Abstract
Purpose
Here, we propose an integrative analysis of genome-wide methylation and gene expression to provide new insight into the biological mechanisms of Cognitive behavioral therapy (CBT) in pediatric obsessive-compulsive disorder (OCD).
Patients and Methods
Twelve children and adolescents with OCD receiving CBT for the first time were classified as responders or non-responders after eight weeks of CBT. Differentially methylated positions (DMPs) and gene co-expression modules were identified using specific R software packages. Correlations between the DMPs and gene co-expression modules were investigated.
Results
Two genes were enriched with significant DMPs (Δβ > ± 0.2, FDR-adjusted p-value < 0.05): PIWIL1 and MIR886. The yellowgreen module of co-expressed genes was associated with CBT response (FDR-adjusted p-value = 0.0003). Significant correlations were observed between the yellowgreen module and the CpGs in PIWIL1 and MIR886 (p < 0.008). Patients showing hypermethylation in these CpGs presented an upregulation in the genes in the yellowgreen module.
Conclusion
Taken together, the preliminary results of this systems-level approach, despite the study limitations, provide evidence that the epigenetic regulation of ncRNAs could be a predictor of CBT response.
Limitations
The sample size limited the statistical power, and given that the study was hypothesis-driven, our results should be seen as preliminary.
Introduction
Obsessive-compulsive disorder (OCD) is a serious and common mental disorder with an estimated prevalence of 1% to 3% in children and adolescents.Citation1 Pediatric OCD is associated with significant distress and marked interpersonal, academic, and occupational impairments,Citation2 which can have a detrimental impact on psychosocial development.Citation3
Treatments for OCD include pharmacological approaches using selective serotonin reuptake inhibitors (SSRI) and psychological approaches involving cognitive behavioral therapy (CBT). The recommended first-line treatment for pediatric OCD is CBT. It has been proven to be more effective than no intervention and while comparable to treatment with SSRIs, CBT has a lower risk-to-benefit ratio compared to medication and a higher acceptability among patients and their families.Citation4 However, there is significant variability in how children and adolescents with OCD respond to CBT, with 39% of patients showing adequate remission of their symptoms.Citation5 Similar variability is observed when patients with early-onset OCD are treated with SSRI monotherapy (22% remission rates) or when CBT is combined with an SSRI (54% remission rates).Citation6
Clinical guidelines recommend CBT as the first-line treatment for patients with mild to moderate symptoms. It can be combined with an SSRI as the initial treatment in more severe cases or when there is no adequate response to CBT alone.Citation7,Citation8 Although the severity of OCD symptoms may help in guiding treatment selection, the observed variability in remission rates highlights the importance of identifying moderators and predictors of response to help clinicians optimize the initial treatment for a particular patient.
Several factors have been proposed as predictors of a poorer outcome to CBT in pediatric OCD, such as an older age, the severity of symptoms and impairment, co-morbidities and a family history of OCD.Citation9 However, their importance and validity as predictors remain controversial. Genetic variants represent a potential source of predictors, with the study of such variants referred to as therapy genetics. The first evidence of the contribution of genetic variants to psychological therapy response came from candidate gene studies; however, these findings have proven to be difficult to replicate.Citation10,Citation11 Recently, genome-wide association studies (GWAS) on outcomes following psychological therapy were published for both children and adults with anxiety disorders.Citation12,Citation13 However, these studies were underpowered to detect the small effect size of single genetic variants with genome-wide significance.
Several investigations have explored the epigenetic process of DNA methylation and differential gene expression in treatment response. Early studies using candidate gene (BDNF, NGF, FKBP5, and MAO-A) approaches have demonstrated that changes in DNA methylation may underlie response to psychological therapies in a variety of disorders including OCD.Citation14–Citation21 A small number of studies have examined the role of gene expression and the response to psychological therapy: two studies using FKBP5 as a candidate gene in post-traumatic stress disorder and two studies using genome-wide expression analysis in anxiety disorders.Citation12,Citation22–Citation24
Here, we performed a genome-wide methylation analysis using peripheral blood obtained after eight weeks of CBT from a cohort of children and adolescents with a diagnosis of OCD who were drug-naïve and never previously treated with psychological interventions. Furthermore, we integrated the methylation and gene expression profiles using samples from the same individuals. The main objective of the present study was to provide new insight into the biological mechanisms of CBT and to identify new candidate biomarkers of CBT response.
Materials and Methods
Subjects
Twelve children and adolescents aged between 8 and 16 years who were receiving CBT for the first time participated in the present study. None of the participants had been treated previously with antidepressants or other psychotropic drugs, or with psychological therapies. Patients were diagnosed using the Diagnostic and Statistical Manual of Mental Disorders-V (DSM-V).Citation25 The study was carried out at the Child and Adolescent Psychiatry and Psychology Service of the Institute of Neuroscience at the Hospital Clinic of Barcelona. The study was naturalistic and the treatment was established according to the Clinical Guidelines for the Treatment of Obsessive-Compulsive Disorder of the Child and Adolescent Psychiatry and Psychology Service. All procedures were approved by the Hospital Clínic ethics committee. Written informed consent was obtained from all the parents and verbal informed consent was given by all the participants following explanation of the procedures involved. All experiments were performed in accordance with relevant guidelines and regulations. This study was conducted in accordance with the Declaration of Helsinki.
Cognitive-behavioral therapy counseling consisted of one session that covered the psycho-educational aspects of OCD (nature of OCD, clinical characteristics and principles of behavior therapy) and twelve sessions (two sessions every week during the first month and a weekly session during the second month) based on exposure and response prevention.
Information on illness severity was obtained during the initial phase of the study using the Children’s Yale-Brown Obsessive Compulsive Scale (CYBOCS).Citation26 The same scale was administered after 8 weeks of CBT. Treatment response was evaluated using the percentage of improvement as follows: ((CYBOCS8weeks- CYBOCSbasal)/ CYBOCS basal)*100. Patients were classified as responders or non-responders according to the percentage of improvement after 8 weeks of CBT. Responders were patients with an improvement > 35%, while non-responders were those with an improvement < 25%. Patients with an improvement > 25% and < 35% were classified as partial responders.Citation27
Biological Samples
Two blood samples from each participant were collected before the start of CBT: one in EDTA (BD Vacutainer K2EDTA tubes; Becton Dickinson, Franklin Lakes, New Jersey, USA) and another in PAXgene Blood RNA tubes (Qiagen, Valencia, CA). Genomic DNA was extracted using the MagNA Pure LC DNA Isolation Kit and a MagNA Pure LC 2.0 instrument (Roche Diagnostics GmbH, Mannheim, Germany). DNA concentration and quality were measured using a NanoDrop 2000 spectrophotometer (Thermo Fisher Scientific, Surrey, CA). Genome-wide DNA methylation was profiled at the CEGEN-PRB3-ISCIII using the Illumina Infinium MethylationEPIC BeadChip Kit. Total RNA was isolated in accordance with the manufacturer’s protocol (PAXgene Blood RNA kit). RNA quality and quantity were measured by an Agilent Bioanalyzer 2100 (Santa Clara, CA). 1 μg of purified RNA from each of the samples was submitted to the Kompetenzzentrum fur Fluoreszente Bioanalytik Microarray Technology (KFB, BioPark Regensburg GmbH, Regensburg, Germany) for labeling and hybridization using Human Genome U219 array plates (Affymetrix, Santa Clara, CA, USA), following the manufacturer’s protocols.
Genome-Wide DNA Methylation Profiling
Raw intensity data (.IDAT) files were received and bioinformatics processes were conducted in house using the Chip Analysis Methylation Pipeline (ChAMP) Bioconductor package.Citation28 Raw IDAT files were used to load the data into the R environment with the champ.load function, which also allows for the probe QC and removal steps to occur simultaneously. Probes with weak signals (p < 0.01) (n = 3103), cross-reactive probes (n = 11), non-CpG probes (n = 2952), probes with < 3 beads in at least 5% of the samples per probe (n = 10,683), probes that bound to SNP sites (n = 96,500), and sex chromosome probes (n = 61,734) were all considered problematic for the accurate detection of downstream methylation. After removing these probes, 736,109 probes remained for downstream analysis. β values were then normalized using the champ.norm function, specifically with the beta-mixture quartile method (BMIQ function). Cell counts were measured using the champ.refbase function. The following cells were counted: CD8+ T cells, CD4+ T cells, natural killer (NK) cells, B cells, monocytes, and granulocytes. Next, the singular value decomposition (SVD) method was performed by champ.SVD to assess the amount and significance of the technical batch components, along with any potential confounding variables (eg, sex, age, and cell count) in our dataset. Using the champ.runCombat function, Combat algorithms were applied to correct for slide and array (significant components detected by the SVD method). No effect of sex, age or cell count was detected.
After filtering, normalization, and the detection of batches and covariates, differentially methylated positions (DMPs) were identified using the champ.DMP function, which implements the limma package to calculate the p-value for differential methylation using a linear model (FDR-adjusted p-values < 0.05). An absolute value of the difference between the β-value medians (Δβ) of responders and non-responders higher than 0.2 was set as the cut-off value to decrease the number of significant CpGs and identify sites with the more biologically relevant methylation differences.
Genome-Wide Expression Analysis and the WGCNA Procedure
Microarray data preprocessing was performed using the Babelomics 5 suite (http://www.babelomics.org/).Citation29 The data were standardized using robust multichip analysis. Multiple probes mapping to the same gene were merged using the average as the summary of the hybridization values. Co-expression modules were identified using the R software package for weighted gene co-expression network analysis (WGCNA).Citation30 The co-expression analysis involved constructing a matrix of pairwise correlations between all pairs of genes across all selected samples. Next, the matrix was raised to a soft-thresholding power (β = 8 in this study) to obtain an adjacency matrix. To identify modules of co-expressed genes, we constructed the topological overlap-based dissimilarity, which was then used as input to average linkage hierarchical clustering. This step resulted in a clustering tree (dendrogram) whose branches were identified for cutting based on their shape, using the dynamic tree-cutting algorithm. The above steps were performed using the automatic network construction and module detection function (blockwiseModules in WGCNA), with the following parameters: minModuleSize of 30, reassignThreshold of 0, and mergeCutHeight of 0.25. The modules were then tested for their associations with the trait by correlating module eigengenes with trait measurements.
We then used ClueGO v2.1, a Cytoscape plug-in, to perform a gene set enrichment analysis, as described previously.Citation31 Briefly, we selected the unstructured terms of biological processes from Gene Ontology (GO). Only terms with an adjusted p-value < 0.05 and experimental evidence were selected for analysis. Genes involved in significant modules were mapped to their enriched term based on the hypergeometric test (two-sided), with the p-value being corrected by the Benjamini-Hochberg method. ClueGO created a functional module network in which the different GO terms were clustered according to the strength of the association between the terms calculated using chance-corrected kappa statistics.
Statistical Analysis
Data were analyzed using the SPSS 22.0 software (IBM, Chicago, IL, USA). The normality of continuous variables was tested using the Kolmogorov–Smirnov and Shapiro–Wilk tests, while the equality of the variance between the groups was assessed using Levene’s test. Two-tailed p-values < 0.05 were considered to be of statistical significance. In genes enriched with DMPs significantly associated with CBT response (Δβ > 0.2, FDR-adjusted p-values < 0.05), the β values of the most significant DMPs in each gene were tested for correlation, using Spearman’s rank correlation coefficient, with the eigengene values of the modules significantly associated with CBT response in the WGCNA.
Results
An overview of the study design is shown in . shows the demographic and clinical characteristics of the study participants. As can be observed, there were non-significant differences between the responders and non-responders for age, sex, symptom severity at baseline and family history of OCD. Although non-significant, a higher percentage of non-responders (100%) than responders (50%) presented co-morbidities.
Table 1 Demographic and Clinical Characteristics of the Study Participants
Genome-Wide Methylation Analysis
We classified 55,149 probes as significant DMPs (FDR-adjusted p-values < 0.05). However, this included DMPs with very small differences in methylation between responders and non-responders. Therefore, a cut-off of Δβ > ± 0.2 was applied, which identified 89 DMPs with methylation changes that were more likely to be biologically relevant (Supplementary Table 1).
The 89 significant CpGs mapped to 70 genes. Two of these genes were enriched with significant DMPs (FDR-adjusted p-value < 0.05, Δβ > ± 0.2) and were selected for subsequent analysis: PIWIL1 and MIR886. PIWIL1 was enriched with five CpGs that were significantly hypermethylated in the non-responders. These DMPs were upstream of the transcription start site (from +1500 to +200 bp), in a region that, according to the UCSC browser, includes a CpG island and a DNase hypersensitive site (). The most significant CpG in PIWIL1 (cg13861644) is included in the Blood Brain DNA Methylation Comparison Tool (https://epigenetics.essex.ac.uk/bloodbrain/),Citation32 showing a significant correlation (p < 0.05) between methylation levels in the blood and in the prefrontal cortex (r = 0.76), entorhinal cortex (r = 0.83), superior temporal gyrus (r = 0.77) and cerebellum (r = 0.73) (Supplementary Figure 1).
Figure 2 (A) Distribution of significant DMPs (FDR-adjusted p-value < 0.05, Δβ > ± 0.2) in the PIWIL1 gene and methylation β values in responders and non-responders. (B) Distribution of significant DMPs (FDR-adjusted p-value < 0.05, Δβ > ± 0.2) in the MIR886 gene and methylation β values in responders and non-responders. (C) Module eigengene values (y-axis) for the yellowgreen module in individual samples (x-axis). Black bars indicate non-responders, while gray bars indicate responders. (D) Scatter plots showing correlations between yellowgreen module eigengene values (x-axis) and methylation β values of the cg13861644 in PIWIL1 (y-axis). Black points correspond to non-responders, while gray points correspond to responders. (E) Scatter plots showing correlations between yellowgreen module eigengene values (x-axis) and methylation β values of the cg04481923 in MIR886 (y-axis). Black points correspond to non-responders, while gray points correspond to responders.
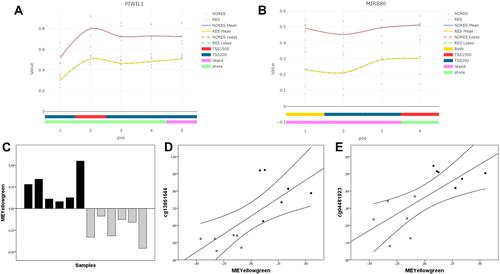
MIR886 was enriched with four DMPs that were significantly hypomethylated in the responders. These CpGs were upstream of the transcription start site (from +1500 to +200 bp), a region that, according to the UCSC browser, includes a promoter region enriched with H3K27AC marks in all the cell lines considered by ENCODE (). The four CpGs in MIR886 are included in the Blood Brain DNA Methylation Comparison Tool, showing a significant correlation (p < 0.05) between methylation levels in the blood and in the prefrontal cortex (r > 0.89), entorhinal cortex (r = 0.95), superior temporal gyrus (r > 0.92) and cerebellum (r > 0.52) (Supplementary Figure 2).
Genome-Wide Expression Analysis
We applied WGCNA to genome-wide expression data, which identified 70 gene co-expression modules (Supplementary Figure 3). One module, the yellowgreen (197 genes), showed a significant correlation with CBT response (r = −0.85, FDR-corrected p-value = 0.0003). The yellowgreen module contained genes that were upregulated in non-responders to CBT ().
To explore the biological mechanism associated with the genes of the yellowgreen module, we performed a gene set enrichment analysis using the unstructured terms of biological processes from Gene Ontology (GO). We identified five clusters involving ten significant terms (Bonferroni-corrected p-value < 0.05) () that were related to DNA replication, chemotaxis, hormone metabolism and catecholamine transport.
Table 2 Gene Set Enrichment Analysis of Biological Processes from Gene Ontology (GO) Obtained for the Yellowgreen Module. The Table Shows the GO Terms Identified, Their Cluster Distribution According to ClueGO, Their Bonferroni-Corrected p-values and the Associated Genes Found in the Yellowgreen Module
Integrative Analysis of Methylation and Gene Expression
We next investigated the possible relationship between the differences in DNA methylation between the responders and non-responders and the gene co-expression modules that were associated with CBT response. We analyzed the correlation between the β values of the most significant DMP in the PIWIL1 and MIR886 genes and the module eigengene values. There were significant correlations between the yellowgreen module and the cg13861644 in PIWIL1 (r = 0.74, p = 0.005) and the cg04481923 in MIR886 (r = 0.72, p = 0.008). Patients showing higher methylation in these CpGs showed an upregulation of the genes in the yellowgreen module ( and ).
We also analyzed the correlation between the β values of the most significant DMP in the PIWIL1 and its expression. The Human Genome U219 array plates only includes probes for the PIWIL1 gene but not for the MIR886. Non-significant correlation between methylation and expression was detected between cg13861644, the most significant DMP in the PIWIL1 gene, and its expression in the microarray. Although non-responders showed lower gene expression of PIWIL1 (4.5±1.9), in agreement with the observed hypermethylation, than responders (5.3±0.3), the difference was not significant (p>0.05).
Discussion
To our knowledge, the present study is the first to analyze and integrate differences in DNA methylation and gene expression in association with CBT response in the peripheral blood of children and adolescents with early-onset OCD. We identified two genes, PIWIL1 and MIR886, that were enriched in significant CpG sites that showed meaningful differences (Δβ > ± 0.2) in the methylation level between responders and non-responders and also a strong correlation in DNA methylation between the blood and brain. These CpGs showed higher methylation levels in non-responders and were associated with a module of 197 genes that were co-expressed and upregulated in the non-responders. Interestingly, PIWIL1 and MIR886 are involved in the tight control of gene expression with non-coding RNAs (ncRNAs). Small ncRNAs have roles in neuronal function, cognition, learning and memory.Citation33
PIWIL1 encodes a Piwi-like protein that forms an evolutionarily-conserved gene regulatory mechanism together with the Piwi-interacting RNAs (piRNAs), a class of small ncRNAs. Piwi proteins and piRNAs are found primarily within the male germline, where they are necessary for germ cell maintenance and spermatogenesis because they protect the genome by silencing transposon expression at both the epigenetic and post-transcriptional levels.Citation34–Citation37 In addition to their role in germline genome defence, there is growing recognition that the Piwi pathway is involved in neuronal development throughout the lifespan and in neuronal gene regulation in the adult brain.Citation38–Citation42 Moreover, functional disruption of the Piwi pathway has indicated that it is also involved in learning and memory and in the regulation of behavioral responses to the environment.Citation43 These findings are consistent with the strong association between coding mutations in the Piwi genes in humans and autism.Citation44
MIR886 is a Pol III non-coding RNA 886 gene (nc886), which was previously proposed to encode a pre-miR-886 or an RNA component of the vault complex referred to as vtRNA2-1.Citation45 However, a later study did not find any evidence that nc886 gives rise to microRNAs or that it associates with the vault complex.Citation46 This gene was previously shown to be elevated in Friedreich’s ataxia and differentially methylated in Parkinson’s disease.Citation47–Citation49 nc886 has a CpG island in its upstream region that is maternally imprinted.Citation50 Genomic imprinting is the monoallelic expression of a subset of genes in a conserved, parent-of-origin fashion. The frequency of imprinting of the nc886 CpG island in children has been associated with the genetic background and has also been linked to the mother’s age and season of conception, indicating that genetic and environmental factors may affect the establishment of imprinting, which is closely associated with human physiology.Citation50,Citation51 Changes in gene expression of imprinted sites within the placenta, including of MIR886, that are suggestive of an altered imprinting status have been linked to newborn neurobehavioral outcomes.Citation52
The genes in the yellowgreen module are associated with several biological processes such as DNA replication, chemotaxis, hormone metabolism and catecholamine transport. These results agree with those of one of the two studies using genome-wide expression analysis of CBT response in anxiety disorders, which identified similar GO terms of “DNA transcription and elongation” and “positive regulation of chemotaxis”.Citation12
Although correlations between DNA methylation in promoter regions and gene expression have been reported,Citation53 in our study we did not observed this effect. This could be due to the small sample size of our study. However, it could also be related to the complex mechanisms implicated in the epigenetic regulation of gene expression. The hypermethylation observed in the promoter region of PIWIL1 could not affect the basal expression of this gene but could modify its regulation by transcription factors that participate in the modulatory effects exerted by CBT therapy.
The findings of this study should be interpreted by bearing in mind several important limitations. The sample size limited the statistical power of the study and made it difficult to detect small or modest effects on DNA methylation or gene expression. Given that the study was hypothesis-driven and due to the small sample size, our results should be seen as preliminary and should be considered as exploratory findings that require further confirmation. However, it should be noted that our sample comprised patients with early-onset OCD. Thus, the sample represented a homogeneous clinical population who had not been previously treated and who were at the initial stages of the illness. Moreover, several potential confounders were controlled for, such as age, smoking status, pharmacological treatment and the course of the disease. We also controlled for blood cell composition, as DNA methylation is cell type-specific and different cell compositions between samples could affect the methylation data obtained.
In conclusion and despite the study limitations, our results provide evidence that the epigenetic regulation of ncRNAs could be a predictor of CBT response and might be related to differences in the expression of genes involved in biological processes associated with CBT response. Our results have to be replicated in large samples before using the methylation level of these specific genes as predictive biomarkers with clinical application.
Data Sharing Statement
The data that support the findings of this study are available from the corresponding author upon reasonable request.
Role of Funding Sources
Funding sources had no further role in study design; in the collection, analysis and interpretation of data; in the writing of the report; and in the decision to submit the paper for publication.
Author Contributions
All authors made a significant contribution to the work reported, whether that is in the conception, study design, execution, acquisition of data, analysis and interpretation, or in all these areas; took part in drafting, revising or critically reviewing the article; gave final approval of the version to be published; have agreed on the journal to which the article has been submitted; and agree to be accountable for all aspects of the work.
Acknowledgments
This work was supported by the Alicia Koplowitz Foundation; Ministerio de Economía y Competitividad- Instituto de Salud Carlos III- Fondo Europeo de Desarrollo Regional (FEDER)-Unión Europea (PI16/01086, PI19/01122). Support was also given by the CERCA Programme/the Government of Catalonia, Secretaria d’Universitats i Recerca del Departament d’Economia i Coneixement to the “Child Psychiatry and Psychology Group” (2017SGR881) and to the “Clinical Pharmacology and Pharmacogenetics Group” (2017SGR1562). The authors thank the Language Advisory Service at the University of Barcelona for manuscript revision. The authors also thank all subjects and their families for the time and effort spent on this study.
Disclosure
The authors declare no conflicts of interest for this work.
References
- Heyman I, Fombonne E, Simmons H, et al. Prevalence of obsessive-compulsive disorder in the British nationwide survey of child mental health. Br J Psychiatry. 2001;179(4):324–329. doi:10.1192/bjp.179.4.324
- Piacentini J, Bergman RL, Keller M, McCracken J. Functional impairment in children and adolescents with obsessive-compulsive disorder. J Child Adolesc Psychopharmacol. 2003;13(Suppl 1):S61–S69. doi:10.1089/104454603322126359
- Stewart SE, Geller DA, Jenike M, et al. Long-term outcome of pediatric obsessive-compulsive disorder: a meta-analysis and qualitative review of the literature. Acta Psychiatr Scand. 2004;110(1):4–13. doi:10.1111/j.1600-0447.2004.00302.x
- Uhre CF, Uhre VF, Lønfeldt NN, et al. Systematic Review and Meta-Analysis: cognitive-Behavioral Therapy for Obsessive-Compulsive Disorder in Children and Adolescents. J Am Acad Child Adolesc Psychiatry. 2020;59(1):64–77. doi:10.1016/j.jaac.2019.08.480
- de Haan E. Effective treatment Of OCD? J Am Acad Child Adolesc Psychiatry. 2006;45(4):383. doi:10.1097/01.chi.0000205697.73873.c1
- March J, Silva S, Petrycki S, et al. Fluoxetine, cognitive-behavioral therapy, and their combination for adolescents with depression: treatment for Adolescents With Depression Study (TADS) randomized controlled trial. JAMA. 2004;292(7):807–820.
- Geller DA, March J, American Academy of Child and Adolescent Psychiatry (AACAP). Practice parameter for the assessment and treatment of children and adolescents with obsessive-compulsive disorder. J Am Acad Child Adolesc Psychiatry. 2012;51(1):98–113. doi:10.1016/j.jaac.2011.09.019
- National Institute for Health and Clinical Excellence (NICE). Obsessive-Compulsive Disorder: Core Interventions in the Treatment of Obsessive-Compulsive Disorder and Body Dysmorphic Disorder. London: NICE; 2005.
- Turner C, O’Gorman B, Nair A, O’Kearney R. Moderators and predictors of response to cognitive behaviour therapy for pediatric obsessive-compulsive disorder: a systematic review. Psychiatry Res. 2018;261:50–60. doi:10.1016/j.psychres.2017.12.034
- Lester KJ, Eley TC. Therapygenetics: using genetic markers to predict response to psychological treatment for mood and anxiety disorders. Biol Mood Anxiety Disord. 2013;3(1):4. doi:10.1186/2045-5380-3-4
- Lester KJ, Roberts S, Keers R, et al. Non-replication of the association between 5HTTLPR and response to psychological therapy for child anxiety disorders. Br J Psychiatry. 2016;208(2):182–188. doi:10.1192/bjp.bp.114.154997
- Coleman JR, Lester KJ, Roberts S, et al. Separate and combined effects of genetic variants and pre-treatment whole blood gene expression on response to exposure-based cognitive behavioural therapy for anxiety disorders. World J Biol Psychiatry. 2017;18(3):215–226. doi:10.1080/15622975.2016.1208841
- Rayner C, Coleman JRI, Purves KL, et al. A genome-wide association meta-analysis of prognostic outcomes following cognitive behavioural therapy in individuals with anxiety and depressive disorders. Transl Psychiatry. 2019;9(1):150. doi:10.1038/s41398-019-0481-y
- Perroud N, Salzmann A, Prada P, et al. Response to psychotherapy in borderline personality disorder and methylation status of the BDNF gene. Transl Psychiatry. 2013;3(1):e207. doi:10.1038/tp.2012.140
- Roberts S, Lester KJ, Hudson JL, et al. Serotonin transporter methylation and response to cognitive behaviour therapy in children with anxiety disorders. Transl Psychiatry. 2014;4(9):e444. doi:10.1038/tp.2014.83
- Ziegler C, Richter J, Mahr M, et al. MAOA gene hypomethylation in panic disorder-reversibility of an epigenetic risk pattern by psychotherapy. Transl Psychiatry. 2016;6(4):e773. doi:10.1038/tp.2016.41
- Schiele MA, Thiel C, Deckert J, Zaudig M, Berberich G, Domschke K. Monoamine Oxidase A Hypomethylation in Obsessive-Compulsive Disorder: reversibility By Successful Psychotherapy? Int J Neuropsychopharmacol. 2020;23(5):319–323. doi:10.1093/ijnp/pyaa016
- Roberts S, Keers R, Breen G, et al. DNA methylation of FKBP5 and response to exposure-based psychological therapy. Am J Med Genet B Neuropsychiatr Genet. 2019;180(2):150–158. doi:10.1002/ajmg.b.32650
- Schiele MA, Thiel C, Kollert L, et al. Oxytocin Receptor Gene DNA Methylation: a Biomarker of Treatment Response in Obsessive-Compulsive Disorder? Psychother Psychosom. 2020:1–7. doi:10.1159/000509910
- Schiele MA, Thiel C, Weidner M, et al. Serotonin transporter gene promoter hypomethylation in obsessive-compulsive disorder - Predictor of impaired response to exposure treatment? J Psychiatr Res. 2020;132:18–22. doi:10.1016/j.jpsychires.2020.09.034
- Schiele MA, Ziegler C, Kollert L, et al. Plasticity of Functional MAOA Gene Methylation in Acrophobia. Int J Neuropsychopharmacol. 2018;21(9):822–827. doi:10.1093/ijnp/pyy050
- Levy-Gigi E, Szabó C, Kelemen O, Kéri S. Association among clinical response, hippocampal volume, and FKBP5 gene expression in individuals with posttraumatic stress disorder receiving cognitive behavioral therapy. Biol Psychiatry. 2013;74(11):793–800. doi:10.1016/j.biopsych.2013.05.017
- Szabó C, Kelemen O, Kéri S. Changes in FKBP5 expression and memory functions during cognitive-behavioral therapy in posttraumatic stress disorder: a preliminary study. Neurosci Lett. 2014;569:116–120. doi:10.1016/j.neulet.2014.03.059
- Roberts S, Wong CCY, Breen G, et al. Genome-wide expression and response to exposure-based psychological therapy for anxiety disorders. Transl Psychiatry. 2017;7(8):e1219. doi:10.1038/tp.2017.177
- American Psychiatric Association. Diagnostic and Statistical Manual of Mental Disorders. Fifth ed. Arlington, VA: American Psychiatric Association; 2013.
- Scahill L, Riddle MA, McSwiggin-Hardin M, et al. Children’s Yale-Brown Obsessive Compulsive Scale: reliability and validity. J Am Acad Child Adolesc Psychiatry. 1997;36(6):844–852. doi:10.1097/00004583-199706000-00023
- Mataix-Cols D, de la Cruz LF, Nordsletten AE, Lenhard F, Isomura K, Simpson HB. Towards an international expert consensus for defining treatment response, remission, recovery and relapse in obsessive-compulsive disorder. World Psychiatry. 2013;15(1):80–81. doi:10.1002/wps.20299
- Tian Y, Morris TJ, Webster AP, et al. ChAMP: updated methylation analysis pipeline for Illumina BeadChips. Bioinformatics. 2017;33(24):3982–3984. doi:10.1093/bioinformatics/btx513
- Alonso R, Salavert F, Garcia-Garcia F, et al. Babelomics 5.0: functional interpretation for new generations of genomic data. Nucleic Acids Res. 2015;43(W1):W117–121. doi:10.1093/nar/gkv384
- Langfelder P, Horvath S. WGCNA: an R package for weighted correlation network analysis. BMC Bioinform. 2008;9(1):559. doi:10.1186/1471-2105-9-559
- Bindea G, Mlecnik B, Hackl H, et al. ClueGO: a Cytoscape plug-in to decipher functionally grouped gene ontology and pathway annotation networks. Bioinformatics. 2009;25(8):1091–1093. doi:10.1093/bioinformatics/btp101
- Hannon E, Lunnon K, Schalkwyk L, Mill J. Interindividual methylomic variation across blood, cortex, and cerebellum: implications for epigenetic studies of neurological and neuropsychiatric phenotypes. Epigenetics. 2015;10(11):1024–1032. doi:10.1080/15592294.2015.1100786
- Fiorenza A, Barco A. Role of Dicer and the miRNA system in neuronal plasticity and brain function. Neurobiol Learn Mem. 2016;135:3–12. doi:10.1016/j.nlm.2016.05.001
- Deng W, Lin H. miwi, a murine homolog of piwi, encodes a cytoplasmic protein essential for spermatogenesis. Dev Cell. 2002;2(6):819–830. doi:10.1016/S1534-5807(02)00165-X
- Kuramochi-Miyagawa S, Kimura T, Ijiri TW, et al. Mili, a mammalian member of piwi family gene, is essential for spermatogenesis. Development. 2004;131(4):839–849. doi:10.1242/dev.00973
- Carmell MA, Girard A, van de Kant HJ, et al. MIWI2 is essential for spermatogenesis and repression of transposons in the mouse male germline. Dev Cell. 2007;12(4):503–514. doi:10.1016/j.devcel.2007.03.001
- Iwasaki YW, Siomi MC, Siomi H. PIWI-Interacting RNA: its Biogenesis and Functions. Annu Rev Biochem. 2015;84:405–433.
- Zhao PP, Yao MJ, Chang SY, et al. Novel function of PIWIL1 in neuronal polarization and migration via regulation of microtubule-associated proteins. Mol Brain. 2015;8:39. doi:10.1186/s13041-015-0131-0
- Ponnusamy M, Yan KW, Liu CY, Li PF, Wang K. PIWI family emerging as a decisive factor of cell fate: an overview. Eur J Cell Biol. 2017;96(8):746–757. doi:10.1016/j.ejcb.2017.09.004
- Lee KS, Park JL, Lee K, et al. nc886, a non-coding RNA of anti-proliferative role, is suppressed by CpG DNA methylation in human gastric cancer. Oncotarget. 2014;5(11):3944–3955. doi:10.18632/oncotarget.2047
- Nandi S, Chandramohan D, Fioriti L, et al. Roles for small noncoding RNAs in silencing of retrotransposons in the mammalian brain. Proc Natl Acad Sci U S A. 2016;113(45):12697–12702. doi:10.1073/pnas.1609287113
- Leighton LJ, Zhao Q, Li X, et al. A Functional Role for the Epigenetic Regulator ING1 in Activity-induced Gene Expression in Primary Cortical Neurons. Neuroscience. 2018;369:248–260. doi:10.1016/j.neuroscience.2017.11.018
- Leighton LJ, Wei W, Marshall PR, et al. Disrupting the hippocampal Piwi pathway enhances contextual fear memory in mice. Neurobiol Learn Mem. 2019;161:202–209. doi:10.1016/j.nlm.2019.04.002
- Iossifov I, O’Roak BJ, Sanders SJ, et al. The contribution of de novo coding mutations to autism spectrum disorder. Nature. 2014;515(7526):216–221. doi:10.1038/nature13908
- Landgraf P, Rusu M, Sheridan R, et al. A mammalian microRNA expression atlas based on small RNA library sequencing. Cell. 2007;129(7):1401–1414. doi:10.1016/j.cell.2007.04.040
- Lee EJ, Banerjee S, Zhou H, et al. Identification of piRNAs in the central nervous system. RNA. 2011;17(6):1090–1099. doi:10.1261/rna.2565011
- Mahishi LH, Hart RP, Lynch DR, Ratan RR. miR-886-3p levels are elevated in Friedreich ataxia. J Neurosci. 2012;32(27):9369–9373. doi:10.1523/JNEUROSCI.0059-12.2012
- Masliah E, Dumaop W, Galasko D, Desplats P. Distinctive patterns of DNA methylation associated with Parkinson disease: identification of concordant epigenetic changes in brain and peripheral blood leukocytes. Epigenetics. 2013;8(10):1030–1038. doi:10.4161/epi.25865
- Kaut O, Schmitt I, Tost J, et al. Epigenome-wide DNA methylation analysis in siblings and monozygotic twins discordant for sporadic Parkinson’s disease revealed different epigenetic patterns in peripheral blood mononuclear cells. Neurogenetics. 2017;18(1):7–22. doi:10.1007/s10048-016-0497-x
- Silver MJ, Kessler NJ, Hennig BJ, et al. Independent genomewide screens identify the tumor suppressor VTRNA2-1 as a human epiallele responsive to periconceptional environment. Genome Biol. 2015;16(1):118. doi:10.1186/s13059-015-0660-y
- Carpenter BL, Zhou W, Madaj Z, et al. Mother-child transmission of epigenetic information by tunable polymorphic imprinting. Proc Natl Acad Sci U S A. 2018;115(51):E11970–E11977. doi:10.1073/pnas.1815005115
- Green BB, Kappil M, Lambertini L, et al. Expression of imprinted genes in placenta is associated with infant neurobehavioral development. Epigenetics. 2015;10(9):834–841. doi:10.1080/15592294.2015.1073880
- Chen C, Zhang C, Cheng L, et al. Correlation between DNA methylation and gene expression in the brains of patients with bipolar disorder and schizophrenia. Bipolar Disord. 2014;16(8):790–799. doi:10.1111/bdi.12255