Abstract
Breast cancer remains the leading cause of cancer-related mortality in women. Comprehensive genomics, proteomics, and metabolomics studies are emerging that offer an opportunity to model disease biology, prognosis, and response to specific therapies. Although many biomarkers have been identified through advances in data mining techniques, few have been applied broadly to make patient-specific decisions. Here, we review a selection of breast cancer prognostic indicators and their implications. Our goal is to provide clinicians with a general evaluation of emerging computational methodologies for outcome prediction.
Introduction
One in eight women develops breast cancer, the most common cause of malignancy in females. Although the majority of patients now survive for many years after initial diagnosis and therapy, a significant subpopulation remains at risk of metastatic relapse. These women have a median survival time of less than 1.5 years at the time of relapse,Citation1 with only 10% expected to survive 10 years after diagnosis.Citation2
The ability to classify patients into clinically relevant subgroups to allow for precise therapy is urgently needed. Traditional tumor stratification is based largely on morphology, but is relevant in less than one-quarter of invasive breast carcinomas.Citation3 However, novel computational models are emerging as powerful tools to address this deficiency. Recent work has integrated critical observations in the biology of breast cancer, including gene deletions, translocations, and locus amplification;Citation4,Citation5 biomarkers from high-throughput “-omics” technologies such as genomics, proteomics, and metabolomics; and long recognized outcome variables such as tumor size, histologic grade, axillary nodal status, and estrogen receptor (ER) status. We anticipate that these biomarkers will emerge as an effective molecular classification or guide for the determination of prognosis and the development of tailored therapy (reviewed by Gruver et alCitation3).
However, much work regarding validation of these approaches is still required. For example, single biomarkers have not proven highly informative in most women. Somatic mutations with the potential ability to act as a biomarker were noted to occur in single genes (eg, TP53, PIK3CA, and GATA3) in <10% of 825 patients with primary breast cancers.Citation6 Similarly, only three multi-gene signatures are incorporated into current clinical practice.Citation7–Citation9 Effective and clinically applicable prognostic indicators will also require the ability to address the emerging importance of the intra-tumoral heterogeneity of breast cancer.Citation10
This review will not address specific clinical prognostic variables as they have been reviewed extensively by others.Citation11–Citation14 Instead, we have chosen to evaluate emergent prognostic indicators for breast cancer, focusing on computational methodologies and strategies to identify a molecular indicator (a biomarker or set of biomarkers). We are interested particularly in statistical and computational methodologies based on cutting-edge “-omics” technologies, moving from the conventional single oncogene/tumor suppressor gene strategy to one that exploits advances in systems biology. To achieve our goal, we performed a systematic search of all the English language literature regarding computational breast cancer prognosis, using a MEDLINE search for the period from January 2005 to July 2013. This review will begin with the utility of single molecular biomarkers and multi-gene signatures, followed by an evaluation of novel system-biology based analyses that infer information from high-throughput “-omics” data. Importantly, we will discuss exciting possibilities in the development of new integrative methodologies.
Identification of prognostic molecular signatures
Examination of genetic variation, genomic association, or transcriptomic alterations in large study cohorts allows the selection of single or multiple prognostic markers that can stratify patients into groups showing distinct outcomes (). It may also allow the specific tailoring of care. Sample size and biological hypotheses are distinguishing features of prognostic marker studies.Citation15,Citation16 In terms of sample size, a practical clinically based prognostic index for patients with metastatic breast cancer was recently proposed by a retrospective analysis of 2,322 patients after primary treatment.Citation2 In parallel, others have focused on using biological determinants. For example, the Cancer Genome Atlas Network has incorporated the four major breast cancer subtypes with five genetic and epigenetic factors (genomic DNA copy numbers, DNA methylation, exome and messenger RNA expression, microRNA sequencing, and reverse-phase protein expression).Citation6 The results are promising for understanding the biological underpinning of subtype-specific prognostic indicators. However, there remains a critical gap between an integrative model of “omics”-data and clinical variables. Describing biological pathways underlining clinical biomarkers in a large sample size could provide the opportunity to significantly improve our understanding of the biology and heterogeneity of breast cancers. For instance, the enrichment patterns of gene sets associated with embryonic stem cell (ESC) identity in the expression profiles of various human tumor types is associated with poor outcome in breast cancer.Citation17
Single gene prognostic determinants
For specific tumor subtypes, the expression of single critical genes can serve as prognostic indicators (reviewed by Adam Maciejczyk).Citation18 Germ-line mutations of BRCA1 are extensively used for early detection of familial breast cancer, and are predictive for 15%–20% of women with a family history of breast cancer, and 60%–80% of patients with combined breast and ovarian cancer.Citation19 Additional prognostic factors include enhanced RAD21 and cohesin expression, which has been associated with resistance to chemotherapy in high-grade luminal, basal, and HER2 breast cancers.Citation20 Transcription factor muscle segment homeobox 2 (Msx2) expression has been implicated in an increased likelihood of tumor cell death via apoptosis in invasive breast cancers.Citation21 Enhanced expression of the anterior gradient-2 (AGR2) protein, which occurs in the presence of ER antagonist tamoxifen, confers poor prognosis in ER-positive breast cancers.Citation22
The most common computational method to assess these critical biomarkers is the hazard Cox regression model.Citation23 Given a time after diagnosis for values of the predictor variables, the model produces a survival function for the probability that the binary event of interest (eg, death or survival at the endpoint) occurs. In this context, additional computational models to improve the prediction have been proposed, including Bayesian network analysis evaluating probabilistic relationships among candidate genesCitation24 and support vector machine methodologies.Citation25 A review of standard survival analyses and the use of the outstanding Bioconductor tool suite is available at http://cran.r-project.org/web/views/Survival.html.
Current status of multi-gene prognostic determinants
Multi-gene signatures are most often derived from transcriptomic microarray and sequence data.Citation26–Citation28 These signatures not only have potential for classification and prognosis,Citation29 but they can also predict specific tumor sub-phenotypes such as resistance to radiation.Citation30,Citation31 Generally, these multi-gene models can classify patients into subgroups with either distinct outcomes or diverse treatment responses in an unsupervised manner. As an example, the DNA content of breast adenocarcinomas can be classified as either stable, conferring good prognosis, or unstable, conferring poor prognosis.Citation32
To identify multi-gene signatures or pathways, computational pattern learning algorithms have been successfully applied to transcript or mass spectrometry profiles. These algorithms can be roughly categorized into three groups: 1) unsupervised data mining, eg, hierarchical clustering,Citation7,Citation33 topographic projection,Citation34 and other methodologies; 2) supervised classifiers, eg, decision trees,Citation35 and Prediction Analysis of Microarray (PAM);Citation9 and 3) semi-supervised learning models.Citation36 In supervised algorithms, sample labels such as good- or poor-outcome are required to train the model before making decisions. In contrast, unsupervised algorithms are data-driven. In a semi-supervised model, the algorithm makes decisions based on both the raw data and the input labels, allowing partial labeling. Which method to choose depends on study purpose – for example, if a certain phenotype is known to be an important factor in identifying the multi-gene signature, one should select supervised methodologies to make use of that information.
Clinical application of prognostic determinants
In terms of single determinants, the expression levels of four genes (ER, PR, HER2, and Ki67), and combinations thereof, have been shown to have a strong prognostic impact (reviewed by Gökmen-Polar et alCitation37). Missense mutations, eg, within the tumor suppressor gene p53 or increased levels of urokinase-type plasminogen activator (uPA) and/or plasminogen activator inhibitor-1 (PAI-1), which indicates poor clinical outcome, have been included by the American Society of Clinical Oncology 2007 Update of Recommendations for the Use of Tumor Markers in Breast Cancer.Citation38 Other single-gene determinants with somatic mutations are sensitive to targeted therapy; for instance, active ESR1 mutations in ER-positive metastatic breast cancer.Citation39
Several multi-gene–based commercial prognostic testing methodologies are now available. The MammaPrint® 70-gene signature assay (Agendia, Inc., Irvine, CA, USA) stratifies patients’ outcomes and allows personalized therapeutic prediction.Citation7,Citation40,Citation41 Two additional clinical decision-making assays, the Oncotype DX 21-gene assayCitation42 (Genomic Health, Inc. (Redwood City, CA, USA) and a clinically updated version of the intrinsic subtype PAM50 assayCitation43 (ARUP Laboratories, Salt Lake City, UT, USA), have been compared. Predicting prognostic intrinsic subtypes among 151 patients, investigators observed good agreement between the 21-gene and PAM50 assays for high and low prognostic risk assignment.Citation44
Overall, molecular prognostic determinants have been successfully developed as adjuvant tools for innovative diagnostic, prognostic, and therapeutic approaches. As shown by Albain et al,Citation45 changes in treatment decision after review of multi-gene signatures occurred in 30% of individuals predominantly from chemotherapy plus endocrine therapy to endocrine therapy alone with an associated diminution in treatment-related toxicity.
However, there are several limitations in the utility of either single gene or multi-gene prognostic signatures in routine practice, including requirement of fresh or frozen tissues to measure uPA/PAI-1 and deliberate measurement compared with a gold standard or “housekeeping genes” for single gene determinants. Another limitation for both single and multi-gene signatures is that they are positive in only a specific subtype of breast cancer. For instance, the 70-gene assay can only identify potential chemotherapy benefits in high-risk patients.Citation45 In another study, the discordance rate was approximately 30% between the clinicopathologic risk categories given by the 70-gene assay and the 21-gene assay.Citation45 Both assays are particularly limited in assigning high-risk status to ER-negative patients.Citation46
How do we overcome these challenges?
Key issues with current multi-gene signature methodologies include specificity of prediction and standardization across diagnostic platforms. Li et al have reported that many randomly selected genes show predictive power for cancer prognosis in one dataset, while losing predictive power in other datasets.Citation47 Therefore, an evaluation using independent datasets is necessary to assess a prognostic model or indicator. Recently, Venet et alCitation28 compared 47 published breast cancer outcome signatures to mimic signatures made of random genes. Surprisingly, they found that 60% of reported gene signatures of identical size were equally predictive of “mimic” gene signatures. Of particular concern, 23% of the signatures were worse than random, and more than 90% of mimic signatures with 100 genes were significant outcome predictors.Citation28 Thus, the deficiencies associated with the reproducibility of multi-gene–based prediction prevent effective usage at this time.
The complexity and discrepancy of single- or multi-gene signatures also precludes easy extraction of biologically and therapeutically relevant information. A clue may be provided by observations that suggest that although genetic alterations between patients differ, they frequently involve common pathways. It is therefore critical to identify relevant pathways involved in breast cancer progression and detect the corresponding indicators that are prognostic in different tumor subtypes or patients.
Novel strategies for prognostic indicator development
Incorporation of network or pathway information into prognostic biomarker discovery could significantly improve prediction performance. For this purpose, investigators have used a large number of supervised machine-learning approaches (ie, inferring knowledge from data with labeled samples) to take advantage of prior knowledge. For example, data mining on gene expression profiling reveals an association between hyperactivity in the PI3K pathway, lowered ER levels, and resistance to endocrine therapy.Citation48 Unfortunately, there is no single “one-size-fits-all” algorithm, integrating accuracy, stability, and interpretability of gene selection.Citation49 lists methods to identify prognostic determinants based on gene expression in breast cancer. Two recent reviews discuss prognostication of tumor mutations using pathway and network analysis.Citation50,Citation51
Table 1 Methods to identify prognostic determinants based on gene expression in breast cancer
Using biological hypothesis-based gene selection and interpretation, we and others have identified transcriptional prognostic indicators (). As prognostic biomarkers, these models calculate a score per sample and use a simple threshold value of 1 to dichotomize patients into prognostic groups. Pitroda et al piloted a tumor endothelium-derived inflammatory signature consisting of six genes that were associated with poor outcome in multiple cancers.Citation52 Similarly, in multiple adult tumors, we found that histologically poorly differentiated tumors display an ESC-associated expression imbalance, ie, preferential overexpression of targets of ESC-associated transcription factors (NANOG, OCT, SOX2, and MYC) combined with underexpression of Polycomb-regulated genes.Citation53 This finding is in agreement with the known significance of survival difference between patients with the expression pattern of these ESC-like signatures and other patients.Citation17 More recently, the coactivation of the ESC marker MYC and oncogene HER2 was associated with an acquisition of a self-renewal phenotype that is associated with poor outcome.Citation54
However, the lack of standards for measurement across various studies, assays, and diagnostic platforms makes it difficult to translate individual findings into clear clinical applications. A popular solution is to compare the median scores of a calculated indicator in a measured cohort,Citation52 whereas adding new patients changes the estimation of median score and thus the final decision.
Relative expression analysis of gene-set pairs (RXA-GSP), is a novel methodology that we have pioneered to translate prognostic gene targets for individualized treatment planning.Citation53 RXA-GSP is built on three principles: 1) each individual has both favorable and unfavorable prognostic factors for breast cancer. It is the imbalance that determines the individual outcome (). For example, NANOG expression is an indicator of poor prognosis stimulating the growth and metastasis of breast cancer cells, whereas KLF4 is a favorable prognostic indicator inhibiting these processes.Citation55 2) The correlation between two factors is, at least in some cases, more significant than the overall expression of either. For example, SIRT1 and DBC1 are both overexpressed in breast tumor tissue, but the correlation between their levels of expression is diminished.Citation56 3) We employ a hypothesis-based and experimentally derived approach to the identification of candidates.
Figure 1 Illustration of RXA-GSP method.
Notes: This prognostic indicator is the ratio between scores (eg, expression values) of poor prognostic markers versus that of good prognostic markers. It has the ability to integrate different scales of data, bridging cancer biology with the clinic by employing both hypothesis-based and experimentally derived gene-set selection.
Abbreviation: RXA-GSP, relative expression analysis with gene-set pairs.
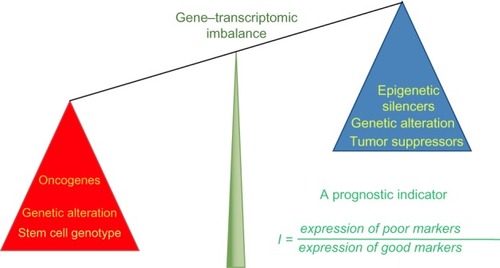
Using RXA-GSP, a resultant indicator is computed as the optimal combination of candidate genes that was trained from several large cohorts, and validated in independent cohorts.Citation53 Considering the heterogeneity of breast cancer in each individual patient along with the relative expression of gene-set pairs and the correlations between them, RXA-GSP allows the computationally driven derivation of individualized prognostic indicators, bridging cancer biology with the clinic through gene expression analysis.
Next generation integrative prognostic methods
To date, the majority of studies focused on prognosis have integrated clinical variables with gene expression or biological pathway data.Citation53,Citation57,Citation58 However, attractive opportunities are now available to integrate comprehensive genotype, metabolomics, and phenotype data. Success should facilitate robust prognostic and therapeutic prediction for each patient. Indeed, such success in this endeavor will have significant impact across biomedicine.
However, this potential is limited by the challenges of the sheer size and complexity of high-throughput data resources, often resulting in significant imprecision in data usage. Classical statistical models are frequently insufficient for integrating “omics”-data with other resources, so specific problems are dealt with on a case-by-case basis due to the lack of a coherent overarching method of analysis. Indeed, we and others, have proposed the development of integrated breast cancer prognostic indicators to provide insights into significant variables.Citation53,Citation59,Citation60
Eklund et alCitation59 have addressed this problem through an eScience–Bayes approach, complementing a Bayesian probability models’ ability to incorporate gene expression with text mining, modeling highly complex problems with high performance computing. Applying this model to several sets of independent gene expression data results in consistently accurate prediction of breast cancer metastases across cohorts.Citation59 Though such methods show promise for tailoring specific models to complex problems with high accuracy, their application is cumbersome, as they require manual selection of genes for which we have prior knowledge. This deficiency is related to a lack of a standard for displaying scientific text in formats that are computationally readable. Another obvious weakness is that a microarray represents a single snapshot of the patient.
New avenues of patient-specific tumor phenotyping are also facilitating prognosis prediction. These methodologies include the evaluation of risk associated with inherited genetic variation or genome-wide association studies (GWAS).Citation61,Citation62 Similarly, epigenetic changes in breast cancer cells (such as modifications in histone acetylation and DNA methylation at specific gene regulatory elements) have been implicated in breast oncogenesis.Citation6,Citation63 These observations, coupled with the emerging use of epigenetic-modifying agents suggest an era of genomics-based therapy selection.Citation33,Citation64,Citation65 For example, stromal/microenvironmental elements affect the prognosis, modifying tumor invasion and/or drug targeting.Citation66,Citation67
Thus, the effectiveness of interpretations of multi-gene assays likely depends on adjustment for other factors. A breakthrough work, Pathway Recognition Algorithm using Data Integration on Genomic Models (PARADIGM),Citation60 integrates copy number, mRNA expression, and pathway interaction data to a personalized pathway-by-sample matrix that clusters patients into distinct prognostic subgroups. This integration algorithm has been recently applied to depict comprehensive molecular portraits of human breast tumors.Citation6
In summary, we believe biological hypothesis-driven computational models that integrate current significant variables in multiple facets, including transcriptomic alterations, chromatin modification, and stroma response, will provide significant insight not only into the biology of breast cancer, but also into determining the prognosis of individual patients.
Conclusion
Challenges remain for breast cancer prognosis due to its complexity and the lack of standardization among models and “omics”-datasets. Specifically, what impedes the convenience and simplicity necessary for clinical application is the lack of precision molecular indicators that help individualized therapeutic decisions. Ideally, a prognostic indicator performs in a specific, sensitive, inexpensive, and easy manner. We expect an integrative computational model of “omics”-data and clinical variables to significantly improve our understanding of the biology and heterogeneity of breast cancers. This is likely to be achieved through either enlarging the sample size or characterizing a specific subtype with given phenotypes. The latter has been recently recognized, though controversial, hypothesizing a collection of genuinely variable malignances that happen to originate from breast epithelium.Citation68 In conclusion, a prognostic indicator should clearly delineate the risk group for an individual, in terms of tumor growth, invasion, and metastatic potential, and/or the likelihood of response to a given therapeutic modality.
Acknowledgments
This research was supported by NIH grant R21 CA167305-01A1. We appreciate Zakir Gowani for his careful proofreading.
Supplementary material
Table S1 Selected instances from literature search
References
- van’t VeerLJDaiHvan de VijverMJGene expression profiling predicts clinical outcome of breast cancerNature2002415687153053611823860
- RennstamKAhlstedt-SoiniMBaldetorpBPatterns of chromosomal imbalances defines subgroups of breast cancer with distinct clinical features and prognosis. A study of 305 tumors by comparative genomic hybridizationCancer Res200363248861886814695203
- PaikSShakSTangGA multigene assay to predict recurrence of tamoxifen-treated, node-negative breast cancerN Engl J Med2004351272817282615591335
- KronenwettUPlonerAZetterbergAGenomic instability and prognosis in breast carcinomasCancer epidemiology, biomarkers & prevention: a publication of the American Association for Cancer Research, cosponsored by the American Society of Preventive Oncology200615916301635
- BacacMProveroPMayranNStehleJCFuscoCStamenkovicIA mouse stromal response to tumor invasion predicts prostate and breast cancer patient survivalPLoS One20061e3217183660
- SuhKSCrutchleyJMKoochekAReciprocal modifications of CLIC4 in tumor epithelium and stroma mark malignant progression of multiple human cancersClin Cancer Res200713112113117200346
- ConlinAKSeidmanADUse of the Oncotype DX 21-gene assay to guide adjuvant decision making in early-stage breast cancerMolecular diagnosis & therapy200711635536018078353
- RodriguezBAChengASYanPSEpigenetic repression of the estrogen-regulated Homeobox B13 gene in breast cancerCarcinogenesis20082971459146518499701
- WeiYXiaWZhangZLoss of trimethylation at lysine 27 of histone H3 is a predictor of poor outcome in breast, ovarian, and pancreatic cancersMolecular carcinogenesis200847970170618176935
- KimSJNakayamaSMiyoshiYDetermination of the specific activity of CDK1 and CDK2 as a novel prognostic indicator for early breast cancerAnnals of oncology: official journal of the European Society for Medical Oncology / ESMO2008191687217956886
- HanHJRussoJKohwiYKohwi-ShigematsuTSATB1 reprogrammes gene expression to promote breast tumour growth and metastasisNature2008452718418719318337816
- Ben-PorathIThomsonMWCareyVJAn embryonic stem cell-like gene expression signature in poorly differentiated aggressive human tumorsNat Genet200840549950718443585
- ParkerJSMullinsMCheangMCSupervised risk predictor of breast cancer based on intrinsic subtypesJ Clin Oncol20092781160116719204204
- SungJYKimRKimJELeeJBalance between SIRT1 and DBC1 expression is lost in breast cancerCancer Sci201010171738174420412117
- GevenslebenHGohringUJButtnerRComparison of MammaPrint and TargetPrint results with clinical parameters in German patients with early stage breast cancerInternational journal of molecular medicine201026683784321042777
- LaniganFGremelGHughesRHomeobox transcription factor muscle segment homeobox 2 (Msx2) correlates with good prognosis in breast cancer patients and induces apoptosis in vitroBreast Cancer Res2010124R5920682066
- CreightonCJFuXHennessyBTProteomic and transcriptomic profiling reveals a link between the PI3K pathway and lower estrogen-receptor (ER) levels and activity in ER+ breast cancerBreast Cancer Res2010123R4020569503
- XuHYanMPatraJEnhanced RAD21 cohesin expression confers poor prognosis and resistance to chemotherapy in high grade luminal, basal and HER2 breast cancersBreast Cancer Res2011131R921255398
- LittlepageLEAdlerASKouros-MehrHThe transcription factor ZNF217 is a prognostic biomarker and therapeutic target during breast cancer progressionCancer discovery20122763865122728437
- PitrodaSPZhouTSweisRFTumor endothelial inflammation predicts clinical outcome in diverse human cancersPLoS One2012710e4610423056240
- KimWKimKSLeeJEDevelopment of novel breast cancer recurrence prediction model using support vector machineJournal of breast cancer201215223023822807942
- FarynaMKonermannCAulmannSGenome-wide methylation screen in low-grade breast cancer identifies novel epigenetically altered genes as potential biomarkers for tumor diagnosisFASEB J201226124937495022930747
- FaschingPAPharoahPDCoxAThe role of genetic breast cancer susceptibility variants as prognostic factorsHuman molecular genetics201221173926393922532573
- HuangYZhangHCaiJOverexpression of MACC1 and Its significance in human Breast Cancer ProgressionCell & bioscience2013311623497677
- YangXVasudevanPParekhVPenevACunninghamJMBridging cancer biology with the clinic: relative expression of a GRHL2-mediated gene-set pair predicts breast cancer metastasisPLoS One201382e5619523441166
- NagataTShimadaYSekineSPrognostic significance of NANOG and KLF4 for breast cancerBreast Cancer20122119610122528804
Disclosure
The authors report no conflicts of interest in this work.
References
- RavnanMCRavnanSLWalbergMPMetastatic breast cancer: a review of current and novel pharmacotherapyFormulary201146130146
- PuenteJLópez-TarruellaSRuizAPractical prognostic index for patients with metastatic recurrent breast cancer: retrospective analysis of 2,322 patients from the GEICAM Spanish El Alamo RegisterBreast Cancer Res Treat2010122259160020063196
- GruverAMPortierBPTubbsRRMolecular pathology of breast cancer: the journey from traditional practice toward embracing the complexity of a molecular classificationArch Pathol Lab Med2011135554455721526953
- BunessAKunerRRuschhauptMPoustkaASültmannHTreschAIdentification of aberrant chromosomal regions from gene expression microarray studies applied to human breast cancerBioinformatics200723172273228017599933
- RennstamKAhlstedt-SoiniMBaldetorpBPatterns of chromosomal imbalances defines subgroups of breast cancer with distinct clinical features and prognosis. A study of 305 tumors by comparative genomic hybridizationCancer Res200363248861886814695203
- Cancer Genome Atlas NetworkComprehensive molecular portraits of human breast tumoursNature20124907418617023000897
- van ’t VeerLJDaiHvan de VijverMJGene expression profiling predicts clinical outcome of breast cancerNature2002415687153053611823860
- PaikSShakSTangGA multigene assay to predict recurrence of tamoxifen-treated, node-negative breast cancerN Engl J Med2004351272817282615591335
- ParkerJSMullinsMCheangMCSupervised risk predictor of breast cancer based on intrinsic subtypesJ Clin Oncol20092781160116719204204
- ShahSPRothAGoyaRThe clonal and mutational evolution spectrum of primary triple-negative breast cancersNature2012486740339539922495314
- RochéHCancers du sein : évolution des grands concepts thérapeutiques [Evolving strategies over time for treatment of breast cancer]Bull Cancer20131009857863 French24004610
- RakhaEAPitfalls in outcome prediction of breast cancerJ Clin Pathol201366645846423618694
- RakhaEAReis-FilhoJSBaehnerFBreast cancer prognostic classification in the molecular era: the role of histological gradeBreast Cancer Res201012420720804570
- de BoerMvan DijckJABultPBormGFTjan-HeijnenVCBreast cancer prognosis and occult lymph node metastases, isolated tumor cells, and micrometastasesJ Natl Cancer Inst2010102641042520190185
- McGuireWLClarkGMPrognostic factors and treatment decisions in axillary-node-negative breast cancerN Engl J Med199232626175617611594018
- RogersCELovedayRLDrewPJGreenmanJMolecular prognostic indicators in breast cancerEur J Surg Oncol200228546747812217298
- Ben-PorathIThomsonMWCareyVJAn embryonic stem cell-like gene expression signature in poorly differentiated aggressive human tumorsNat Genet200840549950718443585
- Adam MaciejczykANew prognostic factors in breast cancerAdv Clin Exp Med201322151523468257
- CudaGCannataroMQuaresimaBProteomic profiling of inherited breast cancer: identification of molecular targets for early detection, prognosis and treatment, and related bioinformatics toolsApolloniBMarinaroMTagliaferriRNeural Nets2859Berlin, GermanySpringer2003245257
- XuHYanMPatraJEnhanced RAD21 cohesin expression confers poor prognosis and resistance to chemotherapy in high grade luminal, basal and HER2 breast cancersBreast Cancer Res2011131R921255398
- LaniganFGremelGHughesRHomeobox transcription factor muscle segment homeobox 2 (Msx2) correlates with good prognosis in breast cancer patients and induces apoptosis in vitroBreast Cancer Res2010124R5920682066
- HrstkaRNenutilRFourtounaAThe pro-metastatic protein anterior gradient-2 predicts poor prognosis in tamoxifen-treated breast cancersOncogene201029344838484720531310
- KalbfleischJDPrenticeRLThe Statistical Analysis of Failure Time DataHoboken, NJJohn Wiley & Sons, Inc1980
- LisboaPJWongHHarrisPSwindellRA Bayesian neural network approach for modelling censored data with an application to prognosis after surgery for breast cancerArtif Intell Med200328112512850311
- KimWKimKSLeeJEDevelopment of novel breast cancer recurrence prediction model using support vector machineJ Breast Cancer201215223023822807942
- MassaguéJSorting out breast-cancer gene signaturesN Engl J Med2007356329429717229957
- FuJJeffreySSTranscriptomic signatures in breast cancerMol Biosyst20073746647217579771
- VenetDDumontJEDetoursVMost random gene expression signatures are significantly associated with breast cancer outcomePLoS Comput Biol2011710e100224022028643
- Reis-FilhoJSPusztaiLGene expression profiling in breast cancer: classification, prognostication, and predictionLancet201137898051812182322098854
- GeeJMNicholsonRIExpanding the therapeutic repertoire of epidermal growth factor receptor blockade: radiosensitizationBreast Cancer Res20035312612912793892
- LacombeJMangeAAzriaDSolassolJIdentification de marqueurs prédictifs de la réponse à la radiothérapie par approche protéomique [Identification of predictive biomarkers to radiotherapy outcome through proteomics approaches]Cancer Radiother20131716269 quiz 70, 72. French23287796
- KronenwettUPlonerAZetterbergAGenomic instability and prognosis in breast carcinomasCancer Epidemiol Biomarkers Prev20061591630163516985023
- HanHJRussoJKohwiYKohwi-ShigematsuTSATB1 reprogrammes gene expression to promote breast tumour growth and metastasisNature2008452718418719318337816
- SivaraksaMLoweDPredictive gene lists for breast cancer prognosis: a topographic visualisation studyBMC Med Genomics20081818419801
- AnunciaçãoOGomesBCVingaSGasparJOliveiraALRueffJA data mining approach for the detection of high-risk breast cancer groupsAdv Bioinformatics2010744351
- ShiMZhangBSemi-supervised learning improves gene expression-based prediction of cancer recurrenceBioinformatics201127213017302321893520
- Gökmen-PolarYBadveSBreast cancer prognostic markers: where are we now?MLO Med Lab Obs201244922242523002506
- HarrisLFritscheHMennelRAmerican Society of Clinical OncologyAmerican Society of Clinical Oncology 2007 update of recommendations for the use of tumor markers in breast cancerJ Clin Oncol200725335287531217954709
- SegalCVDowsettMEstrogen receptor mutations in breast cancer – new focus on an old targetClin Cancer Res20142071724172624583794
- SlodkowskaEARossJSMammaPrint 70-gene signature: another milestone in personalized medical care for breast cancer patientsExp Rev Mol Diagn200995417422
- GevenslebenHGöhringUJBüttnerRComparison of MammaPrint and TargetPrint results with clinical parameters in German patients with early stage breast cancerInt J Mol Med201026683784321042777
- ConlinAKSeidmanADUse of the Oncotype DX 21-gene assay to guide adjuvant decision making in early-stage breast cancerMol Diagn Ther200711635536018078353
- PratAParkerJSFanCPerouCMPAM50 assay and the three-gene model for identifying the major and clinically relevant molecular subtypes of breast cancerBreast Cancer Res Treat2012135130130622752290
- KellyCMBernardPSKrishnamurthySAgreement in risk prediction between the 21-gene recurrence score assay (Oncotype DX®) and the PAM50 breast cancer intrinsic Classifier™ in early-stage estrogen receptor-positive breast cancerOncologist201217449249822418568
- AlbainKSPaikSvan’t VeerLPrediction of adjuvant chemotherapy benefit in endocrine responsive, early breast cancer using multigene assaysBreast200918Suppl 3S141S14519914534
- SotiriouCPusztaiLGene-expression signatures in breast cancerN Engl J Med2009360879080019228622
- LiJLenferinkAEDengYIdentification of high-quality cancer prognostic markers and metastasis network modulesNat Commun201013420975711
- CreightonCJFuXHennessyBTProteomic and transcriptomic profiling reveals a link between the PI3K pathway and lower estrogen-receptor (ER) levels and activity in ER+ breast cancerBreast Cancer Res2010123R4020569503
- CunYFröhlichHFPrognostic gene signatures for patient stratification in breast cancer: accuracy, stability and interpretability of gene selection approaches using prior knowledge on protein-protein interactionsBMC Bioinformatics2012136922548963
- HofreeMShenJPCarterHGrossAIdekerTNetwork-based stratification of tumor mutationsNat Methods201310111108111524037242
- RaphaelBJDobsonJROesperLVandinFIdentifying driver mutations in sequenced cancer genomes: computational approaches to enable precision medicineGenome Med201461524479672
- PitrodaSPZhouTSweisRFTumor endothelial inflammation predicts clinical outcome in diverse human cancersPLoS One2012710e4610423056240
- YangXVasudevanPParekhVPenevACunninghamJMBridging cancer biology with the clinic: relative expression of a GRHL2-mediated gene-set pair predicts breast cancer metastasisPLoS One201382e5619523441166
- NairRRodenDLTeoWSc-Myc and Her2 cooperate to drive a stem-like phenotype with poor prognosis in breast cancerOncogene Epub9232013
- NagataTShimadaYSekineSPrognostic significance of NANOG and KLF4 for breast cancerBreast Cancer20142119610122528804
- SungJYKimRKimJELeeJBalance between SIRT1 and DBC1 expression is lost in breast cancerCancer Sci201010171738174420412117
- SunYGoodisonSLiJLiuLFarmerieWImproved breast cancer prognosis through the combination of clinical and genetic markersBioinformatics2007231303717130137
- ShenROlshenABLadanyiMIntegrative clustering of multiple genomic data types using a joint latent variable model with application to breast and lung cancer subtype analysisBioinformatics200925222906291219759197
- EklundMSpjuthOWikbergJEAn eScience-Bayes strategy for analyzing omics dataBMC Bioinformatics20101128220504364
- VaskeCJBenzSCSanbornJZInference of patient-specific pathway activities from multi-dimensional cancer genomics data using PARADIGMBioinformatics20102612i237i24520529912
- FaschingPAPharoahPDCoxAThe role of genetic breast cancer susceptibility variants as prognostic factorsHum Mol Genet201221173926393922532573
- RafiqSTapperWCollinsAIdentification of inherited genetic variations influencing prognosis in early-onset breast cancerCancer Res20137361883189123319801
- FarynaMKonermannCAulmannSGenome-wide methylation screen in low-grade breast cancer identifies novel epigenetically altered genes as potential biomarkers for tumor diagnosisFASEB J201226124937495022930747
- ConnollyRStearnsVEpigenetics as a therapeutic target in breast cancerJ Mammary Gland Biol Neoplasia2012173–419120422836913
- DongPKaneuchiMKonnoYWatariHSudoSSakuragiNEmerging therapeutic biomarkers in endometrial cancerBioMed Res Int2013201313036223819113
- RundqvistHJohnsonRSTumour oxygenation: implications for breast cancer prognosisJ Intern Med2013274210511223844914
- MoisaAFritzPEckAGrowth/adhesion-regulatory tissue lectin galectin-3: stromal presence but not cytoplasmic/nuclear expression in tumor cells as a negative prognostic factor in breast cancerAnticancer Res2007274B2131213917695496
- PusztaiLIwamotoTBreast cancer prognostic markers in the post-genomic eraBreast Cancer Res Treat2011125364765020464478