographic variables that could account for differences in reported concerns or challenges were accounted for. These variables included self-reported gender (0 male, 1
female, 2
nonbinary) and underrepresented racial minority status, based on students’ self-reported race/ethnicity (0
non-URM, 1
URM).
4.3.3 Analysis
We explored students’ perceived challenges at the end of the course (RQ5-RQ8) by computing frequencies of the categories of challenges mentioned. To assess differences in proportions across groups (RQ6: female vs. male; URM vs. non-URM; RQ7: reported concerns vs. challenges), Pearson’s tests of independence were performed on four challenges that were mentioned by at least 10% among the sampleFootnote2. To address RQ7—that whether students’ incoming course concerns were consistent with what they later reported as having been challenges that inhibited their success in the course—and RQ8—consistency between concerns and challenges across subgroups of students – we created a consistency indicator for students for whom we had complete data. If a student mentioned something as a concern but then did not respond to the challenge question (or vice versa) their data have been excluded. If a student mentioned an issue as a concern at the start of the course but not as a challenge at the end of the course, we classified it as “eliminated” and if an issue was not mentioned as a concern at the start of the course but was brought up as a challenge at the end of the course, we classified it as “emergent.” If a student mentioned an issue as a concern and later mentioned it again as a challenge, we classified the concern as “consistent.” We explored the consistency indicator among the concerns/challenges that were either mentioned by at least 10% of the students.
5 Results
5.1 Study 1a
5.1.1 RQ1: What Concerns Did Students Have about Their Introductory Statistics Course?
Overall, six concerns were mentioned by at least 10% of students: R coding, understanding concepts, workload, lack of prior knowledge, time management, and performance (see , column “overall”).
Table 2 Frequency (in %) of reported concerns overall and broken down by pre-/mid-COVID cohorts, gender, and URM (Study 1b, n 1417).
5.1.2 RQ2: Did Concerns Differ Based on Student Demographic Characteristics?
Gender
The frequencies by gender (male, female, nonbinary) are reported in the gender column in . Due to the small sample of nonbinary students these students were omitted from analyses. Chi-Square tests of independence were performed to compare female and male students on each of the six concerns mentioned most frequently by the full sample. The following four were significantly more frequently mentioned by females than they were by males: R coding ( (1, N = 1238)
5.461, p = 0.019), understanding concepts (
(1, N = 1238)
18.845,
), lack of prior knowledge (
(1, N = 1238)
12.833,
), and time management (
(1,
)
). There were no significant gender differences for workload and performance. Further, there was a significant difference in the frequency of mentioning no particular concern (
(1, N = 1238)
,
), with 30.9% of male students indicating that they had no particular concerns about the course at the outset of the term, compared to 11.7% of females reporting that they had no concerns about the course.
URM status
Among the six concerns mentioned most frequently by the full sample, three were significantly more frequently mentioned by URM students than they were by non-URM students: understanding concepts ( (1, N = 1237)
, p = 0.005), time management (
(1, N = 1237)
, p = 0.012), and performance (
(1, N = 1237)
). There were no differences by URM status for R coding, prior knowledge, and time management.There were no significant differences in the frequency of students specifically mentioning no particular concerns about the course at the outset of the term between non-URM (23.0%) and URM (20.1%) students (
(1, N = 1239)
, p = 0.254).
5.1.3 RQ3: Did Concerns Vary Depending on Whether Students Took the Course before or during the Pandemic?
Overall, each of the six concerns most frequently mentioned overall were—descriptively—mentioned less frequently during the pandemic than before the pandemic (see , column COVID).
A Chi-Square Test of Independence was performed to assess differences in the proportion of mentioned concerns between the two cohorts on the six most frequently mentioned concerns. There was a significant relationship between cohorts on R coding, ( (1, N = 1268)
), understanding concepts (
(1, N = 1268)
, p = 0.003), lack of prior knowledge (
(1, N = 1268)
, p = 0.035), and time management (
(1, N = 1268)
, p = 0.005). Students who took the course pre-COVID were more likely to report R coding, understanding concepts, lack of prior knowledge, and time management as an incoming course concern compared to students who took the course during COVID. There was no difference across the cohorts in the frequency with which they mentioned workload (
(1, N = 1268)
, p = 0.138) and performance (
(1, N = 1268)
, p = 0.355).
It is worth pointing out that virtual learning (e.g., concerns related to the online/remote nature of the course and technology) was relatively low in frequency overall (5.1%), but was mentioned as a concern by 9.4% of students during COVID.
5.1.4 RQ4: Were Demographic Subgroups (i.e., Based on Gender and URM Status) Differently Affected by the Onset of the Pandemic?
Shifts in reported concerns pre-mid-COVID by gender
Among the six concerns mentioned most frequently by the full sample, we see the highest levels among female students pre COVID (see ). For both female and male students, concerns were more frequently mentioned prior to the pandemic. We see particular shifts in the frequency for female students with significant declines in the most frequently mentioned concerns regarding R coding, understanding concepts, workload, lack of prior knowledge, and time management (for detailed Chi-Square statistics, see ).
Table 3 Concerns (in %) by gender/URM and by pre-/mid-COVID cohort (Study 1a, n = 1417).
More males than females reported having no concerns about the course prior to COVID. During COVID, the percentage of males reporting having no concerns dropped, whereas it increased for female students, however, the percent of males reporting no concerns during COVID was still more than the percent of females reporting no concerns.
Shifts in reported concerns pre-mid-COVID by URM status
Among the six concerns mentioned most frequently by the full sample, we see the highest frequencies in R coding and lack of prior knowledge for non-URM students pre-pandemic, whereas we see the highest levels in understanding concepts, workload, and time management for URM students pre-pandemic. For both groups of students, we see significant declines in the frequencies from pre- to mid COVID for understanding concepts, and increases for concerns related to virtual learning (for detailed Chi-Square statistics, see ). For non-URM students, concerns related to R coding and the lack of prior knowledge were mentioned significantly less frequently during the pandemic than before the pandemic. For URM students, concerns related to workload and time management were mentioned significantly less frequently during the pandemic than before the pandemic.
Overall, non-URM students during the pandemic reported most frequently that they do not have any concerns related to the course, and the frequency for both non-URM and URM students who reported having no course concerns significantly increased for students taking the course during the pandemic.
5.2 Study 1b
5.2.1 RQ5: What Challenges Did Students Perceive as Inhibiting their Success in the Course during the Pandemic?
Four challenges were mentioned by at least 10% of students. The most frequently mentioned challenge is related to the workload of the course with over one third (35.7%) of students mentioning challenges related to workload (i.e., workload, difficulty, time required, and/or pace) as a factor inhibiting their success in the course (see for the frequencies of the most frequently mentioned concerns/challenges, and for the frequencies of all mentioned concerns/challenges). This perceived challenge was followed by virtual learning (12.8%), R coding (12.3%), and challenges with understanding concepts (9.9%).
Table 4 Frequency (in %) of mentioned concerns and perceived challenges for the subsample of students (Study 1b, ).
5.2.2 RQ6: Did Perceived Challenges Differ by Gender or URM Status?
Perceived challenges by gender
Frequencies in reported challenges by gender status are reported in column “Challenges by Gender.” We calculated Pearson’s Chi-Square tests to explore whether there were significant differences between female and male students on the four most frequently mentioned challenges, which were mentioned by at least approximately 10% of the students. Due to the small number of nonbinary students in our sample (<10), nonbinary students were not included in these group comparisons. There were no significant differences in the reported frequencies by gender. Worthy of note is that over 20% of nonbinary students reported personal struggles related to physical and mental health as perceived challenges.
Perceived challenges by URM status
Frequencies in reported challenges by URM status are reported in column “Challenges by URM.” Chi-Square tests by URM status revealed significant differences in the reported frequencies regarding R coding ( (1, n = 401)
, p = 0.016) with significantly higher frequency for non-URM students. In contrast, workload (
(1,
)
,
) was significantly more frequently mentioned as a perceived challenge by URM students.
5.2.3 RQ7: To What Degree Did Students’ Initial Concerns during the Pandemic Appear to Be Warranted? That is, were They Consistent with What They Later Perceived as Challenges?
documents the frequencies of issues that were emergent (i.e., something that was not mentioned as a concern at the start of the course but was brought up as a challenge at the end of the course), eliminated (i.e., something that was mentioned as a concern at the start of the course but not as a challenge at the end of the course) and consistent (something that was mentioned as an incoming course concern and a later perceived challenge). The frequencies with which students mentioned each concern (eliminated + consistent) and challenge (emergent + consistent) are also reported.
Table 5 Frequency (in number and %) of mentioned concerns and perceived challenges based on Consistency Indicator (Study 1b, n = 524. Note: includes only students who provided responses for both open-ended questions).
The most hopeful finding was that five concerns saw high elimination rates. For example, students who had concerns about R Coding (see R Coding row) at the beginning of the course (n = 90), 88% (n = 79) no longer perceived it as a challenge at the end of the term. Similarly, students who had concerns about understanding concepts, lack of prior knowledge, performance, and time management at the beginning of the term, three-quarters or more no longer perceived it as a challenge at the end of the term. The most discouraging finding was that two concerns saw relatively high rates of consistency and emergence. For example, among those students who expressed a concern about workload at the start of the term (n = 49), 43% (n = 21) mentioned it as a challenge at the end of the course. Furthermore, 31% (n = 123) did not mention workload at the start of the term but mentioned it at the end. Among students who expressed a concern about virtual learning at the start of the term, 42% mentioned it as a challenge at the end of the course. Furthermore, 10% of students mentioned virtual learning at the end of the term though they hadn’t mentioned it at the start of it. Mixed results were found for R coding concerns. There was a high elimination rate for this concern, with 88% of students who expressed R coding as a concern at the start of the term but not mentioning it as a challenge at the end of the term. However, 10% of students did not mention R coding at the start of the term but did mention it at the end.
5.2.4 RQ8: Did the Degree of Consistency between Concerns and Challenges Vary by Gender or URM Status?
documents the frequencies by gender and URM status of emergent, eliminated, and consistent issues. The frequencies with which students mentioned each concern (eliminated + consistent) and challenge (emergent + consistent) are also reported. The patterns of concerns by gender and URM were similar to the overall patterns described above.
Table 6 Frequency of mentioned concerns and perceived challenges based on Consistency Indicator by Gender and URM (Study 1b, n = 524. Note: includes only students who provided responses for both open-ended questions).
6 Discussion
In this study, we sought to explore qualitative student responses regarding incoming course concerns and later perceived course challenges in introductory statistics and (a) how the frequency in concerns varied between female and male students, URM and non-URM students, and between students who took the course prior to the pandemic and during it; and (b) how perceived challenges varied by gender and URM status, and whether students’ initial concerns were consistent with what they later reported as challenges. We identified a wide range of course concerns and perceived course challenges that may be leveraged to improve undergraduates’ experiences in introductory statistics courses.
There are eight main takeaways from our findings: (a) coming into the course, students were concerned about R coding, understanding concepts, workload, prior knowledge, time management, and performance; (b) female students and URM students reported being more concerned than their counterparts; (c) course-related concerns were more frequently mentioned prior to the pandemic, whereas certain concerns that were most directly related to the pandemic (e.g., virtual learning and inaccessibility of resources) were more frequently mentioned during the pandemic; (d) there were differences in reported concerns between students who were enrolled in the course pre- versus mid-pandemic, by gender, and by URM status, (e) at the end of the course, students perceived the workload, virtual learning, R coding, and understanding concepts as the most challenging components of the course; (f) although there were no differences in perceived challenges by gender, URM students reported being more challenged by workload, whereas non-URM students reported being more challenged by the R coding component; (g) overall, there were relatively low rates of consistency of concerns over time, that is, students’ incoming course concerns were not consistent with what they later perceived as actual challenges; most concerns diminished substantially over time; (h) rates of consistency between concerns and challenges did not vary by gender or URM status.
6.1 Incoming Course Concerns in Introductory Statistics
Undergraduate students who were enrolled in the introductory statistics course prior to and during COVID expressed a wide range of concerns coming into the course. The most frequently mentioned concerns were directly related to the components of the course itself, such as R coding, understanding concepts, workload, prior knowledge, time management, and performance. Some of these commonly mentioned concerns included areas that “may be actionable by the instructor, such as those related to course structure” (Meaders et al. Citation2020, p. 210), including concerns related to workload (i.e., pace of the class). Other commonly mentioned concerns were related to the students themselves and their incoming preparation, knowledge, and skills (e.g., having the necessary knowledge or experience to succeed in the course) or study strategies (e.g., time management), which may indicate topics that could be addressed at the start of the course.
6.2 Differences in the Frequency of Reported Concerns by Gender, URM, and COVID Cohort
Our findings regarding incoming course concerns in introductory statistics adds to the increasing list of differences between how female versus male students and URM versus non-URM students experience STEM courses (see Meaders et al. Citation2020; Barber et al. 2021; Hartzell, Hagen, and Devereux Citation2021).
In our study, among the concerns most frequently mentioned by the full sample, there were differences by gender and URM, with females and URM students being more concerned than their counterparts about understanding concepts and time management. Furthermore, females were more concerned than were males about R coding and lack of prior knowledge. Understanding concepts and time management were more frequently mentioned by URM students than they were by non-URM students.
Understanding the experiences of students who are traditionally underrepresented in STEM fields (including statistics) and how they differ from their counterparts is important in the design of learning contexts that better support these students. For example, next steps could include embedding interventions or additional supports for lowering concerns among female students related to R coding and lack of prior knowledge through messaging (e.g., highlighting that the textbook is set up for all students to succeed, regardless of their coding/programming background). Future research includes investigating why these differences emerge: What mechanisms lead to female students’ feeling more concerned about R coding and lack of prior knowledge or URM students being more concerned about understanding concepts, time management, and performance? STEM learning environments often result in lower performance for students who identify with underrepresented and traditionally marginalized and minoritized groups, including women, Black, Latinx, and Indigenous American students (Wang and Degol Citation2013; Riegle-Crumb, King, and Irizarry Citation2019; Fong et al. Citation2021). Several contextual factors exist that contribute to these differences including societal stereotypes about who will succeed in STEM fields and a curriculum that emphasizes more masculine and Caucasian values (Rosenzweig and Wigfield Citation2016). Exploring stereotype threat within the context of the same introductory statistics course could potentially shed light onto the question why female and URM students tend to be more concerned as they enter the introductory statistics course, which will be key in designing interventions.
Interestingly, the most common concerns were more frequently mentioned by students who were enrolled in the course prior to the pandemic than by students who were enrolled in the course during the pandemic. Consistent with this, more students during the pandemic than before it stated that they had no concerns. There are ways that the pandemic might have had a positive impact on students. What Blankstein, Frederick, and Wolff-Eisenberg (Citation2020) saw as disconnection from peers might have played out in our sample as less concern for social comparison. Both positive and negative aspects of proximity to peers were likely reduced by the pandemic.
While it was somewhat unexpected to see fewer concerns mid-COVID than pre-COVID, it must be considered that although the frequency of certain concerns may have gone down, this does not necessarily mean that the magnitude of concern also went down. While we consider the open-ended question a strength of our study, we acknowledge that it does not measure the magnitude of students’ concerns. Further, with the onset of the pandemic, fewer concerns were reported that were related to the course itself. Most likely, priorities changed, making concerns directly tied to the course (e.g., R coding, understanding concepts, lack of prior knowledge) seem insignificant compared to other issues students were contending with (e.g., sickness and deaths of friends or family, loss in family income, mental health issues). It may have been that these bigger issues overshadowed course-specific concerns. Concerns that one would expect to be mentioned more frequently during the pandemic because of their close relation to it—virtual learning and inaccessibility of resources—were indeed (descriptively) more frequently mentioned during the pandemic. However, they were still mentioned by less than 10% of the students.
Several contextual factors must be kept in mind. First, the open-ended question was not specifically designed to capture struggles related to COVID. There is a high likelihood that students may have experienced a wide range of other concerns which they did not report during the survey, as (a) the question was phrased relatively broadly, to capture concerns related to the course (i.e., course-based concerns) and (b) students might not have thought that this was the place for them to voice (personal) concerns outside of the course. Nevertheless, as seen in this study, students still reported concerns (and challenges) outside of the course, such as external obligations.
Second, it is important to consider the greater context regarding the timing of the surveys. COVID was also the time of Black Lives Matter protests, political polarization, and exhaustion. We chose to define this period in relation to COVID because it was the reason that the course was delivered in a different format, but it has to be kept in mind that this phase in history is multi-faceted and defined not by the pandemic alone.
Third, it is important to keep in mind that students in this sample were from one selective, predominantly White/Asian institution. It is very likely that those students have different concerns than do students from different institutions. For example, students in our sample did not report concerns related to financial resources or the lack thereof. However, there is a possibility that this concern would have come up—particularly during the pandemic—among students from other institutions. Thus, it is crucial for future research to explore “concerns of students at other institution types to capture the variety of concerns and assess if different interventions are required to provide those students with positive course experiences” (Meaders et al. 2021, p. 212).
Finally, we did not differentiate between students who took the course at the beginning of the pandemic (i.e., Spring 2020) and students who took the course later. The latter may have been more accustomed to remote/online learning. They might also have suffered more from an accumulation of social deprivation and Zoom fatigue.
6.3 Differences in the Frequency of Reported Concerns between Pre-/Mid-Pandemic Concerns and Either Gender or URM Status
When looking at COVID by gender, for each of the six most frequently mentioned concerns, the highest frequency appeared among females before COVID. The finding that female students reported higher levels of concern than did male students is consistent with prior work (Meaders et al. Citation2020). When looking at COVID by URM status, for four of the six most frequently mentioned concerns (i.e., understanding concepts, workload, time management, and performance), the highest frequency appeared among URM students before COVID. That URM students mentioned performance as a concern more frequently than did non-URM students—both prior and during the pandemic—stands in contrast to prior studies. For example, Hartzell, Hagen, and Devereux (Citation2021) found that majority students were more concerned with the impacts of the pandemic on their performance and GPA than were URM students. While the frequency of reporting performance as a course concern decreased from roughly 18% to 14% for URM students, they still reported performance as a concern more frequently than non-URM students. As mentioned previously, it will be crucial for future research to explore concerns mentioned by URM students at different institutions, as it is likely that the concerns will vary considerably and it will be important to design interventions that target the mechanisms causing these differences.
As mentioned above, we can’t say with certainty that course concerns went away with COVID. It may have been that the things that were concerning prior to the pandemic were less salient to students when they went remote and they took a back seat to concerns both in and outside of class, related to the pandemic. However, if the reduction in the levels of students’ concerns during the pandemic were real, that is, that reports of concerns went down because students were more hopeful about the course rather than because they had bigger things to worry about than a class, then “returning to normal” makes addressing pre-pandemic concerns particularly important. “Back to normal” might mean “back to not great.”
6.4 Perceived Challenges in Introductory Statistics and Differences by Gender and URM
At the end of the course, four challenges were mentioned by at least 10% of students: workload, virtual learning, R coding, and challenges with understanding concepts. Two concerns that were not mentioned very frequently as an incoming course concern saw an increase in frequency as a perceived course challenge, namely external obligations and pandemic related course challenges.
URM students reported being more challenged than their non-URM peers by workload, whereas non-URM students reported being more challenged than their URM peers by R coding. Similar to prior research documenting that URM and majority students were as likely to struggle with internet access (Hartzell, Hagen, and Devereux Citation2021), URM and non-URM students in our sample reported similarly frequently that the virtual component of the course (which includes issues with technology) was a barrier in them succeeding in the course. There were no differences in perceived challenges by gender. Interestingly, prior research has revealed that “as courses increase in their percentage of female students, levels of mid-semester concern decrease for all students and at a higher rate for female students” (Meaders et al. Citation2020, p. 211). Similar to what Meaders et al. (Citation2020) found, we found differences between female and male students regarding their incoming course concerns, but not their later reported perceived challenges, which could indicate that as the course advances, classroom environments and thus the perceptions, expectations, and experiences of female students improve at a more rapid rate compared to males, thus, closing the gender gap.
6.5 Incoming Course Concerns and Later Perceived Challenges and Patterns by Gender and URM
When exploring shifts in the reported frequency of incoming course concerns versus later perceived challenges, we found relatively low rates of consistency with concerns about understanding concepts, lack of prior knowledge, performance, or time management dramatically reduced by the end of the term. One potential explanation lies in the design of the textbook, which was used by all students. The textbook (i.e., the base of the introductory statistics course) was intentionally designed for all students, regardless of prior background in programming, with the main aim of promoting deep learning, understanding, and engagement with the course material and a focus on making real life connections. Thus, while students may initially enter the course with concerns regarding the R-coding aspect of the course, lack of prior knowledge, memorization, or understanding concepts, they soon realize that, for example, prior knowledge or memorizing codes/concepts is not necessary in order to succeed in the course. Thus, one potential explanation for the lack of consistency in these components being mentioned as an incoming course concern versus an actual perceived challenge by the end of the course is that the textbook does a good job at introducing statistical concepts and R programming for all students. Less encouragingly, the issue of workload had both the highest rate of consistency and the highest rate of emergence. The concern for virtual learning showed a similar pattern, though to a lesser degree. Findings related to R concerns were mixed, with a large reduction in frequency among students who had the concern at the start of the course, but also a relatively high rate of emergence among those who did not. Rates of consistency between concerns and challenges did not vary by gender or URM status.
6.5.1 Strengths, Limitations, and Areas for Future Research
This exploratory study contributes to understanding students’ expectations, experiences, and perceptions in introductory statistics and how those experiences vary by gender, URM, and COVID cohorts. The strengths of our study are 3-fold: First, we used an open-ended survey question to explore students’ incoming course concerns, allowing us to (a) capture more nuanced aspects of their concerns and (b) identify concerns that we would not have been able to capture using closed-ended questions or that we would not have expected a priori. Second, we included data from students enrolled in an introductory statistics course prior to and during the COVID-19 pandemic, providing us with unique insights into how concerns differ across those cohorts. Finally, beyond students’ incoming course concerns at the outset of the course, we asked them about their perceived challenges when looking back at the course allowing us to explore whether their early concerns came to fruition. These strengths allow us to (a) help identify particular pain points among students and within the curriculum, (b) help instructors allay students’ unfounded fears, and (c) guide instructional designers and policymakers as they develop interventions. For example, for students indicating that they had concerns about lack of prior knowledge, skills, or R coding experience, an instructor might verbally emphasize that prior experience is not required, ensuring that all students independent of their background and experience can be successful in this course. Additionally, for students who are concerned about memorizing R codes or concepts, the instructor could demonstrate how to access the R code “cheatsheet” available in the course, and demonstrate in lecture the use of the cheatsheet when writing code. Connecting our understanding to other theoretical work would further inform these applications.
Despite these strengths, several limitations and areas for future research must be acknowledged. First and foremost, the findings of our study are not generalizable to other student populations. As discussed earlier in the discussion, all participants were from one predominantly White/Asian university in California. Student perceptions, expectations, and experiences are highly situational and may vary significantly from one institution to another and for example, female and underrepresented minority students’ experiences may depend on the proportion of female or underrepresented minority students within their institution. It will be vital to explore concerns and challenges of students at other institutions, characterized by different student body demographics and different types of institutions (e.g., community colleges).
Second—and related to our first limitation—the findings are specific to the textbook used by all students. While we consider it a strength of our study that all students used the same textbook as it reduces variability in the course content and thus the content study participants were exposed to, we must acknowledge the likelihood that, for example, students’ perceived challenges at the end of the course are highly specific to the content of said textbook. While this is of value for the course and curriculum developers as it helps in identifying appropriate interventions or instructional practices supporting students’ needs, which can be embedded directly in the textbook to increase students’ experiences, these implications might not apply well to other settings. Future work should also investigate the concerns and perceived challenges of students enrolled in introductory statistics courses that do not use the same textbook.
Third, our analyses do not consider the hierarchical structure of the data (i.e., students clustered within different course sections or students clustered within different majors). Future research could make use of more advanced models to take the hierarchical nature of the course structure into account.
Finally, while we explored concerns among students who took the course prior to and mid pandemic, we did not differentiate within the “mid pandemic” cohorts. It is possible that concerns and experiences among students who took the course right at the onset of the pandemic were different from the experiences among students who had already been accustomed to online/remote learning.
We began data collection for this study before the onset of the COVID-19 pandemic and continued data collection during it. Now, as we reflect on our findings, we are again in a time of transition, with students and their instructors returning to some degree of normality. How much the “new normal” is similar to students’ experiences pre-COVID and how much students’ mid-COVID experiences persist remains to be seen.
Supplemental Material
Download MS Word (25.2 KB)Supplementary Materials
The supplement consists of (1) the Final Coding Scheme, (2) a table documenting differences in pre-/mid-COVID concerns by gender and URM (3) a table documenting the frequency of mentioned concerns and perceived challenges for the subsample of students (Study 1b, ), and (4) all data and code used to perform all analyses presented in this paper.
Disclosure Statement
The authors report there are no competing interests to declare.
Funding
Additional information
Funding
Notes
1 This was an exploratory study, with little available in the way of prior research, especially as it relates to students’ experiences during the COVID pandemic. In light of this, we did not seek to confirm hypotheses and we did not adjust for p-values for multiple comparisons. The reader should take this into account, as we do, when interpreting results.
2 We did not adjust for p-value for multiple comparisons, thus, the reader should take into account the issue of multiple comparisons.
References
- Arcidiacono, P., Aucejo, E. M., and Hotz, V. J. (2016), “University Differences in the Graduation of Minorities in STEM Fields: Evidence from California,” The American Economic Review, 106, 525–562. 10.1257/aer.20130626.
- Barber, P. H., Shapiro, C., Jacobs, M. S., Avilez, L., Brenner, K. I., Cabral, C., Cebreros, M., Cosentino, E., Cross, C., Gonzalez, M. L., Lumada, K. T., Menjivar, A. T., Narvaez, J., Olmeda, B., Phelan, R., Purdy, D., Salam, S., Serrano, L., Velasco, M. J., Marin, E. Z., and Levis-Fitzgerald, M. (2021), “Disparities in Remote Learning Faced by First-Generation and Underrepresented Minority Students During COVID-19: Insights and Opportunities From a Remote Research Experience,” Journal of Microbiology & Biology Education, 22, 1–25. DOI: 10.1128/jmbe.v22i1.2457.
- Blankstein, M., Frederick, J., and Wolff-Eisenberg, C. (2020), “Student Experiences during the Pandemic Pivot”, DOI: 10.18665/.sr.313461.
- Brewer, C. L., Hopkins, J. R., Kimble, G. A., Matlin, M. W., McCann, L. I., McNeil, O. V., Nodine, B. F., Quinn, V. N. and Saundra. (1993), “Curriculum”, In Handbook for Enhancing Undergraduate Education in Psychology, eds. T. V. McGovern, pp. 161—-182, Washington, D.C: American Psychological Association, DOI: 10.1037/10126-006.
- Camilli, G., and Hira, R., (2018), “Introduction to Special Issue—Stem Workforce: STEM Education and the Post-Scientific Society”, Journal of Science Education and Technology, 28, 1–8. DOI: 10.1007/s10956-018-9759-8.
- Cataldi, E. F., Bennett, C. T., and Chen, X. (2018), “First-Generation Students: College Access, Persistence, and Post-Bachelor’s Outcomes,” National Center for Education Statistics. Available at https://nces.ed.gov/pubs2018/2018421.pdf
- Fong, C. J., Kremer, K. P., Cox, C. H. T., and Lawson, C. A. (2021), “Expectancy-value Profiles in Math and Science: A Person-centered Approach to Cross-domain Motivation with Academic and STEM-related Outcomes,” Contemporary Educational Psychology, 65, 101962. DOI: 10.1016/j.cedpsych.2021.101962.
- Friedrich, J., Buday, E., and Kerr, D. (2000), “Statistical Training in Psychology: A National Survey and Commentary on Undergraduate Programs,” Teaching of Psychology, 27, 248–257. DOI: 10.1207/s15328023top2704_02.
- Gal, I., and Ginsburg, L. (1994), “The Role of Beliefs and Attitudes in Learning Statistics: Towards an Assessment Framework,” Journal of Statistics Education, 2, 1–16. DOI: 10.1080/10691898.1994.11910471.
- Garfield, J., and Ben-Zvi, D. (2007), “How Students Learn Statistics Revisited: A Current Review of Research on Teaching and Learning Statistics,” International Statistical Review, 75, 372–396. DOI: 10.1111/j.1751-5823.2007.00029.x.
- Graham, M. J., Frederick, J., Byars-Winston, A., Hunter, A.-B., and Handelsman, J. (2013), “Increasing Persistence of College Students in STEM,” Science (New York, N.Y.), 341, 1455–1456. 10.1126/science.1240487.
- Gray, D. L., Hope, E. C., and Matthews, J. S. (2018), “Black and Belonging at School: A Case for Interpersonal, Instructional, and Institutional Opportunity Structures,” Educational Psychologist, 53, 97–113. DOI: http://dx.doi.org/10.1080/00461520.2017.1421466.
- Griffith, A. L. (2010), “Persistence of Women and Minorities in STEM Field Majors: Is it The School That Matters?,” Economics of Education Review, 29, 911–922. DOI: 10.1016/j.econedurev.2010.06.010.
- Hartzell, S. Y., Hagen, M. M., and Devereux, P. G. (2021), “Disproportionate Impacts of COVID-19 on University Students in Underrepresented Groups: A Quantitative and Qualitative Descriptive Study to Assess Needs and Hear Student Voices,” Journal of Higher Education Management, 36, 144–153.
- Hurtado, S., Newman, C. B., Tran, M. C., and Chang, M. J. (2010), “Improving the Rate of Success for Underrepresented Racial Minorities in STEM Fields: Insights from a National Project,” New Directions for Institutional Research, 2010, 5–15. DOI: 10.1002/ir.357.
- Kosovich, J. J., Flake, J. K., and Hulleman, C. S. (2017), “Short-Term Motivation Trajectories: A Parallel Process Model of Expectancy-Value,” Contemporary Educational Psychology, 49, 130–139. DOI: http://dx.doi.org/10.1016/j.cedpsych.2017.01.004.
- Lazarus, R. S., and Folkman, S. (1984), Stress, Appraisal, and Coping, New York: Springer.
- McGovern, T. V., Furumoto, L., Halpern, D. F., Kimble, G. A., and McKeachie, W. J. (1991), “Liberal Education, Study in Depth, and the Arts and Sciences Major: Psychology,” American Psychologist, 46, 598–605. DOI: 10.1037/0003-066x.46.6.598.
- Meaders, C. L., Lane, A. K., Morozov, A. I., Shuman, J. K., Toth, E. S., Stains, M., Stetzer, M. R., Vinson, E., Couch, B. A., and Smith, M. K. (2020), “Undergraduate Student Concerns in Introductory STEM Courses: What they are, How they Change, and What Influences Them,” Journal for STEM Education Research, 3, 195–216. DOI: 10.1007/s41979-020-00031-1.
- Meaders, C. L., Smith, M. K., Boester, T., Bracy, A., Couch, B. A., Drake, A. G., Farooq, S., Khoda, B., Kinsland, C., Lane, A. K., Lindahl, S. E., Livingston, W. H., Bundy, A. M., McCormick, A., Morozov, A. I., Newell-Caito, J. L., Ruskin, K. J., Sarvary, M. A., Stains, M., St. Juliana, J. R., Thomas, S. R., van Es, C., Vinson, E. L., Vitousek, M. N., and Stetzer, M. R. (2021), “What Questions Are on the Minds of STEM Undergraduate Students and How Can They be Addressed?,” Frontiers in Education, 6, 1–8. DOI: 10.3389/feduc.2021.639338.
- Murphy, L., Eduljee, N. B., and Croteau, K. (2020), “College Student Transition to Synchronous Virtual Classes during the COVID-19 Pandemic in Northeastern United States,” Pedagogical Research, 5, em0078. DOI: 10.29333/pr/8485.
- Panadero, E. (2017), “A Review of Self-Regulated Learning: Six Models and Four Directions for Research,” Frontiers in Psychology, 8, 422. 10.3389/fpsyg.2017.00422.
- Pintrich, P. R. (2004), “A Conceptual Framework for Assessing Motivation and Self-Regulated Learning in College Students,” Educational Psychology Review, 16, 385–407. DOI: 10.1007/s10648-004-0006-x.
- Riegle-Crumb, C., King, B., and Irizarry, Y. (2019), “Does STEM Stand Out? Examining Racial/Ethnic Gaps in Persistence across Postsecondary Fields,” Educational Researcher, 48, 133–144. DOI: 10.3102/0013189X19831006.
- Rosenzweig, E. Q., Hulleman, C. S., Barron, K. E., Kosovich, J. J., Priniski, S. J., and Wigfield, A. (2019), “Promises and Pitfalls of Adapting Utility Value Interventions for Online Math Courses,” The Journal of Experimental Education, 87, 332–352. DOI: http://dx.doi.org/10.1080/00220973.2018.1496059.
- Rosenzweig, E. Q., and Wigfield, A. (2016), “STEM Motivation Interventions for Adolescents: A Promising Start, but Further to Go,” Educational Psychologist, 51, 146–163. DOI: 10.1080/00461520.2016.1154792.
- Son, J. Y., and Stigler, J. W. (2017–2022), Statistics and Data Science: A Modeling Approach, Los Angeles: CourseKata. https://coursekata.org/preview/default/program. Currently available in 5 versions.
- Soria, K. M., Horgos, B., Chirikov, I., and Jones-White, D. (2020), “First-Generation Students’ Experiences During the COVID-19 Pandemic”, Student Experience in the Research University (SERU) Consortium. Retrieved from the University of Minnesota Digital Conservancy, https://hdl.handle.net/11299/214934.
- Wang, M. T., and Degol, J. (2013), “Motivational Pathways to STEM Career Choices: Using Expectancy–Value Perspective to Understand Individual and Gender Differences in STEM Fields,” Developmental Review, 33, 304–340. DOI: 10.1016/j.dr.2013.08.001.
- Xue, Y., and Larson, R. (2015), “Stem Crisis or Stem Surplus? Yes and Yes,” Monthly Labor Review, 1–16. DOI: 10.21916/mlr.2015.14.
- Zabaleta, F. (2007), “The Use and Misuse of Student Evaluations of Teaching,” Teaching in Higher Education, 12, 55–76. DOI: 10.1080/13562510601102131.
- Zimmerman, B. J. (2001), “Theories of Self-Regulated Learning and Academic Achievement: An Overview and Analysis,” in Self-Regulated Learning and Academic Achievement (2nd ed.), eds. B. J. Zimmerman and D. H. Schunk, 1–35. Mahwah, NJ: Erlbaum.