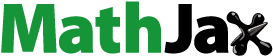
Abstract
Introduction
Computational molecular database screening helps to decrease the time and resources needed for drug development. Reintroduction of generic drugs by second medical use patents also contributes to cheaper and faster drug development processes. We screened, in silico, the Food and Drug Administration-approved generic drug database by means of the One-dimensional Drug Profile Matching (oDPM) method in order to find potential peroxisome proliferator-activated receptor gamma (PPARγ) agonists. The PPARγ action of the selected generics was also investigated by in vitro and in vivo experiments.
Materials and methods
The in silico oDPM method was used to determine the binding potency of 1,255 generics to 149 proteins collected. In vitro PPARγ activation was determined by measuring fatty acid-binding protein 4/adipocyte protein gene expression in a Mono Mac 6 cell line. The in vivo insulin sensitizing effect of the selected compound (nitazoxanide; 50–200 mg/kg/day over 8 days; n = 8) was established in type 2 diabetic rats by hyperinsulinemic euglycemic glucose clamping.
Results
After examining the closest neighbors of each of the reference set’s members and counting their most abundant neighbors, ten generic drugs were selected with oDPM. Among them, four enhanced fatty acid-binding protein/adipocyte protein gene expression in the Mono Mac 6 cell line, but only bromfenac and nitazoxanide showed dose-dependent actions. Induction by nitazoxanide was higher than by bromfenac. Nitazoxanide lowered fasting blood glucose levels and improved insulin sensitivity in type 2 diabetic rats.
Conclusion
We demonstrated that the oDPM method can predict previously unknown therapeutic effects of generic drugs. Nitazoxanide can be the prototype chemical structure of the new generation of insulin sensitizers.
Introduction
Drug development is a highly time-consuming and costly process that usually lasts for approximately 10–12 years and requires about 800–1,000 million US dollars.Citation1 This huge amount of resources can be explained by the high attrition rate of drug candidates during the whole drug development process. In general, it can be estimated that only one out of every 50–100 candidates that enter into the preclinical research phase will finally reach the market; the remaining candidates will be abandoned during the pre-clinical or clinical phase of the development process. This is why both society and the pharmaceutical industry have the drive to find methods to develop cheaper and more effective drugs in the near future. Theoretically, there are several possibilities to shorten the amount of time or decrease the total costs required for drug development. Every activity that can speed up the development process and reduce the time-to-market can bear significant advances. One possible, and perhaps the simplest, way to decrease the amount of resources needed for drug development is by repositioning generic drugs in new therapeutic indications, resulting in the so-called “second medical use” patents.
The reason for the high attrition rate among drug candidates originated from either their inefficacy at treating human pathological conditions or from its safety concerns, since the beneficial and adverse effects can occur over the long-lasting administration of drugs. In addition, drug candidates are unpredictable based on the in vitro and in vivo test systems used to characterize the pharmacokinetic and pharmacodynamic properties of a drug candidate. During the last decade, the cost of drug development significantly increased in parallel with increasingly more rigorous regulations. This resulted in the intention to develop drug candidates that – beside their improved efficacy – are proven to be safe. This led to the quest of establishing new methodologies to bring significant improvements in the predictability of the therapeutic and adverse effect profile of drug candidates. One possible approach is to use molecular docking methods to study the interaction between the drugs and their molecular targets.
Another, less widespread approach is the application of the docking results as virtual affinity fingerprints and using them as an interaction pattern (IP) that describes the general interaction properties of a molecule. Here, the overall information contained in the pattern is used instead of the data that can be concluded from the individual interactions. Such a method is Drug Profile Matching (DPM), which aims to relate the medical effect profiles and target profiles of approximately 1,200 Food and Drug Administration (FDA)-approved small-molecule drugs with their virtual affinity fingerprints (ie, a series of docking scores of the molecule against the ligand binding sites of a predefined set of 149 proteins).Citation2 The starting hypothesis behind DPM is that similar affinity fingerprints refer to similar bioactivity properties (ie, targets and medical effects). We found that there is a strong relationship between the 177 medical effects studied and 77 target categories with the virtual affinity fingerprints of the drugs.Citation3 Receiver operating characteristic analysis and cross-validations proved that the method is robust, and the affinity fingerprints provide enough information to recreate the effect profiles of drugs. In a following study, we studied the contribution of the structural features of the drugs to the high prediction power of the method. We showed that DPM outperformed conventional two-dimensional and three-dimensional structural similarity-based prediction approaches in almost all examined categories.Citation4 Nevertheless, its prediction accuracy is limited if only a few compounds can be used as a learning set for a given effect. Apart from the already known effects, previously unrevealed bioactivities (medical effects, targets, and so on) can also be predicted for the existing drugs by this method. These predictions can be validated by in vitro/in vivo tests or by retrospective literature analyses.
Although there are growing numbers of type 2 diabetic patients, the available remedy for this disease is confined to a limited number of drug classes. The most frequently prescribed medication for the treatment of type 2 diabetes is the group of thiazolidinediones.Citation5 This class of drugs acts through the nuclear receptor peroxisome proliferator-activated receptor gamma (PPARγ), and its activation can be exploited to improve insulin sensitivity in insulin-resistant animals or humans.Citation6 In spite of the fact that this class of drugs reached a blockbuster status shortly after it was introduced into the market, there are currently safety concerns regarding all three representatives of thiazolidinediones. The first marketed thiazolidinedione, troglitazone (Rezulin®; Warner-Lambert, Morris Plains, NJ, USA) was withdrawn from the market due to its hepatotoxicity.Citation7 Rosiglitazone (Avandia®; GlaxoSmithKline, London, UK) was withdrawn from the European market while it was put under selling restrictions in the USA due to its high incidence of cardiovascular events observed in connection with its administration.Citation8 Finally, the sale of pioglitazone (Actos®; Takeda Pharmaceutical Company Limited, Osaka, Japan) was suspended in the German and France markets due to its potential effect to induce bladder cancer.Citation9 On the other hand, these side effects do not seem to be class effects, which can encourage academic and industrial researchers to find a drug candidate with PPARγ receptor agonist activity that does not result in the abovementioned or other side effects.
The aim of the present study was to investigate whether our in silico prediction method could be used for screening generic drugs in order to find new therapeutic indications. To test our methodological approach, the FDA database containing 1,255 generic drugs was screened to predict their PPARγ activation propensities. Then, the in silico selected drugs were tested in vitro using PPARγ receptor-expressing cell cultures to determine whether they are able to activate the PPARγ receptor. Finally, a series of in vivo experiments were carried out by means of hyperinsulinemic euglycemic glucose clamping (HEGC) in a rat model of type 2 diabetes in order to study the PPARγ receptor-related insulin sensitizing effect of a drug showing the most promising in vitro effect.
Materials and methods
Ethical aspects
The study protocol was formally approved by the University of Debrecen Animal Ethics Committee (Debrecen, Hungary). The experiments presented conform to European Community guiding principles for the care and use of laboratory animals. The experimental protocols that were applied had been approved by the local ethical boards of the University of Debrecen, Debrecen, Hungary (08/2007 DEMÁB and 16/2007 DEMÁB).
One-dimensional DPM
The selection of potential PPARγ antagonist candidates was performed using a simpler version of the recently introduced DPM – a systematic, pattern-based, bioactivity prediction method.Citation2 This method uses virtual affinity fingerprints of small molecule compounds to predict their medical effects and targets.Citation2,Citation3 In DPM, the affinity fingerprint is called IP, which consists of a set of docking scores of a compound against the binding sites of a predefined nontarget protein set. The main difference between DPM and One-dimensional DPM (oDPM) presented here () is that the latter method applies a one-dimensional distance-like metric to compare the IPs that were considered as vectors in a multidimensional space, while DPM generates hyperplanes to separate active and inactive compounds in the multidimensional space. The main reason for applying oDPM instead of DPM includes the limited number of compounds in the reference set. In our earlier work, we found that at least ten members are required for a group to reliably perform multivariate statistical methods of DPM;Citation2 with under ten members, an alternative evaluation method is required, like oDPM. Since the detailed mechanism of DPM has been introduced earlier,Citation3 we will only summarize its most important features and point to the differences between oDPM and DPM.
Figure 1 Summary of the oDPM.
Abbreviations: DS, docking score; IP, interaction pattern; oDPM, One-dimensional Drug Profile Matching.
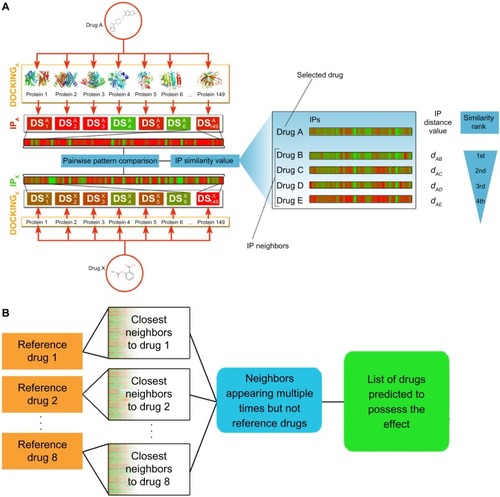
Data collection
A total of 1,255 FDA-approved drug molecules were extracted from the DrugBank database.Citation10 In addition, 149 proteins were collected from the Research Collaboratory for Structural Bioinformatics Protein Data Bank,Citation11 as described in our previous work.Citation2
Docking preparations and calculations
Docking preparations and calculations were performed by AutoDockToolsCitation12 (Molecular Graphics Laboratory, The Scripps Research Institute, La Jolla, CA, USA) and DOVIS 2.0 (DOcking-based VIrtual Screening, Biotechnology High Performance Computing Software Applications Institute, Department of Defense, Frederick, MD, USA) software,Citation13 using the AutoDock4 (Molecular Graphics Laboratory, The Scripps Research Institute) docking engine with its native scoring function.Citation14,Citation15 Preparation procedures were the same as presented earlier.Citation2 Each drug molecule was docked to each protein, and binding free energies were extracted and the minima were imported into a database. Docking runs were performed on a Hewlett-Packard cluster of 104 central processing units (Hewlett-Packard Company, Palo Alto, CA, USA). In sum, 1,255 × 149 = 186,995 dockings were performed repeated 200 times for each drug-protein pair, and were docked and scored by AutoDock4 (Molecular Graphics Laboratory, The Scripps Research Institute).
Reference set
Instead of using a medical effect database, a single-target focused reference set of eight molecules was applied. These known PPARy antagonists were rosiglitazone, bexarotene, gemfbrozil, pioglitazone, alpha-linolenic acid fenofibrate, bezafibrate, and clofibrate.
IP similarity calculations
A similarity coefficient (d) based on the angle enclosed by two IP vectors was used to create the IP similarity matrix. The IP vectors are determined by the docking scores as coordinates in a 149-dimensional space created by the 149 members of the protein set. Cosine angle distance coefficientCitation16 was used to determine the angle between two vectors in the above-described 149-dimensional space as follows:
where dAB is the IP distance value between molecules A and B, and ai and bi are the docking score values of molecules A and B on the i-th protein of n = 149, respectively.
Neighbor-based bioactivity prediction
Based on the IP similarity matrix, similarity lists were generated to each member of the reference set. Applying the cut-off value of d = 3, the number of appearances of the drugs on each list was calculated, and the most abundant compounds were selected for further analysis (). The maximum number of appearances was three.
In vitro study
The PPARγ-activating actions of the in silico-selected generics were tested on a Mono Mac 6 (MM6) cell line. The MM6 is a monoblastic leukemic cell line, which is known to express PPARγ. The activation of PPARγ results in the expression of a wide range of genes; among them, some can be activated solely by the PPARγ. One of them is the fatty acid-binding protein 4/adipocyte protein (FABP4/aP2) gene,Citation17 which was studied.
The study was carried out on 48-well plate, with 200,000 cells per well. Every treatment was done in triplicate. The cells were pretreated with phorbol 12-myristate 13-acetate, which makes the cells similar to macrophages and sensitizes their PPARγ response. After 24 hours, the phorbol 12-myristate 13-acetate was washed out, fresh nutrient solution was added and then the treatment was commenced by increasing the doses (10−8 M to 10−5 M) of the selected drugs in order to obtain their concentration-response curves. Rosiglitazone (10−8 M to 10−5 M) was used as a positive control, and the vehicle (dimethyl sulfoxide, ethanol, or water) as a negative control. After 24 hours of incubation, the cells were lysated in TRIzol® reagent (Life Technologies, Carlsbad CA, USA), and the ribonucleic acid (RNA) was isolated. Then, the samples of RNA were converted into complementary deoxyribonucleic acid by means of reverse transcription polymerase chain reaction, and the PPARγ activity was determined by measuring the expression of the FABP4/aP2 gene’s messenger RNA (mRNA) by real-time reverse transcription polymerase chain reaction. Beside the FABP4/aP2 gene, the mRNA expression of the cyclophilin A housekeeping gene was also determined in the samples. Then, the data obtained from the FABP4/aP2 gene expression were normalized to the cyclophilin A values by means of the ∆∆Ct method and these normalized values were evaluated.
Animals and study design
The experiments were carried out on 5-week-old male Wistar rats. The animals were housed in an animal room with a temperature of 22°C–24°C, and with 50%–70% relative humidity. The lighting was set to 12-hour light and 12-hour dark periods.
Forty-eight animals were used throughout the study, and they were divided into three main groups. The first main group of Wistar rats served as healthy, vehicle-treated controls. These animals were held on a standard laboratory diet (ssniff®, EF R/M Control; ssniff Spezialdiäten GmbH, Soest, Germany), and they drank tap water ad libitum. The second main group of the rats was fed with a high-fat diet (HFD, ssniff®, EF R/M with 20% fat; ssniff Spezialdiäten GmbH, Soest, Germany) for 3 weeks. These rats served as the insulin-resistant, nondiabetic, vehicle treated group. In the first two main groups of rats, eight rats per group were used. The third main group of rats was divided into four subgroups with eight rats per subgroup. These rats were fed HFD for 2 weeks, and they were then treated with streptozotocin (STZ; 50 mg/kg, intraperitoneally). After an additional week, the animals showed stable fasting hyperglycemia. This animal model mimics the two characteristic features of type 2 diabetes. The peripheral insulin resistance was induced by the HFD, while impaired glucose-stimulated insulin secretion was induced by the STZ treatment. This type 2 diabetic animal model was originally established and validated by Reed et al.Citation18 The first subgroup of the third main group was treated with vehicle, and the remaining three subgroups were treated with a daily oral dose of 50 mg/kg, 100 mg/kg, and 200 mg/kg of nitazoxanide, respectively. All treatments lasted for 8 days, and the on the final day, 2 hours after the last vehicle/drug administration, the animals were anesthetized in order to execute the HEGC study for determination of whole-body insulin sensitivity.
Dose selection
As our ultimate goal was to find a generic drug that could be reintroduced in new clinical indications, the important aspect of our drug development paradigm was that the drug could improve insulin sensitivity in similar or lower doses than previously used in animal studies, where the drug demonstrated its efficacy in the originally approved indication (such as nitazoxanide as an antiprotozoal agent). Accordingly, we selected doses of nitazoxanide where the dose range (50–200 mg/kg/day) demonstrated efficacy in reducing oocyst shedding in an immunosuppressed rat model of cryptosporidiosis.Citation19
Determination of insulin sensitivity
The HEGC procedure was performed as described previously.Citation20 In brief, after an overnight fasting, rats were anesthetized with an intraperitoneal injection of 50 mg/kg of sodium thiopental (Thiopental Sandoz®; Sandoz Pharmaceutical PLC, Basel, Switzerland). After a middle sagittal surgical incision on the ventral surface of the neck, the trachea was exposed and a polyethylene tube was introduced into it to allow spontaneous breathing of the animals. Then, the left jugular vein and the left carotid artery were exposed and cleaned from the adhering connective tissues. Insulin and glucose were infused as separate lines of infusion through the two branches of the left jugular vein, while the arterial cannula served for blood pressure monitoring as well as to obtain blood samples (0.1 mL) for subsequent blood glucose and plasma insulin determinations. When the surgery was completed, there was a 30-minute stabilization period; then, a continuous insulin infusion (Humulin R®; Eli Lilly and Company, Indianapolis, IN, USA) at a rate of 3 mU/kg/minute was commenced along with glucose infusion (20% w/v). The rate of glucose infusion was adjusted in order to maintain euglycemia (5.5 ± 0.5 mmol/L). The blood glucose concentration was determined by means of a glucometer (Accu-Chek®; F Hoffmann La-Roche Ltd, Basel, Switzerland) before and at 5-minute intervals during the first 80 minutes, and at 10-minute intervals during the last 40 minutes of the HEGC experiment. In order to determine the fasting and steady-state plasma levels of insulin, additional blood samples were collected (0.5 mL, in 20 μL of ethylenediaminetetraacetic acid and 10 μL of Trasylol®; Bayer AG, Leverkusen, Germany) from the carotid artery immediately before the commencement of insulin infusion and during the steady state of the HEGC, respectively. The blood samples were centrifuged (Centrifuge 5415R; Eppendorf AG, Hamburg, Germany) for 2 minutes at 4°C and 10,000g; then, the plasma was aliquoted, frozen, and stored at −70°C for subsequent determinations. The plasma insulin level was determined by means of a commercially available radioimmunoassay kit (RK-400CT, Institute of Isotopes of the Hungarian Academy of Sciences, Budapest, Hungary). The average glucose infusion rate (GIR; mg/kg/minute) required to maintain the euglycemia during the last 30 minutes of the HEGC characterizes the glucose uptake of the peripheral insulin-sensitive tissues.Citation21
Derivative measures regarding the characterization of the insulin action can be calculated by means of plasma insulin and blood glucose values obtained during the fasting state as well during as the steady state of the HEGC. Accordingly, the insulin sensitivity of the peripheral tissues was characterized by the insulin sensitivity index (ISI), a measure of the amount of glucose taken up by peripheral tissues per unit of plasma insulin concentration.Citation22 Furthermore, the metabolic clearance rate of insulin (MCRI) as an indicator of the rate of disappearance of insulin from the blood was calculated as the insulin infusion rate (mU/kg/minute), divided by the difference between the steady-state and fasting-plasma insulin level, and was expressed as mL/kg/minute.Citation22
Statistical analysis
All data were expressed as mean ± standard deviation (SD) and were analyzed with paired t-tests when possible; otherwise, one-way analysis of variance followed by a modified repeated measures t-test (according to Bonferroni’s method) was used.Citation23
Results
One-dimensional DPM
The basis of the oDPM analysis is that the pairwise similarities between two IPs were considered as vectors in a multidimensional space (). This approach is referred to as “one-dimensional” since the distance of the two IP vectors is a one-dimensional measure. The advantage of this measure is that it reflects the pattern of the docking score values in the profile more than the actual scores. For instance, if two drugs possess the same IP, but with different average docking scores, their d value will be small, suggesting a high level of similarity. On the other hand, a single miscalculated docking score can cause significant error in the distance measurement. This disadvantage is eliminated in DPM.Citation2 It should be mentioned that in the case of small groups (less than ten members), DPM cannot be applied because of the high probability of overfitting.Citation2 Therefore, PPARγ predictions can be obtained only by a simpler method like oDPM, regardless of its generally weaker prediction accuracy when compared to DPM. The average area under the curve value of oDPM on 157 effect groups with at least ten members is 0.62 ± 0.12, while the accuracy of DPM is 0.97 ± 0.03.Citation3
After examining the closest neighbors of the members of the reference set, and after counting the most abundant neighbors of the members, ten generic drugs were selected if they appeared on the list three times: dantrolene, entacapone, ethacrynic acid, ketorolac, tiaprofenic acid, tolmetin, bromfenac, lubiprostone, nitazoxanide, and suprofen (). A further 44 compounds appeared on the list twice, while 116 drugs reached one appearance; all of them were discarded. The IP distance value matrix of these ten compounds is displayed in . It is notable that the compounds are close to each other and seem to form a fairly coherent group that is similar to the reference set (average IP distance values and standard errors for the reference set and the predicted set are 1.37 ± 0.85 and 1.30 ± 0.77, respectively). Lubiprostone is the only compound that is distant from the others. Based on these results, no further refinement of the prediction could be performed; therefore, these ten compounds were piped to in vitro screening.
Table 1 Generic drugs showing PPARγ receptor ligand affinity after the One-dimensional Drug Profile Matching screening
Table 2 Interaction pattern distance values of the predicted ten generic drugs
In vitro study, the effect on FABP4/aP2 gene activity
Six of the ten chemical structures studied () did not alter FABP4/aP2 gene expression in the MM6 cell line (data not shown). On the other hand, bromfenac, nitazoxanide, suprofen, and lubiprostone induced significant elevation in the FABP4/aP2 gene transcript (–). However, the induced elevation in the mRNA expression was distinct among the MM6 cell lines treated with these four chemicals. Suprofen induced a significant elevation in the mRNA expression level, though at its lowest concentration applied (10−8 M); however, increasing doses were not followed by further elevation in mRNA expression, except at its highest dose (10−5 M), but this change was not significant compared to its lower doses. Lubiprostone also induced significant elevation in the mRNA level at its lowest dose (10−8 M) applied; however, the effect induced by increasing its doses was inconsistent, and no dose-dependent elevation in mRNA expression was seen. The other two drugs, bromfenac and nitazoxanide, induced dose-dependent activation of the FABP4/aP2 gene. Bromfenac caused significant elevations in mRNA expression in the two higher doses (10−6 M and 10−5 M), while the nitazoxanide was able to induce significant elevation in 10−7 M concentration, and its effect seemed to be dose-dependent in that its effect on FABP4/aP2 gene activity was nonsignificant in the 10−6 M concentration. The greater SD can explain the observed nonsignificant alteration.
Figure 2 The effect of (A) bromfenac, (B) nitazoxanide, (C) suprofen, and (D) lubiprostone (10−8 M to 10−5 M) on FABP4/aP2 gene expression in MM6 cell lines. Rosiglitazone (10−8 M to 10−6 M) was used as a positive control. *Indicates significant changes from the vehicle-treated (DDDT or ethanol) control.
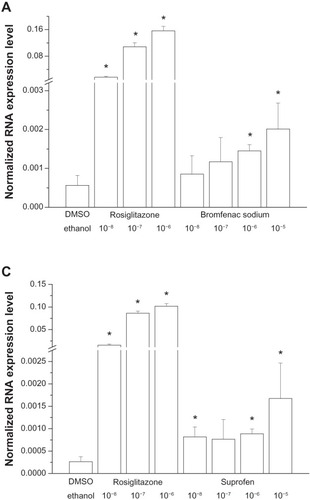
The percentile changes in FABP4/aP2 gene expression were also determined by comparing the effects of the highest applied dose of these four drugs to the effects elicited by their vehicle. In this comparison, lubiprostone proved to be the most effective, showing an approximate 10 times elevation in the normalized mRNA expression level, while nitazoxanide, suprofen and bromfenac showed 7 times, 6 times and 3 times elevation, respectively ().
Figure 3 Relative fold change in the mRNA expression in response to the highest dose of various drugs.
Abbreviation: mRNA, messenger ribonucleic acid.
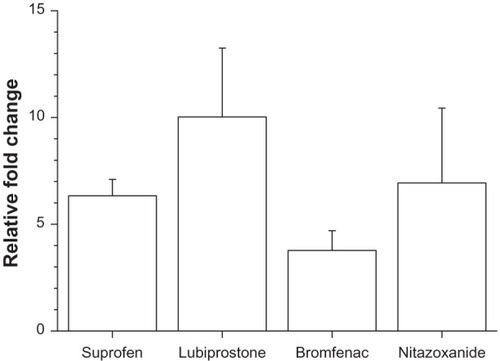
Rosiglitazone as a reference drug induced a concentration-dependent enhancement in FABP4/aP2 gene expression in the MM6 cell line. Moreover, the stimulating effect of rosiglitazone on FABP4/aP2 gene expression was approximately one order of magnitude higher than that observed after administration of either of the other drugs (data not shown). On the other hand, the vehicles failed to evoke any changes in FABP4/aP2 gene expression in the MM6 cell line (data not shown).
In vivo study, determination of whole-body insulin sensitivity
Based on the in vitro data and the available information regarding these generic drugs, we selected nitazoxanide for further study in order to determine its insulin sensitizing effect on type 2 diabetic rats.
The effect of different treatment schedules on the glycemic control of different groups of rats has been summarized in and . Animals held on a HFD became insulin resistant, but not diabetic at the end of the 3-week diet period. Rats treated with intravenous STZ at the end of the second week of the HFD regimen showed not only decreased insulin sensitivity compared to healthy animals, but also marked fasting hyperglycemia (ie, these rats became type 2 diabetic). The vehicle-treated healthy and HFD groups of rats showed significantly lower fasting blood glucose levels and significantly elevated GIR and ISI compared to the vehicle-treated HFD + STZ rats. The nitazoxanide treatment induced a dose-dependent improvement in the glycemic status of the HFD + STZ-treated type 2 diabetic rats. This improvement in glycemic status was characterized by a significant increase in the GIR needed to maintain euglycemia, as well as by the enhancement of the ISI during the HEGC in rats treated with 200 mg/kg nitazoxanide over 8 days. The fasting blood glucose level of the vehicle-treated HFD + STZ rats was significantly elevated compared to the vehicle-treated healthy or HFD rats, and nitazoxanide treatment showed a dose-dependent reduction in the fasting blood glucose level, reaching a statistically significant level at a dose of 200 mg/kg. The fasting plasma insulin level was elevated in all type 2 diabetic groups (HFD + STZ + vehicle, HFD + STZ + nitazoxanide) and the nitazoxanide treatment did not cause a significant change. Finally, no significant difference among the MCRI could be observed in either treatment groups. In addition, no alteration in the resting mean arterial blood pressure was observed ( and ).
Table 3 The effect of 8-day nitazoxanide treatment (50 mg/kg/day, 100 mg/kg/day, and 200 mg/kg/day) on the fasting blood glucose and plasma insulin level, on the resting blood pressure, as well as on body weight
Figure 4 The effect of 8-day NTX treatment (50 mg/kg/day, 100 mg/kg/day, and 200 mg/kg/day per os twice a day) on the GIR, ISI, and on the MCRI. *Indicates statistically significant changes from the HFD + STZ and vehicle-treated groups.
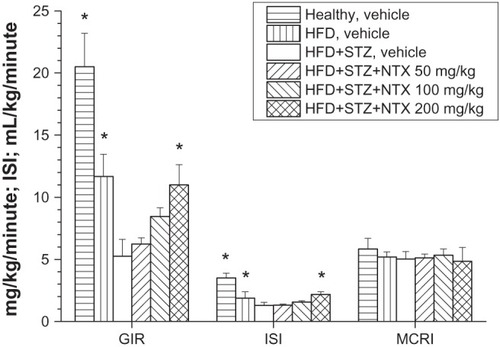
Discussion
The aim of the present study was to find generic drug(s) with PPARγ receptor agonist activity by means of screening the FDA-approved generic molecule database using oDPM methods. As a result of our current work, we have identified ten potential PPARγ ligands by means of the oDPM method. It had been shown that four out of the selected ten drugs were able to induce the expression of the transcript of the FABP4/aP2 gene, which is specific to PPARγ receptor activation. Among these four generics, nitazoxanide was chosen to further study its putative PPARγ receptor agonist action by means of HEGC in a rodent model of type 2 diabetes in order to explore its insulin sensitizing property.Citation18,Citation21
The drug development process became very expensive during the last decades. There have been several attempts made, trying to shorten the time-to-market as well as to decrease the amount of human and financial resources needed for the drug development process. In order to solve these above mentioned difficulties, the interest of the pharmaceutical industry has turned to the renewal of generics. Using drugs at the same or lower concentrations, as well as for similar treatment periods as were used in their original indications, several steps in the development process can be spared, such as conducting time-consuming and costly toxicity studies; an example of this kind of drug renewal was published previously.Citation24 We demonstrated that cicletanine, a phosphodiesterase enzyme 1–5 inhibitor (originally developed for the treatment of hypertension), is able to enhance whole-body insulin sensitivity in insulin-resistant rabbits at lower doses than is required for its vascular effects.Citation24
The actuality of our present study has been underpinned by the fact that the prevalence of diabetes is increasing globally, and the total number of patients suffering from this chronically devastating condition have reached 347 million.Citation25 On the other hand, the number of available drugs used to treat diabetes is limited, and the most frequently prescribed medications are thiazolidinediones, which have several side effects such as fluid retention, edema, congestive heart failure, or bone fractures.Citation26–Citation28 Although these side effects are due to the activation of the PPARγ receptor, conformation of the receptor is the key feature that determines the affinity of corepressors and coactivators to the ligand-bound PPARγ receptor, thereby determining the gene transcription profile and biological response after PPARγ activation.Citation29,Citation30 The diverse regulation of the PPARγ receptor activation and signal transduction pathways was made feasible to design selective PPARγ modulator molecules, which retain the insulin-sensitizing effect, but do not possess those unwanted effects mentioned above.Citation30
In the present study, we used computational modeling of the receptor–ligand interaction, which ensures high throughput screening of large molecule libraries. By screening the FDA-approved generics molecule database, our results supported the original assumption that the in silico oDPM methodology applied could facilitate the initial drug candidate selection if the molecular structure of the target protein is known. Here we combined the advantages of the in silico oDPM method and generics-based drug development strategy. The in silico oDPM method used allows us to select candidates from the molecule library within a relatively short time period. Using generics in new therapeutic indication could shorten the development process. On the other hand, the in silico prediction method has some limitations. For example, it does not allow us to establish whether the established receptor–ligand interaction corresponds to agonist or antagonist properties on the targeted receptor. In order to answer this important question, both in vitro and in vivo experiments were carried out.
oDPM was incapable of finding any differences between the four compounds that were active in vivo, and the rest of the predicted set, which again underlines the importance of in vitro testing and reminds us of the inherent limitations of in silico approaches. Our oDPM method is also currently incapable of predicting the side effects of a compound. Side effect prediction is more difficult than the presented prediction process, since the side effect databases are often incomplete and a lot of questions need to be answered (ie, the ambiguous terminology of the adverse events, the handling of different frequencies of adverse events depending on the exact treatment and dosage, and so on). Of course, the list of the effects and targets of the close IP neighbors of a molecule might still yield clues to its potential side effects (for example, a predicted angiotensin-converting enzyme inhibitory effect can point to possible effects on blood pressure). This opportunity will be addressed in future research, using the DPM method because it performs better than oDPM when studying large groups.
The data from in vitro experiments revealed those generics from our selection list that are able to stimulate the PPARγ receptor in a dose-dependent manner. Finally, in vivo experiments were carried out in order to study whether PPARγ activation results in the enhancement of insulin sensitivity among insulin-resistant, type 2 diabetic rats. Whole-body insulin sensitivity was determined by means of HEGC, the gold standard for determining insulin sensitivity in humans.Citation21 Since the full insulin-sensitizing effect of PPARγ receptor activation requires several days, we treated the animals for 8 days, which has been shown to be sufficient in inducing insulin-sensitizing effects in rodents.Citation31,Citation32
According to our original concept, we intended to investigate the putative insulin sensitizing action of the selected generics using the same dose-range as was used in its original indication. In the present study, we investigated the insulin-sensitizing effect of nitazoxanide, a generic drug that was originally approved as an antiprotozoal agent. Its established mechanism of action is to inhibit the pyruvate ferredoxin oxidoreductase enzyme-dependent electron transfer reaction.Citation33 Beside on its antiprotozoal action, the experimental results suggested that nitazoxanide can also bear antiviral activity due to its blocking properties over the maturation of the viral hemagglutinin, which in turn results in impaired intracellular trafficking and insertion into the host plasma membrane of the hemagglutinin.Citation34 On the other hand no data regarding its putative PPARγ receptor-activating action are available in the literature or in the patent databases. In an immunosuppressed rat model of cryptosporidiosis, nitazoxanide was able to effectively inhibit the oocyte shedding at a daily oral dose of 50–200 mg/kg.Citation19 So, we selected this same dose range and the applied treatment lasted for 8 days, according to the time required for evolution of the effect of PPARγ activation. Although in clinical practice nitazoxanide is usually administered for only 3–5 days to treat protozoal infections, the available preclinical toxicological data suggest that there are no safety concerns when nitazoxanide is used for a longer term.Citation35 These features made nitazoxanide an ideal generic drug candidate to be reintroduced in a new clinical indication as an insulin-sensitizing agent.
The main discovery of the present study is the demonstration of the predictive value of the oDPM method in searching for PPARγ ligands. The positive predictive value of the oDPM method was supported both by in vitro measurement of FABP4/aP2 gene activity after dose-dependent exposure of the MM6 cell line to nitazoxanide, and by in vivo demonstration of the dose-dependent insulin-sensitizing effect of nitazoxanide using an insulin-resistant type 2 diabetic rat model. Although we did not determine PPARγ mRNA or protein expression from the tissue samples after the in vivo experiments, our results did suggest that the improvement in insulin sensitivity could be a consequence of the enhancement in the glucose uptake of the peripheral insulin-sensitive tissues, since both the GIR and the ISI were increased in response to nitazoxanide treatment. On the other hand, the MCRI and the steady-state plasma insulin level were left unaffected by the nitazoxanide treatment. These data indicate that the putative mechanism of action of nitazoxanide is in the enhancement of the insulin sensitivity, which is similar to what was expected after PPARγ stimulation. Moreover, our results demonstrated that nitazoxanide failed to induce body weight gain and elevation in mean arterial blood pressure. It should be noted that the treatment period was only 8 days, and thus changes in either weight gain or systemic blood pressure cannot be ruled out, as they may occur after longer treatment periods. A more comprehensive study investigating the effects of the chronic administration of nitazoxanide on different organ systems will be able to address this issue.
Conclusion
Taken together, our results demonstrate the usefulness of the oDPM method in the screening of a molecule database for lead selection, and we discovered and supported the insulin-sensitizing effect of nitazoxanide, a generic antiprotozoal drug, in both in vivo and in vitro experiments. Using this technology, pharmaceutical companies can speed up the otherwise costly and time-consuming drug development process.
Acknowledgments
This study was supported by the Hungarian National Office for Research and Technology (NKFP_07-A2-2008-0260, GOP-1.1.2-07/1-2008-0004, TÁMOP-4.2.2.-08/1-2008-0014, OM-00174/2008, GOP-1.1.1-07/1-2008-0032, GOP-1.1.1-07/1-2008-0021, GOP-1.2.1-08-2009-0023, KDOP-1.2.1-11-2011-0003, and GOP-1.1.1-11-2012-0435) and the Hungarian Scientific Research Fund (OTKA-75965).
Disclosure
The authors report no conflicts of interest in this work.
References
- Paul SM Mytelka DS Dunwiddie CT How to improve R&D productivity: the pharmaceutical industry’s grand challenge Nat Rev Drug Discov 2010 9 3 203 214 20168317
- Simon Z Peragovics A Vigh-Smeller M Drug effect prediction by polypharmacology-based interaction profiling J Chem Inf Model 2012 52 1 134 145 22098080
- Peragovics Á Simon Z Tombor L Virtual affinity fingerprints for target fishing: a new application of Drug Profile Matching J Chem Inf Model 2013 53 1 103 113 23215025
- Peragovics A Simon Z Brandhuber I Contribution of 2D and 3D structural features of drug molecules in the prediction of drug profile matching J Chem Inf Model Epub 6 29 2012
- Patel CB De Lemos JA Wyne KL McGuire DK Thiazolidinediones and risk for atherosclerosis: pleiotropic effects of PPar gamma agonism Diab Vasc Dis Res 9 2006 3 2 65 71 17058625
- Saltiel AR Olefsky JM Thiazolidinediones in the treatment of insulin resistance and type II diabetes Diabetes 1996 45 12 1661 1669 8922349
- Kohlroser J Mathai J Reichheld J Banner BF Bonkovsky HL Hepatotoxicity due to troglitazone: report of two cases and review of adverse events reported to the United States Food and Drug Administration Am J Gastroenterol 2000 95 1 272 276 10638596
- Nissen SE Wolski K Rosiglitazone revisited: an updated meta-analysis of risk for myocardial infarction and cardiovascular mortality Arch Intern Med 2010 170 14 1191 1201 20656674
- Lewis JD Ferrara A Peng T Risk of bladder cancer among diabetic patients treated with pioglitazone: interim report of a longitudinal cohort study Diabetes Care 2011 34 4 916 922 21447663
- Wishart DS Knox C Guo AC DrugBank: a knowledgebase for drugs, drug actions and drug targets Nucleic Acids Res 2008 36 Database issue D901 D906 18048412
- Berman HM Westbrook J Feng Z The protein data bank Nucleic Acids Res 2000 28 1 235 242 10592235
- Morris GM Huey R Olson AJ Using AutoDock for ligand-receptor docking Curr Protoc Bioinformatics 2008 Chapter 8 Unit 8.14
- Jiang X Kumar K Hu X Wallqvist A Reifman J DOVIS 2.0: an efficient and easy to use parallel virtual screening tool based on AutoDock 4.0 Chem Cent J 2008 2 18 18778471
- Huey R Morris GM Olson AJ Goodsell DS A semiempirical free energy force field with charge-based desolvation J Comput Chem 2007 28 6 1145 1152 17274016
- Morris GM Huey R Lindstrom W AutoDock4 and AutoDockTools4: automated docking with selective receptor flexibility J Comput Chem 2009 30 16 2785 2791 19399780
- Qian G Sural S Gu Y Pramanik S Similarity between Euclidean and cosine angle distance for nearest neighbor queries Journal of the American Society for Information Science 1999 60 9 772 778
- Graves RA Tontonoz P Spiegelman BM Analysis of a tissue-specific enhancer: ARF6 regulates adipogenic gene expression Mol Cell Biol 1992 12 3 1202 1208 1545801
- Reed MJ Meszaros K Entes LJ A new rat model of type 2 diabetes: the fat-fed, streptozotocin-treated rat Metab Clin Exp 2000 49 11 1390 1394 11092499
- Li X Brasseur P Agnamey P Long-lasting anticryptosporidial activity of nitazoxanide in an immunosuppressed rat model Folia Parasitol 2003 50 1 19 22 12735719
- Peitl B Döbrönte R Drimba L Involvement of cholecystokinin in baseline and post-prandial whole body insulin sensitivity in rats Eur J Pharmacol 2010 644 1–3 251 256 20624386
- DeFronzo RA Tobin JD Andres R Glucose clamp technique: a method for quantifying insulin secretion and resistance Am J Physiol 1979 237 3 E214 E223 382871
- Sherwin RS Kramer KJ Tobin JD A model of the kinetics of insulin in man J Clin Invest 1974 53 5 1481 1492 4856884
- Wallenstein S Zucker CL Fleiss JL Some statistical methods useful in circulation research Circ Res 1980 47 1 1 9 7379260
- Peitl B Németh J Pankucsi C Szilvássy Z Insulin sensitization induced by oral cicletanine in conscious rabbits Naunyn Schmiedebergs Arch Pharmacol 2006 373 6 429 439 16955283
- Danaei G Finucane MM Lu Y Singh GM Cowan MJ Paciorek CJ National, regional, and global trends in fasting plasma glucose and diabetes prevalence since 1980: systematic analysis of health examination surveys and epidemiological studies with 370 country-years and 2.7 million participants Lancet 2011 378 9785 31 40 21705069
- Mudaliar S Chang AR Henry RR Thiazolidinediones, peripheral edema, and type 2 diabetes: incidence, pathophysiology, and clinical implications Endocr Pract 2003 9 5 406 416 14583425
- Nesto RW Bell D Bonow RO Thiazolidinedione use, fluid retention, and congestive heart failure: a consensus statement from the American Heart Association and American Diabetes Association Diabetes Care 2004 27 1 256 263 14693998
- Loke YK Singh S Furberg CD Long-term use of thiazolidinediones and fractures in type 2 diabetes: a meta-analysis CMAJ 2009 180 1 32 39 19073651
- Zhang F Lavan BE Gregoire FM Selective modulators of PPAR-gamma activity: molecular aspects related to obesity and side-effects PPAR Res 2007 2007 32696 17389769
- Higgins LS Depaoli AM Selective peroxisome proliferator-activated receptor gamma (PPARgamma) modulation as a strategy for safer therapeutic PPARgamma activation Am J Clin Nutr 2010 91 1 267S 272S 19906796
- Kramer D Shapiro R Adler A Bush E Rondinone CM Insulin-sensitizing effect of rosiglitazone (BRL-49653) by regulation of glucose transporters in muscle and fat of Zucker rats Metab Clin Exp 2001 50 11 1294 1300 11699047
- Chakrabarti R Vikramadithyan RK Misra P Ragaglitazar: a novel PPAR alpha PPAR gamma agonist with potent lipid-lowering and insulin-sensitizing efficacy in animal models Br J Pharmacol 2003 140 3 527 537 12970088
- Hoffman PS Sisson G Croxen MA Antiparasitic drug nitazoxanide inhibits the pyruvate oxidoreductases of Helicobacter pylori, selected anaerobic bacteria and parasites, and Campylobacter jejuni Antimicrob Agents Chemother 2007 51 3 868 876 17158936
- Rossignol JF La Frazia S Chiappa L Ciucci A Santoro MG Thiazolides, a new class of anti-influenza molecules targeting viral hemagglutinin at the post-translational level J Biol Chem 2009 284 43 29798 29808 19638339
- Murphy JR Friedmann JC Pre-clinical toxicology of nitazoxanide – a new antiparasitic compound J Appl Toxicol 1985 5 2 49 52 3889119