ABSTRACT
Peck caused by stink-bug is an important quality factor for rice grading, marketing, and end-use. The current visual method of separating pecky rice kernels from sound kernels is time consuming, tedious, and subject to error. The objective of this research was to develop an objective method for classifying pecky rice kernels and sound rice kernels using visible and near-infrared (NIR) spectroscopy. A diode-array NIR spectrometer, which measured absorbance spectra (log(1/R)) from 400 to 1700 nm, was used to differentiate pecky rice kernels and sound rice kernels individually. Partial least squares (PLS) regression models with three wavelength regions (400–750, 400–1700, and 750–1700 nm) and two-wavelength models were developed. Results showed that both PLS models and two-wavelength models can be used to classify pecky rice kernels. For PLS models, the NIR wavelength region of 750–1,700 nm gave the highest percentage of correct classification for both calibration and validation (100%). For two-wavelength models, the model using wavelengths 480 and 590 nm yielded the highest classification accuracies for both calibration and validation sample sets (99.3%). Stink-bug feeding caused microorganism activity resulting in grain discoloration. The color change made the classification of pecky rice and sound rice kernels possible (accuracy>99%) even when the undamaged area faces the illumination light.
INTRODUCTION
In rice, peck damage is one of the most important quality factors for grading, marketing, and determining end-use. The rice stink-bug, Oebalus pugnax, is a key pest that causes peck damage. It attacks the crop from heading to harvest.Citation[1] The insect inserts it mouthparts into the kernel and consumes the contents. This feeding also causes microorganism activity that results in a discolored area on the grain.Citation2-5 Pecky rice is characterized as a roughly circular lesion, which in some cases appears as a shrunken area. In general, pecky rice kernels are accompanied by discoloration, usually a brown to black discoloration of the whole kernel or portion of the kernel, or a black linear discoloration. Peck damage causes both yield and quality losses that affect rice end-use.Citation6-8
Pecky grains weigh substantially less than normal grains since they are not fully developed or are damaged by fungus which results in a yield loss. Based on an average of 2.7% peck, head rice yield was reduced by 3.8%.Citation[9] Pecky kernels break more easily than undamaged kernels during milling, which may result in a low quality grade depending on the proportion of damaged to undamaged kernels. The U.S. rice grading standard for brown rice classifies pecky rice as damaged kernels. The maximum limit for damaged kernels and red rice combined are 1, 2, 4, 8, and 15% for U.S. No. 1, 2, 3, 4, and 5 designation.Citation[10] It was reported by Warren et al.Citation[11] that in the U.S. market, only whole kernels, red rice, weed seed, and peck were significant quality factors, and that the impact of peck damage is much greater than red rice or weed seed. Peck damage also affects the end-use of rice, especially for the parboiled rice industry. Pecky rice is a severe problem in this industry, since the parboiling process tends to intensify color development in the damaged grains, and most of these grains must be removed before use. Parboilers usually have their own requirements on the allowable level of pecky rice in rice purchased for parboiling. This tolerance is very low because of the additional expenses for removing pecky rice from the parboiled product.Citation[8]
The Grain Inspection, Packers and Stockyard Administration (GIPSA) and local elevators use specific criteria to visually determine pecky rice from sound rice. The rice inspector must be trained to recognize various types of damage that may occur in rice. Also, they must have an ability to judge each instance where the damage is sufficient to be considered below the standard.Citation[10] The inspection often involves hand-picking, separation, and counting. This process is slow and tedious. Therefore, an objective and quick means of classifying pecky rice kernels from sound rice kernels is needed.
Near-infrared (NIR) spectroscopy has been used to measure physicochemical properties of rice, such as moisture content,Citation12-13 protein content,Citation12-14 amylose content,Citation14-16 whiteness,Citation14-15 milling degree,Citation14-16 and cooking quality.Citation13-17 No research has been published on the use of NIR technology to classify pecky rice kernels from sound kernels. Typically, quality factors of rice were measured using bulk or ground samples. However, quantifying the percentage of damaged rice kernels is not possible using bulk or ground samples. The objective of this research was to classify single pecky rice kernels and sound rice kernels using NIR spectroscopy.
MATERIALS AND METHODS
Rice Sample
Brown rice samples were obtained from Jerry Scarver Uncle Ben's Inc. (Greenville, MS). Rice samples were separated into four categories based on proportion and location of peck on the kernels. Categories were sound kernels, large damaged kernels, tail damaged kernels, and middle damaged kernels. Kernels were classified as large damaged kernels when the length of a pecky area covered more than half of the kernel. Tail damaged kernels were defined as those kernels where a peck was located on the tail and the area of the peck was less than half of the kernel. Middle damaged kernels were those where the damaged area was located on the middle portion of the kernel and the area of the peck was less than half of the kernel.
Kernel Color Measurement
The reflectance spectra at 480–750 nm were transferred into L* a* b* color space and the software package Grams/32 (Galactic Industries, Salem, NH) was used for kernel color determination. In the L* a* b* color space, L* varies from 0 (black) to 100 (perfect white); a* ranges from −100 to 100 and measures green when negative and red when positive; and b* varies from −100 to 100, is a measure of blue when negative and yellow when positive.
Spectra Collection
Spectra from pecky and sound single kernels were collected using a diode-array NIR spectrometer (DA7000, Perten Instruments, Springfield, IL). This spectrometer measures visible (400–750 nm) and NIR (750–1700 nm) reflectance at a rate of 30 spectra per second. Rice kernels were individually placed in a black V-shaped trough (12 mm long, 10 mm wide, and 5 mm deep) and illuminated with a quartz tungsten halogen light via a fiber bundle (8 mm diameter) positioned 13 mm from the top of the trough and oriented 45° from vertical. The reflectance probe (2-mm diameter) was oriented vertically 9.5 mm from the top of the trough. The reflectance probe carried the reflectance energy to a spectrometer. A total of 1200 brown rice kernels were measured. For each kernel, 8 spectra were collected and averaged. The wavelength regions 500–750, 750–1700, and 500–1700 nm were used to differentiate pecky and sound rice kernels. A spectrum of the empty trough, used as a baseline, was measured at the beginning of the test and again after every 100 kernels.
Statistical Analysis and Model Development
Spectra were analyzed using partial least squares (PLS) regression from the software package Grams/32. All spectra were mean centered before analysis. Mean centering removes the most common variation from the data set and scales the data set so that the mathematics of the spectral decomposition and correlation perform better. This involves calculating the average of all the spectra in the training set and then subtracting the result from each spectrum. PLS is a multivariate data analysis technique designed to handle intercorrelated regressors. Two-class models were developed to classify pecky and sound rice kernels. The kernels were separated equally into calibration and validation sets based on even and odd numbers. Pecky rice kernels and sound kernels were assigned dummy values of 1 and 2, respectively. A kernel was considered to be categorized correctly if the predicted value lay on the same side of the midpoint of the assigned values. Model performance is reported as the multiple coefficient of determination (r 2), standard error of cross validation (SECV) of each calibration, and standard error of prediction (SEP) of each validation sample set. The number of PLS factors reported were the minimum required to give the high percentage of correct classification.
The SAS DISCRIM procedureCitation[18] was used to develop linear discriminant functions based on two wavelengths (log 1/R values) for classifying pecky rice and sound rice. The two-wavelength models were identified using stepwise and forward regression (STEPWISE and MAXR methods) of the entire wavelengths and regression of the best subsets of log(1/R) data by the RSQUARE method. The STEPWISE and MAXR methods use forward selection to select a subset of the wavelengths or a best-two wavelength model for classifying each spectrum into one of the classes. The wavelengths were chosen to enter or leave the model according to the significance level of an F-test. The MAXR method performs all possible subset regressions and rank models according to r 2 for each number of variables. In consideration of bandwidths of filters that may be 40–50 nm, only wavelength pairs that are at least 100 nm away were considered for the discriminant analysis.
RESULTS AND DISCUSSION
Classification of Pecky Rice and Sound Rice by PLS
The classification results for calibration sample sets and prediction of validation sample sets are summarized in Table . The NIR wavelength region of 750–1700 nm gave the highest percentage (100%) of correct classification for both calibration and validation. The visible wavelength region of 500–750 nm and NIR region (500–1700 nm) gave similar percentages of correct classification. Peck orientation and location had a slight effect on the classification. The greatest percentage of correct classifications (100%) was obtained when a peck area faced the illumination light. The most misclassified kernels were those with the discoloration or peck on the center of the kernel. Results showed that classifying pecky rice and sound rice kernels is possible even when the undamaged area faces the illumination light because stink-bug feeding also causes microorganism activity, resulting in grain discoloration. For PLS models, the number of PLS factors increased as the wavelength region increased. For practical application, the model with fewer PLS factors and using the visible region will allow for differentiation of pecky and sound rice kernels.
Table 1. Summary of Calibration and Validation Results Using PLS Models for Classifying Pecky and Sound Rice Kernelsa
Figure shows the average spectra of pecky and sound rice kernels. In general, the energy absorption of pecky rice kernels was higher in the visible region and lower in the NIR region than that of sound rice kernels. The differences in energy absorption between pecky rice and sound rice kernels indicate differences in color and chemical composition. The greater energy absorption of pecky rice kernels indicates that the color of pecky rice kernels was darker than sound kernels. This is expected because pecky rice kernels are usually accompanied with discoloration. The color differences between pecky and sound rice kernels were proven by L, a, and b values in L*a*b color space (Table ). The a and b values of the sound rice kernels were significant lower than pecky rice kernels. This indicates that pecky rice kernels are darker and more yellow than sound rice kernels.
Table 2. The Color Variations Between Pecky and Sound Rice Kernels Measured as L, a, and b Values in the L*a*b Color Space
Figure 1. Absorption curves for pecky and sound rice kernels. Vertical bar represents one standard deviation.
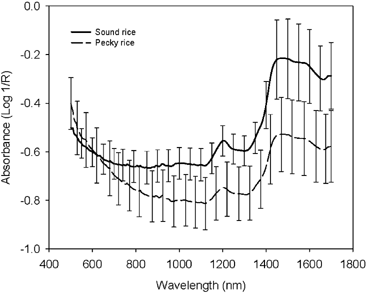
The energy absorption of pecky rice kernels was less than that of sound kernels over the entire NIR wavelength region (Fig. ). This probably was due to the differences in chemical composition and light scattering between pecky and sound rice kernels. The peaks and valleys of the Beta coefficient curve from PLS model show the significant differences in energy absorption between the pecky and sound rice kernels (Fig. ). The peaks between 500 to 600 nm and around 770 nm are related to color difference. The peaks around 1270, 1515, and 1400 nm are related to fiber and starch content.Citation19-20 The pecky rice may have less starch content than sound rice kernels because pecky rice kernels have less weight and more fiber at the damaged area. The peak around 1570 nm represents protein content.Citation[19] Some peaks and valleys in the Beta coefficient curve may represent interactions of moisture, starch, protein, oil, and cellulose caused by peck damage in the rice kernels.
Classification of Pecky Rice and Sound Rice by Two-Wavelength Model
Stepwise and best-subsets regression identified the wavelengths 480 and 875 nm as promising in the visible and NIR regions, respectively, for pairing with some other wavelengths in the 480–1700 nm range. Figure shows that the r 2 of the classification models with wavelengths 480 or 875 nm decreasing as wavelengths increased. This may indicate that more spectral information for classification lay on the visible region than on the NIR region. The classification accuracies for selected wavelength pairs are shown in Table . The classification accuracies for the 480 nm and 590 nm model were comparable to the full-wavelength PLS model in Table . The average classification accuracy was 99.3% for both calibration and validation sample sets. The model that used a visible (480 nm) and an NIR (1095 nm) wavelength yielded lower classification accuracies (97% and 94.3% for calibration and validation sample sets, respectively) than the model using two visible wavelengths. The model that used both NIR wavelengths (875 and 985 nm) yielded the lowest classification accuracies (92% and 90.2% for calibration and validation sample sets, respectively). The results are further indications that visible wavelengths are more important for classifying pecky rice from sound rice than NIR wavelengths.
Table 3. Summary of Calibration and Validation Results Using the Linear Discriminant Functions for Classifying Pecky Rice and Sound Rice Kernelsa
Figure 3. Coefficient of determination (r 2) as a function of single and two wavelengths for single and two-wavelength models.
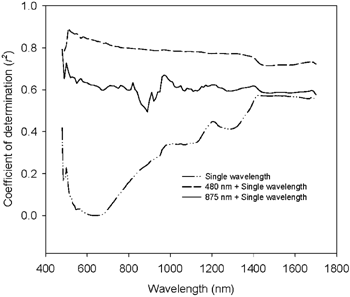
In sorting of snap beans, the greatest difference in light reflectance between white and off-colored (brown, tan, green; poor quality) seeds was observed in the 275–450 nm range; little differences were observed in the NIR region.Citation[21] The reflectance ratio of 440 and 490 nm has been used successfully by Tyson and Clark for detecting aflatoxin contamination in pecans (r 2=0.86).Citation[22] However, it appears that there are some physical properties and chemical constitutions contributing to the classifying pecky rive in the NIR region. The r 2 curve from single-wavelength model showed that r 2 increased as wavelengths increased in the NIR region (Fig. ). This indicates some chemical information beyond the color differences. The peak around 985 nm is close to the second overtone of OH.Citation[23] The peaks around 1210 nm and 1515 nm are related to fiber and protein content.Citation19-20 The peaks of r 2 values of the single-wavelength model had the same pattern as the Beta coefficient of the PLS model.
CONCLUSIONS
PLS full wavelength models and two-wavelength models were developed to classify pecky rice and sound rice kernels. For PLS models, the NIR wavelength region of 750–1700 nm gave the greatest percentage of correct classification for both calibration and validation (100%). The greatest correct classifications (100%) were obtained when a peck area faced the illumination light for wavelength regions of 500–750, 750–1700, and 500–1700 nm. Considering that stink-bug feeding also caused microorganism activity, resulting in discoloration on the grain, this made the classification of pecky and sound rice kernels (accuracy>99%) possible even when the undamaged area faces the illumination light. For two-wavelength models, the model with wavelengths of 480 nm and 590 nm yielded the highest classification accuracies for both calibration accuracies for both calibration (99.3%) and validation (99.3%) sample sets.
Acknowledgments
REFERENCES
- Grigarick , A.A. 1984 . General Problems with Rice Invertebrate Pests and their Control in the United States . Prot Ecol. , 7 ( 1 ) : 105 – 114 .
- Douglas , W.A. and Tullis , E.C. 1950 . Insects and Fungi as Causes of Pecky Rice . USDA Tech. Bull. ,
- Marchetti , M.A. 1984 . The Role of Bipoloris oryaze in Floral Abortion and Kernel Discoloration in Rice . Plant Dis. , 68 ( 2 ) : 288 – 291 .
- Lee , F.N. , Tugwell , N.P. , Weidemann , G.J. and Smith , W.C. 1986 . Microorganisms Associated with Pecky Rice Vol. 21 , 90 Houston, TX : Proc Rice Technical Working Group .
- Hollay, M.E. Rice Stink Bug on Rice: Evaluation of Plant Resistance, Interaction of Field Fungi with Feeding Damage, and Evaluation of Insecticides for Control. Ph.D. Dissertation; Louisiana State University: Baton Rouge, LA, 1987
- Harper, J.K. Developing Economic Thresholds for Rice Stink Bug Management in Texas using Dynamic Programming. Ph.D. Dissertation; Texas A&M University: College Station, TX, 1988
- Fryar, E.O.; Parsch, L.D.; Holder, S.H.; Tugwell, N.P. The Economics of Controlling Peck in Arkansas Rice. Arkansas Farm Research May–June, 1986
- Webb , B.D. 1985 . “ Criteria of Rice Quality in the United States ” . In Rice Chemistry and Technology Edited by: Juliano , B.O. 403 – 442 . St. Paul., MN : Am. Assoc. Cereal Chem. .
- Brorsen , B.W. , Grant , W.R. and Rister , M.E. 1984 . A Hedonic Price Model for Rough Rice Bid/Acceptance Markets . Amer. J. Agric. Econ. , 66 ( 2 ) : 156 – 163 .
- USDA. United States Standards for Rice. U.S. Department of Agriculture: Washington, DC, 1995
- Warren , R.G. , Rister , E.E. and Brorsen , B.W. 1983 . The Value of Rice Quality . Outlook and Situation Rice , 3 ( 1 ) : 8 – 11 .
- Champagene , E.T. , Richard , O.A. , Bett , K.L. , Grimm , C.C. , Vinyard , B.T. , Webb , B.D. , McClung , A.M. , Barton , F.E. , Lyon , B.G. , Moldenhauer , K. , Linscombe , S. , Mohindra , R. and Kohlwey , D. 1996 . Quality Evaluation of U.S.Medium-grain Rice using a Japanese Taste Analyzer . Cereal Chem. , 73 ( 2 ) : 290 – 294 .
- Kawamura , S. , Natsuga , M. and Itoh , K. Visual and Near-infrared Reflectance Spectroscopy for Determining Physicochemical Properties of Rice. Paper No. 983063 . ASAE Annual International Meeting . Orlando, FL
- Barton , F.E. , Windham , W.R. , Champagene , E.T. and Lyon , B.G. 1998 . Optimal Geometries for the Development of Rice Quality Spectroscopic Chemometric Models . Cereal Chem. , 75 ( 3 ) : 315 – 319 .
- Delwiche , S.R. , Bean , M.M. , Miller , R.E. , Webb , B. D. and Williams , P.C. 1995 . Apparent Mylose Content of Milled Rice by Near-infrared Reflectance Spectro photometry . Cereal Chem. , 72 ( 2 ) : 182 – 187 .
- Delwiche , S.R. , McKenzie , K.S. and Webb , B.D. 1996 . Quality Characteristics in Rice by Near-infrared Reflectance Analysis of Whole-grain Milled Samples . Cereal Chem. , 73 ( 2 ) : 257 – 263 .
- Windham , W.R. , Lyon , B.G. , Champagne , A.M. , Barton , E.T. , Webb , B.D. , McClung , F.E. , Moldenhauer , K. , Linscocombe , S. and McKenzie , K.S. 1997 . Prediction of Cooked Rice Texture Quality Using Near-infrared Reflectance Analysis of Whole-grain Milled Samples . Cereal Chem. , 74 ( 5 ) : 626 – 632 .
- SAS. SAS/STAT User's Guide: Version 6, Fourth Edition, Volume I. SAS Inc.: Cary, NC, 1995
- Shenk , J.S. , Workman , J.J. and Westerhaus , M.O. 1992 . “ Application of NIR Spectroscopy to Agricultural Products ” . In Hand Book of Near-Infrared Analysis Edited by: Burnes , D.A. and Ciurczak , E.W. 383 – 432 . New York : Marcel Dekker Inc. .
- Murray , I. and Williams , P.C. 1990 . “ Chemical Principles of Near-infrared Technology ” . In Near-Infrared Technology in Agricultural and Food Industries Edited by: Williams , P.C. and Norris , K. 17 – 34 . St. Paul., MN : Am. Assoc. Cereal Chem. .
- Lee , P.C. , Paine , D.H. and Taylor , A.G. 1998 . Detection and Removal of Off-colored Bean Seeds by Color Sorting . Seed Technology , 20 ( 1 ) : 43 – 55 .
- Tyson , T.W. and Clark , R.L. 1974 . An Investigation of the Fluorescent Properties of Aflatoxin Infected Pecans . Transactions of the ASAE , 17 ( 5 ) : 942 – 944 . 948
- Osborne , B.G. and Fearn , T. 1986 . Near Infrared Spectroscopy in Food Analysis Essex, , U.K : Longman Scientific and Technical Inc. .