Abstract
Monoclonal antibodies have significantly advanced our ability to treat cancer, yet clinical studies have shown that many patients do not adequately respond to monospecific therapy. This is in part due to the multifactorial nature of the disease, where tumors rely on multiple and often redundant pathways for proliferation. Bi- or multi- specific antibodies capable of blocking multiple growth and survival pathways at once have a potential to better meet the challenge of blocking cancer growth, and indeed many of them are advancing in clinical development.1 However, bispecific antibodies present significant design challenges mostly due to the increased number of variables to consider. In this perspective we describe an innovative integrated approach to the discovery of bispecific antibodies with optimal molecular properties, such as affinity, avidity, molecular format and stability. This approach combines simulations of potential inhibitors using mechanistic models of the disease-relevant biological system to reveal optimal inhibitor characteristics with antibody engineering techniques that yield manufacturable therapeutics with robust pharmaceutical properties. We illustrate how challenges of meeting the optimal design criteria and chemistry, manufacturing and control concerns can be addressed simultaneously in the context of an accelerated therapeutic design cycle. Finally, to demonstrate how this rational approach can be applied, we present a case study where the insights from mechanistic modeling were used to guide the engineering of an IgG-like bispecific antibody.
Introduction
The development of therapies to treat oncogene addicted solid tumors represents an important milestone in cancer treatment. Monoclonal antibodies (mAbs) such as bevacizumab, trastuzumab, cetuximab and panitumumab have significantly improved patient outcomes, and over two hundred therapeutic mAbs are currently being tested in clinical development.Citation2 However, it has become apparent that tumors driven by single oncogenes are not typical, and treatment often results in activation of resistance mechanisms, which in turn also require targeted intervention.Citation3,Citation4 For example, in multiple pre-clinical models of trastuzumab resistance, inhibition of IGF-1R restores sensitivity to trastuzumab.Citation5,Citation6 Combinations of targeted agents have been evaluated in the clinic,Citation7 but so far, with limited clinical successCitation8,Citation9 and considerable cost. The need to inhibit multiple targets, either due to resistance or the fact that many tumors are driven by multiple growth factor pathways, has led to increased interest in bispecific antibodies. To date, molecules in this class were mostly designed in an empirical fashion.Citation10–Citation13 Further, the pharmaceutical properties of these bispecific antibodies were almost invariably inferior to those of monospecific mAbs. These factors represent challenges to the development of bispecific anti-cancer therapies. We believe that significant added benefit from targeting multiple cancer survival pathways can be derived from increased work upfront to identify and engineer bispecific antibodies with optimal characteristics. Our iterative approach consists of computational simulation to identify the best targeting strategies and design specifications, engineering of inhibitors that possess these characteristics, and experimental validation of the formulated therapeutic hypothesis (). We separate this engineering framework into two categories: selection of an appropriate molecular format with robust pharmaceutical properties and computational simulation to identify the best targets and optimal therapeutic design characteristics. To illustrate our iterative approach, we present an integrated case study on the engineering of an IgG-like bispecific antibody () through a simulation-guided therapeutic design cycle.
Considerations of Molecular Format and Pharmaceutical Properties in Bispecific Antibody Design
One of the main advantages of mAbs is their ability to bind tightly to virtually any extracellular target. This property is driven by two features of antibody variable regions: the large flat surface of six complementarity determining regions (CDRs) and the antibody's two binding arms that double the number of binding events, increasing apparent affinity. In bispecific antibodies, targeting two molecules simultaneously also results in improved binding characteristics. Once one arm of the antibody is bound to an extracellular target, the second arm is restricted to a narrow region above the plasma membrane (about 100 angstroms), and is, therefore, concentrated near the cell surface. This results in a much faster secondary binding event that is not limited by diffusion. The acceleration of a secondary binding event is called avidity and was mathematically described first by SegalCitation14,Citation15 and later by Goldstein.Citation16 Both affinity and avidity are rationally engineerable properties, as the former can be improved via in silico affinity maturation,Citation17 and the latter can be enhanced by engineering additional targeting arms to the same or different antigen present on the cell surface.Citation18,Citation19 In addition to their binding capability, antibodies possess four main effector functions mediated by their Fc domain: antibody-dependent cellular cytotoxicity (ADCC) and antibody-dependent cellular phagocytosis (ADCP), both of which, in humans, are mediated by interactions with activating FcγRI, FcγRIIa/c, FcγRIIIa and inhibitory FcγRIIb receptors;Citation20 complement-dependent cytotoxicity (CDC) that is triggered by antibody binding to and activation of components of the complement systemCitation21 and extended half-life that is mediated via active recycling by the neonatal Fc receptor (FcRn).Citation22 All of these functions can be tuned to optimize the effectiveness of an anti-cancer therapy,Citation21,Citation23–Citation25 and are preferably retained in a bispecific molecule.
The variable fragment (Fv), composed of the variable heavy (VH) and variable light (VL) domains of an IgG antibody, is a minimal antibody fragment that displays full antigen binding. This affinity is typically retained when these variable domains can be successfully fused into a single chain construct (scFv), and it is not surprising that engineered scFvs have become highly favored building blocks for antibody engineering.Citation26 This use of scFvs has likewise extended to bispecific antibody design. A majority of the developed bispecific formats feature one or several scFv modules attached to the N- or C-terminus of an IgG heavy chain or IgG light chain via a low complexity linker.Citation12,Citation27–Citation29 Another bispecific antibody format was termed dual variable domain immunoglobulin (DVD-Ig).Citation13 A DVD-Ig consists of an IgG heavy chain with a second VH domain connected to its N-terminus by a short linker, and an IgG light chain with a second VL domain similarly connected to its N-terminus. The added VH/VL domains form a pair with specificity for one antigen while the regular VH/VL from the antibody form a separate binding site with specificity for a different antigen.
The bivalent format of IgG-like antibodies has one potential limitation: cross-linking of cell surface antigens can trigger undesirable signaling events in an uncontrolled manner. To address this challenge, tunable monovalent bispecific antibody formats have been developed. MetMab, a one armed anti-c-Met therapeutic antibody created by incorporating asymmetric “knobs-into-holes” into the Fc fragment,Citation30 has been shown to be effective in models of pancreatic cancerCitation31 and is being investigated in multiple clinical trials. This “knobs-into-holes” format has been recently extended to incorporate an antibody fragment targeting EGFR, giving rise to a functionally monovalent bispecific molecule targeting EGFR/ErbB1 and c-Met/HGFR.Citation32 Gunasekaran et al. described an alternative implementation of the “knobs-into-holes” concept involving engineering complementarily charged surfaces into the Fc fragment.Citation33 Davis et al. described a strand-exchange engineered domain (SEED) approach that used modified asymmetric Fc containing fragments from human IgG and IgA to form heteromeric monovalent antibodies.Citation34 Finally, Bostrom et al. described a novel engineering approach to construct bifunctional Fab fragments that can bind either HER2 or VEGF with high affinity.Citation35 When combined in a canonical antibody molecule, these Fab fragments will engage HER2 or VEGF with different valences that will depend on the cellular environment and growth factor concentration.
Another important component of a bispecific antibody design is the optimization of pharmaceutical properties. A therapeutic molecule must be stable, remain soluble over an extended period of time and possess robust manufacturability profile. Bispecific antibodies are typically less stable than mAbs, and initially may not possess adequate pharmaceutical properties for development. They can be stabilized through molecular engineering, through downstream formulation activities or as most commonly practiced, through the combination of both approaches.
The importance of rationally minimizing the product characteristics that complicate the development activities, often termed chemistry, manufacturing and control (CMC) liabilities, in small molecule drug candidates has been long recognized, and rules to predict druglikeness of such molecules have been proposed.Citation36 In recent years, the increased understanding of the physical and chemical parameters that govern the behavior of complex macromolecules have allowed antibody engineers to use predictive approaches to assess the fitness of IgG-based molecules by evaluating unfavorable sequence features such as: non-canonical disulfides or unpaired cysteines,Citation37 extra glycosylation sites,Citation38 tyrosine sulfation motifs,Citation39 solvent accessible methionines,Citation40 asparagine deamidation motifsCitation41 and acid cleavage sites.Citation42 Extra glycosylation sites and asparagine deamidation sites are quite common in natural antibody sequences. In fact, over 20% of the variable domains of heavy chains are reported to be glycosylated,Citation43 and over 5% of germline genes contain asparagine-glycine deamidation motifs. Deamidation rates in antibodies can be reliably estimated using the method proposed by Robinson,Citation41 which states that structurally constrained loops do not form a succinimide intermediate efficiently and therefore are stable.
While the canonical N-linked glycosylation motif (NXS and NXT, where X is any amino acid but proline) can be easily detected in antibody sequences, O-linked glycosylation sites are more difficult to recognize. Recently, several O-linked modifications of variable domains of antibody light chains have been reported, mostly in the proximity of GS rich sequence motifs.Citation44,Citation45 These small sugar modifications were detected by mass-spectroscopy techniques that are very informative in highlighting such post-translational modifications.Citation46 Many approaches to improve the affinity and stability of a candidate molecule at the discovery stage exist, e.g., structure-guided design,Citation47 focused library screeningCitation48 and yeast display;Citation49 and, we find it beneficial to remove these potential CMC liabilities in early proof-of-concept molecules.
Other CMC liabilities, such as aggregation and immunogenicity are more challenging from an engineering perspective. Not only are they both multifaceted properties, but it is also very difficult to adequately evaluate them in small scale biochemical and biophysical testing. Therefore, they tend to be detected late in development. Arguably the best approach to reduce antibody immunogenicity is through humanization.Citation50 This approach has been extensively validated through the number of humanized antibodies that have been well-tolerated in the clinic. In addition, immunogenicity of antibodies depends on their stability,Citation51 as protein aggregates typically trigger more potent immune responses than their monomeric forms.Citation52
Engineering of antibody solubility is a daunting task, as the property is also a composite of several physicochemical parameters.Citation53 Nevertheless, a number of methods to combat insolubility have been proposed. Pepinsky et al. used glycoengineering, isotype switching and structure-guided mutagenesis, to increase the solubility of a mAb.Citation54 Chennamsetty et al. described an unbiased approach to improving stability with respect to aggregation.Citation55,Citation56 It relies on molecular dynamics simulations to calculate a parameter called surface aggregation propensity and have applied this technique to introduce stabilizing amino acids in the aggregation-prone regions of antibodies. Interestingly, in their analysis of antibodies they found that aggregation-prone regions often co-locate with functionally important regions that confer Fc receptor or antigen binding functions and, therefore, cannot be easily removed.
Such a coupling between molecular function and pharmaceutical properties in antibodies is common. It can significantly complicate optimization of bispecific antibodies, as even larger parts of their sequences are located in functionally important regions. Therefore, to enable successful engineering it is important to identify critical molecular functions and optimal design characteristics.
Computational Simulation to Identify Best Targets and Optimal Therapeutic Design Characteristics
Computational simulation is a valuable tool for guiding drug development decisions, particularly in the clinic. Population pharmacokinetic (PK) modeling is a mature example of the use of models to optimize dose scheduling and clinical trial designs.Citation57 For therapies with known targets, it is possible to utilize computational simulation much earlier in the design process. Advances in multiplex, high-throughput quantitative protein measurement technologies have enabled observation of the complex dynamics that occur in cellular signaling networks.Citation58–Citation60 These data permit the creation of network models which capture the mechanistic behaviors of biological systems that are deregulated in diseases such as cancer.Citation59,Citation61–Citation63 By simulating potential inhibitors through network modeling it is possible to identify optimal therapeutic strategies,Citation64 more accurately predict design perameters and thereby accelerate the pace of drug development.
Traditionally, selection of a pharmaceutical agent for targeted therapies begins with a known target that is selected by consideration of a multitude of molecular, biological and physiological data. However, even in well-studied and heavily targeted biological systems, there are opportunities for new discoveries. These opportunities can be realized with the aid of models simulating pathways or networks. The ErbB pathway has long been the focus of cancer research due to the high expression of certain ErbB receptors in specific cancer types. For example, HER2/ErbB2 is gene amplified in some breast cancersCitation65 and EGFR/ErbB1 is highly expressed in colon cancers and non-small cell lung cancer.Citation66,Citation67 For these two members of the pathway, there are already approved mAb antagonists and small molecule tyrosine kinase inhibitors (cetuximab, erlotinib, trastuzumab, lapatinib). These therapeutics perturb the activation of downstream intracellular signaling networks by extracellular stimuli. It is difficult, however, to determine what represents an optimal therapeutic strategy given the complexity of the ErbB signaling network (). In addition to EGFR/ErbB1 and HER2/ErbB2 (which is devoid of ligand binding activity), there are two other members of the ErbB pathway: ErbB3 (kinase-dead) and ErbB4. All four receptors can homodimerize and heterodimerize to various degrees following ligand activation, which is an essential step required for intracellular signal transmission by these receptors. Following dimerization, the receptors can be internalized and recycled at rates that depend on the type of dimer, as well as on the activation status of downstream signaling pathways such as the PI3K pathway.
The complexity of the ErbB network, combined with the ability to measure the abundance and activation state of key components, makes the network an attractive system for computational modeling.Citation68 Through simulation of network models a number of biological phenomena have been explained, such as the dimerization-dependence of receptor trafficking,Citation69 control of signal amplification through feedback loopsCitation62 and ligand-dependence of signal propagation.Citation59 To find an optimal strategy for inhibiting the ErbB pathway, a network model was built to describe the activation of PI3K/AKT signaling, in response to ErbB receptor activation by the ligands betacellulin and heregulin which selectively activate EGFR/ErbB1 and ErbB3, respectively.Citation63 The mechanistic model represented the processes of: ligand binding; receptor dimerization; receptor trafficking and signal propagation, with a series of reactions defined by mass-action kinetics. In order to make reliable predictions, mechanistic models have to first be trained using temporal and dose-dependent experiments that capture key dynamic events, specifically the activation of the ErbB receptors and PI3K/AKT signaling. Network components with the greatest influence were identified by sensitivity analysis,Citation71 where each component of the network was subtly perturbed and the relative contribution to the downstream output was assessed (). Using these methods, a computational model of the ErbB network was generated that identified the heterodimerization-dependent, meagerly expressed ErbB3 receptor as the strongest activator of PI3K/AKT signaling in the presence of either heregulin or betacellulin. Notably, this in silico observation remained true in cell lines expressing relatively low levels of ErbB3 and 10-fold higher levels EGFR/ErbB1 or HER2/ErbB2.
In addition to identifying optimal targets, mechanistic modeling can be also used to determine the optimal therapeutic design characteristics. In the case of targeting the kinase-dead ErbB3, optimization simulations of a therapeutic mAb explored several design characteristics, such as binding to ErbB3, preventing heregulin binding and blockade of dimerization with a special focus to block ligand-induced EGFR/ErbB1-ErbB3 dimerization. The affinity required to achieve maximal inhibition of AKT phosphorylation was determined through simulation of inhibitors with a range of dissociation rate constants. From this simulation a 1 nanomolar affinity was predicted to be sufficient for maximal inhibitor potency, with higher affinity inhibitors displaying only limited improvement ().
Mechanistic modeling can also be used to compare in silico identified therapeutics, such as the above-mentioned “optimal ErbB3” mAb, with clinically-relevant therapies. To this end, the efficacy of the ErbB3 antibody was compared with cetuximab (an antibody to EGFR/ErbB1), pertuzumab (a HER2/ErbB2 dimerization blocking antibody) and lapatinib (a tyrosine kinase inhibitor targeting EGFR/ErbB1 and HER2/ErbB2). Using parameters obtained from literature sources, model simulation of the performance of the four ErbB inhibitors revealed that in the presence of heregulin or betacellulin only the ErbB3 mAb inhibitor could potently block ErbB3 mediated signaling. The other inhibitors only blocked ErbB3 signaling by one of the ligands at a time (either beta-cellulin or heregulin-induced ErbB3 signaling) or displayed an overall poor ability to block ErbB3 signaling, i.e., lapatinib (). These predictions were experimentally confirmed and strongly supported a therapeutic opportunity for an ErbB3 mAb as the most potent inhibitor for blocking the ErbB network in situations where tumor growth is driven by ligands, as opposed to receptor overexpression.Citation70 This network biology approach of simulating the ErbB pathway was used to design the anti-ErbB3 antibody MM-121, and should be generally applicable to cellular pathways where dynamic, quantitative biological information can be obtained and to all classes of mechanism-based therapeutics, e.g., tyrosine kinase inhibitors, multispecific antibodies.
Engineering of an IgG-Like Bispecific Antibody Molecule through a Simulation-Guided Therapeutic Design Cycle
All bispecific molecules bind their targets in a manner dependent on the affinity for each target, avidity-enhanced crosslinking ability and the relative abundance of each target. The added complexity of a bispecific interacting with a biological system creates an even greater opportunity to utilize mechanistic modeling to guide engineering efforts. Designing a bispecific optimized for potent inhibition also requires knowledge of the affinity of competing ligands and dimerization partners, if such exist, as well as the relative strength of each potential target for activating common downstream signaling cascades and subsequent cell growth and survival mechanisms. The desired overall cell binding (apparent KD) can be achieved through multiple rounds of affinity and avidity improvements, which can first be explored through simulation to guide engineering efforts towards the most suitable molecular format and a streamlined optimization route. Simulation can also be used to explore the performance of a bispecific molecule concept for permutations of target affinity, avidity and target expression levels.
Consider a bispecific antibody designed to inhibit two cell surface growth factor receptors (GFR1, GFR2) with a single binding moiety of the bispecific directed towards each target. Simulating the dose-response behavior of the bispecific in this system reveals that GFR1 is more potently inhibited when GFR2 is more highly expressed and less potently inhibited when GFR2 is more scarcely expressed (). Therefore, the ability of the bispecific antibody to inhibit GFR1 depends on the avidity created by its binding to GFR2. This phenomenon is specific to both the relative expression of the targets and the relative affinities of the binding moieties of the bispecific antibody towards the targets: inhibition of GFR2 is less affected by the expression of GFR1, as the bispecific antibody is simulated to bind to GFR2 with a higher affinity than GFR1 (). For a bispecific inhibitor, this receptor level-dependent behavior can seriously limit overall efficacy. This is depicted in the simulated effect on AKT, a downstream intracellular readout common to both pathways: poor inhibition of pAKT generation is predicted when GFR2 is under-expressed, as GFR1 is not sufficiently inhibited (). Simulation of this model system reveals that the performance of bispecific inhibitors towards each target can be highly dependent on the relative expression of both targets; this information can be useful for therapeutic design.
Selection of a therapeutic is often based on experiments testing potency in one or two representative cell lines. The choice of cell line is highly target dependent and, when multiple targets are to be inhibited, the landscape of possible target expression profiles can be very broad, thereby confounding selection of the optimal inhibitor during the screening phase without exhaustive experimentation. Furthermore, the target expression profiles observed in cell lines may not adequately cover the expression patterns anticipated in actual tumors. The impact of receptor level dependence on the potency of a bispecific can be extensively explored through simulation of many hypothetical cancer cells where the expression level of each receptor target is varied over a clinically-relevant range and the IC50 of each target and downstream readout is calculated. The shape of a calculated IC50 response surface depends on the underlying pathway interactions. In , the region where the bispecific inhibitor would be most efficacious is depicted; the asymmetry is due to the implementation of GFR2 as the stronger activator of downstream signaling. Simulation of a bispecific with single binding moieties to each target reveals that the most potent inhibition of the downstream target is centered on regions of equal target expression (). In fact, when either target is overexpressed by as little as 5-fold, the IC50 value can increase as much as 100-fold, which represents a substantial loss in potency.
Mapping actual target levels from tumor cell lines or clinical samples onto the IC50 response surface can guide therapeutic improvement efforts by answering the question of whether the region of most potent inhibition overlaps with the relevant patient population. Strategies to shift the region of predicted optimal potency to treat a different or broader patient population can also be explored first through simulation. IgG-like bispecific antibodies can have two binding moieties towards each target and therefore have same-target avidity in addition to cross-target avidity, improving the effective binding affinity for each target. Simulation of the IgG-like bispecific antibody that has monovalent binding affinities equal to the bivalent bispecific molecule shows that this format is less dependent on cross-target avidity for performance: the region of optimal potency is broader than the monovalent bispecific (). Therefore, in the case where the goal is to treat a broad patient population, the model prediction would suggest the use of an IgG-like bispecific design (Fitzgerald J, et al. unpublished results).
The benefit of affinity maturation in improving potency can also be examined through simulation prior to committing resources to a deficient strategy. Simulating downstream target inhibition across the receptor space by a non-optimal bispecific antibody identifies areas of poor inhibition, particularly when GFR1 is more highly expressed () and predicts that a significant increase in potency can be achieved by increasing the monovalent binding affinity towards GFR1 by 10-fold (). This can be achieved through lowering the dissociation rate constant of the GFR1-directed module in an affinity maturation campaign. The downstream target is inhibited significantly more potently both where the GFR1 is more highly expressed and where the GFR2 is more highly expressed, indicating that affinity maturation towards one target will enhance the potency towards the second target through cross-target avidity. This approach can be used to establish the objective of an affinity maturation campaign; for example, obtaining a desired potency towards one target while controlling the potency towards a second target to limit target-mediated toxicity.
These considerations were set as a criterion for an optimization campaign of a proof-of-concept IgG-like bispecific therapeutic molecule, the result of which showed potent inhibition of growth factor-induced signaling and tumor inhibition in xenograft models, validating the design criteria (Fitzgerald J, et al. unpublished results). This molecule comprised an IgG antibody framework directed at GFR1 and two C-terminally fused scFv modules directed at GFR2, but was not suitable for downstream development, as it contained unstable scFv modules that did not have sufficient intrinsic stability. This phenomenon is well-understood,Citation72 and engineering of scFv modules for stability by a variety of techniques including linker optimization,Citation12 disulfide engineering,Citation73 targeted mutagenesis,Citation74 co-variation analysis,Citation75 loop grafting on a stable framework,Citation76 structure-guided design,Citation77 focused design,Citation78 and phage display has been described.Citation79
To combine optimization of the affinity and stability of the scFv modules within a single campaign, we used a combination of structure-guided design, yeast surface display and micro-scale biophysical characterization. We designed structure-guided scFv variants where we introduced stability enhancing mutations, mutated motifs conferring potential CMC liabilities, removed atypical framework amino acids, and introduced variation in low diversity portions of the CDRs. Since yeast cells have eukaryotic posttranslational modification and polypeptide export machinery we monitored surface expression levels that were reported to predict thermal stability and soluble secretion efficiency.Citation80 In addition we developed a thermal challenge “cook-and-bind” protocol (Fitzgerald J, et al. unpublished results). When tested using this assay, the unstable scFv modules unfolded, while the stable high affinity molecules retained binding to the antigen and therefore were enriched (). After we isolated individual clones, we challenged scFvs fused to the yeast surface and selected the clones based on the residual affinity measured by a flow cytometry (). Thermostable scFv modules that showed over 10-fold improvement in Kd on the yeast surface were produced as soluble proteins and those showing improved antigen binding, inhibition of growth factor signaling and acceptable stability were selected (Fitzgerald J, et al. unpublished results). These optimized scFv modules were C-terminally fused to the growth factor receptor 1 antibody. The resulting IgG-like molecules were expressed in a transient expression system,Citation81 purified using protein A chromatography and profiled using biophysical, biochemical and cell signaling assays.
Among many useful biophysical techniques, we found differential scanning fluorescence and thermal inactivation assays to be most informative at the 1 to 5 µg scale.Citation82,Citation83 These give complementary information on the rate of the unfolding and the rate of aggregation for the least stable protein domain that qualitatively predicts the serum and aggregation stability of the IgG-like molecule ( and B). The importance of having a robust yet sensitive micro-scale assay is difficult to overestimate, as it directly translates into the ability to interrogate a larger number of diverse candidates with a single design campaign. The improved potency and stability of the engineered IgG-like bispecific molecule was confirmed using a normal scale assay, such as binding to cells that express both targets (). This demonstrates that approaches featuring parallel focused engineering of the modules of a multifunctional molecule followed by high-throughput production and characterization () are generally applicable to improving potency and manufacturability of targeted bispecific antibody-like molecules in the context of a single therapeutic design cycle.
Conclusions
In recent years we have achieved a significant understanding of the critical properties of antibodies that make them powerful therapeutic agents. These insights, informed by clinical successes and failures, have led to a significant interest in a second generation of multi-targeted antibody-based molecules designed to have potent mechanisms of action, high affinities and robust pharmaceutical properties. While many challenges remain, we anticipate that these engineered molecules will have broad clinical utility not just in treating tumors where both targets are highly expressed, but by blocking latent escape routes from single target inhibition. However, to fully realize the opportunity afforded by bispecific antibodies a systematic process is required to manage the increased complexity involved in their design. We believe that it is the integrated use of the computational simulation to guide selection of optimal targets, affinities and molecular formats and the therapeutic design technologies to rapidly achieve targeted potency and stability characteristics that will lead to the discovery of bispecific antibodies that revolutionize patient care.
Figures and Tables
Figure 1 Optimal design of bispecific antibodies. (A) Iterative simulation-guided therapeutic design cycle. (B) Schematic view of Ig-like bispecific molecule.
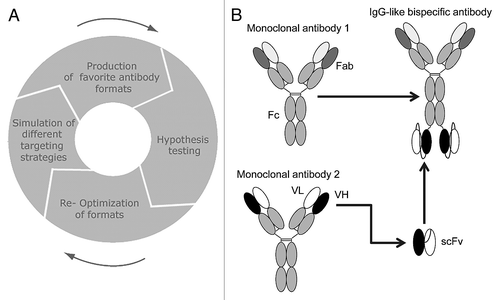
Figure 2 Simulation of the ErbB network predicts design of an optimal ErbB3 therapeutic. (A) Complexity of the ErbB network depicted graphically: ligand binding, receptor dimerization, receptor trafficking and intracellular signaling were captured in a mass-action based kinetic model. (B) Simulated perturbation of each protein in the ErbB network was used to identify the sensitivity of the downstream signal, phospho-Akt, towards each protein under either heregulin or betacellulin stimulation. (C) Dose responsiveness of Akt to an anti-ErbB3 antibody was examined for a variety of affinity binding constants through variation of the dissociation rate. (D) Different strategies to inhibit ErbB3 were examined computationally and experimentally. This figure was originally published by Schoeberl et al.Citation63 and is reprinted with permission.
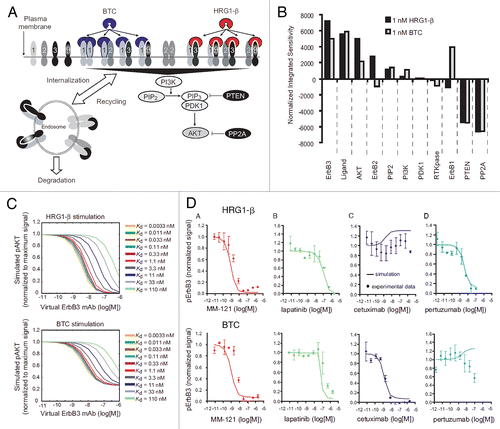
Figure 3 Ability of a bispecific to co-inhibit two pathways is dependent on relative receptor levels. The effect of a bispecific inhibitor on the active level of (A) GFR1, (B) GFR2 and (C) a common downstream signal, Akt, was simulated for cells expressing equal amounts of each target (red), ten times more of GFR1 (blue) and ten times more of GFR2 (green). Due to the lower affinity of the bispecific towards GFR1, potency of GFR1 inhibition decreases when the GFR2 level decreases (left, blue), indicating the effect of avidity. Failure to inhibit GFR1 potently leads to an inability to properly inhibit pAkt (right). The ability to inhibit GFR2 is unaffected by the level of GFR1 due the stronger affinity of the bispecific towards GFR2.
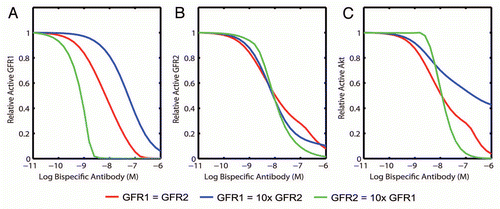
Figure 4 Simulation can be used to identify limitations of potential therapeutics and propose improvement strategies that can broaden clinical utility. A model describing the binding of a ligand-blocking bispecific inhibitor to two target receptors that both activate a common downstream signal (pAkt) was simulated with inhibitor pre-treatment of 1 h and dual ligand stimulation for 15 min. The dose-response of pAkt to the bispecific was investigated over a 1,000-fold range of each target receptor to create an IC50 response surface. (A) Simulation of a bispecific molecule with one binding moiety towards each target. (B) The IC50 response surface was re-simulated with a tetravalent bispecific inhibitor that increases the region of potent inhibition. (C) Simulation of a non-optimal IgG-like bispecific inhibitor. (D) The effect of affinity maturation of the tetravalent bispecific on downstream target inhibition was simulated by decreasing the monovalent dissociation rate towards GFR1 10-fold.
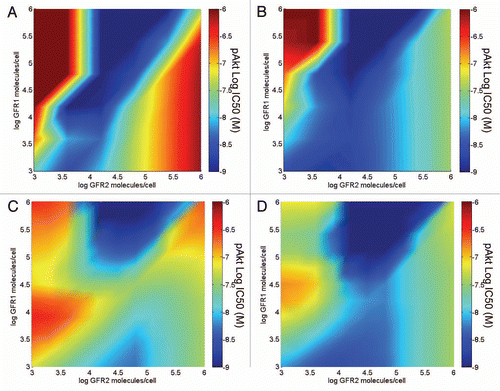
Figure 5 Concurrent optimization of scFv affinity and stability by covalent yeast display. (A) Binding of post thermal challenge isolated yeast displayed scFv modules to soluble GFR2-Fc by fluorescence-activated cell sorting. (B) Thermal challenge assay on optimized scFv modules covalently attached to yeast surface confirms their higher thermal stability. The residual binding activity to GFR2-Fc remaining after heat stress for 5 min at 65°C was measured by fluorescence-activated cell sorting.
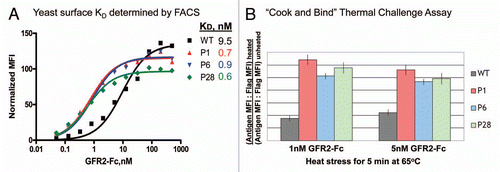
Figure 6 Comparative analysis of the properties of proof-of-concept and optimized bispecific antibodies. (A and B) Micro-scale triage composed of differential scanning fluorescence profiling and thermal inactivation assays allow the selection of bispecific with improved serum and aggregation stabilities. The proof-of-concept bispecific molecule is inferior to a stabilized one in both microscopic (measured by differential scanning fluorimetry (A)) and macroscopic properties [measured by percent of binding activity remaining after 3 day incubation in mouse serum at 37°C (B)]; (C) Optimized bispecific antibody display stronger cellular binding compared to proof-of-concept one. The binding to cancer cell line expressing both GFR1 and GFR2 was measured by fluorescence-activated cell sorting.
![Figure 6 Comparative analysis of the properties of proof-of-concept and optimized bispecific antibodies. (A and B) Micro-scale triage composed of differential scanning fluorescence profiling and thermal inactivation assays allow the selection of bispecific with improved serum and aggregation stabilities. The proof-of-concept bispecific molecule is inferior to a stabilized one in both microscopic (measured by differential scanning fluorimetry (A)) and macroscopic properties [measured by percent of binding activity remaining after 3 day incubation in mouse serum at 37°C (B)]; (C) Optimized bispecific antibody display stronger cellular binding compared to proof-of-concept one. The binding to cancer cell line expressing both GFR1 and GFR2 was measured by fluorescence-activated cell sorting.](/cms/asset/9fa80748-afb2-4394-8c6a-1936674cb4b3/kmab_a_10915299_f0006.gif)
Figure 7 A workflow for rational engineering of a bispecific antibody. The engineering path is separated in three major stages. At the proof-of-concept stage, we focus on identifying optimal design criteria and create 1–2 molecules that trigger the desired biological responses. At the therapeutic design stage, we pursue parallel optimization of the modules of the bispecific molecule. We assemble the antibody optimized modules into bispecific molecules and evaluate them relative to the optimal design criteria. At the candidate selection stage, the properties of the lead and differentiated back-ups are selected through normal scale assays.
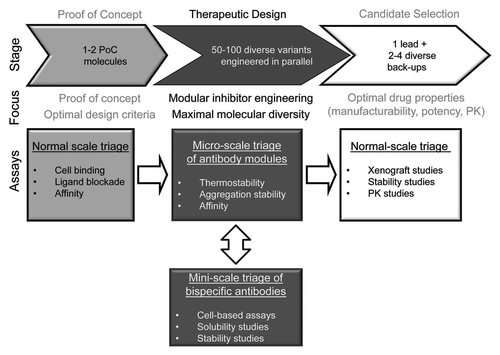
Acknowledgments
We thank Lihui Xu, Jason Baum, Kathy Zhang, Yang Jiao, Neeraj Kohli, Steven Su and Rachel Rennard for developing and optimizing the experimental approaches described in this manuscript. We thank Brian Harms, Jeffrey D. Kearns and Bin Li for their valuable insights into computational techniques for modeling antibody based therapeutics. We greatly appreciate critical input on the manuscript from Seth Fidel, Kathleen Petrozzelli, Marco Muda and Stephen Sazinsky. We are indebted to Ulrik Nielsen and Birgit Schoeberl for championing the dual use of antibody engineering and network simulation in early therapeutic design.
References
- Chames P, Baty D. Bispecific antibodies for cancer therapy: the light at the end of the tunnel?. mAbs 2009; 1:539 - 547
- Nelson AL, Dhimolea E, Reichert JM. Development trends for human monoclonal antibody therapeutics. Nat Rev Drug Discov 2010; 9:767 - 774
- Gossage L, Eisen T. Targeting multiple kinase pathways: a change in paradigm. Clin Cancer Res 2010; 16:1973 - 1978
- Chen LF, Cohen EEW, Grandis JR. New strategies in head and neck cancer: understanding resistance to epidermal growth factor receptor inhibitors. Clin Cancer Res 2010; 16:2489 - 2495
- Huang X, Gao L, Wang S, McManaman JL, Thor AD, Yang X, et al. Heterotrimerization of the growth factor receptors erbB2, erbB3 and insulin-like growth factor-i receptor in breast cancer cells resistant to herceptin. Cancer Res 2010; 70:1204 - 1214
- Browne BC, Crown J, Venkatesan N, Duffy MJ, Clynes M, Slamon D, et al. Inhibition of IGF1R activity enhances response to trastuzumab in HER-2-positive breast cancer cells. Ann Oncol 2011; 22:68 - 73
- Cirstea D, Vallet S, Raje N. Future novel single agent and combination therapies. Cancer J 2009; 15:511 - 518
- Reidy DL, Vakiani E, Fakih MG, Saif MW, Hecht JR, Goodman-Davis N, et al. Randomized, phase II study of the insulin-like growth factor-1 receptor inhibitor IMC-A12, with or without cetuximab, in patients with cetuximab- or panitumumab-refractory metastatic colorectal cancer. J Clin Oncol 2010; 28:4240 - 4246
- Hecht JR, Mitchell E, Chidiac T, Scroggin C, Hagenstad C, Spigel D, et al. A randomized phase IIIB trial of chemotherapy, bevacizumab and panitumumab compared with chemotherapy and bevacizumab alone for metastatic colorectal cancer. J Clin Oncol 2009; 27:672 - 680
- Lu D, Zhang H, Koo H, Tonra J, Balderes P, Prewett M, et al. A fully human recombinant IgG-like bispecific antibody to both the epidermal growth factor receptor and the insulin-like growth factor receptor for enhanced antitumor activity. J Biol Chem 2005; 280:19665 - 19672
- Baeuerle Pa, Reinhardt C. Bispecific T-cell engaging antibodies for cancer therapy. Cancer Res 2009; 69:4941 - 4944
- Michaelson JS, Demarest SJ, Miller B, Amatucci A, Snyder WB, Wu X, et al. Anti-tumor activity of stability-engineered IgG-like bispecific antibodies targeting TRAIL-R2 and LTbetaR. mAbs 2009; 1:128 - 141
- Wu C, Ying H, Grinnell C, Bryant S, Miller R, Clabbers A, et al. Simultaneous targeting of multiple disease mediators by a dual-variable-domain immunoglobulin. Nat Biotechnol 2007; 25:1290 - 1297
- Dower SK, DeLisi C, Titus JA, Segal DM. Mechanism of binding of multivalent immune complexes to Fc receptors. 1. Equilibrium binding. Biochemistry 1981; 20:6326 - 6334
- Dower SK, Titus JA, DeLisi C, Segal DM. Mechanism of binding of multivalent immune complexes to Fc receptors. 2. Kinetics of binding. Biochemistry 1981; 20:6335 - 6340
- Wofsy C, Goldstein B. Cross-linking of Fcgamma receptors and surface antibodies. Theory and application. J Immunol 1990; 145:1814 - 1825
- Lippow SM, Wittrup KD, Tidor B. Computational design of antibody-affinity improvement beyond in vivo maturation. Nat Biotechnol 2007; 25:1171 - 1176
- Dong J, Sereno A, Snyder WB, Miller BR, Tamraz S, Doern A, et al. Stable IgG-like bispecific antibodies directed towards the type I insulin-like growth factor receptor demonstrate enhanced ligand blockade and anti-tumor activity. J Biol Chem 2011; 286:4703 - 4717
- Robinson MK, Hodge KM, Horak E, Sundberg a L, Russeva M, Shaller CC, et al. Targeting ErbB2 and ErbB3 with a bispecific single-chain Fv enhances targeting selectivity and induces a therapeutic effect in vitro. Br J Cancer 2008; 99:1415 - 1425
- Cohen-Solal JFG, Cassard L, Fridman WH, Sautès-Fridman C. Fcgamma receptors. Immunol Lett 2004; 92:199 - 205
- Di Gaetano N, Cittera E, Nota R, Vecchi A, Grieco V, Scanziani E, et al. Complement Activation Determines the Therapeutic Activity of Rituximab In Vivo J. Immunol 2003; 171:1581 - 1587
- Brambell FWR, Hemmings WA, Morris IG. A Theoretical Model of γ-Globulin Catabolism. Nature 1964; 203:1352 - 1355
- Clynes RA, Towers TL, Presta LG, Ravetch JV. Inhibitory Fc receptors modulate in vivo cytoxicity against tumor targets. Nat Med 2000; 6:443 - 446
- Zalevsky J, Chamberlain AK, Horton HM, Karki S, Leung IWL, Sproule TJ, et al. Enhanced antibody half-life improves in vivo activity. Nat Biotechol 2010; 28:157 - 159
- Akewanlop C, Watanabe M, Singh B, Walker M, Kufe DW, Hayes DF. Phagocytosis of Breast Cancer Cells Mediated by Anti-MUC-1 Monoclonal Antibody, DF3 and Its Bispecific Antibody. Cancer Res 2001; 61:4061 - 4065
- Glockshuber R, Malia M, Pfitzinger I, Plueckthun A. A comparison of strategies to stabilize immunoglobulin Fv-fragments. Biochemistry 1990; 29:1362 - 1367
- Coloma MJ, Morrison SL. Design and production of novel tetravalent bispecific antibodies. Nat Biotechnol 1997; 15:159 - 163
- Orcutt KD, Ackerman ME, Cieslewicz M, Quiroz E, Slusarczyk AL, Frangioni JV, et al. A modular IgG-scFv bispecific antibody topology. Protein Eng Des Sel 2010; 23:221 - 228
- Dimasi N, Gao C, Fleming R, Woods RM, Yao XT, Shirinian L, et al. The design and characterization of oligospecific antibodies for simultaneous targeting of multiple disease mediators. J Mol Biol 2009; 393:672 - 692
- Ridgway JB, Presta LG, Carter P. “Knobs-into-holes” engineering of antibody CH3 domains for heavy chain heterodimerization. Protein Eng 1996; 9:617 - 621
- Jin H, Yang R, Zheng Z, Romero M, Ross J, Bou-Reslan H, et al. MetMAb, the one-armed 5D5 anti-c-Met antibody, inhibits orthotopic pancreatic tumor growth and improves survival. Cancer Res 2008; 68:4360 - 4368
- Bossenmaier B, Brinkmann U, Klein C, Niederfellner G, Schaefer W, Schanzer JM, et al. Bispecific Anti-ErbB1/Anti-c-Met Antibodies 2010; WO/2010/11555
- Gunasekaran K, Pentony M, Shen M, Garrett L, Forte C, Woodward A, et al. Enhancing antibody Fc heterodimer formation through electrostatic steering effects: Applications to bispecific molecules and monovalent IgG. J Biol Chem 2010; 285:19637 - 19646
- Davis JH, Aperlo C, Li Y, Kurosawa E, Lan Y, Lo KM, et al. SEEDbodies: fusion proteins based on strand-exchange engineered domain (SEED) CH3 heterodimers in an Fc analogue platform for asymmetric binders or immunofusions and bispecific antibodies. Protein Eng Des Sel 2010; 23:195 - 202
- Bostrom J, Yu SF, Kan D, Appleton Ba, Lee CV, Billeci K, et al. Variants of the Antibody Herceptin That Interact with HER2 and VEGF at the Antigen Binding Site. Science 2009; 323:1610 - 1614
- Lipinski C. Experimental and computational approaches to estimate solubility and permeability in drug discovery and development settings. Adv Drug Deliv Rev 2001; 46:3 - 26
- Proba K, Honegger A, Plückthun A. A natural antibody missing a cysteine in VH: consequences for thermodynamic stability and folding. J Mol Biol 1997; 265:161 - 172
- Qian J, Liu T, Yang L, Daus A, Crowley R, Zhou Q. Structural characterization of N-linked oligosaccharides on monoclonal antibody cetuximab by the combination of orthogonal matrix-assisted laser desorption/ionization hybrid quadrupole-quadrupole time-of-flight tandem mass spectrometry and sequential enzymatic digestion. Anal Biochem 2007; 364:8 - 18
- Moore KL. Protein tyrosine sulfation: a critical post-translation modification in plants and animals. Proc Natl Acad Sci USA 2009; 106:14741 - 14742
- Wang W, Vlasak J, Li Y, Pristatsky P, Fang Y, Pittman T, et al. Impact of methionine oxidation in human IgG1 Fc on serum half-life of monoclonal antibodies. Mol Immunol 2011; 48:860 - 866
- Robinson NE, Robinson AB. Deamidation of human proteins. Proc Natl Acad Sci USA 2001; 98:12409 - 12413
- Liu H, Gaza-Bulseco G, Sun J. Characterization of the stability of a fully human monoclonal IgG after prolonged incubation at elevated temperature. J Chromatogr B Analyt Technol Biomed Life Sci 2006; 837:35 - 43
- Beck A, Wagner-Rousset E, Bussat MC, Lokteff M, Klinguer-Hamour C, Haeuw JF, et al. Trends in Glycosylation, Glycoanalysis and Glycoengineering of Therapeutic Antibodies and Fc-Fusion Proteins. Curr Pharm Biotechnol 2008; 9:482 - 501
- Martinez T, Pace D, Brady L, Gerhart M, Balland A. Characterization of a novel modification on IgG2 light chain. Evidence for the presence of O-linked mannosylation. J Chromatogr A 2007; 1156:183 - 187
- Valliere-Douglass JF, Brady LJ, Farnsworth C, Pace D, Balland A, Wallace A, et al. O-fucosylation of an antibody light chain: characterization of a modification occurring on an IgG1 molecule. Glycobiology 2009; 19:144 - 152
- Wagner-Rousset E, Bednarczyk A, Bussat MC, Colas O, Corvaïa N, Schaeffer C, et al. The way forward, enhanced characterization of therapeutic antibody glycosylation: comparison of three level mass spectrometry-based strategies. J Chromatogr B Analyt Technol Biomed Life Sci 2008; 872:23 - 37
- Clark La, Boriack-Sjodin PA, Eldredge J, Fitch C, Friedman B, Hanf KJM, et al. Affinity enhancement of an in vivo matured therapeutic antibody using structure-based computational design. Protein Sci 2006; 15:949 - 960
- Miller BR, Demarest SJ, Lugovskoy A, Huang F, Wu X, Snyder WB, et al. Stability engineering of scFvs for the development of bispecific and multivalent antibodies. Protein Eng Des Sel 2010; 23:549 - 557
- Boder ET, Midelfort KS, Wittrup KD. Directed evolution of antibody fragments with monovalent femtomolar antigen-binding affinity. Proc Natl Acad Sci USA 2000; 97:10701 - 10705
- Tsurushita N, Hinton PR, Kumar S. Design of humanized antibodies: from anti-Tac to Zenapax. Methods 2005; 36:69 - 83
- Schellekens H. Bioequivalence and the immunogenicity of biopharmaceuticals. Nat Rev Drug Discov 2002; 1:457 - 462
- Rosenberg AS. Effects of protein aggregates: an immunologic perspective. The AAPS J 2006; 8:501 - 507
- Kvam E, Sierks MR, Shoemaker CB, Messer A. Physico-chemical determinants of soluble intrabody expression in mammalian cell cytoplasm. Protein Eng Des Sel 2010; 23:489 - 498
- Pepinsky RB, Silvian L, Berkowitz Sa, Farrington G, Lugovskoy A, Walus L, et al. Improving the solubility of anti-LINGO-1 monoclonal antibody Li33 by isotype switching and targeted mutagenesis. Protein Sci 2010; 19:954 - 966
- Chennamsetty N, Helk B, Voynov V, Kayser V, Trout BL. Aggregation-prone motifs in human immunoglobulin G. J Mol Biol 2009; 391:404 - 413
- Voynov V, Chennamsetty N, Kayser V, Helk B, Trout BL. Predictive tools for stabilization of therapeutic proteins. MAbs 2009; 1:580 - 582
- Zhao P, Zhang L, Grillo JA, Liu Q, Bullock JM, Moon YJ, et al. Applications of Physiologically Based Pharmacokinetic (PBPK) Modeling and Simulation During Regulatory Review. Clin Pharmacol Ther 2011; 89:259 - 267
- Wolf-Yadlin A, Hautaniemi S, Lauffenburger DA, White FM. Multiple reaction monitoring for robust quantitative proteomic analysis of cellular signaling networks. Proc Natl Acad Sci USA 2007; 104:5860 - 5865
- Chen WW, Schoeberl B, Jasper PJ, Niepel M, Nielsen UB, Lauffenburger DA, et al. Input-output behavior of ErbB signaling pathways as revealed by a mass action model trained against dynamic data. Mol Syst Biol 2009; 5:239
- Gaudet S, Janes KA, Albeck JG, Pace EA, Lauffenburger DA, Sorger PK. A compendium of signals and responses triggered by prodeath and prosurvival cytokines. Mol Cell Proteomics 2005; 4:1569 - 1590
- Birtwistle MR, Hatakeyama M, Yumoto N, Ogunnaike BA, Hoek JB, Kholodenko BN. Ligand-dependent responses of the ErbB signaling network: experimental and modeling analyses. Mol Syst Biol 2007; 3:144
- Kiyatkin A, Aksamitiene E, Markevich NI, Borisov NM, Hoek JB, Kholodenko BN. Scaffolding protein Grb2-associated binder 1 sustains epidermal growth factor-induced mitogenic and survival signaling by multiple positive feedback loops. J Biol Chem 2006; 281:19925 - 19938
- Schoeberl B, Pace EA, Fitzgerald JB, Harms BD, Xu L, Nie L, et al. Therapeutically targeting ErbB3: a key node in ligand-induced activation of the ErbB receptor-PI3K axis. Sci Signal 2009; 2:31
- Fitzgerald JB, Schoeberl B, Nielsen UB, Sorger PK. Systems biology and combination therapy in the quest for clinical efficacy. Nat Chem Biol 2006; 2:458 - 466
- Ross JS. Breast cancer biomarkers and HER2 testing after 10 years of anti-HER2 therapy. Drug News Perspect 2009; 22:93 - 106
- Yen LC, Uen YH, Wu DC, Lu CY, Yu FJ, Wu IC, et al. Activating KRAS mutations and overexpression of epidermal growth factor receptor as independent predictors in metastatic colorectal cancer patients treated with cetuximab. Ann Surg 2010; 251:254 - 260
- Hirsch FR, Varella-Garcia M, Bunn PA, Di Maria MV, Veve R, Bremmes RM, et al. Epidermal growth factor receptor in non-small-cell lung carcinomas: correlation between gene copy number and protein expression and impact on prognosis. J Clin Oncol 2003; 21:3798 - 3807
- Citri A, Yarden Y. EGF-ERBB signalling: towards the systems level. Nat Rev Mol Cell Biol 2006; 7:505 - 516
- Hendriks BS, Wiley HS, Lauffenburger D. HER2-mediated effects on EGFR endosomal sorting: analysis of biophysical mechanisms. Biophys J 2003; 85:2732 - 2745
- Schoeberl B, Faber AC, Li D, Liang MC, Crosby K, Onsum M, et al. An ErbB3 antibody, MM-121, is active in cancers with ligand-dependent activation. Cancer Res 2010; 70:2485 - 2494
- Riel NAW van. Dynamic modelling and analysis of biochemical networks: mechanism-based models and model-based experiments. Brief Bioinform 2006; 7:364 - 374
- Wörn A, Plückthun A. Stability engineering of antibody single-chain Fv fragments. J Mol Biol 2001; 305:989 - 1010
- Reiter Y, Brinkmann U, Webber KO, Jung SH, Lee B, Pastan I. Engineering interchain disulfide bonds into conserved framework regions of Fv fragments: improved biochemical characteristics of recombinant immunotoxins containing disulfide-stabilized Fv. Protein Eng Des Sel 1994; 7:697 - 704
- Wörn A, Plückthun A. Mutual stabilization of VL and VH in single-chain antibody fragments, investigated with mutants engineered for stability. Biochemistry 1998; 37:13120 - 13127
- Wang N, Smith WF, Miller BR, Aivazian D, Lugovskoy AA, Reff ME, et al. Conserved amino acid networks involved in antibody variable domain interactions. Proteins 2009; 76:99 - 114
- Jung S, Plückthun A. Improving in vivo folding and stability of a single-chain Fv antibody fragment by loop grafting. Protein Eng 1997; 10:959 - 966
- Jordan JL, Arndt JW, Hanf K, Li G, Hall J, Demarest S, et al. Structural understanding of stabilization patterns in engineered bispecific Ig-like antibody molecules. Proteins 2009; 77:832 - 841
- Miller BR, Demarest SJ, Lugovskoy A, Huang F, Wu X, Snyder WB, et al. Stability engineering of scFvs for the development of bispecific and multivalent antibodies. Protein Eng Des Sel 2010; 1 - 9
- Jung S, Honegger A, Plückthun A. Selection for improved protein stability by phage display. J Mol Biol 1999; 294:163 - 180
- Shusta EV, Kieke MC, Parke E, Kranz DM, Wittrup KD. Yeast polypeptide fusion surface display levels predict thermal stability and soluble secretion efficiency. J Mol Biol 1999; 292:949 - 956
- Baldi L, Muller N, Picasso S, Jacquet R, Girard P, Thanh HP, et al. Transient gene expression in suspension HEK-293 cells: application to large-scale protein production. Biotechnol Prog 2005; 21:148 - 153
- Lavinder JJ, Hari SB, Sullivan BJ, Magliery TJ. High-throughput thermal scanning: a general, rapid dye-binding thermal shift screen for protein engineering. J Am Chem Soc 2009; 131:3794 - 3795
- Miller BR, Glaser SM, Demarest SJ. Rapid screening platform for stabilization of scFvs in Escherichia coli. Methods Mol Biol 2009; 525:279 - 289