Abstract
This study assesses temporal variability and source contributions of PM1 (particles with aerodynamic diameter ≤ 1.0 µm) samples (n=51; November 2009–February 2010) from an urban location at Kanpur (26.30°N; 80.13°E; 142 m above mean sea-level) in the Indo-Gangetic Plain (IGP). A study period from November to February is preferred owing to massive loading of particulate matter in entire IGP. PM1 varies from 18 to 348 (Avg±SD: 113±72) µg m−3 in this study. A total of 11 trace metals, five major elements and four water-soluble inorganic species (WSIS) have been measured. Mass fraction of total metals (∑metals=trace+major) centres at 18±14 %, of which nearly 15 % is contributed by major elements. Furthermore, ∑WSIS contributes about 26 % to PM1 mass concentration. Abundance pattern among assessed WSIS in this study follows the order:
≈
>
> Cl−. The K-to-PM1 mass fraction (Avg: 2 %) in conjunction with air-mass back trajectories (AMBT) indicates that the prevailing north-westerly winds transport biomass burning derived pollutants from upwind IGP. A recent version of positive matrix factorisation (PMF 5.0) has been utilised to quantify the contribution of fine-mode aerosols from various sources. The contribution from each source is highly variable and shows a strong dependence on AMBT. Events with predominant contribution from biomass burning emission (>70 %) indicate origin of air-masses from source region upwind in IGP. One of the most interesting features of our study relates to the observation that secondary aerosols (contributing as high as ~60 % to PM1 loading) are predominantly derived from stationary combustion sources (
/
ratio: 0.30±0.23). Thus, our study highlights a high concentration of PM1 loading and atmospheric fog prevalent during wintertime can have a severe impact on atmospheric chemistry in the air-shed of IGP.
To access the supplementary material to this article, please see Supplementary files under ‘Article Tools’.
1. Introduction
Indo-Gangetic Plain (IGP) is considered as the most populated and highly polluted regions in India. Previous studies have made attempts to highlight the source regions of large-scale biomass burning emissions in upwind IGP (Rengarajan et al., Citation2007; Rajput et al., Citation2011, Citation2014c). Temporal variability and emission budget from upwind IGP further suggests that biomass (post-harvest agricultural-waste and bio-fuels) burning emission is a predominant source of fine-mode aerosols on an annual and seasonal basis (Rajput et al., Citation2014a, Citation2014b; Rastogi et al., Citation2014; Singh et al., Citation2014). MODIS (MODerate resolution Imaging Spectroradiometer) imageries exhibit haze advection towards marine atmospheric boundary layer over the Bay of Bengal (BoB) during NW-wind system prevailing from November to February (Ramanathan et al., Citation2007). Thus, IGP outflow under prevailing NW-winds has a profound impact on the ocean biogeochemistry of the BoB. Furthermore, implications of high aerosol loading, on the modification of cloud micro-physical properties and thereby higher frequency of severe drought and flood events in IGP and Chinese locations, have been highlighted in an earlier study (Menon et al., Citation2002).
IGP has a large stretch from northwest to northeast region in India and is the source region of highly scattered biomass burning emission. In sharp contrast, some regions in IGP are well known for industrial activities, for example, the study region Kanpur (Tripathi et al., Citation2005). Thus, biomass burning emissions and fossil-fuel combustion are all-year active sources of fine-mode aerosols in IGP. However, based on recent unequivocal evidences, it can be summarised that IGP outflow under NW-wind system shows characteristics with predominance of biomass burning emissions (Srinivas et al., Citation2011; Rajput et al., Citation2013). High loading of fine-mode aerosols and fog formation during wintertime (December–February) is a conspicuous feature in IGP (Singh and Gupta, Citation2015). This study has been carried out from an urban-cum-industrial area at Kanpur in central IGP. We assess here temporal variability of PM1 and associated metals and water-soluble inorganic species (WSIS) during November 2009–February 2010. Furthermore, source apportionment of PM1 using positive matrix factorisation (PMF) (version 5.0) has been assessed for various sources contributing to fine-mode aerosols at the Kanpur region.
2. Aerosol sampling and chemical analysis
2.1. PM1 sampling
Sampling location at Kanpur is situated in the central part of the IGP. Kanpur is one of the densely populated and polluted cities in Northern India. IGP experiences extreme weather conditions: harsh summer (April–June; Temp: ~45 °C; wind: 8–10 m/s, SW) and cold winters (December–February; Temp:<5 °C; wind: ~3 m/s, N to NW). Low visibility has been witnessed owing to severe haze and fog events here during wintertime. In this study, PM1 samples (n=51) have been collected using our own-developed and field-tested low volume air-sampler (calibrated flow rate: 10 LPM) (Chakraborty and Gupta, Citation2010). Each sample was collected onto pre-conditioned Teflon filters (47 mm diameter). PM1 sampling has been carried out on the rooftop of a 12 m tall building (Western Lab Extension) in the premises of Indian Institute of Technology (IIT) Kanpur (lat: 26.30°N; long: 80.13°E; 142 m above mean sea-level). Aerosol sampling was carried out continuously for 8–10 h (air filtered: ~5 m3) during the daytime from November 2009 to February 2010. These filters were stored at −19 °C until analysis. PM1 mass loading has been ascertained gravimetrically on an analytical balance after equilibrating filters (pre- and post-sampling) at about 35 % RH and 25 °C temperature.
2.2. Chemical analysis
For the determination of WSIS (
,
,
and Cl−), aerosol sample was extracted with Milli-Q water (resistivity: 18.2 MΩ cm) using a standardised sonication technique (10 mL×3; 5 min each) and then the particles were allowed for gravity settling. Subsequently, these aqueous extracts were transferred gently in the vials for determination of water-soluble ions on ion-chromatograph (Compact IC 761, Metrohm). A mixture of Na2CO3 (3.2 mM) and NaHCO3 (1.0 mM) was used for the elution of anions, whereas 0.7 mM dipicolinic acid solution with 0.01 % conc. HNO3 (15.2 N, Seastar; Suprapure, 67–70 % GR grade, Fisher, Leicestershire, UK) has been used for cation. Furthermore, a separate portion of PM1 sample was treated with 20 mL of conc. HNO3 (15.2 N, Seastar; Suprapure, 67–70 % GR grade, Fisher, Leicestershire, UK) at ~180 °C on a hot-plate till near dryness for the digestion of trace metals (As, Cd, Co, Cr, Cu, Ni, Pb, Se, V, Zn and Mn) and major elements (Na, K, Ca, Fe and Mg). Subsequently, the final volume was made to 100 mL using Milli-Q water. Then, it was filtered through a 0.22-µm Teflon filter. Quantitative determination of all metals reported herein has been performed on Inductively Coupled Plasma-Optical Emission Spectrometer (ICP-OES; iCAP 6300, Thermo Scientific) (Chakraborty and Gupta, Citation2010). A five-point calibration (R2>0.999) on ICP-OES was achieved using a multi-element standard solution (Fluka, 54704). Quality assurance and control pertaining to the data have been assessed in this study based on assessment of several blank filters and repeat analysis. We have processed a total of four blanks in batches along with samples for water-soluble ions and metal analysis (reference is made to for method detection limit: MDL). All concentrations of constituents in PM1 reported herein are blank corrected values (). Repeat analysis (n=6 for metals and n=5 for ions) of aerosol samples suggest that analytical uncertainty of the measurements is within±6 %.
Table 1. Analytical result on chemical constituents in PM1 (this study)
3. Results and discussion
3.1. Ambient records on temporal variability of metals and secondary aerosols: regional scenario
In this study, PM1 mass concentration varies from 18 to 348 (Avg±SD: 113±72) µg m−3 (a). Mass fraction of total metals (∑metals/PM1; As, Co, Ca, Cd, Cr, Cu, Fe, Mg, Ni, Pb, Se, V, Zn, K, Na and Mn) centres at 18±14 % (b) of which ~15 % is contributed by major elements (Σ major elements/PM1=15±12 %; Ca, Fe, Mg, K and Na). Average concentration of individual metal is given in . ∑WSIS-to-PM1 contribution averages at 26±12 % (c; in this study, ∑WSIS=
+
+
+Cl−). Average mass contributions of
,
,
and Cl− to PM1 are 9±7 %, 3±3 %,12±5 % and 2±2 %, respectively (). K (fine-mode) concentration varies from 0 to 3.32 (1.38±1.15) µg m−3. It is important to mention here that on certain sampling days, K concentration is ~0 µg m−3 (d). We have discussed source contribution in conjunction with air-mass back trajectories (AMBT) below in detail. Impact of local sources (inferred from short trajectories) and long-range transport (long trajectories) on the source contribution of PM1 at the receptor site has also been discussed therein.
Fig. 1 Temporal variability of: (a) PM1; (b, c) mass fractions of metals and water-soluble inorganic species (WSIS) and (d) K (fine-mode) mass concentration.
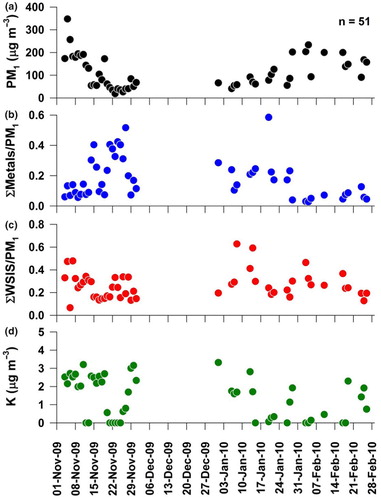
Aerosol acidity due to non-sea-salt (nss)-
is indexed in terms of
-to-
equivalent ratio less than 1. In general,
-to-
equivalent ratio of 1 suggests that all
is neutralised by
. However,
-to-
equivalent ratio greater than 1 (e.g. most of the data points in this study) suggests that
is in excess or
is a limiting reactant. In this context, previous studies have suggested that excess
can lead to the formation of aerosol phase
(Pathak et al., Citation2009). In this study,
-to-
equivalent ratio varies from 0.31 to 6.93 (Avg±SD: 2.37±1.57; a) and
/
mass ratio varies from 0.05 to 1.13 (0.30±0.23; b). It is important to mention here that temporal trends in the variability of
/
mass ratio follow a quite similar trend to
-to-
equivalent ratio. This observation is in good agreement with previous studies focusing on secondary aerosol formation (Pathak et al., Citation2009). Summing up, in this study all
is neutralised by
at Kanpur location in IGP. Furthermore, temporal variability among
,
and
indicates predominant association of
and
with
(a and b).
3.2. Distribution pattern among WSIS: a global perspective
Mass closure of WSIS among
,
,
and Cl− from this study (urban) and some other geographical locations (urban, rural, coastal and high-altitude) in the world is shown in . It is important to mention here that for comparison in PM1 samples (this study), we have adopted the Aerodyne Aerosol Mass Spectrometer (AMS) data set (wintertime) from European and Chinese locations. As shown in , it is obvious that atmospheric abundance pattern among these WSIS (Avg: 31.40 µg m−3) follows a pattern
≈
(40 %) >
(14 %) > Cl− (6 %) at the study location (Kanpur; IGP). It can be seen from that ∑WSIS concentration averages at 8.19 µg m−3 at Zurich (urban), 10.04 µg m−3 at Payerne (rural) and 2.10 µg m−3 at Montsec (a high-altitude location) with a common trend of
>
≈
>Cl− (Lanz et al., Citation2010; Ripoll et al., Citation2015). Thus, a common feature in IGP at Kanpur and European locations discussed herein is the complete neutralisation of
by
. In sharp contrast, if we compare the data set from different locations in China, ∑WSIS concentration centres at 44.65, 20.60, 19.69 and 10.33 µg m−3 at Beijing (urban), Shenzhen (urban), Kaiping (rural) and HKUST (a coastal location) (He et al., Citation2011; Huang et al., Citation2011; Zhang et al., Citation2014; Li et al., Citation2015). By and large these data sets from Chinese locations follow a trend
>
≈
>Cl−. Summing up, owing to the source variability and prevailing meteorological conditions, mass composition of WSIS exhibit a spatial variability. Furthermore, relatively high
concentration at Chinese locations has implications on the regional aerosol acidity and organosulphate formation (Surratt et al., Citation2008).
Fig. 3 Spatial variability in chemical composition of WSIS (here SWSIS=


![Fig. 3 Spatial variability in chemical composition of WSIS (here SWSIS= Display full size+ Display full size+ Display full size+ Cl−) in PM1 from some of the urban, rural, coastal and high-altitude locations. Also shown is the nitrate-to-sulphate mass ratio for these locations. Mass concentration of ∑WSIS [given in brackets] is in µg m−3.](/cms/asset/14ac0535-4935-4444-9344-a016dd21f952/zelb_a_11827484_f0003_ob.jpg)
In this study, at Kanpur location (urban; IGP)
/
mass ratio averages at 0.30±0.23. This ratio is nearly similar to 0.64 (Beijing; urban), 0.41 (Shenzhen; urban), 0.32 (Kaiping; rural) and 0.26 (HKUST; coastal) in Chinese locations (He et al., Citation2011; Huang et al., Citation2011; Zhang et al., Citation2014; Li et al., Citation2015). Thus, from this study and those over Chinese locations it is obvious that
concentration is more than the
concentration during wintertime. In a sharp contrast,
/
mass ratio of 1.82 (Zurich; urban), 3.27 (Payerne; rural) and 2.00 (Montsec; a high-altitude locations) are reported from different geographical locations during wintertime in Europe (Lanz et al., Citation2010; Ripoll et al., Citation2015). These studies suggest dominance of
over
at those locations in Europe. Thus, excess abundance of
(post to
neutralisation), volatility of
and source variability is attributable to alter
/
mass ratio on a spatial-temporal scale.
3.3. Source apportionment of PM1: PMF approach
This study utilises PMF (version 5.0) (Paatero et al., Citation2014; Rajput et al., Citation2015) for assessing source apportionment of PM1 over the study location at Kanpur in IGP. PMF is basically a receptor model and a multivariate factor analysis tool which only considers non-negative data matrix. The model works on assumption of mass conservation, and therefore, utilises a mass balance approach which leads to identify and quantify the plausible sources of atmospheric aerosols. The fundamental logic of PMF is based on the estimation of point-by-point least square minimisation which facilitates to extract the information from each and every species and sample. PMF analysis splits each data matrix into two matrices: one is the source contribution matrix (G) and the other one is source profile matrix (F). Mathematically,1
where i can vary from 1, 2 … n samples; j from 1, 2 … m species and k from 1, 2 … p factors. E is the residual matrix.
There are two modes of analysis in PMF: true and robust mode. In true mode, PMF analysis is carried out with equal weight on all data points whereas in the robust mode outliers are given less weight. Minimisation of Q value has been achieved (Supplementary Fig. 1a) in this study (robust mode) using the following approach:2
where, h ij (robustness) is introduced to reduce the effect of outliers.
To estimate uncertainties we have adopted the following approach:3
where p j is uncertainty proportional parameter and MDL j is method detection limit for the jth species (Gupta and Mandaria, Citation2013).
One of the important aspects of PMF involves the Fpeak analysis. Actually, there may exist a non-singular matrix T (of dimension p×p) such that when T and T−1 are multiplied to make solution matrices G and F, respectively, the resulting matrices G* and F* which also satisfies the non-negative constrain, and thus, will form an alternate solution. Fpeak analysis is assessed to remove rotational ambiguity in the model output. At present, we have used Fpeak from +1 to −1 (Supplementary Fig. 1b).
PMF was run (with 15 % uncertainty) for present data set (n=51) with 21 parameters as input to the model. Two species (As, Co) were marked as ‘Bad’ because of too many missing values. S/N ratio for the remaining species was observed and species for which S/N ratio was greater than 2.0 has been marked as ‘Strong’ (a). PMF analysis has been carried out with 20 run and six factor solutions. With this methodology, it was observed that all base number runs were converging and also all Q run values were between 10 % range of minimum Q value (b). This is an indication of a stable Q robust value. Thus, 20 run number and six factor solution fits model with a good measure.
Fig. 4 PMF analysis showing: (a) Species labelled as strong and weak (arbitrary scale on Y-axis) based on residual and regression analyses. Species with asterisk (As and Co) represent the bad species. (b) Variability of Q-Robust as a function of run number.
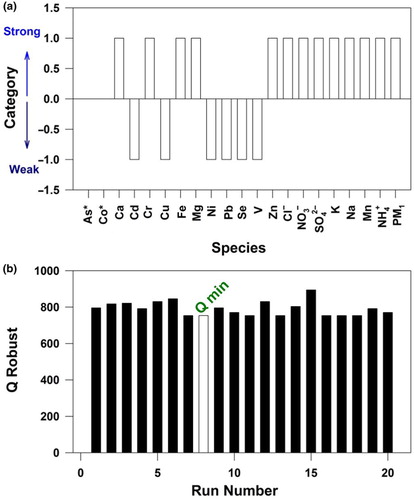
Furthermore, in the base model result, residual analysis was inspected. For a good model fit, the residual should follow a normal distribution pattern. It was observed that Pb, Se, V, Cd and Ni are exceeding +3 to −3 range of residual analysis, and therefore, these species are marked as ‘Weak’ and the model was re-run under identical conditions. This time it was observed that all species are in the range of +3 to −3. Moreover, regression analysis for different species was also carried out to assess R2 values. It was observed that only Cu has a low R2 value (<0.1). Therefore, this species is marked as ‘Weak’ species (a) and the model was re-run under an identical condition to achieve global minima Q value.
Summing up, PMF analysis has been carried out with a model uncertainty of 15 % to achieve global minima for Q (Robust; b). Finally, it has been assured that all runs are converging and all Q robust values fall in the range of 10 % of minimum Q robust value. Most of the species were found in the range of +3 to −3 in residual analysis (16 out of 21). Thus, residual analysis shows that our results (PMF) represent a good fit based on the normal distribution pattern for most of the species (between +3 and −3). A normal distribution pattern in residual analysis indicates for non-randomness of data. R2 value between observed and predicted PM1 mass concentration was observed as 0.76, suggesting that PMF analysis is a good fit model in this study (n=51).
3.4. Overall source contribution of PM1
Based on PMF analysis, in this study, we show (a–f) percentage contribution of all resolved sources (n=6) influencing aerosol contribution at Kanpur site during winter. The temporal variability of each source contribution () facilitates to better understand predominant impacts of a particular source on a given sampling event. We will now discuss the factor loading of essential constituents and overall source contribution of PM1. Subsequently, we will discuss source apportionment in conjunction with AMBT.
3.4.1. Factor 1: coal combustion
High amount of Se which is 40.6 % of the total and Mn (44.3 %), Fe (0.7 %) and Zn (4.2 %) are comprised in this factor which indicates their origin from coal combustion/industries. Contribution of
(16.7 %) further indicates this factor to be coal combustion. Coal combustion is widely used for producing electricity in thermal power plants. This source contributed 6.5 %.
3.4.2. Factor 2: leather tanneries
Kanpur is a hub for major leather industry in IGP. High amount of Cr (63.3 % of the total) with Fe (32.1 %) indicates this factor to be from the leather industries. This factor contributed 9 %.
3.4.3. Factor 3: industrial emission and construction activities
High amount of Ca which is 69.5 % of the total with Na indicates their emission from cement industries and construction activities. High content of Mg (54.9 %) and Fe (33 %) indicates for their emission from industries where iron-ore is used as a raw material (most likely from steel industry). High loading of Pb (30 % of total) indicates its origin from paint industry. This source contributed 13.5 %.
3.4.4. Factor 4: diesel emission
High content of V (34.5 % of total) with Mn (32.4 %),
(12.6 %), Zn (76.8 %) and Cl− (23.1 %) is present in this factor which is indicative of their co-production from diesel emission. This source contributes 8.2 %.
3.4.5. Factor 5: secondary aerosol formation
In this factor we observe high amounts of
(64.3 % of the total) with
(56.4) and
(48.6). Primary source of SO2 includes biomass burning emission, coal-based thermal power plant, vehicular exhaust and brick industries. This factor contributed 40.1 %.
3.4.6. Factor 6: biomass burning
This source contributes 22.7 % of PM1 with a high loading of K (78.5 % of the total) and low loading of Fe (16.8 %). Contribution of Fe in biomass burning assigned factor is in agreement with the previous studies reporting biomass burning emissions as one of the sources of water-soluble Fe. Many previous studies have shown a strong correlation of potassium with organic and elemental carbon (OC and EC) derived from biomass burning emissions. In this study, we have not assessed carbonaceous aerosols. Thus, one of the future projections from this study relates to assessing the carbonaceous aerosols too.
3.5. Percentage source contribution in conjunction with AMBT
HYSPLIT (Hybrid Single Particle Lagrangian Integrated Trajectory) model is run to infer about the AMBT at receptor site (Draxler and Rolph, Citation2003). 5d AMBTs (1000 m) above mean sea level, or amsl) are shown in for all sampling days in this study. From , it is obvious that NW-winds favor predominantly long-range transport of air pollutants from upwind IGP during November–February. During the same period, previous studies have assessed the impact of long-range transport across IGP in marine atmospheric boundary layer over the BoB. It is important to mention that these AMBTs show pathways quite similar to those inferred from MODIS satellite imageries depicting advection of haze over the BoB (Ramanathan et al., Citation2007).
Fig. 6 Percentage contribution of resolved sources in conjunction with air-mass back trajectories (5 d; 1000 m above mean sea level) on sampling days at Kanpur (shown by a circle) shows the predominance of: (a) coal combustion; (b) leather tanneries; (c) industrial emission and construction activities; (d) diesel emission; (e) secondary aerosols and (f) biomass burning emissions.
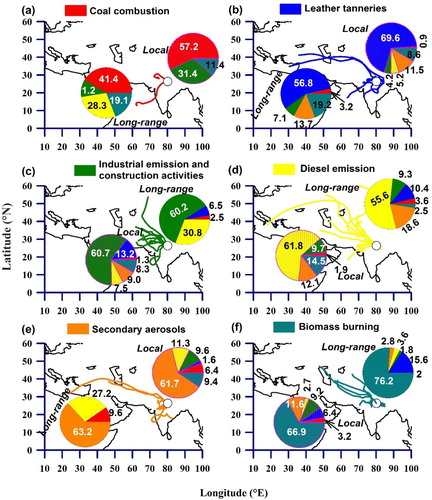
As aforementioned that for n=51 sampling events at Kanpur, PMF analysis shows aerosols contribution fromsix sources (). As shown in , on each given sampling day a particular source contributes predominantly. So, we have sorted out n=51 sampling events based on the prominent source on that day and coupled this information with AMBT (). Thus, for each source (), we couple information on AMBT to characterise the source contribution (%) into local sources (short AMBT) and long-range transport (long AMBT; ). For example, there is a difference in source contribution associated with local versus long-range transport (through west-coast of India) event when coal combustion is the predominant source (local: 57 %; long-range transport: 41 %) of PM1 at Kanpur (a). Likewise, events with pronounced impact from leather tanneries show distinct source contribution for local (70 %) versus air-mass originated from the Middle East (57 %; b). Events with predominance from industrial emission and construction activities (60 %; c), diesel emission (local: 62 %; long-range transport: 56 %; d) and secondary aerosols (60 %; e) also show differences associated with short and long AMBTs (originating from Middle East, Kazakhstan and Russia). It is important to mention here that for most of the above cases during the study period (November–February), long-range transport was associated with NW-winds. Interestingly, biomass burning emission contribution varies from ~10 to 20 %, while the other sources are predominant and the origin of air-masses point to source region other than the upwind IGP. However, there are events when the impact from biomass burning emissions is predominant (local: 67 %; long-range transport: 76 %; f). Interestingly, not only the events associated with long-range transport from upwind IGP but the local sources also relate to the predominance of biomass burning emissions on some sampling events (f). Thus, our study shows that there are local sources of biomass burning emissions too in and around the Kanpur. Summing up, this study assesses aerosol chemical characteristics and impact of sources coupled with AMBTs in central IGP (Kanpur).
4. Conclusions and implications
PM1 samples have been studied to assess temporal variability and plausible emission sources of its constituents during the period (November 2009–February 2010), impacting study location at Kanpur in the IGP. High variability in mass concentrations of PM1 (18–348 µm−3) and associated chemical constituents is attributable to local sources, long-range transport and prevailing meteorological conditions. Average
-to-
equivalent ratio of 2.4 and
/
mass ratio of 0.30 has been observed in this study. Based on trace metals, major elements and water-soluble inorganic components data (n=51 samples), a total of six source factors contributing to PM1 mass have been resolved using PMF analysis tool. The contribution from each source is highly variable and shows strong dependence on AMBT. Events with predominant contribution from biomass burning emission (>70 %) are coupled with the source origin upwind in IGP. Thus, emission source strength and its variability, and formation of secondary aerosols call for long-term ground-based measurements of ambient atmospheric aerosol constituents. Seasonal data on mass concentrations of ammonium, nitrate and sulphate in conjunction with trace metals and major elements reported in this manuscript is of utmost relevance for Chemical Transport Modeling of secondary aerosols formation. This study has implications to the impact of aerosol mixing state on radiative forcing.
Supplementary File
Download MS Word (98.5 KB)5. Acknowledgements
This study has been carried out using internal funds from IIT Kanpur. The authors thank two anonymous reviewers for their constructive suggestions and Dr. Kaarle Hämeri for editorial handling of this manuscript.
Notes
To access the supplementary material to this article, please see Supplementary files under ‘Article Tools’.
References
- Chakraborty A. , Gupta T . Chemical characterization and source apportionment of submicron (PM1) aerosol in Kanpur region. Aerosol Air Qual. Res. 2010; 10: 433–445.
- Draxler R. R., Rolph G. D. HYSPLIT (Hybrid Single-Particle Lagrangian Integrated Trajectory) Model Access via NOAA ARL READY. 2003. Website (http://www.arl.noaa.gov/ready/hysplit4.html). NOAA Air Resources Laboratory, Silver Spring, MD.
- Gupta T., Mandaria A. Sources of submicron aerosol during fog dominated wintertime at Kanpur. Environ. Sci. Pollut. Res. 2013. 20, 5615–5629. DOI: http://dx.doi.org/10.1007/s11356-11013-11580-11356.
- He L.-Y., Huang X.-F., Xue L., Hu M., Lin Y., co-authors. Submicron Aerosol analysis and organic source apportionment in an urban atmosphere in Pearl River Delta of China using high-resolution aerosol mass spectrometry. Atmos. Chem. Phys. 2011; 116 D12304. DOI: http://dx.doi.org/12310.11029/12010JD014566.
- Huang X.-F. , He L.-Y. , Hu M. , Canagaratna M. R. , Kroll J. H. , co-authors . Characterization of submicron aerosols at a rural site in Pearl River Delta of China using an Aerodyne High-Resolution Aerosol Mass Spectrometer. Atmos. Chem. Phys. 2011; 11: 1865–1877.
- Lanz V. A. , Prévôt A. S. H. , Alfarra M. R. , Weimer S. , Mohr C. , co-authors . Characterization of aerosol chemical composition with aerosol mass spectrometry in Central Europe: an overview. Atmos. Chem. Phys. 2010; 10: 10453–10471.
- Li Y. J. , Lee B. P. , Su L. , Fung J. C. H. , Chan C. K . Seasonal characteristics of fine particulate matter (PM) based on high-resolution time-of-flight aerosol mass spectrometric (HR-ToF-AMS) measurements at the HKUST Supersite in Hong Kong. Atmos. Chem. Phys. 2015; 15: 37–53.
- Mehta B. , Venkataraman C. , Bhushan M. , Tripathi S. N . Identification of sources affecting fog formation using receptor modeling approaches and inventory estimates of sectoral emissions. Atmos. Environ. 2009; 43: 1288–1295.
- Menon S., Hansen J., Nazarenko L., Luo Y. Climate effects of black Carbon Aerosols in China and India. Science. 2002; 297: 2250–2253. [PubMed Abstract].
- Paatero P. , Eberly S. , Brown S. G. , Norris G. A . Methods for estimating uncertainty in factor analytic solutions. Atmos. Meas. Tech. 2014; 7: 781–797.
- Pathak R. K. , Wu W. S. , Wang T . Summertime PM2.5 ionic species in four major cities of China: nitrate formation in an ammonia-deficient atmosphere. Atmos. Chem. Phys. 2009; 9: 1711–1722.
- Rajput P. , Mandaria A. , Kachawa L. , Singh D. K. , Singh A. K. , co-authors . Wintertime source-apportionment of PM1 from Kanpur in the Indo-Gangetic Plain. Clim. Change. 2015; 1: 503–507.
- Rajput P. , Sarin M. M. , Kundu S. S . Atmospheric particulate matter (PM2.5), EC, OC, WSOC and PAHs from NE-Himalaya: abundances and chemical characteristics. Atmos. Pollut. Res. 2013; 4: 214–221.
- Rajput P. , Sarin M. M. , Rengarajan R . High-precision GC-MS analysis of atmospheric polycyclic aromatic hydrocarbons (PAHs) and isomer ratios from biomass burning emissions. J. Environ. Protect. 2011; 2: 445–453.
- Rajput P. , Sarin M. M. , Sharma D. , Singh D . Atmospheric polycyclic aromatic hydrocarbons and isomer ratios as tracers of biomass burning emissions in Northern India. Environ. Sci. Pollut. Res. 2014a; 21: 5724–5729.
- Rajput P., Sarin M. M., Sharma D., Singh D. Characteristics and emission budget of carbonaceous species from post-harvest agricultural-waste burning in source region of the Indo-Gangetic Plain. Tellus B. 2014b; 66 21026. DOI: http://dx.doi.org/10.3402/tellusb.v66.21026.
- Rajput P. , Sarin M. M. , Sharma D. , Singh D . Organic aerosols and inorganic species from post-harvest agricultural-waste burning emissions over northern India: impact on mass absorption efficiency of elemental carbon. Environ. Sci. Process. Impacts. 2014c; 16: 2371–2379.
- Ramanathan V., Ramana M. V., Roberts G., Kim D., Corrigan C., co-authors. Warming trends in Asia amplified by brown cloud solar absorption. Nature. 2007; 448: 575–578. [PubMed Abstract].
- Rastogi N., Singh A., Singh D., Sarin M. M. Chemical characteristics of PM2.5 at a source region of biomass burning emissions: Evidence for secondary aerosol formation. Environ. Pollut. 2014; 184: 563–569. [PubMed Abstract].
- Rengarajan R. , Sarin M. M. , Sudheer A. K . Carbonaceous and inorganic species in atmospheric aerosols during wintertime over urban and high-altitude sites in North India. J. Geophys. Res. Atmos. 2007; 112: D21307.
- Ripoll A. , Minguillón M. C. , Pey J. , Jimenez J. L. , Day D. A. , co-authors . Long-term real-time chemical characterization of submicron aerosols at Montsec (southern Pyrenees, 1570 m a.s.l.). Atmos. Chem. Phys. 2015; 15: 2935–2951.
- Singh A. , Rajput P. , Sharma D. , Sarin M. M. , Singh D . Black Carbon and elemental Carbon from Postharvest Agricultural-Waste Burning Emissions in the Indo-Gangetic Plain. Adv. Meteorol. 2014; 2014: 10.
- Singh D. K., Sharma S., Habib G., Gupta T. Speciation of atmospheric polycyclic aromatic hydrocarbons (PAHs) present during fog time collected submicron particles. Environ. Sci. Pollut. Res. 2015. 22, 12458–12468. DOI: http://dx.doi.org/10.1007/s11356-11015-14413-y.
- Srinivas B. , Kumar A. , Sarin M. M. , Sudheer A. K . Impact of continental outflow on chemistry of atmospheric aerosols over tropical Bay of Bengal. Atmos. Chem. Phys. Discuss. 2011; 11: 20667–20711.
- Surratt J. D., Gómez-González Y., Chan A. W. H., Vermeylen R., Shahgholi M., co-authors. Organosulfate formation in biogenic secondary organic aerosol. J. Phys. Chem. A. 2008; 112: 8345–8378. [PubMed Abstract].
- Tripathi S. N., Dey S., Tare V., Satheesh S. K. Aerosol black carbon radiative forcing at an industrial city in northern India. Geophys. Res. Lett. 2005; 32 L08802. DOI: http://dx.doi.org/10.1029/2005GL022515.
- Zhang J. K. , Sun Y. , Liu Z. R. , Ji D. S. , Hu B. , co-authors . Characterization of submicron aerosols during a month of serious pollution in Beijing, 2013. Atmos. Chem. Phys. 2014; 14: 2887–2903.