Abstract
A rapidly increasing number of modules in degree programmes now utilise virtual learning environments. A main feature of virtual learning environments, to which their adoption by many educators is attributed, is automated assessment. Automated assessment is particularly attractive where the number of students taking a module is large. As mathematics is an essential pre-requisite for those studying engineering courses and is compulsory in almost every engineering programme, classes tend to be large. However, there is ongoing scrutiny of the various aspects of virtual learning environments, including automated assessment. This paper is based on a case study that highlights aspects of the impact of automated assessment by studying the performance of students taking an engineering mathematics module where automated assessment is utilised in the form of quizzes.
Introduction
Networks have now become commonplace, computer technology more readily available and affordable to the public and, as reported in many studies (CitationBrohn, 1986; Gbomita, 1997; McDowell, 1995; Stephens et al., 1998; Thelwall, 2000 and Zakrzewski and Bull, 1998), virtual learning environments (VLEs) are now generally reliable. As a result of such advances in information technology, VLEs are fast becoming an integral part of many taught courses.
VLEs provide a number of advantages compared to conventional face-to-face learning. They can utilise repositories that can be accessed at all times, enabling the effective use of time and making catching up easier. They also enable automatic assessment and instant feedback that makes self-assessment possible and results in an improved learning experience (CitationBrohn, 1986; Gbomita, 1997; McDowell, 1995; Stephens et al., 1998; Thelwall, 2000 and Zakrzewski and Bull, 1998).
Automated assessment is one of the main benefits provided by utilising VLEs, and has made them appealing to many educators. Automated assessment drastically reduces time spent on assessment by instructors to as low as 30%. (Where no electronic assessment is used, about 75% of the time given to a module is normally spent on assessment (CitationSmaill, 2005)). Revision without using valuable lecture time is made possible, lowering running costs and increasing efficiency (CitationGriffin and Gudlaugsdottir, 2006). The use of automated assessment is therefore expected to increase the quality of education, as instructors should have more time to concentrate on improving and updating the delivery and content of courses (CitationJuedes, 2003).
Given that that a decline in mathematics skills has been reported (CitationDavis et al., 2005), automated assessment can play a vital role in improving student learning in engineering mathematics. However, varied opinions have been presented on the suitability and effectiveness of automated assessment, with some studies presenting no improvement and negative feedback while others have reported positive results (CitationSmailes, 1998). It is in this light that this work evaluates automated assessment by studying the performance of students taking an engineering mathematics course with VLE-based automatically assessed quizzes. Quiz questions requiring single answers were utilised that fulfil the evaluation and procedural knowledge of the revised Bloom’s taxonomy (CitationColeman et al., 1998). Single answer questions also eliminate the effects of other pedagogical factors as much as possible in the evaluation of automated assessment.
The details of this study are presented in the next section, followed by the results of the study and analysis and finally the conclusions reached by the study.
The study
This study is based on a group of first year students on a foundation degree programme in electrical and mechanical engineering in 2006. This is a two year programme for students who do not qualify for the conventional degree programme. At the end of the programme students who perform exceptionally well may transfer to one of the conventional electrical and mechanical engineering degree programmes. Students should have a minimum UCAS tariff of 140 points at GCE/A-level or equivalent and passes equivalent to GCSE grade C or above in at least four subjects, including English and mathematics.
The study was carried out on an introductory mathematics module in the first semester of the first year which covered the fundamentals of engineering mathematics. The topics covered included algebra, co-ordinate geometry and statistics. The pedagogical goals were for students to acquire knowledge of and skills in the listed topics and, as a result, to be able to identify and apply appropriate methods to solve different problems or obtain information.
The group constituted of 52 students, of which 42 (81 %) were male and ten (19 %) were female. Face-to-face lectures and tutorials were provided every week, each lasting two hours, throughout the duration of the course. In addition, the students had a two hour supervised laboratory session every week in which they utilised software tools to solve mathematical problems.
The E-learning component was employed using a commercial VLE package, WebCT (now Blackboard — see http://www.blackboard.com). The web-based resource constituted learning resources that included tutorials, lecture slides, notes, laboratory exercises, quizzes and self-test exercises. It also had auxiliary facilities such as a module handbook, calendar, tutorials, bulletin board and email. Such a combination of face-to-face lectures and the use of VLEs has been reported to be students’ preferred learning environment (CitationSmailes, 1998 and Davis et al., 2005). The module was assessed by a series of online quizzes and computer-based laboratory exercises (solving problems utilising software tools and then submitting reports electronically) and a hand-written test at the end of the module. The mark distribution for the different assessments making up the module, namely laboratory exercises, quizzes and the written test, were 10, 25 and 65% respectively.
Quizzes and self-test exercises
There were four assessed quizzes in total. These were held fortnightly from the third week of the course and were intended to provide a stimulus for learning and revising material learnt in lectures. The quizzes were supervised (to authenticate the identity of the students and to minimise cheating by ensuring that students worked independently at their consoles) and were to be completed within an hour. The number of questions per quiz varied. A password was provided at the beginning of each quiz as a further security measure. There were two dyslexic students in the class who did not require any additional time to complete the quizzes.
Self-test exercises covering material similar to that in the next quiz were posted at least a week prior to each quiz. These provided the opportunity for students to test themselves and practise at their own pace without drawing on the instructor’s time. The quizzes are also ideal for students who find it difficult to participate in face-to-face sessions and they also cater for different learning styles, for instance those with a kinesthetic and reading inclination. Studies have shown that through the use of automated quizzes, performance improves rapidly at each attempt of an exercise (CitationBrown, 2004). There were five self-test exercises. The material in the last exercise was not tested in a quiz. The questions in the quizzes and self-test exercises had randomly generated variables. A scheme similar to that of CitationChen (2004) was utilised, where each time a student attempted a quiz or exercise the variables were set to different values. In this instance the variables were one or more numbers representing some quantity in a question such as angles, length, data values or constants in equations. As a result each student had different variable values for the same question. This made copying and assistance from fellow students more difficult. An example question is shown in .
As the questions in the exercises simply required a single answer this enabled the effectiveness of the automated system to be assessed, rather than other pedagogical factors. The feedback provided by the system was the correct answer only - additional feedback and a discussion of the problems were provided in the next class. In this way students were not tempted to enter wrong answers so as to obtain the worked solutions of the problem, which in some cases can be detrimental (CitationChen, 2004). Indeed, students did exhibit this behaviour, especially in the first self-test exercises. This behaviour greatly diminished in subsequent exercises presumably because variables had different values each time the exercises were accessed. Feedback was provided in weekly tutorial sessions.
Statistics on the students’ use and grades on both of the quizzes and self-test exercises were automatically logged by the VLE (WebCT). The VLE also logged other activities such as the frequency of accessing resources.
Written test
The written test was held at the end of the semester when all VLE based quizzes had been completed. It consisted of 23 questions. Of these, nine were from topics covered in the quizzes and the first four self-test exercises (algebra, trigonometry, vectors and geometry). Six questions were from statistics, the topic covered by the last self-test exercise, which did not have an accompanying quiz. Eight questions were in areas not covered in the quizzes. These questions were on formulae, functions and algebraic expressions. However, it should be noted that all topics covered in the written test had been covered in lectures and tackled in the weekly tutorial sessions. The duration of the written test was two hours and it was closed book.
Results
The confidence intervals for means in the results presented here were determined using the analysis of variance (ANOVA) statistical test. The results show the average mark and its respective confidence interval. The ANOVA F figure and probability (p) of the null hypothesis are also given. The implied null hypothesis in this instance is that the effect being tested for is not true. This corresponds to an ANOVA F figure of one. The confidence interval level shown in all cases in this work is 95%. shows the distribution of students as a function of the number of quizzes and self-test exercises they took.
shows the average mark and their respective 95% confidence intervals for grades obtained in the written test by students as a function of the number of assessed quizzes they attempted. Only results for students who took between two and four quizzes are shown, as the students who took fewer than two quizzes are small in number and hence may distort the results. The probability of these results assuming null hypothesis is 0.028. This is less than 0.05, implying that students performed better the larger the number of quizzes they attempted.
The performance as a function of the number of self-test exercises is shown in . The probability of this result assuming null hypothesis is 0.058 (F = 2.473), implying that the number of self-tests taken by a student may be a factor as it is close to 0.05. This result is attributed to the observed behaviour of students during self-test exercises, for instance entering wrong answers so as to obtain the worked solutions of problems. This behaviour was mainly exhibited for the first self-test exercise. Students were discouraged from this practice in subsequent exercises when they realised that the variables changed each time they accessed a question. As a result some students only viewed questions without attempting to enter answers. Similar behaviour is reported by CitationSmail (2005) where students attempted to win-out by memorising answers.
Figure 4 Average test score and 95% confidence interval for mean as a function of self-test exercises taken
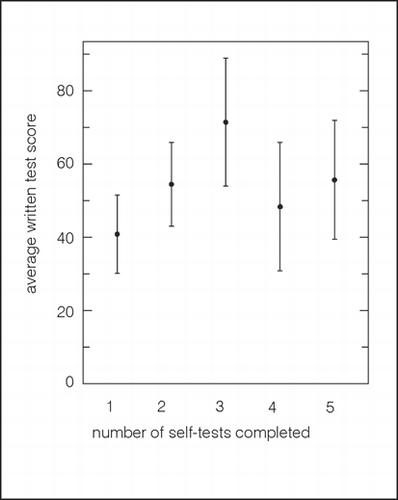
shows a comparison of the performance in the written test on the nine questions relating to topics covered in the quizzes of students who completed the quizzes and those who did not. Although the probability for the null hypothesis is 0.093 (F = 1.49) it should be noted that students who completed the quiz performed better on average in six of the nine questions.The results in the three questions that suggest otherwise are attributed to a combination of the fact that the number of students who did not complete the quizzes was much smaller than those that did (about 10 compared to about 40) and that two students in the group who did not complete any quizzes scored 93 and 74% in the written test, much higher than the class average of 50%. The lower limit of the 95% confidence interval is lower for students who did not complete corresponding quizzes for two (questions 1 and 7) of the three questions. Furthermore, question 3 was on fractions, a topic most students would already have been conversant in, hence rendering the influence of completing the quizzes of little consequence.
shows the performance in the written test on topics covered in the last self-test exercise of those students who completed the exercise compared with those who did not. Note that the last self-test exercise did not have a corresponding assessed quiz. In this instance, although the 95% mean confidence interval overlaps for the two groups, for most questions the students who completed the exercise performed better on average in five out of the six questions.
For the results in the class population is grouped into the categories listed in .
Figure 5 Average test score per question on topic covered in quizzes and 95% confidence interval for mean.
C: completed respective quiz;
N: did not take quiz
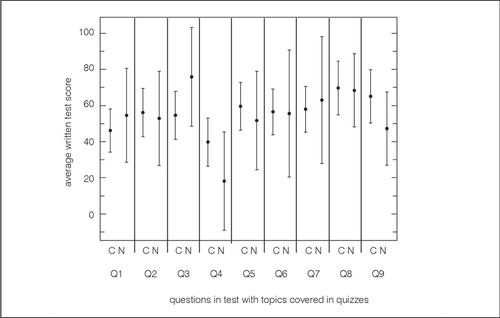
Figure 6 Average test score per question on topic covered in statistics self-test and 95% confidence interval for mean.
C: completed self-test;
N: did not take self-test
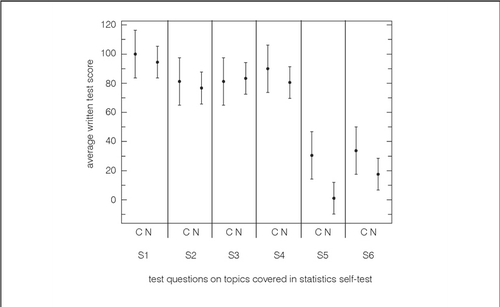
Table 1 Definition of Groups
Figure 7a Average test score per group for questions on topics covered in quizzes and 95% confidence interval for mean

Group B is not shown in the results due to the small amount of data in this category. Here the probability for the null hypothesis for the mean is 0.063, however, the mean is observed to be highest for group D who had the highest number of quizzes and self-test exercises. shows results for the same groups for questions in the written test in the following categories: (A) topics covered in the quiz; (S) topics covered in self-test exercises and (N) other topics. It is evident that the performance in topics covered in quizzes and self-tests is significantly greater than that in topics not covered.
The overall performance by all students in questions covered in quizzes, self-tests and other topics is shown in . The results clearly show better performance in questions that were previously covered in quizzes and self-test exercises. There is also no overlap of the confidence intervals of questions covered in either quizzes or the statistics self-test with questions not covered.
A survey of students on this course indicated that their feelings were generally amicable towards the VLE, with 78.5% of the 46 respondents finding nothing negative about automated assessment. Similar attitudes to automated assessment have been recorded with students preferring computer quizzes to conventional tests (CitationReinhardt, 1995; Chirwa, 2006 and Mastascusa, 1997).
Conclusion
The results presented here suggest that students performed better in topics that were covered in quizzes and self-test exercises. Taking into account that areas not covered in automated assessments were covered in tutorials it can be concluded that automated assessment contributes to more effective learning. Here, too, there are no indications that automated assessment significantly deters performance, which is in agreement with some studies (CitationColeman et al., 1998).
Figure 7b Average test score per group for questions on topics covered in quizzes and 95% confidence interval for mean.
A: questions on topics covered in quiz;
S: questions on topics covered in statistics self test;
N: questions on topics not covered in quiz or statistics self-test
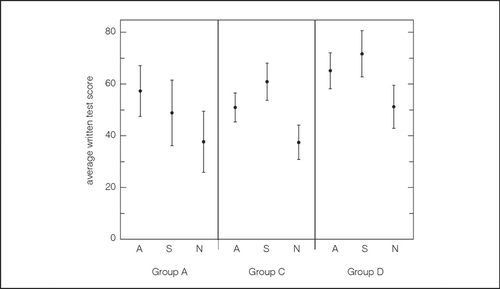
Figure 8 Overall average test score per group for questions on topics covered in quizzes and 95% confidence interval for mean.
A: questions on topics covered in quiz;
S: questions on topics covered in statistics self test;
N: questions on topics not covered in quiz or statistics self-test
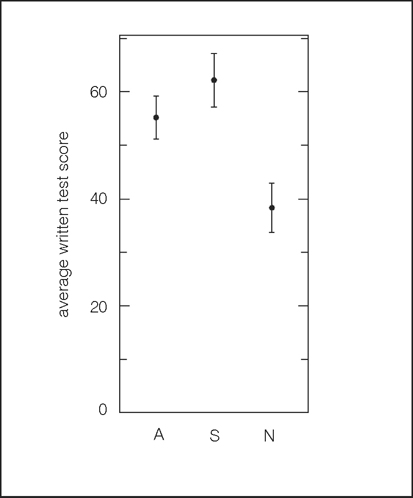
Although savings in time when using automated assessment are negative for low numbers of students in some studies (CitationSmaill, 2005), the benefit of allowing students to test themselves and learn from their mistakes privately (CitationChen, 2004) is, however, not diminished. They are therefore an effective means of implementing assessment driven learning. However, they are inhibited by the limitations of the complexity of solutions that can be implemented compared to those possible in the conventional handwritten form. Another positive feature of VLE based quizzes is the ability to generate different variable values in the same questions for different students, minimising cheating and resulting in more effective learning of the principles required to solve problems.
In view of the above, a programme incorporating automated assessment is not likely to be detrimental. The programme can further be improved by the implementation of automated assessment in appropriate pedagogical contexts, for instance as a component of a larger problem. Once automated assessment has been set up it is no longer labour intensive and becomes easier to implement than conventional ‘manual’ assessment exercises, especially for larger groups of students.
It should be noted that, although the sample size in this study is too small to be conclusive in its own right, this work contributes to existing studies in this area and provides a clearer understanding of the effects of automated testing. The results from the self-test exercises and the quizzes indicate that students apply themselves more to assessed work (CitationRace, 2001) and, as a result, the use of automated assessment is likely to have a positive pedagogical effect.
References
- Blackboard Inc. http://www.blackboard.com [accessed 30 April 2008].
- BrohnD.M. (1986) The use of computers in assessment in higher education, Assessment and Evaluation in Higher Education, 11 (3), 231-239.
- BrownR.W. (2004) Undergraduate Summative Assessment Experiences. 34th ASEE/IEEE Frontiers in Education Conference, 20-23 October 2004, Savannah, GA, USA.
- ChenP.M. (2004) An automated feedback system for computer organization projects. IEEE Transactions on Education, 47 (2), 232-240.
- ChirwaL.C. (2006) Use of E-learning in Engineering Mathematics. International Conference on Innovation, Good Practice and Research in Engineering Education, 24-26 July 2006, Liverpool, UK.
- ColemanJ.N., KinnimentD.J., BurnsF.P., ButlerT.J. and KoelmansA.M. (1998) Effectiveness of Computer-Aided Learning as a Direct Replacement for Lecturing in Degree-Level Electronics. IEEE Transactions on Education, 41 (3), 177-184.
- DavisL.E., HarrisonM.C., PalipanaA.S, WardJ. P. (2005) Assessment Driven Learning of Mathematics for Engineering Students. International Journal of Electrical Engineering Education, 42 (1), 63-72.
- GbomitaV. (1997) The adoption of microcomputers for instruction: implication for emerging instructional media implementation. British Journal of Educational Technology, 28 (2), 87-101.
- GriffinF. and GudlaugsdottirS. (2006) Using Online Randomised Quizzes to Boost Student Performance in Mathematics. 7th international Conference on Information Technology Based Higher Education Engineering, 10-13 July 2006, NSW.
- JuedesD.W. (2003) Experiences in Web-Based Grading. 33rd ASEE/IEEE Frontiers in Education Conference, 5-8 November 2003, Boulder, CO, USA.
- McDowellL. (1995) Effective teaching and learning on foundation and access courses in engineering, science and technology. European Journal of Engineering Education, 20 (4), 417-425.
- MastascusaE.J. (1997) Incorporating “Computer-Graded” Components Into Electronic Lessons. Frontiers in Education Conference, 5-8 November 1997, Boulder, CO, USA.
- Moura SantosA., SantosP.A., Dion´ýsioF.M., DuarteP. (2002) On-line assessment in undergraduate mathematics: An experiment using the system CAL for generating multiple choice questions. 2nd International Conference on the Teaching of Mathematics, 1-6 July 2002, Crete, Greece. Available online from http://www.math.uoc.gr/~ictm2/Proceedings/pap139.pdf [accessed 30 April 2008].
- RaceP. (2001) The Lecturer’s Toolkit: A practical guide to learning, teaching and assessment, 2nd edition. London: Kogan Page.
- ReinhardtA. (1995) New Ways to Learn. BYTE, 20, 50-72.
- SmailesJ. (1998) CALculating Success? Available from http://www.icbl.hw.ac.uk/ltdi/evalstudies/essuccess.htm [accessed 30 April 2008].
- SmaillR.C. (2005) The Implementation and Evaluation of OASIS: A Web-Based Learning and Assessment Tool for Large Classes. IEEE Trans. Education, 48 (4), 658-663.
- StephensD, BullJ. and WadeW. (1998) Computer-assisted assessment: suggested guidelines for an institutional strategy. Assessment and Evaluation in Higher Education, 23 (3) 283-294.
- ThelwallM. (2000) Computer based assessment: a versatile educational tool. Computers and Education, 34 (1), 37-49.
- ZakrzewskiS. and BullJ. (1998) The mass implementation and evaluation of computer-based assessments. Assessment and Evaluation in Higher Education, 23 (2), 141-152.