Abstract
In this AMEE Guide, we consider the design and development of self-administered surveys, commonly called questionnaires. Questionnaires are widely employed in medical education research. Unfortunately, the processes used to develop such questionnaires vary in quality and lack consistent, rigorous standards. Consequently, the quality of the questionnaires used in medical education research is highly variable. To address this problem, this AMEE Guide presents a systematic, seven-step process for designing high-quality questionnaires, with particular emphasis on developing survey scales. These seven steps do not address all aspects of survey design, nor do they represent the only way to develop a high-quality questionnaire. Instead, these steps synthesize multiple survey design techniques and organize them into a cohesive process for questionnaire developers of all levels. Addressing each of these steps systematically will improve the probabilities that survey designers will accurately measure what they intend to measure.
Introduction: Questionnaires in medical education research
Surveys are used throughout medical education. Examples include the ubiquitous student evaluation of medical school courses and clerkships, as well as patient satisfaction and student self-assessment surveys. In addition, survey instruments are widely employed in medical education research. In our recent review of original research articles published in Medical Teacher in 2011 and 2012, we found that 37 articles (24%) included surveys as part of the study design. Similarly, surveys are commonly used in graduate medical education research. Across the same two-year period (2011–2012), 75% of the research articles published in the Journal of Graduate Medical Education used surveys.
Despite the widespread use of surveys in medical education, the medical education literature provides limited guidance on the best way to design a survey (Gehlbach et al. Citation2010). Consequently, many surveys fail to use rigorous methodologies or “best practices” in survey design. As a result, the reliability of the scores that emerge from surveys is often inadequate, as is the validity of the scores’ intended interpretation and use. Stated another way, when surveys are poorly designed, they may fail to capture the essence of what the survey developer is attempting to measure due to different types of measurement error. For example, poor question wording, confusing question layout and inadequate response options can all affect the reliability and validity of the data from surveys, making it extremely difficult to draw useful conclusions (Sullivan Citation2011). With these problems as a backdrop, our purpose in this AMEE Guide is to describe a systematic process for developing and collecting reliability and validity evidence for survey instruments used in medical education and medical education research. In doing so, we hope to provide medical educators with a practical guide for improving the quality of the surveys they design for evaluation and research purposes.
A systematic, seven-step process for survey scale design
The term “survey” is quite broad and could include the questions used in a phone interview, the set of items employed in a focus group and the questions on a self-administered patient survey (Dillman et al. Citation2009). Although the processes described in this AMEE Guide can be used to improve all of the above, we focus primarily on self-administered surveys, which are often referred to as questionnaires. For most questionnaires, the overarching goals are to develop a set of items that every respondent will interpret the same way, respond to accurately and be willing and motivated to answer. The seven steps depicted in , and described below, do not address all aspects of survey design nor do they represent the only way to develop a high-quality questionnaire. Rather, these steps consolidate and organize the plethora of survey design techniques that exist in the social sciences and guide questionnaire developers through a cohesive process. Addressing each step systematically will optimize the quality of medical education questionnaires and improve the chances of collecting high-quality survey data.
Table 1. A seven-step, survey scale design process for medical education researchers.
Questionnaires are good for gathering data about abstract ideas or concepts that are otherwise difficult to quantify, such as opinions, attitudes and beliefs. In addition, questionnaires can be useful for collecting information about behaviors that are not directly observable (e.g. studying at home), assuming respondents are willing and able to report on those behaviors. Before creating a questionnaire, however, it is imperative to first decide if a survey is the best method to address the research question or construct of interest. A construct is the model, idea or theory that the researcher is attempting to assess. In medical education, many constructs of interest are not directly observable – student satisfaction with a new curriculum, patients’ ratings of their physical discomfort, etc. Because documenting these phenomena requires measuring people’s perceptions, questionnaires are often the most pragmatic approach to assessing these constructs.
In medical education, many constructs are well suited for assessment using questionnaires. However, because psychological, non-observable constructs such as teacher motivation, physician confidence and student satisfaction do not have a commonly agreed upon metric, they are difficult to measure with a single item on a questionnaire. In other words, for some constructs such as weight or distance, most everyone agrees upon the units and the approach to measurement, and so a single measurement may be adequate. However, for non-observable, psychological constructs, a survey scale is often required for more accurate measurement. Survey scales are groups of similar items on a questionnaire designed to assess the same underlying construct (DeVellis 2003). Although scales are more difficult to develop and take longer to complete, they offer researchers many advantages. In particular, scales more completely, precisely and consistently assess the underlying construct (McIver & Carmines Citation1981). Thus, scales are commonly used in many fields, including medical education, psychology and political science. As an example, consider a medical education researcher interested in assessing medical student satisfaction. One approach would be to simply ask one question about satisfaction (e.g. How satisfied were you with medical school?). A better approach, however, would be to ask a series of questions designed to capture the different facets of this satisfaction construct (e.g. How satisfied were you with the teaching facilities? How effective were your instructors? and How easy was the scheduling process?). Using this approach, a mean score of all the items within a particular scale can be calculated and used in the research study.
Because of the benefits of assessing these types of psychological constructs through scales, the survey design process that we now turn to will focus particularly on the development of scales.
Step 1: Conduct a literature review
The first step to developing a questionnaire is to perform a literature review. There are two primary purposes for the literature review: (1) to clearly define the construct and (2) to determine if measures of the construct (or related constructs) already exist. A review of the literature helps to ensure the construct definition aligns with related theory and research in the field, while at the same time helping the researcher identify survey scales or items that could be used or adapted for the current purpose (Gehlbach et al. Citation2010).
Formulating a clear definition of the construct is an indispensable first step in any validity study (Cook & Beckman Citation2006). A good definition will clarify how the construct is positioned within the existing literature, how it relates to other constructs and how it is different from related constructs (Gehlbach & Brinkworth Citation2011). A well-formulated definition also helps to determine the level of abstraction at which to measure a given construct (the so-called “grain size”, as defined by Gehlbach & Brinkworth Citation2011). For example, to examine medical trainees’ confidence to perform essential clinical skills, one could develop scales to assess their confidence to auscultate the heart (at the small-grain end of the spectrum), to conduct a physical exam (at the medium-grain end of the spectrum) or to perform the clinical skills essential to a given medical specialty (at the large-grain end of the spectrum).
Although many medical education researchers prefer to develop their own surveys independently, it may be more efficient to adapt an existing questionnaire – particularly if the authors of the existing questionnaire have collected validity evidence in previous work – than it is to start from scratch. When this is the case, a request to the authors to adapt their questionnaire will usually suffice. It is important to note, however, that the term “previously validated survey” is a misnomer. The validity of the scores that emerge from a given questionnaire or survey scale is sensitive to the survey’s target population, the local context and the intended use of the scale scores, among other factors. Thus, survey developers collect reliability and validity evidence for their survey scales in a specified context, with a particular sample, and for a particular purpose.
As described in the Standards for Educational and Psychological Testing, validity refers to the degree to which evidence and theory support a measure’s intended use (AERA, APA, & NCME Citation1999). The process of validation is the most fundamental consideration in developing and evaluating a measurement tool, and the process involves the accumulation of evidence across time, settings and samples to build a scientifically sound validity argument. Thus, establishing validity is an ongoing process of gathering evidence (Kane Citation2006). Furthermore, it is important to acknowledge that reliability and validity are not properties of the survey instrument, per se, but of the survey’s scores and their interpretations (AERA, APA, & NCME Citation1999). For example, a survey of trainee satisfaction might be appropriate for assessing aspects of student well-being, but such a survey would be inappropriate for selecting the most knowledgeable medical students. In this example, the survey did not change, only the score interpretation changed (Cook & Beckman Citation2006).
Many good reasons exist to use, or slightly adapt, an existing questionnaire. By way of analogy, we can compare this practice to a physician who needs to decide on the best medical treatment. The vast majority of clinicians do not perform their own comparative research trials to determine the best treatments to use for their patients. Rather, they rely on the published research, as it would obviously be impractical for clinicians to perform such studies to address every disease process. Similarly, medical educators cannot develop their own questionnaires for every research question or educational intervention. Just like clinical trials, questionnaire development requires time, knowledge, skill and a fair amount of resources to accomplish correctly. Thus, an existing, well-designed questionnaire can often permit medical educators to put their limited resources elsewhere.
Continuing with the clinical research analogy, when clinicians identify a research report that is relevant to their clinical question, they must decide if it applies to their patient. Typically, this includes determining if the relationships identified in the study are causal (internal validity) and if the results apply to the clinician’s patient population (external validity). In a similar way, questionnaires identified in a literature search must be reviewed critically for validity evidence and then analyzed to determine if the questionnaire could be applied to the educator’s target audience. If survey designers find scales that closely match their construct, context and proposed use, such scales might be useable with only minor modification. In some cases, the items themselves might not be well written, but the content of the items might be helpful in writing new items (Gehlbach & Brinkworth Citation2011). Making such determinations will be easier the more the survey designer knows about the construct (through the literature review) and the best practices in item writing (as described in Step 4).
Step 2: Conduct interviews and/or focus groups
Once the literature review has shown that it is necessary to develop a new questionnaire, and helped to define the construct, the next step is to ascertain whether the conceptualization of the construct matches how prospective respondents think about it (Gehlbach & Brinkworth Citation2011). In other words, do respondents include and exclude the same features of the construct as those described in the literature? What language do respondents use when describing the construct? To answer these questions and ensure the construct is defined from multiple perspectives, researchers will usually want to collect data directly from individuals who closely resemble their population of interest.
To illustrate this step, another clinical analogy might be helpful. Many clinicians have had the experience of spending considerable time developing a medically appropriate treatment regimen but have poor patient compliance with that treatment (e.g. too expensive). The clinician and patient then must develop a new plan that is acceptable to both. Had the patient’s perspective been considered earlier, the original plan would likely have been more effective. Many clinicians have also experienced difficulty treating a patient, only to have a peer reframe the problem, which subsequently results in a better approach to treatment. A construct is no different. To this point, the researcher developing the questionnaire, like the clinician treating the patient, has given a great deal of thought to defining the construct. However, the researcher unavoidably brings his/her perspectives and biases to this definition, and the language used in the literature may be technical and difficult to understand. Thus, other perspectives are needed. Most importantly, how does the target population (the patient from the previous example) conceptualize and understand the construct? Just like the patient example, these perspectives are sometimes critical to the success of the project. For example, in reviewing the literature on student satisfaction with medical school instruction, a researcher may find no mention of the instructional practice of providing students with video or audio recordings of lectures (as these practices are fairly new). However, in talking with students, the researcher may find that today’s students are accustomed to such practices and consider them when forming their opinions about medical school instruction.
In order to accomplish Step 2 of the design process, the survey designer will need input from prospective respondents. Interviews and/or focus groups provide a sensible way to get this input. Irrespective of the approach taken, this step should be guided by two main objectives. First, researchers need to hear how participants talk about the construct in their own words, with little to no prompting from the researcher. Following the collection of unprompted information from participants, the survey designers can then ask more focused questions to evaluate if respondents agree with the way the construct has been characterized in the literature. This procedure should be repeated until saturation is reached; this occurs when the researcher is no longer hearing new information about how potential respondents conceptualize the construct (Gehlbach & Brinkworth Citation2011). The end result of these interviews and/or focus groups should be a detailed description of how potential respondents conceptualize and understand the construct. These data will then be used in Steps 3 and 4.
Step 3: Synthesize the literature review and interviews/focus groups
At this point, the definition of the construct has been shaped by the medical educator developing the questionnaire, the literature and the target audience. Step 3 seeks to reconcile these definitions. Because the construct definition directs all subsequent steps (e.g. development of items), the survey designer must take care to perform this step properly.
One suitable way to conduct Step 3 is to develop a comprehensive list of indicators for the construct by merging the results of the literature review and interviews/focus groups (Gehlbach & Brinkworth Citation2011). When these data sources produce similar lists, the process is uncomplicated. When these data are similar conceptually, but the literature and potential respondents describe the construct using different terminology, it makes sense to use the vocabulary of the potential respondents. For example, when assessing teacher confidence (sometimes referred to as teacher self-efficacy), it is probably more appropriate to ask teachers about their “confidence in trying out new teaching techniques” than to ask them about their “efficaciousness in experimenting with novel pedagogies” (Gehlbach et al. Citation2010). Finally, if an indicator is included from one source but not the other, most questionnaire designers will want to keep the item, at least initially. In later steps, designers will have opportunities to determine, through expert reviews (Step 5) and cognitive interviews (Step 6), if these items are still appropriate to the construct. Whatever the technique used to consolidate the data from Steps 1 and 2, the final definition and list of indicators should be comprehensive, reflecting both the literature and the opinions of the target audience.
It is worth noting that scholars may have good reasons to settle on a final construct definition that differs from what is found in the literature. However, when this occurs, it should be clear exactly how and why the construct definition is different. For example, is the target audiences’ perception different from previous work? Does a new educational theory apply? Whatever the reason, this justification will be needed for publication of the questionnaire. Having an explicit definition of the construct, with an explanation of how it is different from other versions of the construct, will help peers and researchers alike decide how to best use the questionnaire both in comparison with previous studies and with the development of new areas of research.
Step 4: Develop items
The goal of this step is to write survey items that adequately represent the construct of interest in a language that respondents can easily understand. One important design consideration is the number of items needed to adequately assess the construct. There is no easy answer to this question. The ideal number of items depends on several factors, including the complexity of the construct and the level at which one intends to assess it (i.e. the grain size). In general, it is good practice to develop more items than will ultimately be needed in the final scale (e.g. developing 15 potential items in the hopes of ultimately creating an eight-item scale), because some items will likely be deleted or revised later in the design process (Gehlbach & Brinkworth Citation2011). Ultimately, deciding on the number of items is a matter of professional judgment, but for most narrowly defined constructs, scales containing from 6 to 10 items will usually suffice in reliably capturing the essence of the phenomenon in question.
The next challenge is to write a set of clear, unambiguous items using the vocabulary of the target population. Although some aspects of item-writing remain an art form, an increasingly robust science and an accumulation of best practices should guide this process. For example, writing questions rather than statements, avoiding negatively worded items and biased language, matching the item stem to the response anchors and using response anchors that emphasize the construct being measured rather than employing general agreement response anchors (Artino et al. Citation2011) are all well-documented best practices. Although some medical education researchers may see these principles as “common sense”, experience tells us that these best practices are often violated.
Reviewing all the guidelines for how best to write items, construct response anchors and visually design individual survey items and entire questionnaires is beyond the scope of this AMEE Guide. As noted above, however, there are many excellent resources on the topic (e.g. DeVillis 2003; Dillman et al. Citation2009; Fowler 2009). To assist readers in grasping some of the more important and frequently ignored best practices, presents several item-writing pitfalls and offers solutions.
Table 2. Item-writing “best practices” based on scientific evidence from questionnaire design research.
Another important part of the questionnaire design process is selecting the response options that will be used for each item. Closed-ended survey items can have unordered (nominal) response options that have no natural order or ordered (ordinal) response options. Moreover, survey items can ask respondents to complete a ranking task (e.g. “rank the following items, where 1 = best and 6 = worst”) or a rating task that asks them to select an answer on a Likert-type response scale. Although it is outside the scope of this AMEE Guide to review all of the response options available, questionnaire designers are encouraged to tailor these options to the construct(s) they are attempting to assess (and to consult one of the many outstanding resources on the topic; e.g. Dillman et al. Citation2009; McCoach et al. Citation2013). To help readers understand some frequently ignored best practices and present several common mistakes designers commit when writing and formatting their response options. In addition, because Likert-type response scales are by far the most popular way of collecting survey responses – due, in large part, to their ease of use and adaptability for measuring many different constructs (McCoach et al. Citation2013) – provides several examples of five- and seven-point response scales that can be used when developing Likert-scaled survey instruments.
Figure 1 Visual-design “best practices” based on scientific evidence from questionnaire design research.
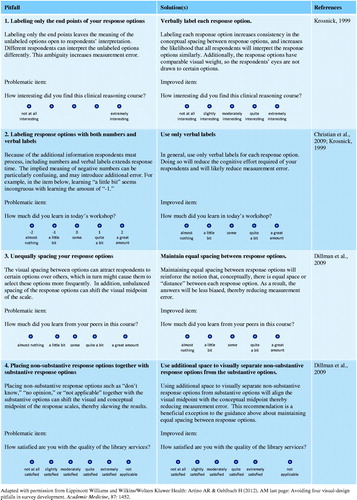
Table 3. Examples of various Likert-type response options.
Once survey designers finish drafting their items and selecting their response anchors, there are various sources of evidence that might be used to evaluate the validity of the questionnaire and its intended use. These sources of validity have been described in the Standards for Educational and Psychological Testing as evidence based on the following: (1) content, (2) response process, (3) internal structure, (4) relationships with other variables and (5) consequences (AERA, APA & NCME Citation1999). The next three steps of the design process fit nicely into this taxonomy and are described below.
Step 5: Conduct expert validation
Once the construct has been defined and draft items have been written, an important step in the development of a new questionnaire is to begin collecting validity evidence based on the survey’s content (so-called content validity) (AERA, APA & NCME Citation1999). This step involves collecting data from content experts to establish that individual survey items are relevant to the construct being measured and that key items or indicators have not been omitted (Polit & Beck Citation2004; Waltz et al. Citation2005). Using experts to systematically review the survey’s content can substantially improve the overall quality and representativeness of the scale items (Polit & Beck Citation2006).
Steps for establishing content validity for a new survey instrument can be found throughout the literature (e.g. McKenzie et al. Citation1999; Rubio et al. Citation2003). Below, we summarize several of the more important steps. First, before selecting a panel of experts to evaluate the content of a new questionnaire, specific criteria should be developed to determine who qualifies as an expert. These criteria are often based on experience or knowledge of the construct being measured, but, practically speaking, these criteria also are dependent on the willingness and availability of the individuals being asked to participate (McKenzie et al. Citation1999). One useful approach to finding experts is to identify authors from the reference lists of the articles reviewed during the literature search. There is no consensus in the literature regarding the number of experts that should be used for content validation; however, many of the quantitative techniques used to analyze expert input will be impacted by the number of experts employed. Rubio et al. (Citation2003) recommends using 6–10 experts, while acknowledging that more experts (up to 20) may generate a clearer consensus about the construct being assessed, as well as the quality and relevance of the proposed scale items.
In general, the key domains to assess through an expert validation process are representativeness, clarity, relevance and distribution. Representativeness is defined as how completely the items (as a whole) encompass the construct, clarity is how clearly the items are worded and relevance refers to the extent each item actually relates to specific aspects of the construct. The distribution of an item is not always measured during expert validation as it refers to the more subtle aspect of how “difficult” it would be for a respondent to select a high score on a particular item. In other words, an average medical student may find it very difficult to endorse the self-confidence item, “How confident are you that you can get a 100% on your anatomy exam”, but that same student may find it easier to strongly endorse the item, “How confident are you that you can pass the anatomy exam”. In general, survey developers should attempt to have a range of items of varying difficulty (Tourangeau et al. Citation2000).
Once a panel of experts has been identified, a content validation form can be created that defines the construct and gives experts the opportunity to provide feedback on any or all of the aforementioned topics. Each survey designer’s priorities for a content validation may differ; as such, designers are encouraged to customize their content validation forms to reflect those priorities.
There are a variety of methods for analyzing the quantitative data collected on an expert validation form, but regardless of the method used, criterion for the acceptability of an item or scale should be determined in advanced (Beck & Gable Citation2001). Common metrics used to make inclusion and exclusion decisions for individual items are the content validity ratio, the content validity index and the factorial validity index. For details on how to calculate and interpret these indices, see McKenzie et al. (Citation1999) and Rubio et al. (Citation2003). For a sample content validation form, see Gehlbach & Brinkworth (Citation2011).
In addition to collecting quantitative data, questionnaire designers should provide their experts with an opportunity to provide free-text comments. This approach can be particularly effective for learning what indicators or aspects of the construct are not well-represented by the existing items. The data gathered from the free-text comments and subsequent qualitative analysis often reveal information not identified by the quantitative data and may lead to meaningful additions (or subtractions) to items and scales (McKenzie et al. Citation1999).
There are many ways to analyze the content validity of a new survey through the use of expert validation. The best approach should look at various domains where the researchers have the greatest concerns about the scale (relevance, clarity, etc.) for each individual item and for each set of items or scale. The quantitative data combined with qualitative input from experts is designed to improve the content validity of the new questionnaire or survey scale and, ultimately, the overall functioning of the survey instrument.
Step 6: Conduct cognitive interviews
After the experts have helped refine the scale items, it is important to collect evidence of response process validity to assess how prospective participants interpret your items and response anchors (AERA, APA & NCME Citation1999). One means of collecting such evidence is achieved through a process known as cognitive interviewing or cognitive pre-testing (Willis Citation2005). Similar to how experts are utilized to determine the content validity of a new survey, it is equally important to determine how potential respondents interpret the items and if their interpretation matches what the survey designer has in mind (Willis Citation2005; Karabenick et al. Citation2007). Results from cognitive interviews can be helpful in identifying mistakes respondents make in their interpretation of the item or response options (Napoles-Springer et al. Citation2006; Karabenick et al. Citation2007). As a qualitative technique, analysis does not rely on statistical tests of numeric data but rather on coding and interpretation of written notes from the interview. Thus, the sample sizes used for cognitive interviewing are normally small and may involve just 10–30 participants (Willis & Artino Citation2013). For small-scale medical education research projects, as few as five to six participants may suffice, as long as the survey designer is sensitive to the potential for bias in very small samples (Willis & Artino Citation2013).
Cognitive interviewing employs techniques from psychology and has traditionally assumed that respondents go through a series of cognitive processes when responding to a survey. These steps include comprehension of an item stem and answer choices, retrieval of appropriate information from long-term memory, judgment based on comprehension of the item and their memory and finally selection of a response (Tourangeau et al. Citation2000). Because respondents can have difficulty at any stage, a cognitive interview should be designed and scripted to address any and all of these potential problems. An important first step in the cognitive interview process is to create coding criteria that reflects the survey creator’s intended meaning for each item (Karabenick et al. Citation2007), which can then be used to help interpret the responses gathered during the cognitive interview.
The two major techniques for conducting a cognitive interview are the think-aloud technique and verbal probing. The think-aloud technique requires respondents to verbalize every thought that they have while answering each item. Here, the interviewer simply supports this activity by encouraging the respondent to keep talking and to record what is said for later analysis (Willis & Artino Citation2013). This technique can provide valuable information, but it tends to be unnatural and difficult for most respondents, and it can result in reams of free-response data that the survey designer then needs to cull through.
A complementary procedure, verbal probing, is a more active form of data collection where the interviewer administers a series of probe questions designed to elicit specific information (Willis & Artino Citation2013; see for a list of commonly used verbal probes). Verbal probing is classically divided into concurrent and retrospective probing. In concurrent probing, the interviewer asks the respondent specific questions about their thought processes as the respondent answers each question. Although disruptive, concurrent probing has the advantage of allowing participants to respond to questions while their thoughts are recent. Retrospective probing, on the other hand, occurs after the participant has completed the entire survey (or section of the survey) and is generally less disruptive than concurrent probing. The downside of retrospective probing is the risk of recall bias and hindsight effects (Drennan Citation2003). A modification to the two verbal probing techniques is defined as immediate retrospective probing, which allows the interviewer to find natural break points in the survey. Immediate retrospective probing allows the interviewer to probe the respondent without interrupting between each item (Watt et al. Citation2008). This approach has the potential benefit of reducing the recall bias and hindsight effects while limiting the interviewer interruptions and decreasing the artificiality of the process. In practice, many cognitive interviews will actually use a mixture of think-aloud and verbal probing techniques to better identify potential errors.
Table 4. Examples of commonly used verbal probes.
Once a cognitive interview has been completed, there are several methods for analyzing the qualitative data obtained. One way to quantitatively analyze results from a cognitive interview is through coding. With this method, pre-determined codes are established for common respondent errors (e.g. respondent requests clarification), and the frequency of each type of error is tabulated for each item (Napoles-Springer et al. Citation2006). In addition, codes may be ranked according to the pre-determined severity of the error. Although the quantitative results of this analysis are often easily interpretable, this method may miss errors not readily predicted and may not fully explain why the error is occurring (Napoles-Springer et al. Citation2006). As such, a qualitative approach to the cognitive interview can also be employed through an interaction analysis. Typically, an interaction analysis attempts to describe and explain the ways in which people interpret and interact during a conversation, and this method can be applied during the administration of a cognitive interview to determine the meaning of responses (Napoles-Springer et al. Citation2006). Studies have demonstrated that the combination of coding and interaction analysis can be quite effective, providing more information about the “cognitive validity” of a new questionnaire (Napoles-Springer et al. Citation2006).
The importance of respondents understanding each item in a similar fashion is inherently related to the overall reliability of the scores from any new questionnaire. In addition, the necessity for respondents to understand each item in the way it was intended by the survey creator is integrally related to the validity of the survey and the inferences that can be made with the resulting data. Taken together, these two factors are critically important to creating a high-quality questionnaire, and each factor can be addressed through the use of a well-designed cognitive interview. Ultimately, regardless of the methods used to conduct the cognitive interviews and analyze the data, the information gathered should be used to modify and improve the overall questionnaire and individual survey items.
Step 7: Conduct pilot testing
Despite the best efforts of medical education researchers during the aforementioned survey design process, some survey items may still be problematic (Gehlbach & Brinkworth Citation2011). Thus, the next step is to pilot test the questionnaire and continue collecting validity evidence. Two of the most common approaches are based on internal structure and relationships with other variables (AERA, APA & NCME Citation1999). During pilot testing, members of the target population complete the survey in the planned delivery mode (e.g. web-based or paper-based format). The data obtained from the pilot test is then reviewed to evaluate item range and variance, assess score reliability of the whole scale and review item and composite score correlations. During this step, survey designers should also review descriptive statistics (e.g. means and standard deviations) and histograms, which demonstrate the distribution of responses by item. This analysis can aid in identifying items that may not be functioning in the way the designer intended.
To ascertain the internal structure of the questionnaire and to evaluate the extent to which items within a particular scale measure a single underlying construct (i.e. the scale’s uni-dimensionality), survey designers should consider using advanced statistical techniques such as factor analysis. Factor analysis is a statistical procedure designed to evaluate “the number of distinct constructs needed to account for the pattern of correlations among a set of measures” (Fabrigar & Wegener 2012, p. 3). To assess the dimensionality of a survey scale that has been deliberately constructed to assess a single construct (e.g. using the processes described in this study), we recommend using confirmatory factor analysis techniques; that said, other scholars have argued that exploratory factor analysis is more appropriate when analyzing new scales (McCoach et al. Citation2013). Regardless of the specific analysis employed, researchers should know that factor analysis techniques are often poorly understood and poorly implemented; fortunately, the literature is replete with many helpful guides (see, for example, Pett et al. Citation2003; McCoach et al. Citation2013).
Conducting a reliability analysis is another critical step in the pilot testing phase. The most common means of assessing scale reliability is by calculating a Cronbach’s alpha coefficient. Cronbach’s alpha is a measure of the internal consistency of the item scores (i.e. the extent to which the scores for the items on a scale correlate with one another). It is a function of the inter-item correlations and the total number of items on a particular scale. It is important to note that Cronbach’s alpha is not a good measure of a scale’s uni-dimensionality (measuring a single concept) as is often assumed (Schmitt Citation1996). Thus, in most cases, survey designers should first run a factor analysis, to assess the scale’s uni-dimensionality and then proceed with a reliability analysis, to assess the internal consistency of the item scores on the scale (Schmitt Citation1996). Because Cronbach’s alpha is sensitive to scale length, all other things being equal, a longer scale will generally have a higher Cronbach’s alpha. Of course, scale length and the associated increase in internal consistency reliability must be balanced with over-burdening respondents and the concomitant response errors that can occur when questionnaires become too long and respondents become fatigued. Finally, it is critical to recognize that reliability is a necessary but insufficient condition for validity (AERA, APA & NCME Citation1999). That is, to be considered valid, survey scores must first be reliable. However, scores that are reliable are not necessarily valid for a given purpose.
Once a scale’s uni-dimensionality and internal consistency have been assessed, survey designers often create composite scores for each scale. Depending on the research question being addressed, these composite scores can then be used as independent or dependent variables. When attempting to assess hard-to-measure educational constructs such as motivation, confidence and satisfaction, it usually makes sense to create a composite score for each survey scale than it does to use individual survey items as variables (Sullivan & Artino Citation2013). A composite score is simply a mean score (either weighted or unweighted) of all the items within a particular scale. Using mean scores has several distinct advantages over summing the items within a particular scale or subscale. First, mean scores are usually reported using the same response scale as the individual items; this approach facilitates more direct interpretation of the mean scores in terms of the response anchors. Second, the use of mean scores makes it clear how big (or small) measured differences really are when comparing individuals or groups. As Colliver et al. (Citation2010) warned, “the sums of ratings reflect both the ratings and the number of items, which magnifies differences between scores and makes differences appear more important than they are” (p. 591).
After composite scores have been created for each survey scale, the resulting variables can be examined to determine their relations to other variables that have been collected. The goal in this step is to determine if these associations are consistent with theory and previous research. So, for example, one might expect the composite scores from a scale designed to assess trainee confidence for suturing to be positively correlated with the number of successful suture procedures performed (since practice builds confidence) and negatively correlated with procedure-related anxiety (as more confident trainees also tend to be less anxious). In this way, survey designers are assessing the validity of the scales they have created in terms of their relationships to other variables (AERA, APA & NCME Citation1999). It is worth noting that in the aforementioned example, the survey designer is evaluating the correlations between the newly developed scale scores and both an objective measure (number of procedures) and a subjective measure (scores on an anxiety scale). Both of these are reasonable approaches to assessing a new scale’s relationships with other variables.
Concluding thoughts
In this AMEE Guide, we described a systematic, seven-step design process for developing survey scales. It should be noted that many important topics related to survey implementation and administration fall outside our focus on scale design and thus were not discussed in this guide. These topics include, but are not limited to, ethical approval for research questionnaires, administration format (paper vs. electronic), sampling techniques, obtaining high response rates, providing incentives and data management. These topics, and many more, are reviewed in detail elsewhere (e.g. Dillman et al. Citation2009). We also acknowledge that the survey design methodology presented here is not the only way to design and develop a high-quality questionnaire. In reading this Guide, however, we hope medical education researchers will come to appreciate the importance of following a systematic, evidence-based approach to questionnaire design. Doing so not only improves the questionnaires used in medical education but it also has the potential to positively impact the overall quality of medical education research, a large proportion of which employs questionnaires.
Glossary
Closed-ended question – A survey question with a finite number of response categories from which the respondent can choose.
Cognitive interviewing (or cognitive pre-testing) – An evidence-based qualitative method specifically designed to investigate whether a survey question satisfies its intended purpose.
Concurrent probing – A verbal probing technique wherein the interviewer administers the probe question immediately after the respondent has read aloud and answered each survey item.
Construct – A hypothesized concept or characteristic (something “constructed”) that a survey or test is designed to measure. Historically, the term “construct” has been reserved for characteristics that are not directly observable. Recently, however, the term has been more broadly defined.
Content validity – Evidence obtained from an analysis of the relationship between a survey instrument’s content and the construct it is intended to measure.
Factor analysis – A set of statistical procedures designed to evaluate the number of distinct constructs needed to account for the pattern of correlations among a set of measures.
Open-ended question – A survey question that asks respondents to provide an answer in an open space (e.g. a number, a list or a longer, in-depth answer).
Reliability – The extent to which the scores produced by a particular measurement procedure or instrument (e.g. a survey) are consistent and reproducible. Reliability is a necessary but insufficient condition for validity.
Response anchors – The named points along a set of answer options (e.g. not at all important, slightly important, moderately important, quite important and extremely important).
Response process validity – Evidence of validity obtained from an analysis of how respondents interpret the meaning of a survey scale’s specific survey items.
Retrospective probing – A verbal probing technique wherein the interviewer administers the probe questions after the respondent has completed the entire survey (or a portion of the survey).
Scale – Two or more items intended to measure a construct.
Think-aloud interviewing – A cognitive interviewing technique wherein survey respondents are asked to actively verbalize their thoughts as they attempt to answer the evaluated survey items.
Validity – The degree to which evidence and theory support the proposed interpretations of an instrument’s scores.
Validity argument – The process of accumulating evidence to provide a sound scientific basis for the proposed uses of an instrument’s scores.
Verbal probing – A cognitive interviewing technique wherein the interviewer administers a series of probe questions specifically designed to elicit detailed information beyond that normally provided by respondents.
Notes on contributors
ANTHONY R. ARTINO, Jr., PhD, is an Associate Professor of Preventive Medicine and Biometrics. He is the Principal Investigator on several funded research projects and co-directs the Long-Term Career Outcome Study (LTCOS) of Uniformed Services University (USU) trainees. His research focuses on understanding the role of academic motivation, emotion and self-regulation in a variety of settings. He earned his PhD in educational psychology from the University of Connecticut.
JEFFREY S. LA ROCHELLE, MD, MPH, is an Associate Program Director for the Internal Medicine residency at Walter Reed National Military Medical Center and is the Director of Integrated Clinical Skills at USU where he is an Associate Professor of Medicine. His research focuses on the application of theory-based educational methods and assessments and the development of observed structured clinical examinations (OSCE). He earned his MD and MPH from USU.
KENT J. DEZEE, MD, MPH, is the General Medicine Fellowship Director and an Associate Professor of Medicine at USU. His research focuses on understanding the predictors of medical student success in medical school, residency training and beyond. He earned his MD from The Ohio State University and his MPH from USU.
HUNTER GEHLBACH, PhD, is an Associate Professor at Harvard’s Graduate School of Education. He teaches a course on the construction of survey scales, and his research includes experimental work on how to design better scales as well as scale development projects to develop better measures of parents’ and students’ perceptions of schools. In addition, he has a substantive interest in bringing social psychological principles to bear on educational problems. He earned his PhD from Stanford’s Psychological Studies in Education program.
Declaration of interest: Several of the authors are military service members. Title 17 U.S.C. 105 provides that “Copyright protection under this title is not available for any work of the United States Government”. Title 17 U.S.C. 101 defines a United States Government work as a work prepared by a military service member or employee of the United States Government as part of that person’s official duties.
The views expressed in this article are those of the authors and do not necessarily reflect the official views of the Uniformed Services University of the Health Sciences, the U.S. Navy, the U.S. Army, the U.S. Air Force, or the Department of Defense.
Portions of this AMEE Guide were previously published in the Journal of Graduate Medical Education and Academic Medicine and are used with the express permission of the publishers (Gehlbach et al. Citation2010; Artino et al. Citation2011; Artino & Gehlbach Citation2012; Rickards et al. Citation2012; Magee et al. 2013; Willis & Artino Citation2013).
References
- American Educational Research Association (AERA), American Psychological Association (APA) & National Council on Measurement in Education (NCME). 1999. Standards for education and psychological testing. Washington, DC: American Educational Research Association
- Artino AR, Gehlbach H, Durning SJ. 2011. AM last page: Avoiding five common pitfalls of survey design. Acad Med 86:1327
- Artino AR, Gehlbach H. 2012. AM last page: Avoiding four visual-design pitfalls in survey development. Acad Med 87:1452
- Beck CT, Gable RK. 2001. Ensuring content validity: An illustration of the process. J Nurs Meas 9:201–215
- Christian LM, Parsons NL, Dillman DA. 2009. Designing scalar questions for web surveys. Sociol Method Res 37:393–425
- Colliver JA, Conlee MJ, Verhulst SJ, Dorsey JK. 2010. Reports of the decline of empathy during medical education are greatly exaggerated: A reexamination of the research. Acad Med 85:588–593
- Cook DA, Beckman TJ. 2006. Current concepts in validity and reliability for psychometric instruments: Theory and application. Am J Med 119:166.e7–166.e16
- DeVellis RF. 2003. Scale development: Theory and applications. 2nd ed. Newbury Park, CA: Sage
- Dillman D, Smyth J, Christian L. 2009. Internet, mail, and mixed-mode surveys: The tailored design method. 3rd ed. Hoboken, NJ: Wiley
- Drennan J. 2003. Cognitive interviewing: Verbal data in the design and pretesting of questionnaires. J Adv Nurs 42(1):57–63
- Fabrigar LR, Wegener DT. 2012. Exploratory factor analysis. New York: Oxford University Press
- Fowler FJ. 2009. Survey research methods. 4th ed. Thousand Oaks, CA: Sage
- Gehlbach H, Artino AR, Durning S. 2010. AM last page: Survey development guidance for medical education researchers. Acad Med 85:925
- Gehlbach H, Brinkworth ME. 2011. Measure twice, cut down error: A process for enhancing the validity of survey scales. Rev Gen Psychol 15:380–387
- Kane MT. 2006. Validation in educational measurement. 4th ed. Westport, CT: American Council on Education/Praeger
- Karabenick SA, Woolley ME, Friedel JM, Ammon BV, Blazevski J, Bonney CR, De Groot E, Gilbert MC, Musu L, Kempler TM, Kelly KL. 2007. Cognitive processing of self-report items in educational research: Do they think what we mean? Educ Psychol 42(3):139–151
- Krosnick JA. 1999. Survey research. Annu Rev Psychol 50:537–567
- Magee C, Byars L, Rickards G, Artino AR. 2013. Tracing the steps of survey design: A graduate medical education research example. J Grad Med Educ 5(1):1–5
- McCoach DB, Gable RK, Madura JP. 2013. Instrument development in the affective domain: School and corporate applications. 3rd ed. New York: Springer
- McIver JP, Carmines EG. 1981. Unidimensional scaling. Beverly Hills, CA: Sage
- McKenzie JF, Wood ML, Kotecki JE, Clark JK, Brey RA. 1999. Establishing content validity: Using qualitative and quantitative steps. Am J Health Behav 23(4):311–318
- Napoles-Springer AM, Olsson-Santoyo J, O’Brien H, Stewart AL. 2006. Using cognitive interviews to develop surveys in diverse populations. Med Care 44(11):s21–s30
- Pett MA, Lackey NR, Sullivan JJ. 2003. Making sense of factor analysis: The use of factor analysis for instrument development in health care research. Thousand Oaks, CA: Sage Publications
- Polit DF, Beck CT. 2004. Nursing research: Principles and methods. 7th ed. Philadelphia: Lippincott, Williams, & Wilkins
- Polit DF, Beck CT. 2006. The content validity index: Are you sure you know what’s being reported? Critique and recommendations. Res Nurs Health 29:489–497
- Rickards G, Magee C, Artino AR. 2012. You can’t fix by analysis what you’ve spoiled by design: developing survey instruments and collecting validity evidence. J Grad Med Educ 4(4):407–410
- Rubio DM, Berg-Weger M, Tebb SS, Lee ES, Rauch S. 2003. Objectifying content validity: Conducting a content validity study in social work research. Soc Work Res 27(2):94–104
- Schmitt N. 1996. Uses and abuses of coefficient alpha. Psychol Assess 8:350–353
- Schwarz N. 1999. Self-reports: How the questions shape the answers. Am Psychol 54:93–105
- Sullivan G. 2011. A primer on the validity of assessment instruments. J Grad Med Educ 3(2):119–120
- Sullivan GM, Artino AR. 2013. Analyzing and interpreting data from Likert-type scales. J Grad Med Educ 5(4):541–542
- Tourangeau R, Rips LJ, Rasinski KA. 2000. The psychology of survey response. New York: Cambridge University Press
- Waltz CF, Strickland OL, Lenz ER. 2005. Measurement in nursing and health research. 3rd ed. New York: Springer Publishing Co
- Watt T, Rasmussen AK, Groenvold M, Bjorner JB, Watt SH, Bonnema SJ, Hegedus L, Feldt-Rasmussen U. 2008. Improving a newly developed patient-reported outcome for thyroid patients, using cognitive interviewing. Quality of Life Research 17:1009–1017
- Weng LJ. 2004. Impact of the number of response categories and anchor labels on coefficient alpha and test-retest reliability. Educ Psychol Meas 64:956–972
- Willis GB, Artino AR. 2013. What do our respondents think we’re asking? Using cognitive interviewing to improve medical education surveys. J Grad Med Educ 5(3):353–356
- Willis GB. 2005. Cognitive interviewing: A tool for improving questionnaire design. Thousand Oaks, CA: Sage Publications