Abstract
Background
China is one of the countries with the fastest growing prevalence of diabetes mellitus (DM) in the world. This study intended to investigate the association of single nucleotide polymorphisms (SNPs) of FHL5 and LPA with DM risk in the Chinese population.
Methods
This case-control study involved 1,420 Chinese individuals (710 DM patients and 710 controls). Four candidate loci (rs2252816/rs9373985 in FHL5 and rs3124784/rs7765781 in LPA) were successfully screened. The association of SNPs with DM risk was assessed by logistic regression analysis. Differences in clinical characteristics among subjects with different genotypes were analyzed by one-way analysis of variance.
Results
Overall analysis indicated that rs3124784 was associated with an increased risk of DM. Stratification analysis showed that rs3124784 significantly increased DM risk in different subgroups (male, non-smoking, non-drinking, and BMI > 24), while rs7765781 increased DM risk only in participants with BMI ≤ 24. Rs2252816 was associated with the course of DM. We also found that rs2252816 GG genotype and rs9373985 GG genotype were linked to the increased cystatin c in DM patients.
Conclusion
The genetic polymorphisms of LPA may be associated with DM risk in the Chinese population, which will provide useful information for the prevention and diagnosis of DM.
Introduction
Diabetes mellitus (DM), characterized by chronic hyperglycemia and disturbances in carbohydrate, protein and fat metabolism, is mainly caused by defective or dysfunctional insulin secretion. DM can be classified as type 1 diabetes (T1D), type 2 diabetes (T2D), gestational diabetes mellitus (GDM), and other types of diabetes from other causes [Citation1], of which T2D is the most common type of DM, accounting for more than 90% of all types [Citation2]. With economic development, diet changes and the aging of population, DM has become a major public health problem worldwide [Citation3]. China is one of the countries with the fastest growing prevalence of DM in the world and the country with the largest number of diabetic patients. In 2021, the number of diabetic patients in China reached 140 million [Citation4]. As we all know, the acute and chronic complications caused by DM, including multiple organ damage such as blood vessels, eyes, kidneys, and feet, seriously endanger people’s health and quality of life and are the main causes of disability and death in DM patients. The study on the burden of DM has shown that in 2016, the number of DM deaths in China exceeded 140,000, and the mortality rate increased from 6.3/100,000 in 1990 to 10.3/100,000 in 2016 [Citation5]. Genetic factors (mainly the polymorphisms of susceptibility genes) and risk factors (age [Citation6], obesity, smoking, drinking, etc.) play a key role in the occurrence and development of DM and its complications [Citation7,Citation8]. In recent years, a number of genetic polymorphisms related to DM have been found, especially some hotspot genes, such as TCF7L2 [Citation9,Citation10], SLC30A8 [Citation11,Citation12], KCNJ11 [Citation13,Citation14] and so on.
Four-and-a-half LIM domain 5 (FHL5), also known as the ACT gene, is a member of the four-and-a-half LIM domain (FHL) protein family, located on chromosome 6q16.1, containing eight exons [Citation15]. The study has found that FHL5 is expressed in tumor cell lines from leukemia, melanoma and squamous cell carcinoma [Citation16]. Several studies based on genome-wide association studies (GWAS) and meta-analysis have found that polymorphisms of this gene may be associated with the susceptibility to migraine [Citation15,Citation17]. Meanwhile, the study has revealed that FHL5 is correlated with the susceptibility to Alzheimer’s disease (AD) [Citation18]. Some recent studies have shown that there is a certain correlation between AD and T2D. Huang et al. have shown an increased risk of neurocognitive impairment in patients with T2D and AD, and the enrichment analysis of AD and T2D showed that these two diseases are both closely related to synaptic vesicle function and MAPK signaling pathway [Citation19]. Lynn et al. have provide a detailed review of the relationship between DM and AD. Firstly, insulin resistance is present in AD and is also a major component of the pathogenesis of T2D, which can lead to impaired brain glucose metabolism, neurodegeneration and cognitive impairment. Secondly, dysregulation of the insulin receptor and the components of insulin signaling pathway has been reported in these two diseases. In addition, inflammation, oxidative stress, mitochondrial dysfunction, amyloid deposition, and advanced glycation end products can co-occur in these two diseases, AD has been labeled as “type 3 diabetes” [Citation20]. Huang et al. have demonstrated that immune-related biological functions and pathways are closely related to AD, T2D and microvascular complications [Citation21]. Taken together, based on the above research results, we speculated that FHL5 was closely related to the occurrence of DM. Genetic polymorphisms can affect the function of the certain gene [Citation22]. Therefore, FHL5 polymorphisms might be involved in DM.
Lipoprotein(a) gene (LPA) is located on chromosome 6q26 and contains 40 exons. The protein encoded by the LPA gene is a serine protease that constitutes an important part of Lp(a). The study has suggested that Lp(a) may be a biomarker for T2D [Citation23]. The study has shown that increased Lp(a) levels are associated with reduced risk of T2D in a Chinese population with very high cardiovascular risk [Citation24]. Gudbjartsson et al. have reported that Lp(a) concentrations of lower than 10% can increase T2D risk [Citation25]. A study on Lp(a) and DM has pointed out that Lp(a) is negatively correlated with the prevalence of DM and the risk of new-onset DM [Citation26]. Kollerits et al. have shown that high Lp(a) concentrations and low-molecular weight apolipoprotein(a) isoforms are risk predictors of death (infection) in diabetic hemodialysis patients [Citation27]. The study showed that a large number of LPA kringle IV type 2 repeats are associated with an increased risk of T2D in a Chinese population with very high cardiovascular risk [Citation28]. In addition, the study by Singh et al. have demonstrated that two SNPs (rs10455872 and rs3798220) of LPA could affect plasma Lp(a) levels [Citation29]. Hence, we speculated that LPA genetic polymorphisms might be associated with DM. In other words, the genetic polymorphisms of FHL5 and LPA may be involved in the occurrence of DM, but this relevancy has not been studied in the Chinese population.
To best our knowledge, rs2252816, rs9373985, and rs3124784 had not been reported before. While rs7765781 has only been reported to be associated with premature myocardial infarction [Citation30] and premature coronary artery disease [Citation31]. However, an association study by Jiang et al. has found that alleles of the FHL5 polymorphism are associated with reduced expression levels of the FHL5 gene and reduced pre-mRNA alternative splicing levels of the FHL5 gene in a variety of human tissues [Citation17]. Meanwhile, the study has found that SNPs do not lead to amino acid changes in proteins, but may be in linkage disequilibrium with other genetic variants that could potentially affect gene expression or function [Citation32]. And there is evidence that SNPs affect gene expression [Citation33]. Another study has shown that LPA mRNA levels are higher in carriers of LPA SNPs than in noncarriers [Citation34]. Thus, the polymorphisms studied in our study may affect the development of DM through the above functional effects.
Therefore, this study selected four candidate SNPs in FHL5 and LPA in 1,420 Chinese individuals, and we overall assessed SNPs that affect the susceptibility to DM. Meanwhile, the association between these SNPs and DM was further stratified by age, gender, smoking, drinking, the course of DM, retinal degeneration and Body Mass Index (BMI). Our study will enrich the genetic data related to DM in the Chinese population, and the discovered susceptibility loci can be identified as genetic markers for DM risk prediction, so as to better offer theoretical reference for the early prevention and diagnosis of DM.
Materials and methods
Study participants
This was a case-control study officially approved by the Ethics Committee of People’s Hospital of Wanning. All study subjects were enrolled from People’s Hospital of Wanning, Hainan Province, and they gave written informed consent. According to the inclusion and exclusion criteria, a total of 710 unrelated DM patients were assigned to the DM group, and 710 individuals without DM were assigned to the control group. DM patients were newly diagnosed and confirmed by experienced specialists according to the World Health Organization (WHO) 1999 criteria [Citation35]. The inclusion criteria for the DM group were [Citation1]: age ≥ 18 years old [Citation2]; fasting plasma glucose (FPG) ≥ 7.0 mmol/L, or 2-h plasma glucose (2h PG) ≥ 11.1 mmol/L during the oral glucose tolerance test (OGTT), or Hemoglobin A1C (HbA1C) ≥ 6.5%, or previous diagnosis of DM [Citation3]; no family history of DM [Citation4]; Chinese Han [Citation5]; no family history of DM; and [Citation6] good understanding and communication. The inclusion criteria for the control group were [Citation1]: age ≥ 18 years old [Citation2]; FPG < 6.1 mmol/L and OGTT 2h PG < 7.8 mmol/L [Citation3]; no family history of DM [Citation4]; Chinese Han; and [Citation5] good understanding and communication. The exclusion criteria for both groups were [Citation1]: severe consumptive diseases, such as viral infection, hyperthyroidism, malignant tumors, etc. [Citation2]; those who were unwilling to sign the informed consent form and fill in the questionnaire; and [Citation3] the examination items required for the study were incomplete. Besides, a baseline survey of general demographic data (gender, age, height, weight, smoking/drinking status etc.) and disease history (the course and retinal degeneration in DM patients) was conducted by a professional physician for all participants.
Blood sample collection and DNA extraction
Peripheral blood samples were collected from all participants into blood collection tubes containing anticoagulant EDTA and stored in a refrigerator at −20 °C. After that, genomic DNA were extracted using a DNA extraction kit according to the manufactory’s instruction (GoldMag Co. Ltd. Xi’an, China). Eventually, the extracted DNA was stored in an ultra-low temperature freezer (–80 °C) for future research.
Selection of SNPs and SNP genotyping
Rs2252816 G/A and rs9373985 G/C on the FHL5 gene, as well as rs3124784 A/G and rs7765781 C/G on the LPA gene were selected from the 1000 Genomes Project with minor allele frequency (MAF) > 0.05, Hardy-Weinberg equilibrium (HWE) > 0.05, and Tagger r2 < 0.8. The primers were designed by MassARRAY Assay Design software. All SNPs in this study were genotyped using the MassARRAY system (Agena, San Diego, CA, USA). SNP genotypes were generated using iPLEX chemistry. Additionally, MALDI-TOF was applied to obtain profiles of different mass peaks for multiple reactions, and finally genotyping was successfully completed.
Statistical analysis
Demographic characteristics of research respondents including age (t-test) and gender, smoking, and drinking (χ2 test) were tested by SPSS 21.0 (SPSS, Chicago, IL, USA). After that, the genotype frequencies of all SNPs were tested by chi-square to determine whether they satisfied HWE. Odds ratios (ORs) and 95% confidence intervals (CIs) were calculated by logistic regression models to evaluate the association between all candidate SNPs and DM risk. OR < 1 indicated that SNP is a protective factor for DM; OR = 1 indicated that this factor has no effect on DM; and OR > 1 indicated that SNP is a risk factor for DM. A variety of genetic models were evaluated using Plink 1.9 with wild-type alleles as reference. All tests were two-sided, and p < 0.05 was considered statistically significant. Afterwards, the results were analyzed by false positive reporting probability (FPRP) to determine whether they deserved attention. The interaction of four SNPs with the risk of DM was assessed by the multifactorial dimensionality reduction (MDR) method. Differences in clinical characteristics among subjects with different genotypes were analyzed by one-way analysis of variance (ANOVA).
Results
Sample overview
Basic demographic and epidemiological information about the case and control groups is presented in . There were 1,420 unrelated participants in our study, including 710 DM patients [483 males (68.0%) and 227 females (32.0%)] with an average age of 57.68 ± 12.46 years and 710 healthy individuals [481 males (67.7%) and 229 females (32.3%)] with an average age of 57.66 ± 10.42 years. No statistical differences were observed in mean age (p = 0.974), gender distribution (p = 0.909), smoking (p = 0.524), drinking (p = 0.958), and BMI (p = 0.248) between the two groups. However, there was a significant difference in the level of FPG, Total cholesterol (TC), Triglyceride (TG), Urea (Ure), Aspartate aminotransferase (AST), AST/Alanine aminotransferase (ALT), Uric acid (UA) between groups.
Table 1. Characteristics of patients with diabetes mellitus and healthy individuals.
Association between candidate SNPs and DM risk
In this study, the genotype distribution of four SNPs conformed to HWE (p > 0.05), and MAF was greater than 5% in the study population. The specific information about all candidate SNPs is shown in . The functions of SNP were studied through the HaploReg database. The results showed that rs2252816 could affect enhancer histone marks. Rs9373985 could affect motifs changed and selected eQTL hits. Rs3124784 and rs7765781 could affect motifs changed (). In addition, we found significant differences in the allele frequency (p = 0.047) of rs3124784 between DM patients and healthy controls (). The association between four candidate SNPs and the risk of DM is shown in . The results showed that rs3124784 was significantly associated with DM risk, and no correlation was found between the remaining three candidate SNPs and DM risk (p > 0.05). Precisely, rs3124784 significantly increased DM risk under the codominant (AG vs. GG: OR = 1.32, 95% CI 1.04–1.68, p = 0.024), dominant (AG-AA vs. GG: OR = 1.30, 95% CI 1.03–1.64, p = 0.028) and log-additive (OR = 1.24, 95% CI 1.00–1.53, p = 0.047) models.
Figure 1. Comparisons of the genotype frequency and allele frequency of SNPs of FHL5/LPA genes in DM case and healthy control. (a) Comparisons of the genotype frequency of rs2252816 of FHL5 gene between the two groups. (b) Comparisons of the genotype frequency of rs9373985 of FHL5 gene between the two groups. (c) Comparisons of the genotype frequency of rs3124784 of LPA gene between the two groups. (d) Comparisons of the genotype frequency of rs7765781 of LPA gene between the two groups. (e) Comparisons of the allele frequency of rs2252816 of FHL5 gene between the two groups. (f) Comparisons of the allele frequency of rs9373985 of FHL5 gene between the two groups. (g) Comparisons of the allele frequency of rs3124784 of LPA gene between the two groups. (h) Comparisons of the allele frequency of rs7765781 of LPA gene between the two groups. ‘*’ indicate statistical significance at the 0.05 level.
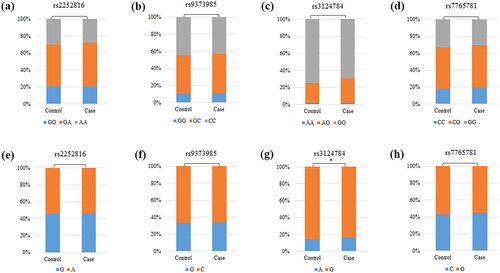
Figure 2. Analysis of the association between susceptibility of DM and SNPs. DM, diabetes mellitus; SNP, single nucleotide polymorphisms; or, odds ratio; CI, confidence interval; p values represent adjusted for age, gender, smoking, and drinking; p < 0.05, bold text and ‘*’ indicate statistical significance.

Table 2. the Basic information and HWE about the selected SNPs.
Association between candidate SNPs and DM risk stratified by clinical characteristics
After stratification by age and gender, the association between four candidate SNPs and DM risk is presented in . Rs7765781 could notably increase the risk of DM in subjects aged ≤ 60 years under a variety of genetic models (allele: OR = 1.29, 95% CI 1.06–1.57, p = 0.010; heterozygote: OR = 1.49, 95% CI 1.09–2.02, p = 0.011; homozygote: OR = 1.60, 95% CI 1.06–2.42, p = 0.025; dominant: OR = 1.51, 95% CI 1.13–2.03, p = 0.005; log-additive: OR = 1.30, 95% CI 1.07–1.59, p = 0.010). Rs7765781 also was a protective factor for DM patients aged >60 years under the following two genetic models (codominant: OR = 0.65, 95% CI 0.44–0.96, p = 0.031; dominant: OR = 0.69, 95% CI 0.48–0.98, p = 0.041). In addition, the rs3124784 polymorphism could notably increase DM risk in male participants (allele: OR = 1.33, 95% CI 1.03–1.71, p = 0.028; codominant: OR = 1.41, 95% CI 1.06–1.89, p = 0.020; dominant: OR = 1.42, 95% CI 1.06–1.89, p = 0.017; log-additive: OR = 1.37, 95% CI 1.05–1.79, p = 0.019).
Table 3. the SNPs associated with susceptibility of diabetes mellitus in the subgroup tests (age and gender).
The association between four candidate SNPs and DM risk stratified by smoking and drinking is presented in . The results indicated that rs3124784 increased the risk of DM in both non-smoking (codominant: OR = 1.46, 95% CI 1.04–2.04, p = 0.027; dominant: OR = 1.40, 95% CI 1.01–1.94, p = 0.042) and non-drinking participants (codominant: OR = 1.42, 95% CI 1.02–1.97, p = 0.038; dominant: OR = 1.38, 95% CI 1.00–1.90, p = 0.049).
Table 4. the SNPs associated with susceptibility of diabetes mellitus in the subgroup tests (smoking and drinking).
The information about the course of DM and retinal degeneration is shown in . The results indicated that rs2252816 was linked to the course of DM under a variety of genetic models (heterozygote: OR = 0.67, 95% CI 0.46–0.97, p = 0.034; homozygote: OR = 0.61, 95% CI 0.38–0.96, p = 0.035; dominant: OR = 0.65, 95% CI 0.46–0.93, p = 0.017; log-additive: OR = 0.77, 95% CI 0.61–0.97, p = 0.026).
Table 5. the SNPs associated with susceptibility of diabetes mellitus in the subgroup tests (course of diabetes mellitus and retinal degeneration).
As presented in , the association between four candidate SNPs and DM risk stratified by BMI indicated that rs3124784 increased the risk of DM in participants with BMI >24 (codominant: OR = 1.57, 95% CI 1.09–2.27, p = 0.015; dominant: OR = 1.57, 95% CI 1.10–2.25, p = 0.014; log-additive: OR = 1.50, 95% CI 1.07–2.11, p = 0.018). Meanwhile, rs7765781 increased the risk of DM in participants with BMI ≤ 24 under the allele (OR = 1.31, 95% CI 1.02–1.69, p = 0.034), codominant (OR = 1.92, 95% CI 1.10–3.33, p = 0.021), recessive (OR = 1.64, 95% CI 1.01–2.68, p = 0.047), and log-additive (OR = 1.36, 95% CI 1.04–1.78, p = 0.023) models.
Table 6. the SNPs associated with susceptibility of diabetes mellitus in the subgroup tests (BMI).
FPRP analysis
Detailed results of the FPRP analysis can be discovered in Table S1. The result showed that the association between rs2252816 and the course of DM under the homozygous gene model was not noteworthy at the prior probability level of 0.25 and FPRP value of 0.2. Meanwhile, the association between rs7765781 and DM risk in participants with BMI ≤ 24 was not noteworthy. The FPRP values of other results were all less than 0.2 at a prior probability level of 0.25, suggesting that these positive results were noteworthy.
MDR analysis
The MDR analysis was carried out to analyze and evaluate SNP-SNP interactions (). The red lines showed that there was a synergistic effect of rs7765781 and rs9373985 on DM risk. The blue lines showed that there was a redundant effect of rs3124784 and rs2252816 on DM risk. Details of SNP-SNP interactions are presented in . The results showed that the best prediction model was the four-site model: rs2252816, rs9373985, rs3124784 and rs7765781 (the largest CVC: 10/10, testing balanced accuracy: 0.501, p < 0.0001).
Figure 3. Dendrogram of SNP-SNP interactions. The colors in the tree diagram represent synergy or redundancy.
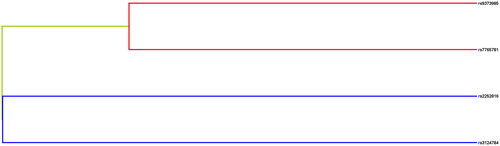
Table 7. SNP–SNP interaction models analyzed by the MDR method.
Differences in clinical characteristics among different genotypes
The associations among the four candidate SNPs and the clinical characteristics of DM patients are shown in . The results indicated that the rs2252816 GG genotype was linked to the reduced Ure in DM patients and the increased cystatin c (Cys-c) in DM patients. The rs9373985 GG genotype was associated with the increased Cys-c.
Table 8. Clinical characteristics of patients (N = 710) based on the genotypes of selected SNPs.
Discussion
This study is the first to analyze the association between the polymorphisms of FHL5 (rs2252816 G/A, rs9373985 G/C) and LPA (rs3124784 A/G, rs7765781 C/G) and DM risk in the Chinese population. We found that LPA polymorphisms (rs3124784 A/G, rs7765781 C/G) were associated with DM risk and played as risk factors in DM.
DM is one of the risk factors for coronary artery disease (CAD) [Citation36]. Previous studies have shown that the LPA gene polymorphisms are associated with CAD [Citation37], and other SNPs of LPA might be correlated with the risk of cardiovascular disease (CVD) and CAD [Citation38,Citation39]. So we speculated that LPA polymorphisms may play a vital role in DM. In the overall analysis, this study found that LPA rs3124784 was associated with an increased risk of DM in the Chinese population. Although no association of LPA polymorphisms with DM risk has been reported, the FPRP analysis in this study further validated that our results were still significant. Therefore, we speculated that LPA rs3124784 was a risk factor for DM in the Chinese population. DM demonstrates high genetic heterogeneity in populations [Citation40], and further validation of our results in different populations is needed.
Former researches have indicated that a variety of risk factors, such as age [Citation6], gender, smoking, drinking, etc [Citation7,Citation8], contribute to the development of DM. However, a study has suggested that BMI and smoking are not independent risk factors for DM [Citation6]. Consequently, our study stratified participants by the above factors, and the results indicated that rs3124784 of LPA significantly increased DM risk in different subgroups (male, non-smoking, non-drinking, BMI > 24), and gender differences were observed in DM risk. Consistent with our results, Zhang et al. have found that genetic polymorphisms can influence T2D risk in male participants [Citation41]. Contrary to our findings, Cui et al. have indicated that genetic polymorphisms are associated with T2D susceptibility in females [Citation42]. Tarnowski et al. have shown that genetic polymorphisms can influence GDM risk in female participants [Citation43]. A study has indicated that there are significant differences in blood glucose levels among male [Citation44]. Globally, more and more males are being diagnosed as DM. CAD mortality due to DM is higher in males than female [Citation45]. The above results suggest that the susceptibility to DM may vary by gender. Contrary to our findings, Zhang et al. have found that genetic polymorphisms influence T2D risk in smoking and drinking participants [Citation41]. Tian et al. have shown that genetic polymorphisms associated with the risk of T2D may be closely related to smoking and drinking [Citation46]. Taken together, the association between rs3124784 and the risk of DM may be affected by smoking and drinking. Research has showed that genetic polymorphism affect BMI [Citation44]. Consistent with our results, Matharoo et al. have revealed that genetic polymorphisms influence T2D risk in participants with high BMI and central obesity [Citation47]. Zhang et al. have found that genetic polymorphisms influence T2D risk in participants with BMI > 24 [Citation41]. Tian et al. have shown that the association of genetic polymorphisms with the risk of T2D might be affected by BMI [Citation46]. In conclusion, the association between rs3124784 and DM risk may be influenced by BMI. We also found that rs2252816 was associated with the course of DM. Consistent with our results, Zhang et al. have found that genetic polymorphisms are linked to the course of T2D [Citation41]. Previous studies of chronic diseases in China have found that the risk of death increases by 13% for each 5-year increase in the course of DM [Citation48]. Therefore, the association between genetic polymorphisms and DM may be affected by the course of the disease.
In addition, we found that the rs2252816 GG genotype and rs9373985 GG genotype were associated with an increased Cys-c in DM patients. It has been found that certain clinical blood parameters may be indicators of biological phenotypes in patients with metabolic diseases [Citation49]. Consistent with our results, the study indicated that the increased levels of Cys-c lead to adverse outcomes in women with high-risk pregnancies and GDM [Citation50]. Therefore, rs2252816 G/A and rs9373985 G/C are risk factors for DM patients.
Taken together, we first studied the correlation of between the polymorphisms of FHL5 and LPA with DM risk. The results presented that LPA is a risk gene for DM. This study increased the understanding of this gene and provided a basis for subsequent studies. Meanwhile, association studies of this gene with DM will help to develop new therapeutic targets for DM. Furthermore, in terms of individualized health management, early screening of these susceptible loci related to DM may help to understand the pathogenesis and progress of DM, and provide reference for the early detection, prevention and personalized treatment of DM.
However, our study has certain limitation due to the molecular mechanism of the polymorphisms of FHL5 and LPA in DM in the Chinese population has not been studied. In the future, we will center on this issue. Despite the above shortcoming, our study provides new insights into the association between the gene polymorphisms of FHL5 (rs2252816 G/A, rs9373985 G/C) and LPA (rs3124784 A/G, rs7765781 C/G) and DM risk.
Conclusion
In summary, this study first investigated DM risk in the Chinese population based on polymorphisms of FHL5 (rs2252816 G/A, rs9373985 G/C) and LPA (rs3124784 A/G, rs7765781 C/G). The results showed that there was a certain association between LPA gene polymorphisms (rs3124784 A/G) and DM risk. Our study further enriched the genetic data on DM susceptibility in the Chinese population, and provided a preliminary molecular basis for DM risk.
Ethical approval and consent to participation
This study fully followed the principles of the Declaration of Helsinki and was approved by the Ethics Committee of People’s Hospital of Wanning. Informed consent from all participants was obtained.
Author contributions
Xuezhong Xu wrote the paper; Xuezhong Xu, Fangyun Liang, Jinmei Chen analyzed and interpreted the data; Yipeng Ding conceived and designed the experiments; Feihong Chen, Lingyi Kong performed the experiments. All authors reviewed the manuscript.
Acknowledge
We are very grateful to all volunteers, clinicians and hospital staff who participated in this study.
Disclosure statement
No potential conflict of interest was reported by the author(s).
Additional information
Funding
References
- Classification and diagnosis of diabetes: standards of medical care in diabetes-2021. Diabetes Care. 2021;44(Suppl 1):s15–s33.
- Haghvirdizadeh P, Mohamed Z, Abdullah NA, et al. KCNJ11: genetic polymorphisms and risk of diabetes mellitus. J Diabetes Res. 2015;2015:908152. doi: 10.1155/2015/908152.
- Zimmet P, Alberti KG, Shaw J. Global and societal implications of the diabetes epidemic. Nature. 2001;414(6865):782–787. doi: 10.1038/414782a.
- Sun H, Saeedi P, Karuranga S, et al. IDF diabetes atlas: global, regional and country-level diabetes prevalence estimates for 2021 and projections for 2045. Diabetes Res Clin Pract. 2022;183:109119. doi: 10.1016/j.diabres.2021.109119.
- Liu M, Liu SW, Wang LJ, et al. Burden of diabetes, hyperglycaemia in China from to 2016: findings from the 1990 to 2016, global burden of disease study. Diabetes Metab. 2019;45(3):286–293. doi: 10.1016/j.diabet.2018.08.008.
- Ou Q, Jin W, Lin L, et al. LASSO-based machine learning algorithm to predict the incidence of diabetes in different stages. Aging Male. 2023;26(1):2205510. doi: 10.1080/13685538.2023.2205510.
- Sluik D, Boeing H, Li K, et al. Lifestyle factors and mortality risk in individuals with diabetes mellitus: are the associations different from those in individuals without diabetes? Diabetologia. 2014;57(1):63–72. doi: 10.1007/s00125-013-3074-y.
- Deshpande AD, Harris-Hayes M, Schootman M. Epidemiology of diabetes and diabetes-related complications. Phys Ther. 2008;88(11):1254–1264. doi: 10.2522/ptj.20080020.
- Reyes-López R, Perez-Luque E, Malacara JM. Relationship of lactation, BMI, and rs12255372 TCF7L2 polymorphism on the conversion to type 2 diabetes mellitus in women with previous gestational diabetes. Gynecol Endocrinol. 2019;35(5):412–416. doi: 10.1080/09513590.2018.1531984.
- Aboelkhair NT, Kasem HE, Abdelmoaty AA, et al. TCF7L2 gene polymorphism as a risk for type 2 diabetes mellitus and diabetic microvascular complications. Mol Biol Rep. 2021;48(6):5283–5290. doi: 10.1007/s11033-021-06537-0.
- Wang Y, Duan L, Yu S, et al. Association between "solute carrier family 30 member 8" (SLC30A8) gene polymorphism and susceptibility to type 2 diabetes mellitus in Chinese Han and minority populations: an updated meta-analysis. Asia Pac J Clin Nutr. 2018;27(6):1374–1390.
- Mashal S, Khanfar M, Al-Khalayfa S, et al. SLC30A8 gene polymorphism rs13266634 associated with increased risk for developing type 2 diabetes mellitus in Jordanian population. Gene. 2021;768:145279. doi: 10.1016/j.gene.2020.145279.
- Makhzoom O, Kabalan Y, Al-Quobaili F. Association of KCNJ11 rs5219 gene polymorphism with type 2 diabetes mellitus in a population of Syria: a case-control study. BMC Med Genet. 2019;20(1):107. doi: 10.1186/s12881-019-0846-3.
- Rastegari A, Rabbani M, Sadeghi HM, et al. Association of KCNJ11 (E23K) gene polymorphism with susceptibility to type 2 diabetes in iranian patients. Adv Biomed Res. 2015;4:1. doi: 10.4103/2277-9175.148256.
- Chu PH, Yeh LK, Lin HC, et al. Deletion of the FHL2 gene attenuating neovascularization after corneal injury. Invest Ophthalmol Vis Sci. 2008;49(12):5314–5318. doi: 10.1167/iovs.08-2209.
- Morgan MJ, Whawell SA. The structure of the human LIM protein ACT gene and its expression in tumor cell lines. Biochem Biophys Res Commun. 2000;273(2):776–783. doi: 10.1006/bbrc.2000.3006.
- Jiang Z, Zhao L, Zhang X, et al. Common variants in KCNK5 and FHL5 genes contributed to the susceptibility of migraine without aura in Han Chinese population. Sci Rep. 2021;11(1):6807. doi: 10.1038/s41598-021-86374-0.
- Wang X, DeKosky ST, Luedecking-Zimmer E, et al. Genetic variation in alpha(1)-antichymotrypsin and its association with Alzheimer’s disease. Hum Genet. 2002;110(4):356–365. doi: 10.1007/s00439-002-0697-3.
- Huang C, Wen X, Xie H, et al. Identification and experimental validation of marker genes between diabetes and Alzheimer’s disease. Oxid Med Cell Longev. 2022;2022:8122532. doi: 10.1155/2022/8122532.
- Lynn J, Park M, Ogunwale C, et al. A tale of two diseases: exploring mechanisms linking diabetes mellitus with Alzheimer’s disease. J Alzheimers Dis. 2022;85(2):485–501. doi: 10.3233/JAD-210612.
- Huang C, Luo J, Wen X, et al. Linking diabetes mellitus with Alzheimer’s disease: bioinformatics analysis for the potential pathways and characteristic genes. Biochem Genet. 2022;60(3):1049–1075. doi: 10.1007/s10528-021-10154-8.
- Price SJ, Greaves DR, Watkins H. Identification of novel, functional genetic variants in the human matrix metalloproteinase-2 gene: role of Sp1 in allele-specific transcriptional regulation. J Biol Chem. 2001;276(10):7549–7558. doi: 10.1074/jbc.M010242200.
- Mahmoodi MR, Najafipour H. Association of C-peptide and lipoprotein(a) as two predictors with cardiometabolic biomarkers in patients with type 2 diabetes in KERCADR population-based study. PLoS One. 2022;17(5):e0268927. doi: 10.1371/journal.pone.0268927.
- Muhanhali D, Zhai T, Cai Z, et al. Lipoprotein(a) concentration is associated with risk of type 2 diabetes and cardiovascular events in a Chinese population with very high cardiovascular risk. Endocrine. 2020;69(1):63–72. doi: 10.1007/s12020-020-02286-5.
- Gudbjartsson DF, Thorgeirsson G, Sulem P, et al. Lipoprotein(a) concentration and risks of cardiovascular disease and diabetes. J Am Coll Cardiol. 2019;74(24):2982–2994. doi: 10.1016/j.jacc.2019.10.019.
- Lamina C, Ward NC. Lipoprotein (a) and diabetes mellitus. Atherosclerosis. 2022;349:63–71. doi: 10.1016/j.atherosclerosis.2022.04.016.
- Kollerits B, Drechsler C, Krane V, et al. Lipoprotein(a) concentrations, apolipoprotein(a) isoforms and clinical endpoints in haemodialysis patients with type 2 diabetes mellitus: results from the 4D study. Nephrol Dial Transplant. 2016;31(11):1901–1908. doi: 10.1093/ndt/gfv428.
- Mu-Han-Ha-Li D-L-D-E, Zhai T-Y, Ling Y, et al. LPA kringle IV type 2 is associated with type 2 diabetes in a Chinese population with very high cardiovascular risk. J Lipid Res. 2018;59(5):884–891. doi: 10.1194/jlr.P082792.
- Singh SS, Rashid M, Lieverse AG, et al. Lipoprotein(a) plasma levels are not associated with incident microvascular complications in type 2 diabetes mellitus. Diabetologia. 2020;63(6):1248–1257. doi: 10.1007/s00125-020-05120-9.
- Rahimi M, Khanahmad H, Gharipour M, et al. Polymorphisms of LPA gene, rs1801693 and rs7765781, are not associated with premature myocardial infarction in the Iranian population. ARYA Atheroscler. 2021;17(5):1–8. doi: 10.22122/arya.v17i0.2369.
- Xu S, Cheng J, Li NH, et al. The association of APOC4 polymorphisms with premature coronary artery disease in a Chinese Han population. Lipids Health Dis. 2015;14:63. doi: 10.1186/s12944-015-0065-7.
- Linnér C, Svartberg J, Giwercman A, et al. Estrogen receptor alpha single nucleotide polymorphism as predictor of diabetes type 2 risk in hypogonadal men. Aging Male. 2013;16(2):52–57. doi: 10.3109/13685538.2013.772134.
- Yang C, Tang R, Ma X, et al. Tag SNPs in long non-coding RNA H19 contribute to susceptibility to gastric cancer in the Chinese Han population. Oncotarget. 2015;6(17):15311–15320. doi: 10.18632/oncotarget.3840.
- Lu W, Cheng YC, Chen K, et al. Evidence for several independent genetic variants affecting lipoprotein (a) cholesterol levels. Hum Mol Genet. 2015;24(8):2390–2400. doi: 10.1093/hmg/ddu731.
- Alberti KG, Zimmet PZ. Definition, diagnosis and classification of diabetes mellitus and its complications. Part 1: diagnosis and classification of diabetes mellitus provisional report of a WHO consultation. Diabet. Med. 1998;15(7):539–553. doi: 10.1002/(SICI)1096-9136(199807)15:7<539::AID-DIA668>3.0.CO;2-S.
- Wilson PW, D’Agostino RB, Levy D, et al. Prediction of coronary heart disease using risk factor categories. Circulation. 1998;97(18):1837–1847. doi: 10.1161/01.cir.97.18.1837.
- Luke MM, Kane JP, Liu DM, et al. A polymorphism in the protease-like domain of apolipoprotein(a) is associated with severe coronary artery disease. Arterioscler Thromb Vasc Biol. 2007;27(9):2030–2036. doi: 10.1161/ATVBAHA.107.141291.
- Chasman DI, Shiffman D, Zee RY, et al. Polymorphism in the apolipoprotein(a) gene, plasma lipoprotein(a), cardiovascular disease, and low-dose aspirin therapy. Atherosclerosis. 2009;203(2):371–376. doi: 10.1016/j.atherosclerosis.2008.07.019.
- Song ZK, Cao HY, Wu HD, et al. A case-control study of the relationship between SLC22A3-LPAL2-LPA gene cluster polymorphism and coronary artery disease in the Han Chinese population. Iran Red Crescent Med J. 2016;18(6):e35387. doi: 10.5812/ircmj.35387.
- García-Chapa EG, Leal-Ugarte E, Peralta-Leal V, et al. Genetic epidemiology of type 2 diabetes in Mexican Mestizos. Biomed Res Int. 2017;2017:3937893. doi: 10.1155/2017/3937893.
- Zhang Y, Zhou X, Dai W, et al. CTNNA3 genetic polymorphism may be a new genetic signal of type 2 diabetes in the Chinese Han population: a case control study. BMC Med Genomics. 2021;14(1):257. doi: 10.1186/s12920-021-01105-8.
- Cui J, Tong R, Xu J, et al. Association between STAT4 gene polymorphism and type 2 diabetes risk in Chinese Han population. BMC Med Genomics. 2021;14(1):169. doi: 10.1186/s12920-021-01000-2.
- Tarnowski M, Malinowski D, Safranow K, et al. MTNR1A and MTNR1B gene polymorphisms in women with gestational diabetes. Gynecol Endocrinol. 2017;33(5):395–398. doi: 10.1080/09513590.2016.1276556.
- Filus A, Trzmiel A, Kuliczkowska-Płaksej J, et al. Relationship between vitamin D receptor BsmI and FokI polymorphisms and anthropometric and biochemical parameters describing metabolic syndrome. Aging Male. 2008;11(3):134–139. doi: 10.1080/13685530802273426.
- Kanaya AM, Grady D, Barrett-Connor E. Explaining the sex difference in coronary heart disease mortality among patients with type 2 diabetes mellitus: a meta-analysis. Arch Intern Med. 2002;162(15):1737–1745. doi: 10.1001/archinte.162.15.1737.
- Tian Y, Xu J, Huang T, et al. A novel polymorphism (rs35612982) in CDKAL1 is a risk factor of type 2 diabetes: a case-control study. Kidney Blood Press Res. 2019;44(6):1313–1326. doi: 10.1159/000503175.
- Matharoo K, Arora P, Bhanwer AJ. Association of adiponectin (AdipoQ) and sulphonylurea receptor (ABCC8) gene polymorphisms with type 2 diabetes in North Indian population of Punjab. Gene. 2013;527(1):228–234. doi: 10.1016/j.gene.2013.05.075.
- Bragg F, Holmes MV, Iona A, et al. Association between diabetes and cause-specific mortality in rural and urban areas of China. Jama. 2017;317(3):280–289. doi: 10.1001/jama.2016.19720.
- Maeda T, Oyama J, Higuchi Y, et al. The correlation between clinical laboratory data and telomeric status of male patients with metabolic disorders and no clinical history of vascular events. Aging Male. 2011;14(1):21–26. doi: 10.3109/13685538.2010.502270.
- Jin H. Increased levels of glycosylated hemoglobin, microalbuminuria and serum cystatin C predict adverse outcomes in high-risk pregnancies with gestational diabetes mellitus. Exp Therap Med. 2020;19(2):1281–1287.