Abstract
Measuring epidemic parameters early in an outbreak is essential to inform control efforts. Using the viral genome sequence and collection date from 78 infections in the 2014 Ebola virus outbreak in Sierra Leone, we estimate key epidemiological parameters such as infectious period duration (approximately 71 hours) and date of the first case in Sierra Leone (approximately April 25th). We also estimate the effective reproduction number, Re, (approximately 1.26), which is the number of secondary infections effectively caused by an infected individual and accounts for public health control measures. This study illustrates that phylodynamics methods, applied during the initial phase of an outbreak on fewer and more easily attainable data, can yield similar estimates to count-based epidemiological studies.
Ebola virus (EBOV) hemorrhagic fever is one of the most alarming infectious diseases known to man, killing up to 90% of its victims typically in less than 2 weeks post-exposure, with no vetted vaccine or cure.Citation1 EBOV was first discovered in 1976 in central Africa, where regular outbreaks have continued to occur in mostly isolated rural communities.Citation2,3 The virus’ recent emergence in West Africa,Citation4 beginning in Guinea in December 2013 and spreading into more populated areas of neighboring Sierra Leone, Liberia, and beyond, has claimed more lives than all previous outbreaks combined; showing no sign of abating.Citation5 Global demand for information from these countries with extremely limited public health resources has highlighted the need for innovative analysis of every bit of data available. A recent study analyzed viral sequences collected from patients in Sierra Leone and determined that the outbreak was fuelled by sustained human-to-human transmission rather than repeated zoonotic spill-overs.Citation6 As of September 22, 2014, the World Health Organization (WHO) has reported 1813 total cases (1640 confirmed) and 593 deaths in Sierra Leone since its first reported case on May 25th.Citation5
Since the initial coining of the term phylodynamics,Citation7 phylogenetic analyses have become ever more powerful and accurate in extracting epidemiological information from pathogen sequence data.Citation8 The way pathogens spread leaves footprints in their genomes and these can be informative if the number of fixated mutations is sufficiently high. Recent methods based on birth-death models now even allow inference of parameters such as the duration of the infectious period or the basic reproduction number, R0, which is the number of secondary infections caused by an infected host.Citation9 When control measures are in place, the number of potential susceptible contacts decreases and therefore the R0 drops to describe the “effective” reproduction number, Re. Practically, controlling an outbreak means reaching an Re below 1, whence the importance of this number. So far, phylodynamics methods have been applied to sampling performed over many years for large epidemics but never to the early stages of an outbreak, i.e. on a timescale of days.
Here, we analyze EBOV sequences from early in its spread to Sierra Leone (May 25-June 18) using state-of-the-art phylogenetic methods to estimate key epidemiological parameters of this ongoing outbreak.
The Study
We used 78 full EBOV genome sequences shared by Ref. (6) to infer phylogenies using Bayesian Markov Chain Monte Carlo (MCMC) through the software package Beast v2.3.1.Citation10 After several preliminary analyses (see the Online material for details), we assumed a strict molecular clock and compared 3 tree priors: a coalescent model with exponential growth (EXP,Citation11), or with Bayesian skyline (BS,Citation11) and also a birth-death (BD) model with saturation of susceptible host availability.Citation12 The best tree prior was a coalescent model with Bayesian skyline but the BD model is more adapted for an epidemiological model.
Using the BD model we found R0 = 1.26 with a 95% Highest Posterior Density (HPD) interval of [1.04-1.54] (), the median duration of the infectious period was 71 hours [47.2-123.8], and the median value for effective susceptible population size, S, was 33,056 [1,103-337,600]. Effective susceptible population size describes the size of the contact network from which the patients came, rather than the total population size of Sierra Leone. The EXP model yielded a growth rate of the infected host population of 0.063 [0.011-0.127] (). The BS model does not compute a quantity like R0 but instead estimates infected population size; it detected a great increase in the number of infected hosts between May 30 and June 9 (). This model was also used to infer the phylogeny of infection (Fig. S1). Inferring the phylogenies allowed us to estimate the rate at which mutations were fixated into the genome (substitution rate). This result is consistent with that of Ref. (6), which found substitution rates similar to ours for the current outbreak (see the Online materials for details) and show that these are higher than those recorded for earlier outbreaks.
Figure 1. (A) Epidemiological predictions for cumulative number of cases in Sierra Leone based on the SIR birth-death (BD) model for the phylogenetic tree prior. The gray region indicates the time during which sequences were collected (from day 147 – 169) and the black dots are the actual cumulative number of cases reported through Sept 10 (Day 253). Light green lines show the trajectories of stochastic model simulations, and the solid dark blue line indicates the deterministic solution (with the dashed dark blue lines indicating 95% confidence intervals and the dotted blue lines the 50% confidence intervals). (B) The same comparison as in panel A, but using the coalescent model with exponential growth (EXP) for the phylogenetic tree prior. (C) Bayesian Skyline skyline with 95% HPD inferred using the BS coalescent model for the phylogenetic tree prior.
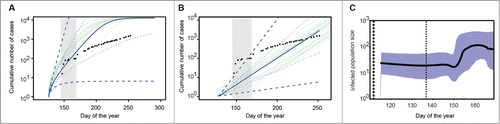
The models differed slightly with respect to dating the first case of EBOV infection in Sierra Leone, where the median common ancestral date and 95% HPD interval [number of days prior to June 18, 2014] were as follows: April 25 [32-88] for BS, May 4 [30-67] for EXP, and May 8 [30-59] for BD. In the widest interval, these models agree on an origin between Apr 12 and May 19. This corroborates the findings of Ref. 6 that suggest 2 lineages circulating in Guinea infected the attendees of one funeral in late April, then went on to seed the outbreak in Sierra Leone. Note that our estimates are robust to the simultaneous introduction of several lineages into the country.
We plotted the median cumulative number of cases along with the 95% HPD interval as predicted by the EXP and BD models, and the actual number of cases reported (black dots). We found that the early predictions (past the shaded area) made by both models were quite close: the reported number of cases falls within the confidence intervals and the observed rate of increase follows that of the prediction (). The EXP model initially underestimated the number of cases, seeming to miss the great increase in early June captured by the BS model (). The BD model predicted a higher number of cases than those reported. This could be due to the dispersed nature of the estimate for initial S (the mean value of the distribution was 89,948 but the median was 33,056), which itself may reflect the variation in size between the smaller villages, where the first cases were detected, and the larger cities (e.g. Kenema population >120,000), where the disease spread with patients seeking treatment. While we used the conservative median value for S, we cannot exclude the possibility that most transmission remained among much smaller communities, leading to over-estimation in the number of infections by our model. Our model also does not account for an exposed stage (individuals who are infected but not yet infectious, ∼2 to 21 daysCitation13), which would act to slow disease spread. The simpler model may artificially drive down our estimate of R0, particularly if incubation periods are closer to the longer end of the range. This could also explain the discrepancy between our median values and the reported data. However, it is difficult to know the accuracy of case counts, as anecdotes from the field suggest under-reporting of cases in West Africa could be widespread. Nevertheless, our future predictions are consistent with those based on classical methods using up-to-date case counts including foci erupting in larger cities (e.g., Freetown: pop. 1.2 million; 5,000 cases predicted by Nov 2nd (Day 306)Citation14). The comparable nature of these results begs the question of whether a more complex model, involving clinical parameter values that may not be available at the beginning of an emergent epidemic, is necessary for sufficiently informing quick management decisions.
Conclusions
Conventionally, R0 and Re are estimated from fitting epidemiological transmission models to infected cases and deaths in an outbreak. Recent studies using those methods found the Sierra Leone outbreak had an R0 of between 2.02 (95% CI; 1.79-2.26)Citation14 and 2.53 (2.41-2.67)Citation15 and the lower bound of previous outbreaks ranged between 1.34 and 3.65.Citation16 However, sequences collected from patients receiving care will account for public health control measures and so these methods should therefore be considered as measuring the Re. Indeed, our estimate is closer to the Re found using conventional count-based methods (ranging between 1.38 and 1.5 for the 2014 Ebola outbreak.Citation14,15,17). The 3-day duration of infectious period we found also falls just below the estimates from previous epidemics in central Africa (ranging from 3.5 to 7 daysCitation18), which could reflect differences among strains or speed of patient isolation. The short time period over which these data were collected is likely responsible for the large margins of error that grow as we project further into the future, so we encourage the epidemiological community to continue adding to the database (one sequence per patient with date and location collected) as samples are made available to help improve both precision and confidence.
Quantifying key epidemiological parameters during an outbreak can be challenging, particularly in emergency situations. This study shows that phylodynamics allows estimation of these parameters from sparse collection of single samples per patient in a population, without the need for details of their contacts or duration of symptoms. Combining such methods with classical epidemiological measurementsCitation19 will help improve the precision of these estimates for real-time epidemic studies, providing public health entities with better information on which to base critical control decisions.
Addendum
During the reviewing process of this article, another study was published that also used phylodynamics methods to estimate several epidemiological parameters.20 Stadler et al.'s results might seem different from ours but this is because they only included 72 of the 78 patients we included in our study. By ignoring these 6 patients that cluster on the top of the phylogeny (see Fig. S1 in the Online materials), they are likely to have less introduction events in their dataset, which should improve their estimate of the date of the origin of the outbreak in Sierra-Leone. At the same time, focusing on the largest cluster of the phylogeny might overestimate disease spread.
Disclosure of Potential Conflicts of Interest
No potential conflicts of interest were disclosed.
976514_Supplementary_Materials.zip
Download Zip (87.8 KB)Acknowledgments
The authors thank Gire et al. (2014) for publishing EBOV genome sequences and collection dates in the timely and open manner that facilitated this research.
Funding
SA is funded by an ATIP-Avenir from CNRS and INSERM. SL, C-LM and JLA were funded by the ANR grant 10-PDOC- 017–01 ‘SPATEVOLEPID’.
Supplemental Material
Supplemental data for this article can be accessed on the publisher's website.
References
- Fauci AS. Ebola – underscoring the global disparities in health care resources. N Engl J Med 2014; 371:1084-6; PMID:25119491; http://dx.doi.org/10.1056/NEJMp1409494
- Pourrut X, Kumulungui B, Wittmann T, Moussavou G, Delicat A, Yaba P, Nkoghe D, Gonzalez JP, Leroy EM. The natural history of Ebola virus in Africa. Microbes Infect 2005; 7:1005-14; PMID:16002313; http://dx.doi.org/10.1016/j.micinf.2005.04.006
- Groseth A, Feldmann H, Strong JE. The ecology of Ebola virus. Trends Microbiol 2007; 15(9):408-16; PMID:17698361; http://dx.doi.org/10.1016/j.tim.2007.08.001
- Baize S, Pannetier D, Oestreich L, Rieger T, Koivogui L, Magassouba N, Soropogui B, Sow MS, Keïta S, De Clerck H. Emergence of Zaire Ebola virus disease in Guinea. N Engl J Med 2014; 371(15):1418-25; PMID:24738640; http://dx.doi.org/10.1056/NEJMoa1404505
- World Health Organization (WHO). Ebola Response Roadmap Update. 2014. Sept. 22; 4 pp.; http://apps.who.int/iris/bitstream/10665/134449/1/roadmapupdate22sept14_eng.pdf?ua=1
- Gire SK, Goba A, Andersen KG, Sealfon RSG, Park DJ, Kanneh L, Jalloh S, Momoh M, Fullah M, Dudas G, et al. Genomic surveillance elucidates Ebola virus origin and transmission during the 2014 outbreak. Science 2014; 345(6202):1369-72; PMID:25214632; http://dx.doi.org/10.1126/science.1259657
- Grenfell BT, Pybus OG, Gog JR, Wood JLN, Daly JM, Mumford JA, Holmes EC, et al. Unifying the epidemiological and evolutionary dynamics of pathogens. Science 2004; 303(5656):327-32; PMID:14726583; http://dx.doi.org/10.1126/science.1090727
- Volz EM, Koelle K, Bedford T. Viral phylodynamics. PLoS Comput Biol 2013; 9(3):e1002947; PMID:23555203; http://dx.doi.org/10.1371/journal.pcbi.1002947
- Stadler T, Kouyos R, von Wyl V, Yerly S, Böni J, Bürgisser P, Klimkait T, Joos B, Rieder P, Xie D, et al. Estimating the basic reproductive number from viral sequence data. Mol Biol Evol 2012; 29(1):347-57; PMID:21890480; http://dx.doi.org/10.1093/molbev/msr217
- Bouchaert R, Heled J, Kühnert D, Vaughan T, Wu CH, Xie D, Suchard MA, Rambaut A, Drummond AJ. BEAST 2: a software platform for Bayesian evolutionary analysis. PLoS Comput Biol 2014; 10(4):e1003537; PMID:24722319; http://dx.doi.org/10.1371/journal.pcbi.1003537
- Drummond AJ, Rambaut A, Shapiro B, Pybus OG. Bayesian coalescent inference of past population dynamics from molecular sequences. Mol Biol Evol 2005; 22(5):1185-1192; PMID:15703244; http://dx.doi.org/10.1093/molbev/msi103
- Leventhal GE, Günthard HF, Bonhoeffer S, Stadler T. Using an epidemiological model for phylogenetic inference reveals density dependence in hiv transmission. Mol Biol Evol 2014; 31:6-17; PMID:24085839; http://dx.doi.org/10.1093/molbev/mst172
- Bwaka MA, Bonnet MJ, Calain P, Colebunders R, De Roo A, Guimard Y, Katwiki KR, Kibadi K, Kipasa MA, Kuvula KJ, et al. Ebola hemorrhagic fever in Kikwit, Democratic Republic of the Congo: clinical observations in 103 patients. J Infect Dis 1999; 179:S1-S7; PMID:9988155; http://dx.doi.org/10.1086/514308
- Aylward B, Barboza P, Bawo L, Bertherat E, Bilivogui P, Blake I, et al; The WHO Ebola Response Team. Ebola virus disaease in West Africa – The first 9 months of the epidemic and forward projections. N Engl J Med 2014; 371:1481-1495; PMID:24678955
- Althaus CL. Estimating the Reproduction Number of Ebola Virus (EBOV) During the 2014 Outbreak in West Africa. Plos Curr Outbreaks 2014. Sep 2. Edition 1. doi:10.1371/currents.outbreaks.91afb5e0f279e7f29e7056095255b288
- House T. Epidemiological dynamics of Ebola outbreaks. Elife 2014; 3; PMID:25217532; http://dx.doi.org/10.7554/eLife.03908
- Nishiura H, Chowell G. Early transmission dynamics of Ebola virus disease (EVD), West Africa, March to August 2014. Euro Surveill 2014; 19(36):20894; PMID:25232919
- Chowell G, Hengartner NW, Castillo-Chavez C, Fenimore PW, Hyman JM. The basic reproductive number of Ebola and the effects of public health measures: the cases of Congo and Uganda. J Theor Biol 2004; 229(1):119-26; PMID:15178190; http://dx.doi.org/10.1016/j.jtbi.2004.03.006
- Rasmussen DA, Ratmann O, Koelle K. Inference for nonlinear epidemiological models using genealogies and time series. PLoS Comput Biol 2011; 7(8): e1002136; PMID:21901082; http://dx.doi.org/10.1371/journal.pcbi.1002136
- Stadler T, Kühnert D, Rasmussen DA, du Plessis L. Insights into the early epidemic spread of ebola in Sierra Leone provided by viral sequence data. PLoS Currents Outbreaks; 2014 Oct 6.; Edition 1; doi:10.1371/currents.outbreaks.02bc6d927ecee7bbd33532ec8ba6a25f