Abstract
A four-feature 3D-pharmacophore model was built from a set of 24 compounds whose activities were reported against the V1/S strain of the Plasmodium falciparum dihydrofolate reductase (PfDHFR) enzyme. This is an enzyme harboring Asn51Ile + Cys59Arg + Ser108Asn + Ile164Leu mutations. The HipHop module of the Catalyst program was used to generate the model. Selection of the best model among the 10 hypotheses generated by HipHop was carried out based on rank and best-fit values or alignments of the training set compounds onto a particular hypothesis. The best model (hypo1) consisted of two H-bond donors, one hydrophobic aromatic, and one hydrophobic aliphatic features. Hypo1 was used as a query to virtually screen Maybridge2004 and NCI2000 databases. The hits obtained from the search were subsequently subjected to FlexX and Glide docking studies. Based on the binding scores and interactions in the active site of quadruple-mutant PfDHFR, a set of nine hits were identified as potential inhibitors.
Introduction
Plasmodium falciparum dihydrofolate reductase (PfDHFR) enzyme is one of the several targets in the treatment of malariaCitation1–5. This enzyme catalyzes the nicotinamide adenine nucleotide phosphate (NADPH) dependent reduction of dihydrofolate (DHF) to tetrahydrofolate (THF), which is essential for DNA synthesis in the parasitic cell. It has been recognized as a specific target for common antifolate-based antimalarial drugs: pyrimethamine (1) and cycloguanil (2) (). These drugs competitively inhibit DNA synthesis, and ultimately lead to parasitic death. However, due to the accumulation of genetic mutationsCitation6–11 at one or more amino acid residues 16, 51, 59, 108, and 164, the parasite has become highly resistant to these antifolate drugs. Thus, the clinical uses of these drugs have been compromised in many parts of the world.
Steric clashes between a particular inhibitor and mutated amino acid residues result in the displacement of a drug from its optimal orientation in its interaction with residue Asp54, which is crucial for inhibitor binding, with consequent loss of inhibitory activity. For instance, in the parasite harboring Ala16Val + Ser108Thr mutation, one of the methyl groups at the 6-position of 2 experiences a severe steric interaction with Val16. This results in the development of resistance of the parasite to 2. On the other hand, in the case of a multiple-mutant parasite, steric clashes of the para-Cl groups of both 1 and 2 with Asn108 (mutated from Ser108) result in the loss of inhibitory activities of these drugs or increase the chance of the parasite to develop cross-resistanceCitation7. These observations led to the conception of steric constraint hypothesis. According to this hypothesis, (i) changing the position of the para-Cl group to the meta position and (ii) the use of compounds which are more flexible than 1 and 2 could avoid steric clashes, and subsequently result in agents that effectively inhibit mutant PfDHFRsCitation7,Citation12–18. WR99210 (3) is one of the typical examples of compounds designed to avoid steric clash with Asn108 of the mutant PfDHFR enzyme (). Inhibitory activity tests indicated that this compound has similar activities against wild-type and multiple-mutant parasites carrying the Asn51Ile + Cys59Arg + Ser108Asn + Ile164Leu mutated DHFR enzyme. However, because of toxicity problems, this compound could not advance into clinical stagesCitation19,Citation20. However, it is still used as a model compound for designing competitive PfDHFR inhibitors of flexible nature.
Virtual screening is a computer-based strategy used to identify new potential lead compounds from large databases of compounds that are subsequently synthesized and tested for their biological activitiesCitation21–23. It has also been employed in the discovery of PfDHFR enzyme inhibitorsCitation7,Citation24. Rastelli et al.Citation25 employed virtual screening, which led to the identification of 12 new leads whose chemical structures are different from those of classical antifolates. Enzyme assay and inhibition studies indicated that the compounds are generally more active toward mutant enzyme than wild-type PfDHFR enzyme. Recently, Dasgupta et al.Citation26 carried out high-throughput in silico screening of a database with consequent in vitro enzymatic assay and cellular culture studies. They identified three novel compounds (RJF001302, RJF00670, and RJF00719) of biguanide analogs. The compounds were found to be active against both wild-type and quadruple-mutant PfDHFRs. In spite of the accumulation of a wealth of information and several efforts, no successful drug has come on to the market to combat malaria. Thus, there is always an urgent and unmet need for new drugs to cure malaria.
In this article, we present some hits obtained from a database search. Catalyst/HipHop software was used to generate the 3D-pharmacophore model from a set of compounds whose antimalarial activities are reported in literatureCitation27. HipHop, also known as a common-feature hypothesis, is used as a method for 3D-pharmacophore model generation in a training set of compounds having high structural homology and a narrow activity range. In this method, no activity data are required. This is because only chemical features common to all training set molecules are taken into account for model generationCitation28,Citation29. A set of nine hits were identified from our screening. Selection of the hits was based on their (i) best-fit values, (ii) binding scores, (iii) binding modes, and (iv) interactions with important amino acid residues in the active site such as Asp54, Leu164, Asn108, and Ile14, which are known to be essential for inhibitory activities of antifolate-based antimalarial drugs. Two docking programs, namely, FlexXCitation30,Citation31 and GlideCitation32,Citation33, were used to carry out calculations on the hits obtained from the database search.
Materials and methods
Hypothesis generation and validation
A data set of 24 compounds for which in vitro inhibitory activities against the multiple-drug esistant (V1/S strain) PfDHFR enzyme reported in the literature were usedCitation27. The chemical structures of the molecules were constructed using the 2D/3D-editor sketcher of Catalyst, and standard 3D-structures were generated and minimized to the nearest local minimum using the molecular mechanics CHARMm force field implemented in the Catalyst programCitation34. In order to represent flexibility, a collection of conformational models were generated for each compound. These models were generated automatically, starting from the local minimized structures, using a “polling” algorithmCitation35,Citation36. The “best conformer generation” option of the Catalyst software was employed. The default value of 255 was used for the maximum number of conformer generations per molecule. All the conformers within a range of 20 kcal/mol, with respect to the global minimum, were employed to build a set of pharmacophore hypotheses. Pharmacophore model generation was carried out using the HipHop module of Accelrys Catalyst software (version 4.10)Citation34. The training set molecules, with their associated conformational models, were rearranged into a spreadsheet and subsequently submitted to Catalyst hypothesis generation using the HipHop module. HipHop accommodates hypotheses with up to 10 different features which are necessary for biological activities of compounds, and are distributed within a three-dimensional (3D) space. Chemical features that are surface-accessible and common in hypothesis generation include hydrogen bond donor, hydrogen bond acceptor, ring aromatic, hydrogen bond acceptor lipid, hydrophobic, hydrophobic aromatic, hydrophobic aliphatic, negative ionizable, positive ionizable, negative charge, and positive chargeCitation37. Different hypotheses were generated by varying the default values of the control parameters and altering the feature selection in order to build the best 3D-pharmacophore model. The hypothesis used in this study was obtained by setting three parameters: misses, feature misses, and complete misses to 3, 2, and 2, respectively. The Principal value of 2 and MaxOmitFeat value of 0 were assigned to the most active compounds (3 and 17). This was to allow the two compounds to map all the features in the hypotheses. For the rest of the compounds, the Principal value of 1 and MaxOmitFeat value of 1 were assigned. Details of these control parameters are given in the Catalyst users’ manualCitation37. The selected features were hydrogen bond donor, positive ionizable, hydrophobic aromatic, ring aromatic, and aliphatic aromatic.
The validity of the model was evaluated based on (i) the presence of important chemical features required for interaction with key amino acid residues (Asp54, Ile14, Leu164) in the active site of the PfDHFR enzyme and (ii) the best-fit values of the training set molecules and/or matching of their chemical features with those of the model.
Database search
The 3D-pharmacophore model (hypo1) was used as a query to search hits from the Maybridge2004 database (59,652 compounds) and NCI2000 database (238,819 compounds). The “best and flexible database search” option of the Catalyst programCitation34 was employed to perform the virtual screening. Primary filters such as Lipinski’s rule-of-fiveCitation38 and restricting the number of rotatable bondsCitation39,Citation40 to ≤7 were applied to reduce the data set. Further screening of the hits was carried out using two docking algorithms,FlexXCitation30,Citation31 and GlideCitation32,Citation33.
Molecular docking studies
The X-ray crystal structure of the quadruple-mutant PfDHFR enzyme (1J3K:pdb)Citation11 was obtained from the RCSB Protein Data Bank and was used in order to model the protein structure in this study. It contains 3 bound into the active site in the presence of NADPH. FlexX- and Glide-based docking programs were used to carry out screening of the hits obtained from the database search. FlexX is a fast, flexible docking method that uses an incremental construction algorithm to place ligands into an active siteCitation30,Citation31. During preparation of the receptor description file (rdf) for FlexX docking, a radius of 6.5Å around the bound ligand was used to define the active siteCitation41. The formal charges were assigned to the molecules before submission for docking calculation. Default parameters were used, and 30 solutions were generated for each compound. For the purpose of prioritization of hits during selection, G-scoreCitation42, PMF-scoreCitation43, D-scoreCitation44, and ChemScoreCitation45 were also estimated.
Another method used for the molecular docking study was Glide. Glide (Grid-based Ligand Docking with Energetics) uses a series of hierarchical filters to search for possible locations in the active site region of the receptorCitation32,Citation33. The properties of a receptor/active site region are represented by a grid that has different sets of fields that provide progressively more accurate scoring of the ligand pose. It uses a Glide score (Gscore) for predicting binding affinity and rank ordering of ligands in the database screen. Another scoring function, which is much better than G-score in selecting the correct pose, and is used along with Gscore, is Emodel. For details of the methodology, refer to the original literatureCitation32,Citation33. Glide v4.5 was used for the calculationsCitation46. During protein preparation for Glide docking in this study, only chain A and cofactor NDP610 of PfDHFR-TS (thymidylate synthase) were retained. All water molecules and the rest of the chains (B, C, and D) were removed from the complex, and the protein was minimized using the “protein preparation wizard” and default parameters. Partial atomic charges were assigned according to the OPLS-AA force field. A radius of 15 Å was selected for the active site cavity during receptor grid generationCitation46. Molecules were drawn in SYBYL7.1, and were imported to the Glide window and subsequently converted into Maestro format using the LigPrep module of Glide. The output was set to give 10 docking poses/ligand, whereas default values were used for other parameters. The flexible docking option and XP mode were used in all calculations.
Results and discussion
Pharmacophore generation
The pharmacophore model was built using a set of 24 compounds (with diverse chemical structures) and the Catalyst program. The data set of compounds consisted of derivatives of 2,4-diaminoquinazoline, 2,4-diamino-5,6,7,8-teterahydroquinazoline, 2,4-diamonopteridine, and also 3 (). The in vitro inhibitory activities of these compounds against the multiple-drug resistant (V1/S strain) PfDHFR enzyme were carried out using the same methodCitation27. The compounds with their associated conformational models were submitted to the Catalyst hypothesis generation (HipHop) run.
Figure 2. Chemical structures of the training set compounds used for pharmacophore model generation.
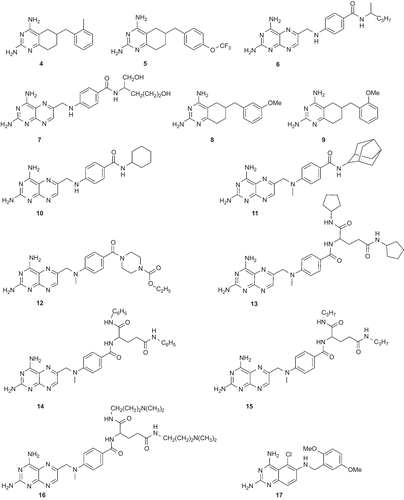
In order to generate the best model, several HipHop runs were carried out by varying the control parameters (data not shown). Among those several runs, the selected HipHop run was obtained by setting three parameters: misses, feature misses, and complete misses to 3, 2, and 2, respectively. The Principal value of 2 and MaxOmitFeat value of 0 were assigned to the most active compounds (3 and 17). For the rest of the compounds, the Principal value of 1 and MaxOmitFeat value of 1 were assignedCitation37. The features selected for this run were hydrogen bond donor (D), positive ionizable (PI), hydrophobic aromatic (Z), ring aromatic (R), and hydrophobic aliphatic (H). This HipHop run resulted in 10 hypotheses (hypo1–10). Their ranking scores ranged from 241.11 to 255.74 kcal/mol (). All of them were four-feature hypotheses. Hypo1–4, 6, 9, and 10 consisted of Z, H, and two Ds whereas hypo5, 7, and 8 each consisted of R, H, and two Ds ().
Table 1. Summary of the hypotheses generated by a Catalyst/HipHop run.
As given in , the first four hypotheses (hypo1 to hypo4) had the same molecular features and close ranking scores. Analysis of best-fit values of the training set compounds against these hypotheses was carried out to choose the best model. The calculated best-fit values indicated hypo1 to be the best model (). Each of the compounds in the training set showed a good best-fit value with respect to this model. Thus, the model was chosen as a query for the virtual screening in order to identify structures that matched its functional and spatial constraints. shows the 3D mappings of 4, 8, 9, 17, 18, 19, and WR99210 (3) onto hypo1.
Table 2. pIC50, number of conformers, best-fit values, and ΔE values of the training set compounds.
Validation of hypo1 and virtual screening
Before performing the actual virtual screening, the model was validated based on (i) the presence of chemical features required for interactions with key amino acid residues (Ile14, Asp54, Leu164) in the active site of PfDHFR. The model had four features (one H, one Z, and two Ds). This observation is consistent with that of known antifolate PfDHFR inhibitors (e.g. 3)Citation6,Citation7,Citation11,Citation25. In this inhibitor, it has been observed that two hydrogen bond donors interact with Asp54 and backbone amino acids (Ile14 or/and Leu164). On the other hand, hydrophobic features interact with amino acid residues such as Met55, Ser111, and Pro113 near the opening of the active site via hydrophobic interactions. For instance, as shown in , hydrogen bond donor features of hypo1 mapped to 2-amino and 4-amino groups. The hydrophobic aromatic feature mapped on the phenyl side chain, whereas the hydrophobic aliphatic feature of the model mapped on the Cl group. Moreover, the conformation of 3, which was extracted from the X-ray crystallographic structure of the quadruple-mutant PfDHFR enzyme, was also found to perfectly overlap onto hypo1 (data not given). Similarly, the features of hypo1 mapped onto the appropriate chemical features of other active compounds (4, 8, 9, 17, and 18) as illustrated in , and (ii) the best-fit values of the training set molecules obtained by mapping onto hypo1 were generally higher than the corresponding values for other hypotheses (). Thus, hypo1 was selected and subsequently used as a query for database searching. The Maybridge2004 and NCI2000 databases were searched using the “best and flexible database search” option of the Catalyst program. The virtual screening resulted in 267 and 255 hits from Maybridge2004 and NCI2000 databases, respectively. To reduce the number of molecules for further analysis, several filtering criteria were used. First, Lipinski’s rule-of-fiveCitation31 was used. According to this rule, any drug-like molecule should be characterized by molecular weight <500, calculated octanol–water partition coefficient (CLogP) <5, number of hydrogen bond donors (HBDs) (OH + NH groups) <5, and number of H-bond acceptors (HBAs) (O + N atoms) <10. Thus, hits with molecular weight >500, number of HBDs >5, and number of HBAs >10 were eliminated from further analysis. Second, to avoid too flexible compounds, those hits having more than seven rotatable bondsCitation39,Citation40 were also eliminated. The remaining 191 hits were subjected to docking into the active site of quadruple-mutant PfDHFR using FlexX and Glide docking programs in order to investigate their binding modes and interactions. To verify the reproducibility of the docking calculations, the bound ligand (3) was extracted from the complex and submitted for one-ligand run calculation. This reproduced top scoring conformations of 3 falling within RMS (root mean square) values of 0.96 Å and 0.489 Å from the bound X-ray conformation for FlexX and Glide docking, respectively. The binding modes of the hits were ranked according to FlexX score and Glide score values. In the case of FlexX docking, other scoring functions such as G-score, PMF-score, D-score, and C-score were also used for further refining of the hits. The hits with better or comparative scores were retained for further analysis. The Catalyst “best-fit” values were calculated for each retained hit using hypo1.
Those hits with “best-fit” value <1.5 were rejected. Based on visual inspection of the binding modes of the hits from both FlexX and Glide docking calculations, together with binding scores and “best-fit” values, a set of nine hits were identified, which belonged to different classes (). The chemical structures of the identified hits are given in , whereas the mappings of representative hits to hypo1 are given in .
Table 3. Docking scores of hits identified from the virtual screening study.
Binding modes (interactions) of the identified hits
As described in previous sections, the identified hits show all the necessary interactions in the active site which are required by antifolate PfDHFR inhibitors for effective inhibition of this enzymeCitation6,Citation7,Citation11,Citation18,Citation25. Their hydrogen bond donor features interact with Asp54 and Ile14 or/and Leu164, whereas the hydrophobic portions interact with amino acid residues Met55, Ser111, Pro113, and other amino acids via hydrophobic interactions. Some of the hits also show H-bond interaction with Asn108. Their binding modes also indicated that there is no unfavorable steric clash with Asn108 (mutated from Ser108), which is known to be responsible for resistance. The detailed binding interactions of some of the hits (NCI0004356, GK-03628, NCI0029588, CD00706, NCI0037722, and NCI0014710) are given in the following sections. Unless and otherwise mentioned specifically, discussion is based on the Glide docking result.
Binding mode of NCI0004356
shows the binding mode of NCI0004356 within the active site of the quadruple-mutant PfDHFR enzyme. As illustrated in this binding model, one of the OH substituents of the aromatic ring forms an H-bond with Asp54 with a bond length value of 2.092 Å. The OH group of the aliphatic side chain interacts with Asn108 and Leu164. This OH group donates an H-bond to the carbonyl group of Leu164, and it accepts an H-bond from the amino group of Asn108. The corresponding H-bond lengths are 2.896 and 1.876 Å, respectively. The long side chain of this hit interacts hydrophobically with Met55, Ser111, and Pro113.
Binding mode of GK-03628
The binding mode of GK-03628 within the active site of the quadruple-mutant PfDHFR enzyme is given in . It interacts with Asp54, Ile14, and Asn108. The OH group interacts with Asp54 (1.949 Å), whereas its amino group interacts with Ile14 (2.897 Å). The S atom of the linker unit accepts an H-bond from the amino group of Asn108 (2.313 Å) (). Visual inspection also showed that its side chain interacts with amino acids (Met55, Ser111, and Pro113) in the hydrophobic region of the active site.
Binding mode of NCI0029588
The binding mode of NCI0029588 within the active site of the quadruple-mutant PfDHFR enzyme is given in . Similar to that of the bound ligand (3), NCI0029588 interacts with Asp54 as well as backbone amino acid residues (Ile14 and Leu164). The H-bond length between the OH group of NCI0029588 and oxygen atom of the carboxylic group of Asp54 is 2.128 Å. The amino group forms H-bonds with Ile14 and Leu164. The corresponding H-bond length values are 1.709 and 1.929 Å, respectively ().
Binding mode of CD00706
The binding mode of CD00706 within the active site of the quadruple-mutant PfDHFR is given in . The OH group interacts with Asp54 via H-bonding (1.714 Å) and one of the NH groups of the thiourea linker unit forms an H-bond with Leu164 (2.260 Å). The second aromatic ring and its OMe substituent interact hydrophobically with Met55, Ser111, and Pro113.
Binding mode of NCI0037722
shows the binding mode of NCI0037722 within the active site of the quadruple-mutant PfDHFR. As illustrated in the model, it forms all the key H-bond interactions in the active site. The Me substituent bearing amino group interacts with Asp54 via H-bonding (1.922 Å), whereas the 4-amino group forms an H-bond with the backbone amino acids Ile14 (2.229 Å) and Leu164 (2.225 Å). As in the case of the hits discussed above, the side chain of NCI0037722 is oriented toward the hydrophobic region of the active site. Thus, it is expected to interact with amino acid residues in this region via hydrophobic interaction ().
Binding mode of NCI0014710
shows the binding mode of NCI0014710 within the active site of the quadruple-mutant PfDHFR. Its two amino groups form a bidentate H-bond interaction with Asp54 (1.616 and 1.867 Å). The N atom of the ring system accepts an H-bond from the NH2 group of Asn108 (2.291 Å). The ring system and its OMe substituents are also expected to interact hydrophobically with amino acid residues such as Ser111, Pro113, and Met55 ().
Conclusions
Pharmacophore modeling is a powerful method in drug design and discovery since it enables medicinal chemists to rapidly identify new potential drugs. In this study we employed Catalyst/HipHop software to generate a hypothesis having four features: two hydrogen bond donors, one aromatic hydrophobic, and one hydrophobic aliphatic. Use of this hypothesis as a query for searching Maybridge2004 and NCI2000 databases helped us to identify a set of nine potential inhibitors of the quadruple-mutant PfDHFR enzyme. The hits were selected based on their docking scores, binding orientations, and interactions with key amino acid residues in the active site (Asp54, Ile14, and Leu164) as well as their interactions in the hydrophobic region of the active site. Because of the flexible nature of their side chains, there are no potential steric clashes with Asn108, which is known to be responsible for resistance of the mutant parasite to antimalarial drugs 1 and 2Citation6,Citation7,Citation10–13,Citation18,Citation25. The hits also fulfill all the properties required by drug-like moleculesCitation47,Citation48. To the best of our knowledge, there are no reports on antimalarial activities of these hits. Thus, we encourage those research teams working in the area of antimalarial drug discovery to carry out activity tests of these hits so that compounds with high activity may be identified to overcome resistance.
Declaration of interest
One of the authors (L.A.) thanks the Government of the Federal Democratic Republic of Ethiopia for financial support.
References
- Kompis IM, Islam K, Then RL. DNA and RNA synthesis: antifolates. Chem Rev 2005;105:593–620.
- Da Cuhan EF, Ramlho TC, Maia ER, de Alenncastro RB. The search for new DHFR inhibitors: a review of patents, January 2001–February 2005. Expert Opin Ther Patents 2005;15:1–20.
- Nzila A. Inhibitors of de novo folate enzymes in P. falciparum. Drug Discov Today 2006;11:939–44.
- Anderson AC, Wright DL. Targeting DHFR in parasitic protozoa. Drug Discov Today 2005;10:121–8.
- Schenell JR, Dyson HJ, Wright PE. Structure, dynamics, and catalytic function of dihydrofolate reductase. Annu Rev Biophys Biomol Struct 2004;33:119–40.
- Lemeke T, Christensen IT, Jorgensen FS. Towards understanding of drug resistance in malaria: three-dimensional structure of P. falciparum DHFR by homology building. Bioorg Med Chem 1999;7:1003–11.
- Rastelli G, Sirawaraporn W, Sompornpisut P, Vilaivan T, Kamchonwongpaisan S, Quarrell R, et al. Interaction of pyrimethamine, cycloguanil, WR99210 and their analogues with P. falciparum dihydrofolate reductase: structural basis of antifolate. Bioorg Med Chem 2000;8:1117–28.
- Delfino RT, Santos-Filho OA, Figueroa-Villar JD. Molecular modeling of wild-type and antifolate resistant mutant P. falciparum DHFR. Biophys Chem 2002;98:287–300.
- Santos-Filho OA, de Alencastrob RD, Figueroa-Villar JD. Homology modeling of wild-type and pyrimethamine/cycloguanil-cross-resistant mutant type P. falciparum DHFR: a model for antimalarial chemotherapy resistance. Biophys Chem 2001;91:305–17.
- Sirawaraporn W, Sathitkul T, Sirawaraporn R, Yuthavong Y, Santi DV. Antifolate-resistant mutants of P. falciparum dihydrofolate reductase. Proc Natl Acad Sci USA 1997;94:1124–9.
- Yuvaniyama J, Chitnumsub P, Kamchonwongpaisan S, Vanichatanankul J, Sirawaraporn W, Taylor P, et al. Insights into antifolate resistance from malarial DHFR-TS structures. Nat Struct Biol 2003;10:357–65.
- Warhurst DV. Antimalarial drug discovery: development of inhibitors of dihydrofolate reductase active in drug resistance. Drug Discov Today 1998;3:538–46.
- Warhurst DV. Resistance to antifolate in P. falciparum DHFR, the causative agent of tropical malaria. Sci Prog 2002;85:89–111.
- McKie JH, Douglas KT, Chan C, Roser SA, Yates R, Read M, et al. Rational drug design approach for overcoming drug resistance: application to pyrimethamine resistance in malaria. J Med Chem 1998;41:1367–70.
- Sardarian A, Douglas KT, Read M, Sims PFG., Hyde JE, Chitnumsub P, et al. Pyrimethamine analogs as strong inhibitors of double and quadruple mutants of dihydrofolate reductase in human malaria. J Biol Chem 2003;1:960–4.
- Kamchonwongpaisan S, Quarrell R, Charoensetakul N, Ponsinet R, Vilaivan T, Vanichtanankul J, et al. Inhibitors of multiple mutants of P. falciparum dihydrofolate reductase and their antimalarial activities. J Med Chem 2004;47:673–80.
- Yuthavong Y, Vilainvan T, Chareonsethakul N, Kamchonwongpaisan S, Sirawaraporn W, Quarrell R, et al. Development of a lead inhibitor for the A16V+S108T mutant of dihydrofolate reductase from the cycloguanil-resistant strain (T9/94) of P. falciparum. J Med Chem 2000;43:2738–44.
- Sichaiwat C, Intaraudom C, Kamchonwongpaisan S, Vanichtanankul J, Thebtaranonth Y, Yuthavong Y. Target guided synthesis of 5-benzyl-2,4-diamonopyrimidines: their antimalarial activities and binding affinities to wild type and mutant dihydrofolate reductases from P. falciparum. J Med Chem 2004;47:345–54.
- Hunt SY, Detering C, Varani G, Jacobus DP, Schiehser GA, Shieh HM, et al. Identification of the optimal third generation antifolate against P. falciparum and P. vivax. Mol Biochem Parasitol 2005;144:198–205.
- Japrung D, Leartsakulpanich U, Chusacultanachai S, Yuthavong Y. Conflicting requirements of P. falciparum dihydrofolate reductase mutations conferring resistance to pyrimethamine-WR99210 combination. Antimicrob Agents Chemother 2007;51:4356–60.
- Klebe G. Foundation review: virtual ligand screening: strategies, perspectives and limitations. Drug Discov Today 2006;11:580–94.
- Muegge I, Oloff S. Advances in virtual screening. Drug Discov Today Tech 2006;3:405–11.
- Alvarez J, Shoichet B. Virtual Screening in Drug Design. Boca Raton, FL: Taylor & Francis, 2005.
- Toyoda T, Brobey RK, Sano G, Horii T, Tomioka N, Itai A. Lead discovery of inhibitors of the dihydrofolate reductase domain of P. falciparum dihydrofolate reductase-thymidylate synthase. Biochem Biophys Res Commun 1997;235:515–19.
- Rastelli G, Pacchioni S, Sirawaraporn W, Sirawaraporn R, Parenti MD, Ferrari AM. Docking and database screening reveal new classes of P. falciparum dihydrofolate reductase inhibitors. J Med Chem 2003;46:2834–45.
- Dasgupta T, Chitnumsub P, Kamchonwongpaisan S, Maneeruttanarungroj C, Nichols SE, Lyons TM, et al. Exploiting structural analysis, in silico screening, and serendipity to identify novel inhibitors of drug-resistant Falciparum malaria. ACS Chem Biol 2009;4:29–40.
- Ommeh S, Nduati E, Mberu E, Kokwaro G, Marsh K, Rosowsky A, et al. In vitro activities of 2,4-diaminoquinazoline and 2,4-diaminopteridine derivatives against P. falciparum. Antimicrob Agents Chemother 2004;48:3711–14.
- Guner OF. Pharmacophore: Perception, Development and Use in Drug Design. La Jolla, CA: International University Line, 2000.
- Kurogi Y, Guner OF. Pharmacophore modeling and three-dimensional database searching for drug design using catalyst. Curr Med Chem 2001; 8:1035–55.
- Rarey M, Kramer B, Lengauer T. Multiple automatic base selection: protein-ligand docking based on incremental construction without manual intervention. J Comput Aided Mol Des 1997;11:369–84.
- Rarey M, Kramer B, Lengauer T, Klebe G. A fast flexible docking method using an incremental construction algorithm. J Mol Biol 1996;261:470–89.
- Friesner RA, Banks JL, Murphy RB, Halgren TA, Klicic JJ, Mainz DT, et al. Glide: a new approach for rapid, accurate docking and scoring. 1. Method and assessment of docking accuracy. J Med Chem 2004;47:1739–49.
- Halgren TA, Murphy RB, Friesner RA, Beard HS, Frye LL, Pollard WT, et al. Glide: a new approach for rapid, accurate docking and scoring. 2. Enrichment factors in database screening. J Med Chem 2004;47:1750–9.
- Catalyst, Version 4.10. San Diego, CA: Accelrys Inc., 2005.
- Smellie A, Teig SL, Towbin P. Polling: promoting conformational variation. J Comput Chem 1995;16:171–87.
- Smellie A, Kahn SD, Teig SL. An analysis of conformational coverage 2. Applications of conformational models. J Chem Inf Comput Sci 1995;35:295–304.
- Catalyst manual, available at http://www.accelrys.com.
- Lipinski CA, Lombardo F, Dominy BW, Feeney PJ. Experimental and computational approaches to estimate solubility and permeability in drug discovery and development settings. Adv Drug Deliv Rev 1997;23:3–25.
- Muegge I. Selection criteria for drug-like compounds. Med Res Rev 2003;23:302–21.
- Oprea TI. Property distribution of drug-related chemical databases. J Comput Aided Mol Des 2000;14:251–64.
- SYBYL 7.1. St. Louis, MO: Tripose Inc., 2005.
- Jones G, Willett P, Glen RC, Leach AR, Taylor R. Development and validation of a genetic algorithm for flexible docking. J Mol Biol 1997;267:727–48.
- Muegge I., Martin YC. A general and fast scoring function for protein-ligand interactions: a simplified potential approach. J Med Chem 1999;42:791–804.
- Kuntz ID, Blaney JM, Oatley SJ, Langridge R, Ferrin TE. A geometric approach to macromolecule-ligand interactions. J Mol Biol 1982;161:269–88.
- Eldridge MD, Murray CW, Auton TR, Paolini GV, Mee RP. Empirical scoring functions: I. The development of a fast empirical scoring function to estimate the binding affinity of ligands in receptor complexes. J Comput Aided Mol Des 1997;11:425–45.
- Schrödinger Suite 2007. New York: Schrödinger, LLC, 2007.
- Proudfoot JR. Drugs, leads, and drug-likeness: an analysis of some recently launched drugs. Bioorg Med Chem Lett 2002;12:1647–50.
- Oprea TI, Allu TK, Fara DC, Rad RF, Ostopovici L, Bologa CG. Lead-like, drug-like or “pub-like”: how different are they? J Comput Aided Mol Des 2007;21:113–19.