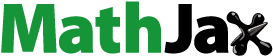
Abstract
The non-receptor tyrosine kinase proline-rich tyrosine kinase 2 (Pyk2) is a critical mediator of signaling from cell surface growth factor and adhesion receptors to cell migration, proliferation, and survival. Emerging evidence indicates that signaling by Pyk2 regulates hematopoietic cell response, bone density, neuronal degeneration, angiogenesis, and cancer. These physiological and pathological roles of Pyk2 warrant it as a valuable therapeutic target for invasive cancers, osteoporosis, Alzheimer’s disease, and inflammatory cellular response. Despite its potential as a therapeutic target, no potent and selective inhibitor of Pyk2 is available at present. As a first step toward discovering specific potential inhibitors of Pyk2, we used an in silico high-throughput screening approach. A virtual library of six million lead-like compounds was docked against four different high-resolution Pyk2 kinase domain crystal structures and further selected for predicted potency and ligand efficiency. Ligand selectivity for Pyk2 over focal adhesion kinase (FAK) was evaluated by comparative docking of ligands and measurement of binding free energy so as to obtain 40 potential candidates. Finally, the structural flexibility of a subset of the docking complexes was evaluated by molecular dynamics simulation, followed by intermolecular interaction analysis. These compounds may be considered as promising leads for further development of highly selective Pyk2 inhibitors.
Introduction
The focal adhesion kinase (FAK) and its homologous FAK-related proline-rich tyrosine kinase 2 (Pyk2) define a distinct family of non-receptor tyrosine kinases that coordinate adhesion and cytoskeletal dynamics with survival and growth signaling. FAK and Pyk2 exhibit ~48% amino acid sequence identity, common phosphorylation sites, and a similar domain structure, which includes an N-terminal four-point one, ezrin, radixin, and moesin (FERM) domain, a kinase domain, three proline-rich regions, and a C-terminal focal adhesion-targeting (FAT) domain. Following integrin or growth factor stimulation, Pyk2 and FAK are autophosphorylated on a tyrosine residue (Y402 and Y397, respectively), which provides a critical binding site for Src kinase. Following its binding, Src phosphorylates additional tyrosines on Pyk2 or FAK, which are important for full activation of the kinases and for their binding to downstream signaling proteins.Citation1 Although FAK is expressed in most cells, Pyk2 exhibits a more restricted expression pattern with the strongest expression in the central nervous system and in hematopoietic cells.Citation2 FAK is a major intracellular signaling component of integrin-mediated cell adhesion and plays a role in signaling pathways mediated by growth factor receptors. PYK2, however, is activated by a variety of extracellular cues, including agonists of G protein-coupled receptors, increase in intracellular calcium concentration, inflammatory cytokines, and stress signals, as well as integrin-mediated cell adhesion.Citation2–Citation4 The differential expression and localization patterns of FAK versus Pyk2 might limit their functional redundancy and may suggest distinct and possibly antagonistic roles in cells.
Pyk2 appears to be important for the organization of cytoskeletal components in a polarized manner during directional motility in response to chemotactic gradients in macrophagesCitation5 and in B cellsCitation6 and for integrin-mediated degranulation response of neutrophils during infection.Citation7 Deletion of Pyk2 in mice leads to increase in bone mass due to enhanced differentiation and activity of osteoprogenitor cellsCitation8 as well as impairment in osteoclast function.Citation9 Pyk2 was also found to regulate skin wound healing by controlling epidermal keratinocyte migration via a pathway that requires the expression and function of matrix metalloproteinases.Citation10 The gene encoding Pyk2 was recently found as one of 11 new susceptibility loci for late-onset Alzheimer’s diseaseCitation11 and as an in vivo marker and modulator of tau toxicity.Citation12 Upregulation of Pyk2 expression has been noted in several human tumors, including glioma, hepatocellular carcinoma, nonsmall cell lung carcinoma, prostate cancer,Citation13 and early and advanced breast cancers.Citation14 The different physiological and pathological roles of Pyk2 present it as a high-value therapeutic target for inflammatory cellular responses, osteoporosis, Alzheimer’s disease, and invasive cancers.
An increase in Pyk2 expression accompanied by a compensatory function for Pyk2 upon FAK loss has been described in FAK-deficient mouse embryonic fibroblasts (MEFs),Citation4,Citation15 following conditional deletion of FAK in macrophages;Citation16 in the in vivo regulation of bone architecture and density;Citation17 and in blood vessel formation during angiogenesis.Citation18 The ability of Pyk2 to adopt a compensatory role in integrin-mediated signaling pathways suggests that strategies that will selectively target FAK might lack efficacy due to Pyk2 compensation, particularly in applications directed toward blocking inflammatory response, osteoporosis, and tumor angiogenesis.
Clinical translation of kinase inhibitors has mainly focused on competitive inhibition of the catalytic domain. While classical kinase inhibitors bind directly to the ATP binding site, this approach has been challenged by the significant sequence and structure conservation of the catalytic domains among different protein kinases. With the exception of cancer therapeutics, where inhibition of multiple kinase targets might gain additional therapeutic benefits, minimizing off-target activity is most often desired in drug design. Therefore, an alternative approach has been the use of bipartite inhibitors that target both the adenosine triphosphate (ATP)-binding site and a less conserved adjacent hydrophobic region formed by the altered conformation of the activation loop containing the DFG (Asp–Phe–Gly) motif. Inhibitors of this group stabilize the inactive conformation of the kinase (DFG-out conformation) and offer a potential of improved selectivity.
Despite significant efforts to develop a potent and selective inhibitor for Pyk2 over the last several years, the available inhibitors for Pyk2 lack the potency, selectivity, or have impaired pharmacokinetics,Citation19,Citation20 and no selective inhibitor has yet proceeded beyond pre-clinical trials, including Pfizer’s lead compound (S)-14a from the series of sulfoximine-substituted molecules. In spite of its increased selectivity for Pyk2 over FAK, N-methylsulfoximine (S)-14a lacks sufficient metabolic stability and is characterized by high in vivo clearance in rats.Citation20
In this study, we used an in silico high-throughput screening approach in order to identify potential Pyk2 kinase inhibitors from a large library of small molecules. Results of these studies uncovered potential lead-like molecules that were subjected to comparative docking and free binding energy calculations, followed by molecular dynamics (MD) simulations in order to identify compounds that are predicted to have affinity and selectivity for Pyk2. A final set of potential candidates with predicted selectivity for Pyk2 was assembled. These candidates may be considered as lead compounds that could be further developed into potent and selective Pyk2 inhibitors.
Methods
Database selection and ligand preparation
We used the commercially available ZINC12 database (http://zinc.docking.org/)Citation21 for virtual screening, which contains more than 35 million purchasable compounds in ready-to-dock, three-dimensional formats. The lead-like subset of compounds, containing six million compounds, was selected on the basis of their properties to allow further optimization. The lead-like criteria properties are molecular weight between 250 and 350 g/mol, predicted partition constant (xLogP) ≤3.5, and number of rotatable bonds (RBs) ≤7. The subset was processed by RaccoonCitation22 utility where missing polar hydrogen atoms were added.
Target preparation and grid generation
Four high-resolution crystal structures of human Pyk2 kinase domain with distinct conformations of the active site and activation loop were selected for our high-throughput in silico screening: 1) apo-Pyk2 (Protein Data Bank [PDB] ID: 3FZO); 2) Pyk2 in complex with the inhibitor PF-431396 (PDB ID: 3FZR); 3) Pyk2 in complex with the inhibitor PF-4618433 (PDB ID: 3FZT);Citation19 and 4) Pyk2 in complex with N-methylsulfonamide 2a (PDB ID: 3H3C).Citation20 The resolutions of the target PDB structures 3FZO, 3FZR, 3FZT, and 3H3C were 2.2, 2.7, 1.95, and 2.0 Å, respectively. Prior to docking simulations, missing side chains and loops were filled using PrimeCitation23 and termini were capped. Hydrogen atoms were added, bond orders were assigned, and ions were removed using the Protein Preparation Wizard.Citation24 Crystallographic water molecules were removed as they contained less than three hydrogen bonds after sampling their orientations at pH 7 using PROPKACitation25 and optimizing their hydrogen bonds. Following this step, the holo form of the structures was minimized by a restrained energy minimization using the OPLS2005 force field and default root-mean-square deviation (RMSD) constraint of 0.3 Å. Refined structures were imported to AutoDockToolsCitation26 where Gasteiger charges were assigned to all atoms and non-polar hydrogen atoms were merged. For each target, three-dimensional affinity grids of dimensions up to 30×18×26 Å were centered around the active site with 1.0 Å spacing.
In silico high-throughput screening
AutoDock VinaCitation27 was used for the docking simulation. The settings of exhaustiveness for finding the global minimums were defined as 4, and only the best ranked poses were retrieved. The screening calculations ran on a double-threaded 480 CPU 2.67 GHz Xeon Linux cluster machine.
Efficiency metrics scoring
To promote hits with favorable affinity and pharmacokinetics, several indices were utilized. The mean accumulated binding (MAB) index was developed to assess the contribution of multiple conformational binding of a molecule without impairing other valid molecules that are predicted to bind fewer structures.
Surface-binding efficiency index (SEI) quantifies the efficiency of the binding affinity based on total polar surface area (TPSA).Citation28
Lipophilic Ligand Efficiency (LLE) estimates the specificity of a molecule in binding to the target relative to the calculated partition constant (xLogP).Citation29
Pareto score optimizes multiple objectives, BEI versus SEI (BvS) simultaneously in a trade-off approach.Citation28
Visual inspection
The top-ranked molecules containing undesirable chemical groups and substructures,Citation30 including toxic and highly metabolized molecules, were filtered via visual inspection. Molecules containing more than four aliphatic or aromatic rings were filtered as too many rings pose a liability in drug design.Citation31,Citation32 In addition, compounds containing more than two merged aromatic rings were excluded to avoid highly planar structures.Citation33,Citation34
Selectivity evaluation and screening
The 240 top filtered molecules were submitted for a second screen in AutoDock Vina and GlideCitation35 to collect the compounds that are selective for Pyk2 compared to FAK. The crystal structures of Pyk2 (PDB ID: 3FZT and 3H3C) and FAK (PDB ID: 1MP8) were prepared as described earlier, including the grid generation for docking in Vina. As a preparation step for docking in Glide, the molecules were prepared using LigPrep WizardCitation36 and grids with similar box size were generated in the Receptor Grid Generation of Schrodinger.Citation37
To assess the docking score more reliably, exhaustiveness in Vina was increased to 8 and the extra-precision (XP) mode was used in Glide. The resulting poses predicted in Glide XP for Pyk2 and FAK were rescored and compared using Prime MM-GBSA (molecular mechanics/generalized born surface area). Prime MM-GBSA is a physics-based method that calculates the force field energies of the bound and unbound states of the protein–ligand complex.Citation38 The van der Waals surface-based surface generalized born 2.0 implicit solvation model was used with the OPLS2005 force field, and residue flexibility was defined throughout the structure. The MM-GBSA binding free energy is defined as follows:Citation39,Citation40
ΔEmm is the difference in energy between the complex structure and the sum of the energies of the unbound ligand and protein. ΔGsol is the difference in the solvation energy of the complex and the sum of the solvation energies for the unbound ligand and protein. ΔGsa is the difference in the surface area energy for the complex and the sum of the surface area energies for the unbound ligand and protein.Citation40 Entropy terms related to the ligand and protein are not incorporated, due to the expensive computational demand and no apparent improvement in many cases.Citation41,Citation42
MD simulation
The final drug candidates were submitted to MD simulation performed using the Desmond packageCitation43 and OPLS2005 force field. The docking models of the most selective ligands as calculated by MM-GBSA were used as the initial coordinates for the MD calculation. The TIP3 explicit water model was used to define the solvent.Citation44 The simulation solvent cell box with periodic boundary conditions was set to orthorhombic shape with a buffer distance of 10 Å to each dimension. The system was neutralized by placing counter ions of 6Na+ and 3Cl− for the structures of Pyk2 and FAK, respectively, and background salt was added to the solvent with a concentration of 0.15 M.Citation45 Prior to the production of molecular dynamic simulations, the system was equilibrated using the default Desmond relaxation protocol. The final state of the system was set to 300 K and 1 atm,Citation39 and the equations of motions were integrated with a 2 fs time step.Citation46 The simulations were performed in the NPT (constant number of atoms, pressure, temperature) ensemble using Nosé–Hoover thermostat with 1 ps relaxation time and Martyana–Tobias–Klein barostat with a 2 ps relaxation time.Citation47 The smooth particle mesh Ewald (PME) algorithmCitation48 was used to deal with long-range electrostatic interactions with a cutoff radius of 9 Å.Citation49 The production of 20 ns long MD simulations was performed on each complex system, and dynamic trajectories were analyzed in Desmonds’ Maestro simulation analysis tools and MATLAB. Quantitative analysis of the ligand–protein molecular interactions was calculated over the MD simulation. The distances as defined in Desmond for hydrogen bonds were <2.5 Å. General hydrophobic interaction distances were within 3.6 Å, and π–cation and π–π distances were within 4.5 Å. Ionic interaction distances were within 3.7 Å, and hydrogen bonding via water bridge molecule distance was <2.7 Å.
Results
In silico high-throughput screening
In order to identify potential inhibitors of Pyk2 kinase domain, an in silico high-throughput screening approach was used. To enhance the sampling of the target conformational space, four different Pyk2 kinase domain crystallographic structures with unique conformations were processed and prepared for in silico screening. The four crystal structures represent different conformations that were induced by ligands that have different selectivity profiles: 1) 3FZO is the apo, unbound state;Citation19 2) 3FZR adopts an active conformation state (DFG-in) and contains an ATP-mimetic inhibitor, PF-431396, with a low selectivity to Pyk2;Citation19 3) 3FZT adopts an inactive conformation (DFG-out) and contains an allosteric inhibitor, PF-4618433, selective to Pyk2;Citation19 and 4) 3H3C adopts an active form (DFG-in) and contains the inhibitor N-methylsulfonamide 2a, which is selective to Pyk2.Citation20 The two-dimensional interaction plots of the ligands are presented in . A library of more than six million purchasable lead-like molecules from the ZINC database was screened against the binding pockets of each of the four structures (active site grids). The primary criterion used to select for initial set of molecules was binding free energy values (ΔG) calculated by AutoDock Vina. A histogram of the affinity scores for 10,000 molecules for each of the structures is plotted in and depicts the highest scores for 3FZT and 3H3C, followed by 3FZR and 3FZO in a semi-logarithmic scale.
Figure 1 Histogram of binding affinities for the top 10,000 compounds docked to Pyk2.
Abbreviation: Pyk2, proline-rich tyrosine kinase 2.
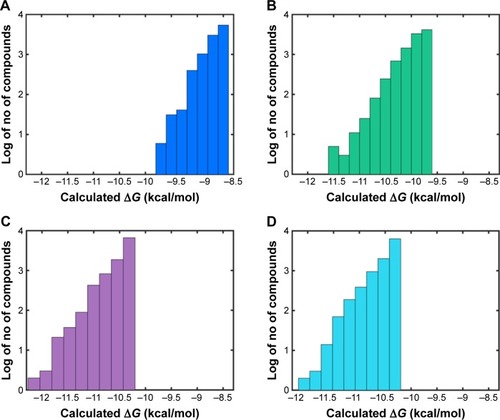
To compare the affinities of the screened compounds to affinities of existing Pyk2 inhibitors, we docked the known inhibitors into their corresponding structures using AutoDock Vina. The calculated binding energies of the reference compounds ranged from −8.7 to −9.3 kcal/mol, which was similar to the range engaged by the top 10,000 molecules for the apo structure 3FZO and was higher than all the top compounds of the rest of the three structures. Therefore, the cutoff for our docking studies was set to include the top 10,000 molecules with the highest affinity for each of the four structures and considered for further evaluation, composing a pool of 40,000 docked modes.
To insure proper target preparation, we calculated the RMSD between the crystallographic poses and the docked poses of PDB IDs 3FZR, 3FZT, and 3H3C (). The RMSD values of the reconstituted pose ranged from 0.41 to 2.57 Å, supporting the target preparation for docking protocols. To support the goodness of predictive docking screens, enrichment experiments were carried out. First, we collected a dataset comprising 124,000 decoys from the Directory of Useful Decoys (DUD) databaseCitation50 and 18 actives known from the literatureCitation20 via Konstanz Information Miner (KNIME). In addition, we collected another dataset containing 800 decoys generated by the DUD-enhanced (DUD-E) serverCitation51 based on the 18 actives. These two datasets were then screened against all four Pyk2 target structures using AutoDock Vina. The docking enrichment plots for four protein targets are shown in . The docking enrichment plots show that the percentage of true ligands found by docking, at any given percentage, of the docking-ranked database is almost always greater compared to being chosen by random selection.
Compound processing and ranking
We analyzed the retrieved compounds by combining the four sets of compounds to a single pool of compounds and scored via affinity and efficiency-based metrics: binding energy (ΔG), MAB, BEI, SEI, and LLE. The top 1,000 molecules for each index were ranked by the corresponding value and are presented as scatter plots in . In an attempt to identify top hits, which are suitable for drug development, and to remove false positives, we used several sub-structural filters by manual review to remove unwanted groups.Citation30 Owing to the potential detrimental effect of polycyclic compounds with a high ring count,Citation31 molecules were additionally filtered out and restricted to a maximal limit of aromatic and aliphatic rings.
Figure 2 Index scores of top 1,000 compounds docked to Pyk2.
Abbreviations: Pyk2, proline-rich tyrosine kinase 2; MAB, mean accumulated binding; BEI, binding efficiency index; SEI, surface-binding efficiency index; LLE, lipophilic ligand efficiency; BvS, BEI versus SEI.
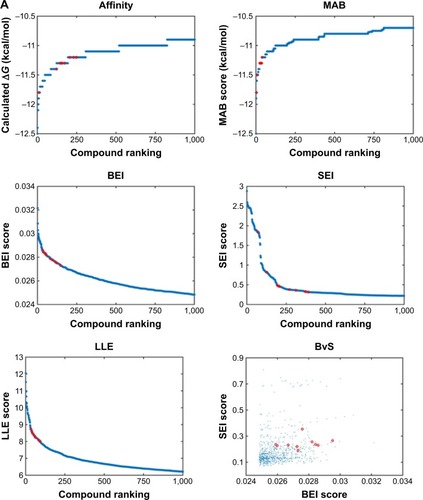
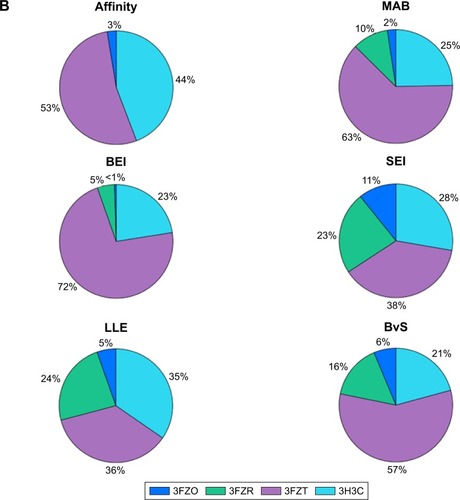
An additional approach for compound selection is the Pareto-based ranking scheme. The Pareto-based ranking scheme takes into account multiple objectives for the optimization/selection processCitation52 and combines their separate contribution in the final ranking. The multiple objectives, BEI and SEI, were optimized by scoring each compound with the sum of molecules dominating it in any of the indices. The top 10 filtered molecules retrieved for every index and the mapping of BvS indices for the top 1,000 molecules are presented in . Pareto ranking is a strategy particularly well suited for competitive multi-objective problems. In many cases, the BEI and SEI objectives are correlated; however, this is not always true,Citation53 and in this study, BEI and SEI were considered as partially competitive multi-object problems.
To visualize target distribution of compounds that displayed the best docking scores and efficiency values for every parameter, a chart summarizing the distribution of the top 1,000 compounds binding to a specific structure is depicted in , where binding is defined as any docking score within the top 10,000 molecules of the corresponding structure. The resulting distribution revealed that 3FZT that possessed a DFG-out conformation was a dominating structure for the majority of the compounds.
Selection of the highest-ranked compounds
From each index, 40 molecules were independently collected for further examination. The two-dimensional structures and molecular weights of the top 5 filtered candidates with the highest ranking per index are shown in , and their physicochemical properties along with the calculated indices are shown in . The total 240 compounds were evaluated for appearance in the literature using KNIMECitation54 nodes, which facilitate querying and retrieving data from the ChEMBLCitation55 bioactivity database via RESTful Web Services.Citation56 These molecules were not detected as known inhibitors for Pyk2.
Table 1 Top 5 filtered predicted hits ranked by six indices
Table 2 Molecular properties and index scores of the five highest ranked filtered compounds for Pyk2
Selectivity prediction by calculation of binding free energies
To evaluate the potential specificity for Pyk2 over FAK among the top retrieved predicted filtered hits, two different approaches were used. To predict the difference in binding association with the targets, we performed a second docking screen with a higher precision using increased exhaustiveness in Vina and Glide XP modes and the generated docked mode of Glide was utilized as an input to additional binding free energy scoring using Prime MM-GBSA. By implementing more precise docking methodologies and combining MM-GBSA, which outperforms docking in predicting the binding affinities to experimental data,Citation57 we can predict more reliably the differences in ligand-binding efficiencies of Pyk2 versus FAK. The correlation coefficient between calculated and experimental binding of Prime MM-GBSA, based on a diverse set of 855 complexes, was reported to be R2=0.63.Citation58 Since the predominant Pyk2 structure that bound the compounds contains a DFG-out conformation, a high-resolution crystal structure of FAK domain that possesses a DFG-out conformation was selected (PDB ID: 1MP8).Citation59 The FAK structure was imported and prepared for docking and was then used as a reference for estimating the selectivity of the top 240 compounds that were selected as mentioned earlier. For selectivity prediction, both the DFG-in and DFG-out conformations were used (PDB ID: 3FZT and 3H3C), as the predicted Vina scores of cognate ligands for both conformations were similar. Only 40 compounds that resulted in favorable binding to Pyk2, as calculated by the difference in at least 2 out of 3 binding energies using Vina, Glide, and Prime MM-GBSA software, were further processed. Using these methods, we selected 20 potential inhibitors ranked by MM-GBSA for 3FZT (ZINC06232011, ZINC02529497, ZINC01646132, ZINC15952140, ZINC18700196, ZINC00173518, ZINC00217347, ZINC35514633, ZINC04975487, ZINC07503677, ZINC03358424, ZINC05078298, ZINC09550062, ZINC03421151, ZINC65845103, ZINC02644767, ZINC03341864, ZINC00269705, ZINC10556478, and ZINC06648526) and 20 potential inhibitors ranked by MM-GBSA for 3H3C (ZINC06232011, ZINC02529497, ZINC18700196, ZINC01646132, ZINC15952140, ZINC05244105, ZINC71894482, ZINC08104814, ZINC00094214, ZINC58514284, ZINC04842554, ZINC00470121, ZINC64790378, ZINC67630577, ZINC00004724, ZINC25251328, ZINC00782941, ZINC82137153, ZINC05626761, and ZINC00617844). Notably, the top 5 predicted selective candidates for 3H3C were among the top 8 selective compounds for 3FZT. Results showed that ZINC06232011 was predicted to be the most selective ligand based on MM-GBSA scoring with Pyk2 (PDB ID 3FZT) ΔG of −35.37 kcal/mol and FAK ΔG of −5.50 kcal/mol, which results in ΔΔG of −29.87 kcal/mol. The top eight candidates (PDB ID 3FZT) were ranked by the energy difference MM-GBSA ΔΔG, and their two-dimensional structures with calculated binding energies are presented in . Although MM-GBSA was the main method to rank the compounds, it should be pointed out that the procedure used a continuum model of the solvent and this approximation can strongly affect the calculated binding energies, which may result in unreliable results.Citation57
Table 3 Candidates docking and MM-GBSA binding energy of Pyk2 and FAK ligand complexes
To support the predictive goodness of this selectivity assay, we compared the predicted binding energy and interaction profile of the native control compounds of PDB IDs 3FZR (PF-431396), 3FZT (PF-4618433), and 3H3C (N-methylsulfonamide 2a) with those obtained using experimental data (). The predicted binding energies agreed with experimental activity assays, and the interaction profile was similar. 3FZR displayed the smallest predicted selectivity (largest ΔΔG), followed by 3H3C and 3FZT. By consensus, the predicted ΔΔG ranked the cognate ligands according to experimental data and thus substantiated our techniques for finding potential selective Pyk2 inhibitors.
Analysis of the identified molecules
The binding poses of the top candidate compounds bound to Pyk2 (3FZT) as predicted by MM-GBSA are given in , and two-dimensional interaction plots are presented in . Docking pose analysis revealed one hydrogen bond between Tyr505 and ZINC06232011, ZINC01646132, and ZINC00217347, in which the last two form π–π interactions with Phe568. Also observed were two hydrogen bonds of ZINC02529497 with Asp567 and with Glu474, respectively, as well as a cation–π interaction with Arg572. Compounds ZINC159521402, ZINC00173518 and ZINC97378786 were involved in a similar interaction forming two hydrogen bonds with Glu474 and one hydrogen bond to Asp657, while the last compound also formed π–π interaction with His547. Interestingly, ZINC18700196 was located furthest away from the ATP-binding site and formed a total of four hydrogen bonds with residues Lys457, Asp567, and Arg572, while still involved in π–π interaction with Phe436 and two cation–π interactions with Arg572. Molecular descriptors of physicochemical properties, ligand efficiency scores, and bound structures with the predicted highest binding affinity are presented in .
Figure 3 Binding poses of the eight candidates in the Pyk2 (PDB ID: 3FZT) binding site.
Abbreviations: Pyk2, proline-rich tyrosine kinase 2; PDB, Protein Data Bank; MM-GBSA, molecular mechanics/generalized Born surface area; DFG, Asp-Phe-Gly.
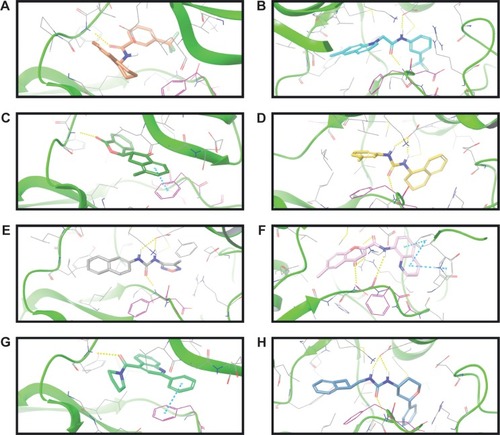
For selectivity prediction, both the DFG-in and DFG-out conformations were used. The predicted Vina scores of cognate ligands for the DFG-out and DFG-in were similar and differed by 1.0–1.5 kcal/mol (which is lower than Vina’s standard error of 2.85 kcal/mol).Citation27 Thus, we decided to use both DFG-in (PDB ID 3FZT) and DFG-out (PDB ID 3H3C) conformations.
An alternative way to interpret the contribution of each scoring profile is to visualize the ranking of the compound instead of its scoring value. The information is displayed in by radar plots, where the value of each property corresponds to the ranking of the score; closer to the center indicates a property with a good result, while far from the center fails to compete with the rest of the compounds.
Figure 4 Radar plot scores of the top 8 eight candidates for Pyk2 (PDB ID: 3FZT).
Abbreviations: Pyk2, proline-rich tyrosine kinase 2; PDB, Protein Data Bank; SEI, surface-binding efficiency index; LLE, lipophilic ligand efficiency; BvS, BEI versus SEI; MAB, mean accumulated binding; BEI, binding efficiency index.
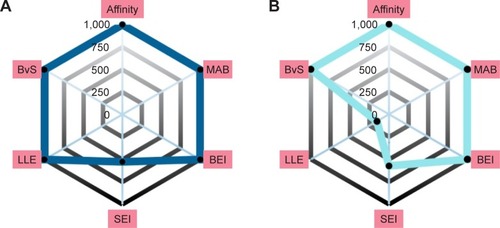

MD simulation
To take into account structural flexibility, the behavior of a subset of the predicted complexes of Pyk2 and FAK was compared by MD simulation. The top 8 Pyk2 DFG-out candidates were incorporated in Desmond, and MD simulation was performed in explicit aqueous solution for 20 ns for each complex (). To explore the dynamic stability, RMSD of protein–ligand complexes of Pyk2 (3FZT) and FAK (1MP8) against their initial structure was generated and analyzed using MATLAB. The backbone RMSDs were stable throughout the simulations, with the exception of compound ZINC02529497, where there was a sudden increase in deviation at 9 ns within the FAK complex ().
Figure 5 RMSDs during MD simulation of Pyk2 (3FZT) and FAK (1MP8) of protein–ligand complexes.
Abbreviations: RMSD, root-mean-square deviation; MD, molecular dynamics; Pyk2, proline-rich tyrosine kinase 2; FAK, focal adhesion kinase; RMSF, root-mean-square fluctuation.

Ligand positional RMSDs were generated to evaluate and compare the binding stability of the lead molecules for each of the targets. Pyk2 complexes demonstrated conformational stability of the ligand in which the RMSD values remained within 0.15 nm (), whereas half of the FAK complexes showed higher RMSD values throughout most of the simulation time (). The computed RMSD values of the ligands ZINC02529497, ZINC01646132, ZINC159521402, and ZINC97378786 in complex with FAK were >0.15 nm and reached 0.25 nm, indicating lower stability.
Root-mean-square fluctuations (RMSFs) were generated to evaluate and compare the residual mobility of Pyk2 and FAK while bound to each of the lead compounds. The RMSFs were integrated along the MD simulation time for each protein–ligand complex and were plotted against the residue number (). In all cases, the computed RMSFs of the residues were lower than 0.6 nm and did not produce any abnormal fluctuations, with the exception of ZINC02529497 and ZINC01646132, which produced fluctuations >0.6 nm in FAK ().
Intermolecular interaction analysis
To determine the stability of protein–ligand binding during the trajectory period of the MD simulation, the intermolecular interactions of ligands in complex with Pyk2 and FAK were analyzed. The intermolecular interaction analysis of the complexes was generated in Desmond and processed in MATLAB, and the time-dependent data were integrated for each interaction type to compare the results as illustrated in . The analysis revealed that ZINC18700196 was superior in binding to Pyk2 compared to FAK in all interaction types: hydrogen bond, hydrophobic, ionic, π–cation, π–π, and water bridge. ZINC159521402 exhibited a similar profile, excluding redundant ionic and π–π interactions, which are very low for Pyk2 and FAK. The compounds ZINC06232011, ZINC02529497, and ZINC97378786 bound preferably to Pyk2 in most of the interactions, particularly by hydrogen bonds and hydrophobic interactions, while π–π and water bridges had similar or lower values for Pyk2. The candidate molecules ZINC01646132 and ZINC00217347 maintained higher amount of hydrogen bonds to FAK, while ZINC00173518 showed more hydrophobic, ionic, and substantial increase in water bridge interactions to FAK. Overall, the MD simulations of the ligand complexes with Pyk2 display stability under dynamic conditions and the analysis supports the binding energy predictions.
Figure 6 Total number of intermolecular interactions of the candidates.
Abbreviations: Pyk2, proline-rich tyrosine kinase 2; FAK, focal adhesion kinase; MD, molecular dynamics; ZINC, Zinc is not commercial.

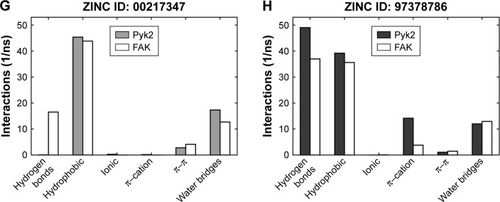
Discussion
The identification of a proper lead compound for a given molecular target is a critical step in the process of drug discovery. Traditional high-throughput screening of large chemical libraries has been a primary source for identification of novel lead compounds. However, the high cost and low hit rate, which are associated with high-throughput screening, have stimulated the development of computational alternatives and the broad application of the cheaper and faster in silico screening approach.Citation60 With an increasing number of targets being identified by high-throughput genomics and proteomics efforts, in silico screening may provide an excellent complementary approach to the traditional high-throughput screening and will potentially improve the speed and efficiency of drug discovery and development processes.
As part of our effort to identify novel candidates for Pyk2 (), potency and several efficiency metrics that attempt to define aspects of compound quality have been used. The tendency to focus on enhancing potency rather than practicing a more holistic approach in drug discovery was suggested to be responsible for failing drug discovery projects with molecules burdened by excessive molecular weight and lipophilicity, referred to as molecular obesity.Citation61 In order to avoid biasing toward molecules where the dominating attractor is potency, we allowed only part of the retrieved predicted hits to be beneficially prioritized for potency. Since the best performing single structure is not known in advance and using multiple fixed protein conformation is useful in predicting active compounds by docking calculations,Citation62,Citation63 we virtually screened four different Pyk2 structures and defined MAB to score the contribution of a well docking compound versus multiple conformations. With the increasing popularity of ligand efficiency indices () and increasing involvement in contemporary drug discovery,Citation64 we aimed to balance potency and ADMET (absorption, distribution, metabolism, excretion and toxicity) with BEI, SEI, LLE, and the combination of BEI and SEI. These indices are among the most used and robust metrics,Citation64–Citation66 and we have implemented them computationally with calculating the binding energy as a surrogate for in vitro potency, as has been proposed by Abad-Zapatero.Citation53 To our knowledge, this is the first study to test the efficiency metrics, including BEI, SEI, and LLE, in an in silico high-throughput screening approach that combines selectivity scoring methodologies and incorporates molecular docking, MM-GBSA, and MD.
Figure 7 Overview of the virtual screen workflow.
Abbreviations: Pyk2, proline-rich tyrosine kinase 2; FAK, focal adhesion kinase; PDB, Protein Data Bank; MD, molecular dynamics; ZINC, Zinc is not commercial.
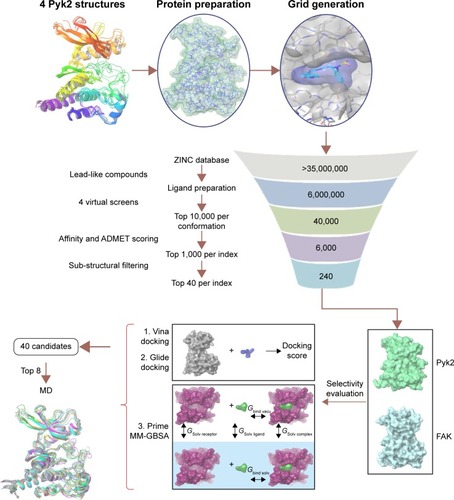
Despite the advances in computational aided drug design and widespread application of docking methods, there are still limitations that affect the accuracy of the predictions. These limitations include unavoidable imprecision of crystal structuresCitation67 and the lack of information about the number and free energy of water molecules.Citation57 In addition, the inhibition of kinase activity is not necessarily a direct indicator of binding affinity, and the idealized conditions of the docking simulations that predict binding free energies rarely reflect the conditions in experimental assays.Citation68
The top predicted selective candidates exhibit fairly low SEI scores, and the Pareto-based BvS score was mostly dominated by BEI, which is also demonstrated in . Among these candidates, LLE was the least enriched metric. Although the initial compound enrichment in the virtual screen was scored by potency, the affinity and MAB rankings show that most of the top predicted selective candidates were not among the top predicted potent compounds.
The high structural similarity of Pyk2 and FAK kinase domains may pose a challenge in discovering novel selective and potent inhibitors. Selective ligands with reduced potency for Pyk2 such as PF-4618433 were observed, and other studies have identified selective compounds but at the expense of potency.Citation69–Citation71 We aimed to avoid focusing mainly on enhancing potency and to fulfill our preconditions by using efficiency metrics; the compounds were subjected to more stringent criteria affecting their affinity. In addition, the affinity of the identified ligands was compromised by small molecular weight, lipophilicity, and polarity.
Clinical experience with kinase inhibitors has demonstrated that inhibition of protein tyrosine kinases should not rely exclusively on modulation of catalytic activity due to specificity issues and the unexpected emergence of resistance. Thus, combining kinase inhibition with approaches that inhibit extra-catalytic modules that regulate effector functions of tyrosine kinases would be a welcome asset to the therapeutic arena. In addition to its tyrosine kinase activity, Pyk2 has scaffolding functions in the formation of multi-protein signaling complexes. Therefore, targeting extra-catalytic protein modules that regulate complex assembly may provide a complementary approach for efficient and specific inhibition of Pyk2. Future studies that will characterize Pyk2-mediated signaling complex formation will be necessary in order to achieve this significant goal.
Acknowledgments
This work was supported by the Israel Science Foundation (grant number 996/12), the Israel Cancer Research Fund (grant number 12-709-RCDA), the Israel Cancer Association (grant number 20141043), the Marie Curie Career Integration Grant (grant number 321556), the Helmsley Charitable Trust Fund (grant number 2012PG-ISL013; to H.G.-H.), and the Leir Foundation and Katz Foundation (to A.O.S.).
Supplementary materials
Figure S1 Two-dimensional representation of intermolecular interactions of Pyk2 inhibitors with the Pyk2 kinase domain.
Abbreviations: Pyk2, proline-rich tyrosine kinase 2; PDB, Protein Data Bank.
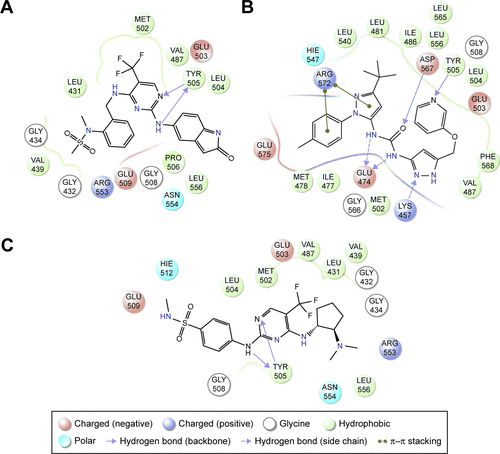
Figure S2 Predicted binding modes’ comparison of Pyk2 crystallographic ligands.
Abbreviations: Pyk2, proline-rich tyrosine kinase 2; PDB, Protein Data Bank.

Figure S3 Docking enrichment plots for four Pyk2 protein targets using DUD.
Abbreviations: Pyk2, proline-rich tyrosine kinase 2; DUD, Directory of Useful Decoys.
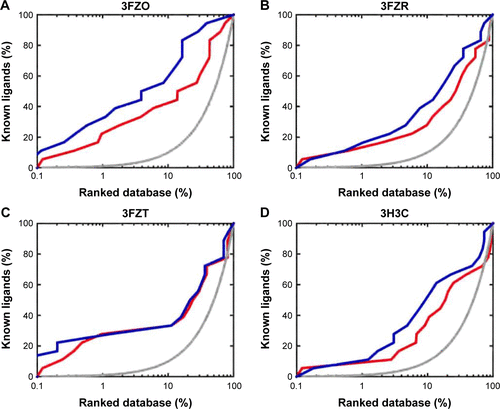
Figure S4 Two-dimensional representation of intermolecular interactions of the candidate ligands of Pyk2 with the Pyk2 kinase domain.
Abbreviations: Pyk2, proline-rich tyrosine kinase 2; ZINC, Zinc is not commercial.
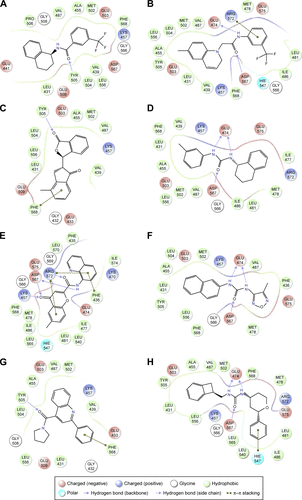
Figure S5 Increase in the number of annual publications using ligand-binding efficiency indices.
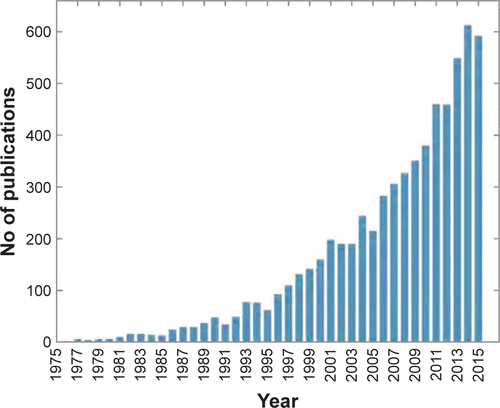
Table S1 Predicted binding energies of the cognate ligands
Table S2 Molecular properties and index scores of the top candidates for Pyk2 (PDB ID: 3FZT)
Disclosure
The authors report no conflicts of interest in this work.
References
- ParkSYAvrahamHKAvrahamSRAFTK/Pyk2 activation is mediated by trans-acting autophosphorylation in a Src-independent mannerJ Biol Chem200427932333153332215166227
- LevSMorenoHMartinezRProtein tyrosine kinase PYK2 involved in Ca(2+) induced regulation of ion channel and MAP kinase functionsNature199537665437377457544443
- LitvakVTianDShaulYDLevSTargeting of PYK2 to focal adhesions as a cellular mechanism for convergence between integrins and G protein-coupled receptor signaling cascadesJ Biol Chem200027542327363274610915788
- SiegDJIlicDJonesKCDamskyCHHunterTSchlaepferDDPyk2 and Src-family protein-tyrosine kinases compensate for the loss of FAK in fibronectin-stimulated signaling events but Pyk2 does not fully function to enhance FAK-cell migrationEMBO J19981720593359479774338
- OkigakiMDavisCFalascaMPyk2 regulates multiple signaling events crucial for macrophage morphology and migrationProc Natl Acad Sci U S A200310019107401074512960403
- GuinamardROkigakiMSchlessingerJRavetchJVAbsence of marginal zone B cells in Pyk-2-deficient mice defines their role in the humoral responseNat Immunol200011313610881171
- KamenLASchlessingerJLowellCAPyk2 is required for neutrophil degranulation and host defense responses to bacterial infectionJ Immunol201118631656166521187437
- BuckbinderLCrawfordDTQiHProline-rich tyrosine kinase 2 regulates osteoprogenitor cells and bone formation, and offers an anabolic treatment approach for osteoporosisProc Natl Acad Sci U S A200710425106191062417537919
- Gil-HennHDestaingOSimsNADefective microtubule-dependent podosome organization in osteoclasts leads to increased bone density in Pyk2(−/−) miceJ Cell Biol200717861053106417846174
- KoppelACKissAHindesADelayed skin wound repair in proline-rich protein tyrosine kinase 2 knockout miceAm J Physiol Cell Physiol201430610C899C90924598361
- LambertJCIbrahim-VerbaasCAHaroldDMeta-analysis of 74,046 individuals identifies 11 new susceptibility loci for Alzheimer’s diseaseNat Genet201345121452145824162737
- DourlenPFernandez-GomezFJDupontCFunctional screening of Alzheimer risk loci identifies PTK2B as an in vivo modulator and early marker of Tau pathologyMol Psychiatry Epub2016426
- LipinskiCALoftusJCTargeting Pyk2 for therapeutic interventionExpert Opin Ther Targets20101419510820001213
- SelitrennikMLevSPYK2 integrates growth factor and cytokine receptors signaling and potentiates breast cancer invasion via a positive feedback loopOncotarget2015626222142222626084289
- LimYLimSTTomarAPyK2 and FAK connections to p190Rho guanine nucleotide exchange factor regulate RhoA activity, focal adhesion formation, and cell motilityJ Cell Biol2008180118720318195107
- OwenKAThomasKSBoutonAHThe differential expression of Yersinia pseudotuberculosis adhesins determines the requirement for FAK and/or Pyk2 during bacterial phagocytosis by macrophagesCell Microbiol20079359660916987330
- RayBJThomasKHuangCSGutknechtMFBotchweyEABoutonAHRegulation of osteoclast structure and function by FAK family kinasesJ Leukoc Biol20129251021102822941736
- WeisSMLimSTLutu-FugaKMCompensatory role for Pyk2 during angiogenesis in adult mice lacking endothelial cell FAKJ Cell Biol20081811435018391070
- HanSMistryAChangJSStructural characterization of proline-rich tyrosine kinase 2 (PYK2) reveals a unique (DFG-out) conformation and enables inhibitor designJ Biol Chem200928419131931320119244237
- WalkerDPZawistoskiMPMcGlynnMASulfoximine-substituted trifluoromethylpyrimidine analogs as inhibitors of proline-rich tyrosine kinase 2 (PYK2) show reduced hERG activityBioorg Med Chem Lett200919123253325819428251
- IrwinJJSterlingTMysingerMMBolstadESColemanRGZINC: a free tool to discover chemistry for biologyJ Chem Inf Model20125271757176822587354
- ForliSHueyRPiqueMESannerMFGoodsellDSOlsonAJComputational protein-ligand docking and virtual drug screening with the AutoDock suiteNat Protoc201611590591927077332
- JacobsonMPPincusDLRappCSA hierarchical approach to all-atom protein loop predictionProteins200455235136715048827
- SastryGMAdzhigireyMDayTAnnabhimojuRShermanWProtein and ligand preparation: parameters, protocols, and influence on virtual screening enrichmentsJ Comput Aided Mol Des201327322123423579614
- SondergaardCROlssonMHRostkowskiMJensenJHImproved treatment of ligands and coupling effects in empirical calculation and rationalization of pKa valuesJ Chem Theory Comput2011772284229526606496
- MorrisGMHueyRLindstromWAutoDock4 and AutoDock Tools4: automated docking with selective receptor flexibilityJ Comput Chem200930162785279119399780
- TrottOOlsonAJAutoDock Vina: improving the speed and accuracy of docking with a new scoring function, efficient optimization, and multithreadingJ Comput Chem201031245546119499576
- Abad-ZapateroCMetzJTLigand efficiency indices as guideposts for drug discoveryDrug Discov Today200510746446915809192
- HopkinsALKeseruGMLeesonPDReesDCReynoldsCHThe role of ligand efficiency metrics in drug discoveryNat Rev Drug Discov201413210512124481311
- KuoLCFragment-based drug design – tools, practical approaches, and examples. PrefaceMethods Enzymol2011493xxixxii21371584
- RitchieTJMacdonaldSJThe impact of aromatic ring count on compound developability – are too many aromatic rings a liability in drug design?Drug Discov Today20091421–221011102019729075
- WaringMJArrowsmithJLeachARAn analysis of the attrition of drug candidates from four major pharmaceutical companiesNat Rev Drug Discov201514747548626091267
- FarrellNKellandLRRobertsJDVan BeusichemMActivation of the trans geometry in platinum antitumor complexes: a survey of the cytotoxicity of trans complexes containing planar ligands in murine L1210 and human tumor panels and studies on their mechanism of actionCancer Res19925218506550721516063
- van BeusichemMFarrellNActivation of the trans geometry in platinum antitumor complexes. Synthesis, characterization, and biological activity of complexes with the planar ligands pyridine, N-methylimidazole, thiazole, and quinoline. Crystal and molecular structure of trans-dichlorobis(thiazole)platinum(II)Inorg Chem1992314634639
- FriesnerRAMurphyRBRepaskyMPExtra precision glide: docking and scoring incorporating a model of hydrophobic enclosure for protein-ligand complexesJ Med Chem200649216177619617034125
- SchrodingerSchrodinger Release 2015–4: LipPrep, Version 3.6New York, NYLLC2015
- TripathiSKMuttineniRSinghSKExtra precision docking, free energy calculation and molecular dynamics simulation studies of CDK2 inhibitorsJ Theor Biol201333487100
- SirinSKumarRMartinezCA computational approach to enzyme design: predicting omega-aminotransferase catalytic activity using docking and MM-GBSA scoringJ Chem Inf Model20145482334234625005922
- BanavathHNSharmaOPKumarMSBaskaranRIdentification of novel tyrosine kinase inhibitors for drug resistant T315I mutant BCR-ABL: a virtual screening and molecular dynamics simulations studySci Rep20144694825382104
- LynePDLambMLSaehJCAccurate prediction of the relative potencies of members of a series of kinase inhibitors using molecular docking and MM-GBSA scoringJ Med Chem200649164805480816884290
- XuLSunHLiYWangJHouTAssessing the performance of MM/PBSA and MM/GBSA methods. 3. The impact of force fields and ligand charge modelsJ Phys Chem B2013117288408842123789789
- GenhedenSKuhnOMikulskisPHoffmannDRydeUThe normal-mode entropy in the MM/GBSA method: effect of system truncation, buffer region, and dielectric constantJ Chem Inf Model20125282079208822817270
- BowersKJChowDEHuafengXScalable algorithms for molecular dynamics simulations on commodity clustersPaper presented at: ACM/IEEE SC 2006 Conference2006Tampa, FL
- JorgensenWChandrasekharJMaduraJImpeyRKleinMComparison of simple potential functions for simulating liquid waterJ Chem Phys198379926
- HedgerGSansomMSKoldsoHThe juxtamembrane regions of human receptor tyrosine kinases exhibit conserved interaction sites with anionic lipidsSci Rep20155919825779975
- LeeHSQiYImWEffects of N-glycosylation on protein conformation and dynamics: protein data bank analysis and molecular dynamics simulation studySci Rep20155892625748215
- MartynaGJTobiasDJKleinMLConstant pressure molecular dynamics algorithmsJ Chem Phys199410154177
- EssmannUPereraLBerkowitzMLDardenTLeeHPedersenLGA smooth particle mesh Ewald methodJ Chem Phys1995103198577
- LawrenzMShuklaDPandeVSCloud computing approaches for prediction of ligand binding poses and pathwaysSci Rep20155791825608737
- HuangNShoichetBKIrwinJJBenchmarking sets for molecular dockingJ Med Chem200649236789680117154509
- MysingerMMCarchiaMIrwinJJShoichetBKDirectory of useful decoys, enhanced (DUD-E): better ligands and decoys for better benchmarkingJ Med Chem201255146582659422716043
- NicolaouCABrownNPattichisCSMolecular optimization using computational multiobjective methodsCurr Opin Drug Discov Devel2007103316324
- Abad-ZapateroCLigand efficiency indices for effective drug discoveryExpert Opin Drug Discov20072446948823484756
- BertholdMRCebronNDillFKNIME: The Konstanz Information MinerBerlin, HeidelbergSpringer2008
- BentoAPGaultonAHerseyAThe ChEMBL bioactivity database: an updateNucleic Acids Res201442Database issueD1083D109024214965
- RichardsonLRubySRestful Web ServicesFirst edSebastopolO’Reilly Media2007
- GenhedenSRydeUThe MM/PBSA and MM/GBSA methods to estimate ligand-binding affinitiesExpert Opin Drug Discov201510544946125835573
- GreenidgePAKramerCMozziconacciJCWolfRMMM/GBSA binding energy prediction on the PDBbind data set: successes, failures, and directions for further improvementJ Chem Inf Model201353120120923268595
- NowakowskiJCroninCNMcReeDEStructures of the cancer-related Aurora-A, FAK, and EphA2 protein kinases from nanovolume crystallographyStructure200210121659166712467573
- GhoshSNieAAnJHuangZStructure-based virtual screening of chemical libraries for drug discoveryCurr Opin Chem Biol200610319420216675286
- HannMMMolecular obesity, potency and other addictions in drug discoveryMed Chem Commun201125349355
- TotrovMAbagyanRFlexible ligand docking to multiple receptor conformations: a practical alternativeCurr Opin Struct Biol200818217818418302984
- CarlsonHAMcCammonJAAccommodating protein flexibility in computational drug designMol Pharmacol200057221321810648630
- MeanwellNAImproving drug design: an update on recent applications of efficiency metrics, strategies for replacing problematic elements, and compounds in nontraditional drug spaceChem Res Toxicol201629456461626974882
- Cortes-CirianoIBenchmarking the predictive power of ligand efficiency indices in QSARJ Chem Inf Model20165681576158727399907
- LiJBaiFLiuHGramaticaPLigand efficiency outperforms pIC50 on both 2D MLR and 3D CoMFA models: a case study on AR antagonistsChem Biol Drug Des20158661501151726198098
- HawkinsPCWarrenGLSkillmanAGNichollsAHow to do an evaluation: pitfalls and trapsJ Comput Aided Mol Des2008223–417919018217218
- MichelJEssexJWPrediction of protein-ligand binding affinity by free energy simulations: assumptions, pitfalls and expectationsJ Comput Aided Mol Des201024863965820509041
- PalmerBDSmaillJBRewcastleGWStructure-activity relationships for 2anilino-6-phenylpyrido[2,3-d]pyrimidin-7(8H)-ones as inhibitors of the cellular checkpoint kinase Wee1Bioorg Med Chem Lett20051571931193515780636
- McCluskeyAKeaneMAWalkomCCThe first two cantharidin analogues displaying PP1 selectivityBioorg Med Chem Lett200212339139311814804
- KolbPPhanKGaoZGMarkoACSaliAJacobsonKALimits of ligand selectivity from docking to models: in silico screening for A(1) adenosine receptor antagonistsPLoS One2012711e4991023185482