Abstract
A combination of the following computational methods: (i) molecular docking, (ii) 3-D Quantitative Structure Activity Relationship Comparative Molecular Field Analysis (3D-QSAR CoMFA), (iii) similarity search and (iv) virtual screening using PubChem database was applied to identify new anthranilic acid-based inhibitors of hepatitis C virus (HCV) replication. A number of known inhibitors were initially docked into the “Thumb Pocket 2” allosteric site of the crystal structure of the enzyme HCV RNA-dependent RNA polymerase (NS5B GT1b). Then, the CoMFA fields were generated through a receptor-based alignment of docking poses to build a validated and stable 3D-QSAR CoMFA model. The proposed model can be first utilized to get insight into the molecular features that promote bioactivity, and then within a virtual screening procedure, it can be used to estimate the activity of novel potential bioactive compounds prior to their synthesis and biological tests.
Introduction
Hepatitis C virus (HCV) constitutes a serious worldwide health concern that impacts approximately 180 million people. Approximately, 60–80% of HCV infections evolved in chronic hepatitis usually lead to liver fibrosis, cirrhosis and hepatocellular carcinomaCitation1,Citation2. Hitherto, the current standard of care (SOC), which combines pegylated alpha interferon and the nucleoside analogue ribavirin, has limited efficacy (approximately 50% of the treated patients achieved sustained virologic response, SVR) and may be associated with a number of side effectsCitation3,Citation4.
In order to address this need, new direct-acting antiviral agents (DAAs) are developed that target viral proteins critical for HCV replication. Recently, the NS3/4 A protease has become a target and two DAAs, boceprevir and telaprevir, in combination with the SOC have been approved as treatment for hepatitis CCitation5,Citation6. Although these new triple combination regimens improved SVR rates and shortened duration of therapy to HCV genotype 1-infected patientsCitation7,Citation8, significant side effects, such as a low barrier to resistance, appeared. Up to date, several antiviral compounds including NS5A inhibitors, nucleoside/nucleotide analogues and non-nucleoside inhibitors (NNIs) that target the NS5B polymerase, are in clinical development.
HCV, a member of the Flaviviridae family, is a small, single-stranded, positive-sense RNA virus. Its genome encodes a single polyprotein of 3010 amino acids with four structural proteins located in the amino terminus and six non-structural proteins encoded in the remainder. On the basis of the genomic variability in a small region of the gene that encodes the viral NS5B polymerase, the naturally occurring variants are classified into six major genotypes (genotypes 1–6) and several subtypesCitation9.
NS5B is the RNA-dependent RNA-polymerase (RdRp), which is responsible for the complete copy of the viral RNA genome of HCV. Due to its significant action, NS5B has been assigned extensive structural and biochemical characterizationCitation10,Citation11, and several crystallographic structures of polymerase have been structurally elucidated. It has been shown that the protein adopts a morphology similar to that of a right hand with “fingers”, “palm” and “thumb” subdomains, common to several polymerasesCitation12. The search for inhibitors of HCV NS5B RdRp has led to investigation of nucleoside and nucleotide inhibitors (NIs) and non-nucleoside inhibitors (NNIs).
NIs are modified nucleosides and nucleotides that can be bound at the active site of polymerase to compete with natural substrates, acting as non-obligate chain terminators. Importantly, due to the conservation of the active site, the efficiency of nucleos(t)ide inhibitors is similar across all HCV genotypes and isolatesCitation13. On the other side, NNIs are chemically diverse small molecules that bind to allosteric sites of the enzyme, probably inducing conformation changes in the RdRp, thus hindering the polymerase from functioning effectively and leading to inhibition of the initiation step in RNA replication. Virtual screening (VS) has already proven its value as a useful tool to search for compounds within large chemical libraries, which are most likely to bind to a drug target. This computational tool has been emerged as a reliable, cost-effective and time-saving technique for the drug discovery. VS has already played a significant role in the discovery of several compounds that have either entered clinical trials or even reached the marketCitation14–16.
There are two broad categories of VS techniques: (a) ligand-based virtual screening and (b) structure-based virtual screeningCitation17–22. For the first category, a model of a receptor can be built, exploiting the common information (pharmacophore or descriptor values) of structurally diverse ligands that bind to the receptor. Then this model can be used to retrieve other potentially active molecular scaffolds. For the second category, the protein target is available, and the candidate ligands are docked into the target and scoring functions are applied to estimate the likelihood that the ligand will bind to the target with high affinity. To date, several studies have been reported in the literature with the objective of identifying novel potent HCV inhibitors using different in silico protocols and workflowsCitation23–34.
In this study, a combination of computational methods of (i) molecular docking, (ii) 3-D quantitative structure activity relationship comparative molecular field analysis (3D-QSAR CoMFA), (iii) similarity search and (iv) virtual screening is used to identify new inhibitors of HCV replication. Efforts were carried out to understand the structural characteristics that affect the binding of 53 anthranilic acid-based analogues with HCV NS5B GT1b polymerase serving as receptorCitation35,Citation36. These compounds have been synthesized and tested as inhibitors of HCV replication, and some of them have been co-crystallized with HCV polymerase, too. In our work, all the compounds were docked into the Thumb pocket 2 (TP-2) of enzyme, and the docking pose of each compound was subsequently used in a receptor-based alignment for the generation of the CoMFA fields. A computational workflow was followed in order to build a 3D-QSAR CoMFA model and is graphically depicted in Scheme 1.
Methods
As aforementioned, a computational workflow has been developed to study potent anthranilic acid analogues as inhibitors of HCV replication in order to predict the inhibitory activity of analogues included in PubChem database. First, a dataset of 53 anthranilic acid-based inhibitors was docked into the TP-2 of the HCV NS5B polymerase for calculating the docking score. Then, the docking pose of each inhibitor was used in a receptor-based alignment for generating the CoMFA fields. A 3D-QSAR CoMFA model was subsequently built to accurately estimate the activity and, finally, within a virtual screening framework anthranilic acid-based analogues included in PubChem database were prioritized for screening.
Dataset
For the development of the 3D-QSAR model, the original dataset consisted of 53 anthranilic acid-based inhibitors ()Citation35,Citation36. These analogues were all tested for inhibition of HCV replication in a biochemical assay using a genotype 1b Δ21NS5B proteinCitation35,Citation36. The 3D structures of anthranilic acid-based analogues were built and adjusted using the Maestro 9.3 (New York, NY) molecular builder. All the hydrogen atoms were added, and the molecules were submitted in full structure optimization using the minimization procedure of MacroModel 9.9Citation37. For the minimization, water was selected as solvent and a standard molecular mechanics energy function (OPLS_2005 force fieldCitation38) and the Polak-Ribiere conjugated gradient method (500 iterations with gradient 0.01 kJ/mol Å)Citation3 were used. The minimized structures were subsequently prepared using LigPrep 2.3Citation39, where all the structures were checked to be correct and ionization states were generated.
Table 1. Structures of 53 anthranilic acid-based analogues.
Preparation of the receptor
There are five co-crystal complex structures of anthranilic acid-based inhibitors available in Protein Data Bank (PDB), with codes 4J08, 4JU3, 4JU4, 4JU7 and 4JJS. Since no significant conformational differences were observed on the crystal structures, the crystal structure with the highest X-ray resolution (PDB ID: 4JU3, 2.00 Å X-ray resolution) was chosen for the docking and was prepared using the Protein Preparation Wizard implementation in Schrödinger suite 2012Citation40. The bond orders and disulfide bonds were assigned, all the hydrogen atoms were added and all the water molecules that separated by a distance of more than 5 Å from the allosteric site of TP-2 were deleted. The Epik 2.0 implementation was used to predict ionization and tautomeric states of the ligand het groupsCitation40,Citation41. The hydrogen-bonding network was optimized by reorienting the hydroxyl groups, amide groups of Asn and Gln residues and by selecting appropriate states and orientations of the imidazole ring in His residues. Finally, using the “impref utility” and the OPLS_2005 force fieldCitation42, the hydrogen atom positions were optimized by keeping all the heavy atoms in place.
Molecular docking
The Glide implemented in Schrödinger was utilized for the molecular docking procedureCitation40. Glide has shown a good reproducibility of co-crystal ligand conformation, which has also been recommended in literature for its accuracy in molecular docking and scoringCitation43. The binding region was defined by a 12 Å × 12 Å × 12 Å box centered in the centroid of the crystal ligand, and the option “docking ligands similar in size to the workspace ligand” was chosen. All other parameters were kept as default. The best pose was the output on the basis of the Glide score and the protein–ligand interactions. The extra precision (XP) mode and the XP GScore were used for the docking and scoring, respectively.
CoMFA analysis
In this study, a “protein-based” alignment was used (the conformations of the studied compounds used for the alignment were chosen by docking these compounds in the TP-2 of RdRp). The molecular alignment is the most sensitive factor that has a significant impact on the 3D-QSAR models. Atomic charges for the aligned molecules were calculated using the Gasteiger–Hückel method, which is a combination of two other charge computational methods: the Gasteiger–MarsiliCitation44 method to calculate the σ component of the atomic charge and the HückelCitation45 method to calculate the π component of the atomic charge. The total charge is the sum of the charges calculated by the two methods. The CoMFA fields are generated by creating a grid around the molecule and calculating the steric and electrostatic potentials at each point on the grid using a charged probe atom. The CoMFA calculations were performed using Tripos Advance CoMFACitation46 module in SYBYL 8.0. The steric and electrostatic field energies were calculated using the Lennard-Jones and the Coulomb potentials, respectively, with a 1/r2 distance-dependent dielectric constant in all intersections of regularly spaced (0.2 nm) grid. A spCitation3 carbon atom with radius of 1.53 Å and charge +1.0 was used as a probe to calculate the steric and electrostatic energies between the probe and the molecules using the standard Tripos force fieldCitation47. The truncation for both the steric and electrostatic energies was set to 30 kcal mol−1. This indicates that any steric or electrostatic field value that exceeds this value will be replaced with 30 kcal mol−1, thus makes a plateau of the fields close to the center of any atom. An optimal number of components (ONC) was designated such the cross-validated r2 was maximal, and the standard error of prediction was minimal.
Partial least square analysis
The partial least square (PLS) approach applied in SYBYL 8.0Citation48,Citation49 was used to derive the 3D-QSAR model and linearly correlate the CoMFA fields to the pIC50 values. The CoMFA fields were used as independent variables and the pIC50 values as dependent variables. PLS was performed in two steps. In the first step, the cross-validation analysis was performed using Leave-One-Out (LOO) method, which was applied to determine the value of the cross-validated r2 (q2), the cross-validated standard error of predictions, SPRESS and the ONC. In the LOO method, one compound is removed from the dataset, and the model is derived involving the rest of the molecules. Then using this model, the activity of the omitted molecule can be predictedCitation50. The model with the highest q2 and the lowest Standard Error Prediction (SEP) was driven for further analysis. The cross-validated r2 is calculated by the following equation:
(1)
where Yobs and Ypred indicate observed and predicted activity values, respectively, and
indicates mean activity value. A model is considered acceptable when the value of q2 exceeds 0.5Citation51.
In the second step, using the ONC obtained from cross-validation analysis, a non-cross-validated method was applied and the non-cross-validated R2 (conventional) is calculated according to the following equation:
(2)
In addition, the statistical significance of the model was described by the standard error of estimate (SEE) and the probability value (F-value). To speed up the analysis and reduce noise, a minimum column filter value σ (2.00 kcal mol−1) was used. The application of non-cross-validated method led to the build of CoMFA model.
3D-QSAR model validation
The predictive capability of the 3D-QSAR CoMFA model was evaluated with an external test set using the molecules that have not been taken into account during the process of developing the modelCitation52. The predictive correlation () is calculated using the following equation:
(3)
where Ypred (test) and Ytest indicate predicted and observed activity values of the test set, respectively, and
indicates mean of observed activity values of the training set. For a predictive QSAR model, the value of
should be more than 0.5.
Furthermore, external predictive power of the developed 3D-QSAR model using test set was examined by considering . The formula is given belowCitation53:
(4)
where r2 and
are squared correlation coefficients between the observed and predicted activities of the test set with and without intercept, respectively.
is a new, rigorous and powerful statistical index reported by Roy et al.Citation54. In their study, they demonstrated that even when the value of q2 is high, this is not a sufficient condition to indicate the predictive capability and power of the model. For a significant external model validation, the value of
should be greater than 0.5. The index
can be calculated using a web application accessible from http://aptsoftware.co.in/rmsquare/. The concept of
can also be applied for the training set.
To assess the predictive power of produced 3D-QSAR model, Enalos Model Acceptability Criteria KNIME node was usedCitation55 that includes all proposed tests by Tropsha. Especially, for continuous QSAR, criteria followed in developing activity/property predictors are as follows: (i) correlation coefficient R between the predicted and observed activities; (ii) coefficients of determination (predicted versus observed activities and observed versus predicted activities
for regressions through the origin); (iii) slopes k and k' of regression lines through the origin. The QSAR model is acceptable if the following conditions are satisfied: (i) q2 > 0.5; (ii) R2 > 0.6; (iii)
and 0.85 ≤ k ≤ 1.15 or
< 0.1 and 0.85 ≤ k′ ≤ 1.15; and (iv)
Citation52,Citation55.
Domain of applicability
When proposing a validated model, it is very important to simultaneously define its limits so that a well-defined applicability domain could indicate those predictions that can be considered reliable. When the model is used to screen new compounds, it is important that these structures fall out the domain of applicability of the model and are filtered out as the model cannot generate reliable predictions for these structures. Domain of applicability can be defined using similarity measurements based on the Euclidean distances among all training and test compounds. The distance of a test compound to its nearest neighbor in the training set is compared to a predefined threshold (APD), and the prediction is considered unreliable when the distance is higher than that. APD was calculated based on the following formula:
(5)
Calculation of <d> and σ was performed as follows: first, the average of Euclidean distances between all pairs of training compounds was calculated. Next, the set of distances that were lower than the average was formulated. <d> and σ were finally calculated as the average and standard deviation of all distances are included in this set. Z is an empirical cutoff value and for this work, it was chosen equal to 0.5Citation56–59. Enalos Domain – similarity node that executes the aforementioned procedure is included in our workflow and was used to assess domain of applicability of the proposed modelCitation56–59. For the calculation of the domain of applicability, we have used Enalos Domain KNIME nodes. These nodes have been developed by Novamechanics Ltd. (Nicosia, Cyprus) and are publicly available through the KNIME Community and the company’s websiteCitation60.
Virtual screening
PubChem database has been recently emerged as a very important source of compounds that could be prioritized for screening for a wide range of targets. We have decided to use PubChem database within our in silico framework to virtual screen compounds that could act as HCV inhibitors. For this purpose, we have developed a KNIME workflow to assess the compounds that will be prioritized for virtual screening. First, our purpose was to mine all anthranilic acid-based analogues within PubChem database. To achieve that, we have used Substructure Matcher node included in Indigo cheminformatics toolkit. All these compounds were compared to the most active compound of our original dataset in terms of similarity given by Indigo Fingerprints. It is known that similarity searching refers to the calculation of the similarity coefficients for a given molecule and each compound included within a database. Among the different proposed methodologies, similarity searching using fingerprints is one of the most widely applied methods for ligand-based screening. Fingerprints are representations of the structural features of a molecule given as a series of binary digits (bits) that account for the presence or absence of particular substructures in the molecule. For this study, Fingerprint Similarity node using Tanimoto metric for similarity computation was used to afford the most similar compounds that could be prioritized for screening.
All the retrieved anthranilic acid-based analogues were virtually screened with the ultimate goal of prioritizing the most promising new synthetic targets. The proposed 3D-QSAR model was used to identify the most active compounds that are proposed for further investigation.
Results and discussion
Molecular docking of analogues into the TP-2 of NS5B HCV polymerase
The TP-2 is a narrow hydrophobic cavity near the base of the thumb domain, approximately 35 Å apart from the active site. Sequence analysis showed that the primary structure of this binding site was relatively well conserved among the known HCV genotypesCitation61. The binding site traverses the thumb from front to back of the RdRp “right hand” but it is relatively shallow and narrow (30 Å × 10 Å × 10 Å). It is bounded by three of the α-helices in the thumb subdomain separated by the link between two of these from the fingertip α-helix/site I region that is 15 Å away and is proximate to the C-terminus of the thumb. The mechanism of action for the inhibitors of TP-2 is unknown. It has been suggested that inhibition is due to blocking association with other proteins that are important for replicationCitation61. The TP-2 consists of a hydrophobic pocket with residues M423, W528, L419, Y477 and R422, while hydrogen bonds are favorable. The observed key interaction between the anthranilic acid-based inhibitors and the enzyme is two hydrogen bonds between the carboxylate oxygens and the backbone amide nitrogens of S476 and Y477.
The molecular docking was first applied to anthranilic acid-based and active inhibitor 8 (ID = 23, ), which it has already co-crystallized with the HCV NS5B polymerase and described by Stammers et al.Citation35. In , the superposition of crystal structure conformation and that obtained in the thumb pocket of compound 8 is depicted. Thus, it is obvious that the algorithm Glide used for molecular docking is able to reproduce crystallographic experimental data for structural similar compounds. Afterward, the 53 anthranilic acid-based molecules were docked into the TP-2 allosteric site of HCV polymerase. The docking results expressed in XP GScore are listed in . The lower the value of XP GScore, the more inhibition ability is expected for the compound. A good correlation was found between the docking scores and the IC50 values.
Figure 1. Superposition of two structures; crystal structure is depicted with green and docked compound 8 with orange (RMSD ∼ 0.2).
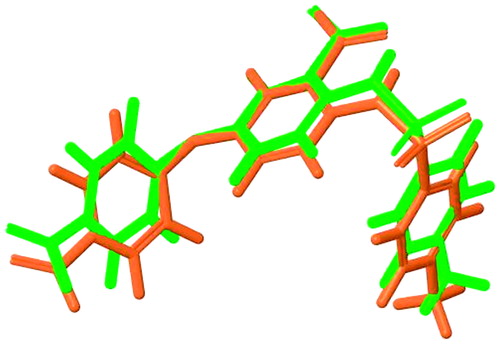
Table 2. The IC50 values, the XP GScore, the pIC50, the predicted pIC50 and the residuals for the anthranilic acid-based analogues.
The molecular docking results of compound 8 of this series are represented in . As aforementioned, the pose that adopted is the same with the co-crystal inhibitor. The right hand side (RHS) of anthranilic acid scaffold (4-methylphenylsulfonamide group) is placed into the deep lipophilic pocket of enzyme and a new hydrogen bonding interaction between the left hand side (LHS) of anthranilic scaffold, and the side chain of R490 is added to the three hydrogen bonds with S476, Y477 and L497 appeared in the X-ray crystallography. No significant differences to the positioning and orientation of the anthranilic acid of other analogues were observed. All anthranilic acid-based derivatives, except from compounds 10a and 18, adopt a binding mode to enable bidentate hydrogen bonding between the analogue carboxylic acid and the backbone N-H’s of S476 and Y477. This hydrogen bonding pattern is similar to a reported inhibitorCitation35.
Dataset alignment
The present CoMFA model was generated using a “protein-based” alignment of the training set consisting of 42 anthranilic acid-based inhibitors. The results of the alignment are depicted in . The active analogue 8 was used as a template molecule for the alignment using 12 atoms () that are common in all analogues. A rigid-body atom-by-atom superimposition of one molecule onto another was performed using the utility “Align database” available in SYBYL 8.0.
3D-QSAR CoMFA model
Inhibitory concentrations (IC50) of the 53 anthranilic acid-based inhibitors were converted into corresponding pIC50 (−log IC50) and used as dependent variables in CoMFA analysis. The data set was classified in a way that it could be obtained a representative training set in terms of molecular structure and diverse bioactivityCitation62–77. The distribution of the activity values for the test set follows the distribution of the activity values for the training set. According to Golbraikh and TropshaCitation78, this approach is correct since representative points of the test set must be close to those of training set and vice versa. The separation of the dataset into training and test set was performed in a commonly used ratio of 4:1. The training set consists of 42 molecules, and the test set consists of 11 molecules (). The Partial Least Squares (PLS) analysis was used for deriving the relationships among the CoMFA fields and the inhibitory activity (IC50 values)Citation49,Citation50. The 3D-QSAR CoMFA model was derived using the standard implementation in SYBYL 8.0 molecular modeling packageCitation46.
CoMFA analysis
CoMFA models are generated using the combination of steric and electrostatic fields. An ONC of five corresponds to the highest cross validated q2 of 0.708 and the lowest SPRESS of 0.376. A non-cross validated of 0.965, a SEE of 0.130 and an F value of 199.276 with zero probability of
show a good statistical correlation between the predicted and the experimental pIC50 values for the non-cross validated form of the CoMFA model. In , the relationship between the predicted and the observed pIC50 values is represented. The CoMFA analysis shows that the relative field contributions are 70.4 and 29.6% for steric and electrostatic field, respectively.
Validation of the 3D-QSAR model – domain of applicability
External validationCitation79,Citation80 was also performed to further assess the stability and the predictive ability of the CoMFA model. This validation was performed using 11 molecules not included in the construction of the model. The predicted pIC50 values are in correlation with the observed ones within the tolerable error range (). The external has a value of 0.820 upholding good correlation between predicted and observed pIC50 values. The proposed model has
values equal to 0.965 and 0.525 for the training and test set, respectively (). Moreover, the Enalos Model Acceptability Criteria KNIME node has been applied to the data. The model passed Tropsha’s recommended tests for predictive ability and the results are depicted in . These results suggest that this alignment can effectively take into consideration the ligand-receptor interactions, the CoMFA model is thus reliable and could be used in the design of new inhibitors of HCV replication within this structural motif of molecules.
Table 3. Statistical parameters of the CoMFA model.
It is important that the limitations of the model are also described via the domain of applicability. This gives an important indication as the user can freely and creatively design novel molecules but will be warned for the reliability of the prediction when the structural characteristics cannot be tolerated by the model. After model validation, the domain of applicability of our model was also defined to ascertain that a given prediction can be considered reliableCitation56–59. Enalos Domain – Similarity node that executes the aforementioned procedure is included in our workflow and was used to assess domain of applicability of the proposed modelCitation56–59. The applicability domain limit value was defined equal to 19.537 based on the equation provided in Methods section. All compounds in the test set had values in the range of the domain of applicability. The predictions for all compounds that fell inside the domain of applicability of the model can be considered reliable.
CoMFA contour maps
The results of a CoMFA analysis were graphically represented by field contour maps. The StDev*Coeff option was selected to control the type of CoMFA fields to be viewed. The default option “contribution” was selected to control the interpretation of the CoMFA fields. In these maps, the areas around the compound that interacts favorably or unfavorably with the receptor are visualized. The contour maps are used to identify the structural features relevant to the biological activity in this series of analogues and their obtained information can be utilized for further design of anthranilic acid-based inhibitors of HCV replication.
The CoMFA fields around the active compound 8 are displayed in . The steric interactions are represented by green and yellow contour maps (); where bulky groups near the green regions increase the inhibitory activity, they cause the opposite effect near the yellow regions. The electrostatic interactions are displayed by red and blue contour maps (); where electronegative groups near the red regions increase the inhibitory activity and they decrease it near the blue regions.
Figure 7. CoMFA StDev*Coeff contour maps around the active compound 8: (a) solid for steric field (green: bulky groups are favored; yellow: bulky groups are disfavored); (b) mesh for electrostatic field (red: electronegative groups are favored; blue: electropositive groups are favored).
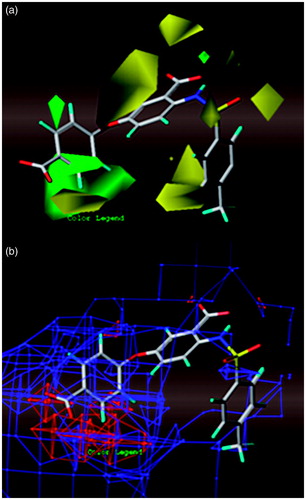
As it can be observed, the yellow regions are more than green ones for steric field in the contour map of the CoMFA model (). The large green contour map and small one around the LHS group of the anthranilic acid scaffold indicate that bulky groups at these positions increase the activity. For example, compounds 4, 5, 9, 10 and 40–47 bearing bulky groups, such as –CF3 on o- or m-position of phenoxy group showed high activities. Similarly, the small green contour map above the atom of nitrogen justifies the high activities of compounds 24, 36, 38 and 40–47, as N-iPr replaces the N-H group. On the contrary, there is a yellow contour map around the RHS group on the anthranilic acid scaffold in the contour map for steric field that indicates low activities of compounds bearing bulky groups on m-position of benzyl ring, such as compounds 5a–c. The second big yellow contour map indicates that the presence of a linker, such as the oxygen linker, between the two rings increases the activity that does not happen with inactive compounds 9a and 10a. Finally, the other three yellow contour maps on the RHS of anthranilic acid scaffold show that bulky groups among the nitrogen atom and the ring are disfavored, while a sulfonamide or carboxamide groups are suitable for this position.
In the contour map for electrostatic field in , there is a huge blue region around the binding site indicating that the introduction of electropositive groups around this position would increase the inhibitory activity. Nevertheless, the big red contour map and the small one around the LHS group of the anthranilic acid scaffold indicate that compounds with electronegative groups in these positions possess higher activity. Considering the positive potential of R490 in the proximity of LHS group, it will be suitable for negative groups, such as carboxyl groups, to be placed in this position (compounds 7 and 8).
Virtual screening
As described in the Materials and Methods section, the produced QSAR model can be used as a useful tool for screening existing databases or virtual libraries in an effort to prioritize potent compounds for experimental evaluation. In this context, a virtual screening of anthranilic acid-based analogues that are included in PubChem database was carried out using a KNIME workflow. First, 160112 compounds containing the anthranilic acid scaffold were retrieved from the PubChem database. These compounds were compared to the active compound within our original database, compound 8 (ID = 23, IC50 = 0.085 μΜ), using fingerprints based on the Tanimoto similarity metric as described in Materials and Methods section. The 1279 compounds with a Tanimoto similarity metric of over 0.80 were selected for prioritization based on the prediction of the proposed CoMFA model that was used to assess the potency of these compounds.
Our approach that is based on the scaffold and fingerprint similarity search within PubChem database resulted in a narrower chemical space that increases the chance of success since our 3D-QSAR was built based on the specific scaffold.
The pIC50 values of the 18 most potent compounds (1vs–18vs) predicted with the established CoMFA model are listed in . All the virtual screening compounds were within the domain of the applicability of the model, and therefore their activity estimations can be accepted with confidence. The predicted compounds were searched for their activity using the PubChem and ChEMBL databases, but no biological data has been found. Our searching was continued in SureChem patent chemistry database that contains a collection of over 15 million chemical structures of United States (US), European Patent (EP) and World Intellectual Property Organization (WIPO) full-text patents since 1976. The information retrieved is summarized in .
Table 4. Structures and pIC50 values of VS compounds.
Table 5. Documents of VS compounds based on SureChem patent chemistry database.
It is notable that compounds 2vs–14vs have been introduced as small molecule entry inhibitors for HIV. In the patent no.: US7429677B2Citation81, it is reported that these compounds are inhibitors of the entry process of the HIV into the host cell. More specifically, they are drugs designed to block HIV from entering the human cell by interfering with various phases of attachment and fusion between HIV and the cell. As the co-infection of HCV and HIV has become a worldwide public health challenge, it is very important that our proposed methodology managed to retrieve and to conclude in these small molecules that have been already proven to act as HIV inhibitors as these small molecules could be potential drug candidates as dual inhibitors of HIV and HCV infection.
Conclusions
In this work, we have successfully developed a computational virtual screening workflow combining Molecular Docking, 3D-CoMFA QSAR and similarity search that was applied to PubChem database with the aim to identify anthranilic acid based analogues that could act as inhibitors of HCV replication. In the proposed approach, we have first developed a robust, validated and predictive 3D-QSAR model that was subsequently used to virtually screen compounds from PubChem database. We have narrowed the chemical space search by focusing only on compounds that contain the anthranilic acid scaffold. Similarity search was then used to retrieve the compounds that are similar to known active inhibitors. In this way, we have managed to identify the most promising compounds from a pool of new analogues and to prioritize a list of compounds for screening. The approach revealed several promising chemistry driven compounds with potential high activity. The workflow can also be used to screen other databases or virtual combinations to identify derivatives with desired activity.
Declaration of interest
Authors report no declarations of interest.
This work is supported by funding under the Seven Research Framework Program of the European Union. Project SYSPATHO (ex. PATHOSYS – HEALTH-F5-2010-260429).
References
- Cohen J. The scientific challenge of hepatitis C. Science 1999;285:26–30
- Lavanchy D. The global burden of hepatitis C. Liver Int 2009;29:74–81
- Hadziyannis SJ, Sette H Jr, Morgan TR, et al. Peginterferon-alpha2a and ribavirin combination therapy in chronic hepatitis C: a randomized study of treatment duration and ribavirin dose. Ann Intern Med 2004;140:346–55
- Manns MP, Wedemeyer H, Cornberg M. Treating viral hepatitis C: efficacy, side effects, and complications. Gut 2006;55:1350–9
- Chang MH, Gordon LA, Fung HB. Boceprevir: a protease inhibitor for the treatment of hepatitis C. Clin Ther 2012;34:2021–38
- Forestier N, Zeuzem S. Telaprevir for the treatment of hepatitis C. Expert Opin Pharmacother 2012;13:593–606
- Kwong AD, McNair L, Jacobson I, George S. Recent progress in the development of selected hepatitis C virus NS3/4A protease and NS5B polymerase inhibitors. Curr Opin Pharmacol 2008;8:522–31
- Sulkowski MS. Specific targeted antiviral therapy for hepatitis C. Curr Gastroenterol Rep 2007;9:5–13
- Simmonds P. Genetic diversity and evolution of hepatitis C virus: 15 years on. J Gen Virol 2004;85:3173–88
- Legrand-Abravanel F, Nicot F, Izopet J. New NS5B polymerase inhibitors for hepatitis C. Expert Opin Invest Drugs 2010;19:963–75
- Beaulieu PL. Non-nucleoside inhibitors of the HCV NS5B polymerase: progress in the discovery and development of novel agents for the treatment of HCV infection. Curr Opin Invest Drugs 2007;8:614–34
- Joyce CM, Steitz TA. Polymerase structures and function: variations on a theme? J Bacteriol 1995;177:6321–9
- Ludmerer SW, Graham DJ, Boots E, et al. Replication fitness and NS5B drug sensitivity of diverse hepatitis C virus isolates characterized by using a transient replication assay. Antimicrob Agents Chemother 2005;49:2059–69
- Zhang L, Sedykh A, Tripathi A, et al. Identification of putative estrogen receptor-mediated endocrine disrupting chemicals using QSAR- and structure-based virtual screening approaches. Toxicol Appl Pharm 2013;272:67–76
- Melagraki G, Afantitis A, Sarimveis H, et al. In silico exploration for identifying structure-activity relationship of MEK inhibition and oral bioavailability for isothiazole derivatives. Chem Biol Drug Des 2010;76:397–406
- Tzanetou E, Liekens S, Kasiotis KM, et al. Antiproliferative novel isoxazoles: modeling, virtual screening, synthesis, and bioactivity evaluation. Eur J Med Chem 2014;81:139–49
- Kim K, Kim ND, Seong B. Pharmacophore-based virtual screening: a review of recent applications. Expert Opin Drug Discov 2010;5:205–22
- Ma D-L, Chan DS-H, Leung C-H. Drug repositioning by structure-based virtual screening. Chem Soc Rev 2013;42:2130–41
- Yang H, Zhong H-J, Leung K-H, et al. Structure-based design of flavone derivatives as c-myc oncogene down-regulators. Eur J Pharm Sci 2013;48:130–41
- Leung C-H, Chan DS-H, Li Y-W, et al. Hit identification of IKKβ natural product inhibitor. BMC Pharmacol Toxicol 2013;14:3
- Ma D-L, Ma VP-Y, Chan DS-H, et al. In silico screening of quadruplex-binding ligands. Methods 2012;57:106–14
- Melagraki G, Afantitis A. Ligand and structure based virtual screening strategies for hit-finding and optimization of hepatitis C (HCV) inhibitors. Curr Med Chem 2011;18:2612–19
- Talele TT, Arora P, Kulkarni SS, et al. Structure-based virtual screening, synthesis and SAR of novel inhibitors of hepatitis C virus NS5B polymerase. Bioorg Med Chem 2010;18:4630–8
- Kim J, Kim K-S, Kim D-E, Chong Y. Identification of Novel HCV RNA-dependent RNA polymerase inhibitors using pharmacophore-guided virtual screening. Chem Biol Drug Des 2008;72:585–91
- Melagraki G, Afantitis Α, Sarimveis H, et al. Identification of a series of novel derivatives as potent HCV inhibitors by a ligand-based virtual screening optimized procedure. Bioorg Med Chem 2007;15:7237–47
- Musmuca I, Caroli A, Mai A, et al. Combining 3-D quantitative structure-activity relationship with ligand based and structure based alignment procedures for in silico screening of new hepatitis C virus NS5B polymerase inhibitors. J Chem Inf Model 2010;50:662–76
- Zhu J, Li Y, Yu H, et al. Insight into the structural requirements of narlaprevir-type inhibitors of NS3/NS4A protease based on HQSAR and molecular field analyses. Comb Chem High Throughput Screen 2012;15:439–50
- Ryu K, Kim ND, Choi SI, et al. Identification of novel inhibitors of HCV RNA-dependent RNA polymerase by pharmacophore-based virtual screening and in vitro evaluation. Bioorg Med Chem 2009;17:2975–82
- Louise-May S, Yang W, Nie X, et al. Discovery of novel dialkyl substituted thiophene inhibitors of HCV by in silico screening of the NS5B RdRp. Bioorg Med Chem Lett 2007;17:3905–9
- Li T, Froeyen M, Herdewijn P. Insight into ligand selectivity in HCV NS5B polymerase: molecular dynamics simulations, free energy decomposition and docking. J Mol Model 2010;16:49–59
- Wang X, Yang W, Xu X, et al. Studies of benzothiadiazine derivatives as hepatitis C virus NS5B polymerase inhibitors using 3D-QSAR, molecular docking and molecular dynamics. Curr Med Chem 2010;17:2788–803
- Davis BC, Thorpe IF. Thumb inhibitor binding eliminates functionally important dynamics in the hepatitis C virus RNA polymerase. Proteins 2013;81:40–52
- Su L, Li L, Li Y, et al. Simple and accurate approaches to predict the activity of benzothiadiazine derivatives as HCV inhibitors. Med Chem Res 2012;21:2079–96
- Ismail MAH, Abou El Ella DA, Abouzid KAM, Mahmoud AH. Integrated structure-based activity prediction model of benzothiadiazines on various genotypes of HCV NS5b polymerase (1a, 1b and 4) and its application in the discovery of new derivatives. Bioorg Med Chem 2012;20:2455–78
- Stammers TA, Coulombe R, Rancourt J, et al. Discovery of a novel series of non-nucleoside thumb pocket 2 HCV NS5B polymerase inhibitors. Bioorg Med Chem Lett 2013;23:2585–9
- Stammers TA, Coulombe R, Duplessis M, et al. Anthranilic acid-based Thumb Pocket 2 HCV NS5B polymerase inhibitors with sub-micromolar potency in the cell-based replicon assay. Bioorg Med Chem Lett 2013;23:6879–85
- MacroModel, version 9.7. New York (NY): Schrödinger, LLC; 2012
- Kaminski GA, Friesner RA, Tirado-Rives J, Jorgensen WL. Evaluation and reparametrization of the OPLS-AA force field for proteins via comparison with accurate quantum chemical calculations on peptides. J Phys Chem B 2001;105:6474–87
- LigPrep, version 2.3. New York (NY): Schrödinger, LLC; 2012
- Schrödinger Suite 2012 Protein Preparation Wizard, Epik version 2.3, Schrödinger, LLC, New York, NY, 2012; Impact version 5.8, Schrödinger, LLC, New York, NY, 2012; Prime version 3.1, Schrödinger, LLC, New York, NY, 2012
- Shelley JC, Cholleti A, Frye LL, et al. Epik: a software program for pK(a) prediction and protonation state generation for drug-like molecules. J Comput Aided Mol Des 2007;21:681–91
- Kaminski GA, Friesner RA, Tirado-Rives J, Jorgensen WL. Evaluation and reparametrization of the OPLS-AA force field for proteins via comparison with accurate quantum chemical calculations on peptides. J Phys Chem B 2001;105:6474–87
- Kontoyianni M, McClellan LM, Sokol GS. Evaluation of docking performance: comparative data on docking algorithms. J Med Chem 2004;47:558–65
- Gasteiger J, Marsili M. Iterative partial equalization of orbital electronegativity – a rapid access to atomic charges. Tetrahedron 1980;36:3219–28
- Purcell WP, Singer JA. A brief review and table of semiempirical parameters used in the Hueckel molecular orbital method. J Chem Eng Data 1967;12:235–46
- SYBYL/QSAR and COMFA, molecular modeling Software packages, version 8.0, 2007, Tripos Inc, 1699 South Hanley Rd, St. Louis, MO; 2007
- Clark M, Cramer III DR, Van Opdenbosch N. Validation of the general purpose tripos 5.2 force field. J Comput Chem 1989;10:982–1012
- Frank I, Feikema J, Constantine N, Kowalski B. Prediction of product quality from spectral data using the partial least-squares method. J Chem Inf Comput Sci 1984;24:20–4
- Rännar S, Lindgren F, Geladi P, Wold S. A PLS kernel algorithm for data sets with many variables and fewer objects. Part 1: theory and algorithm. J Chemometrics 1994;8:111–25
- Cramer III RD, Bunce JD, Patterson DE, Frank IE. Crossvalidation, bootstrapping, and partial least squares compared with multiple regression in conventional QSAR studies. Quant Struct-Act Relat 1988;7:18–25
- Hawkins DM, Basak SC, Mills D. Assessing model fit by cross-validation. J Chem Inf Comput Sci 2003;43:579–86
- Tropsha A. Best practices for QSAR model development, validation, and exploitation. Mol Inf 2010;29:476–88
- Roy K, Chakraborty P, Mitra I, et al. Some case studies on application of ‘‘’’ metrics for judging quality of quantitative structure–activity relationship predictions: emphasis on scaling of response data. J Comput Chem 2013;34:1071–82
- Roy PP, Paul S, Mitra I, Roy K. On two novel parameters for validation of predictive QSAR models. Molecules 2009;14:1660–701
- Melagraki G, Afantitis A. Enalos KNIME nodes: exploring corrosion inhibition of steel in acidic medium. Chem Intel Lab Syst 2013;123:9–14
- Zhang S, Golbraikh A, Oloff S, et al. Novel automated lazy learning QSAR (ALL-QSAR) approach: method development, applications, and virtual screening of chemical databases using validated ALL-QSAR models. J Chem Inf Model 2006;46:1984–95
- Papa E, Kovarich S, Gramatica P. Development, validation and inspection of the applicability domain of QSPR models for physicochemical properties of polybrominated diphenyl ethers. QSAR Comb Sci 2009;28:790–6
- Liu H, Yao X, Gramatica P. The applications of machine learning algorithms in the modeling of estrogen-like chemicals. Comb Chem High Throughput Screening 2009;12:490–6
- Melagraki G, Afantitis A. Enalos InSilicoNano platform: an online decision support tool for the design and virtual screening of nanoparticles. RSC Advances 2014;4:50713–25
- Enalos KNIME Nodes (Novamechanics Ltd). Available from: http://www.novamechanics.com/knime.php
- Love RA, Parge HE, Yu X, et al. Crystallographic identification of a noncompetitive inhibitor binding site on the hepatitis C virus NS5B RNA polymerase enzyme. J Virol 2003;77:7575–81
- Sethi KK, Verma SM. A systematic quantitative approach to rational drug design and discovery of novel human carbonic anhydrase IX inhibitors. J Enzyme Inhib Med Chem 2014;29:571–81
- Melagraki G, Afantitis A, Sarimveis H, et al. QSAR study on para-substituted aromatic sulfonamides as carbonic anhydrase II inhibitors using topological information indices. Bioorg Med Chem 2006;14:1108–14
- Singh S, Supuran CT. 3D-QSAR CoMFA studies on sulfonamide inhibitors of the Rv3588c β-carbonic anhydrase from Mycobacterium tuberculosis and design of not yet synthesized new molecules. J Enzyme Inhib Med Chem 2014;29:449–55
- Agelis G, Resvani A, Koukoulitsa C, et al. Rational design, efficient syntheses and biological evaluation of N,N'-symmetrically bis-substituted butylimidazole analogs as a new class of potent angiotensin II receptor blockers. Eur J Med Chem 2013;62:352–70
- Ghasemi JB, Meftah, N. Docking, CoMFA and CoMSIA studies of a series of sulfonamides derivatives as carbonic anhydrase I inhibitors. J Enzyme Inhib Med Chem 2013;28:320–7
- Mouchlis VD, Melagraki G, Mavromoustakos T, et al. Molecular modeling on pyrimidine-urea inhibitors of TNF-α production: an integrated approach using a combination of molecular docking, classification techniques, and 3D-QSAR CoMSIA. J Chem Inf Model 2012;52:711–23
- Cichero E, Bruno O, Fossa P. Docking-based CoMFA and CoMSIA analyses of tetrahydro-β-carboline derivatives as type-5 phosphodiesterase inhibitors. J Enzyme Inhib Med Chem 2012;27:730–43
- Durdagi S, Papadopoulos M, Papahadjis D, Mavromoustakos T. Combined 3D QSAR and molecular docking studies to reveal novel cannabinoid ligands with optimum binding activity. Bioorg Med Chem Lett 2007;17:6754–63
- Melagraki G, Afantitis A, Sarimveis H, et al. A novel RBF neural network training methodology to predict toxicity to Vibrio fischeri. Mol Div 2006;10:213–21
- Afantitis A, Melagraki G, Sarimveis H, et al. Development and evaluation of a QSPR model for the prediction of diamagnetic susceptibility. QSAR Comb Sci 2008;27:432–6
- Nesmerak K, Toropov AA, Toropova AP, et al. SMILES-based quantitative structure-property relationships for half-wave potential of N-benzylsalicylthioamides. Eur J Med Chem 2013;67:111–14
- Vaidya A, Jain AK, Kumar P, et al. Predicting anti-cancer activity of quinoline derivatives: CoMFA and CoMSIA approach. J Enzyme Inhib Med Chem 2011;26:854–61
- Durdagi S, Mavromoustakos T, Papadopoulos MG. 3D QSAR CoMFA/CoMSIA, molecular docking and molecular dynamics studies of fullarene-based HIV-1 PR inhibitors. Bioorg Med Chem Lett 2008;18:6283–9
- Murumkar PR, Sharma MK, Shinde AC, Bothara KG. Three-dimensional quantitative structure-activity relationship CoMFA/CoMSIA on pyrrolidine-based tartrate diamides as TACE inhibitors. Med Chem Res 2013;22:4192–201
- Yuan J, Pu Y, Yin L. Docking-based three-dimensional quantitative structure-activity relationship (3D-QSAR) predicts binding affinities to aryl hydrocarbon receptor for polychlorinated dibenzodioxins, dibenzofurans, and biphenyls. Environ Toxicol Chem 2013;32:1453–8
- Hao M, Li Y, Zhang S-W, Yang W. Investigation on the binding mode of benzothiophene analogues as potent factor IXa (FIXa) inhibitors in thrombosis by CoMFA, docking and molecular dynamic studies. J Enzyme Inhib Med Chem 2011;26:792–804
- Golbraikh A, Tropsha A. Predictive QSAR modeling based on diversity sampling of experimental datasets for the training and test set selection. Mol Div 2002;5:231–43
- Kar S, Roy K. How far can virtual screening take us in drug discovery? Expert Opin Drug Discov 2013;8:245–61
- Ojha PK, Roy K. First report on exploring structural requirements of alpha and beta thymidine analogs for PfTMPK inhibitory activity using in silico studies. Biosystems 2013;113:177–95
- De Bethune MP, De Meyer S, Hertogs K, et al. Small molecule entry inhibitors, Patent US7429677B2; 30-09-2008