Abstract
Tumour progression locus-2 (Tpl2) is a serine/threonine kinase, which regulates the expression of tumour necrosis factor α. The article describes the development of a robust pharmacophore model and the investigation of structure-activity relationship analysis of quinoline-3-carbonitrile derivatives reported for Tpl2 kinase inhibition. A five point pharmacophore model (ADRRR) was developed and used to derive a predictive atom-based 3-dimensional quantitative structure activity relationship (3D-QSAR) model. The obtained 3D-QSAR model has an excellent correlation coefficient value (r2 = 0.96), Fisher ratio (F = 131.9) and exhibited good predictive power (q2 = 0.79). The QSAR model suggests that the inclusion of hydrophobic substituents will enhance the Tpl2 kinase inhibition. In addition, H-bond donating groups, negative ionic groups and electron withdrawing groups positively contribute to the Tpl2 kinase inhibition. Further, pharmacophoric model was validated by the receiver operating characteristic curve analysis and was employed for virtual screening to identify six potential Tpl2 kinase inhibitors. The findings of this study provide a set of guidelines for designing compounds with better Tpl2 kinase inhibitory potency.
Introduction
Tumour progression locus-2 (Tpl2) is a serine/threonine kinase in the mitogen-activated protein kinase signal transduction cascade. It functions as an upstream kinase of mitogen-activated protein kinase kinase/extracellular signal-regulated kinase (MEK/ERK) and MKK4/JNK signalling. This Tpl2-MEK-ERK signalling module is known to regulate inflammatory pathwaysCitation1–4. Notably, Tpl2 regulates the expression of tumour necrosis factor α (TNF-α), which is critical to the initiation and progression of many inflammatory disorders including rheumatoid arthritis, ankylosing spondylitis and psoriatic arthritisCitation4–8. Growing popularity of the Tpl2 kinase is reflected by the number of publications on the new Tpl2 kinase inhibitorsCitation9–13. The clinical success of TNF-α inhibitors (etanercept, infliximab and adalimumab) has validated the use of anti-TNF-α therapies for treating arthritic and inflammatory diseases and they have necessitated the search for small molecule inhibitors with similar or related mechanism of actionCitation14–17. Due to its physiological importance in cytokine signalling networks and its key role in the production of TNF-α, target specific, small molecule inhibitors of Tpl2 should constitute an effective therapy for TNF-α driven inflammatory disordersCitation14.
The exploratory studies on inhibition of Tpl2 demonstrated the importance of this protein. A series of Tpl2 inhibitors, developed by Wyeth research, have been evaluated for their ability to inhibit Tpl2-MEK-ERK-induced inflammation. Quinolin-3-carbonitril was identified as a potent and selective Tpl2 inhibitor in rheumatoid arthritisCitation18. Hall et al. demonstrated that a small molecule inhibitor of Tpl2 suppressed lipopolysaccharide (LPS) and IL-1β-induced production of TNF by human monocytes, as well as interleukin (IL)-1β-induced production of IL-6, IL-8, prostaglandin E2 and MMPsCitation19. Further, another quinoline-3-carbonitrile-type Tpl2 inhibitor was showed to inhibit the LPS-induced inflammation in ratsCitation20. Further, 15-Deoxy-Δ12, 14-prostaglandin J2 was demonstrated to be efficacious in completely inhibiting the Tpl2 activated by LPS or PaclitaxelCitation21.
Although small molecule inhibitors exist for the targeting of the Tpl2-MEK-ERK pathway, safety might be a serious issue with Tpl2 inhibitors. Until now, only limited number of compound libraries have been reported and a good descriptive quantitative structure-activity relationship (QSAR) analysis for Tpl2 kinase inhibitors is still lackingCitation18–24. However, the number of compound library needs to be increased to achieve structural diversity with more potent and less toxic compounds. Keeping the therapeutic significance of this class of inhibitors in mind and our continuing interest in the development of Tpl2 kinase inhibitors, a 3D-QSAR was proposed on the series of quinoline-3-carbonitrile derivatives reported for Tpl2 kinase inhibition by Hu et al.Citation18. The compounds reported are suitable for the purpose of 3D-QSAR modelling since they have a common scaffold and the biological assays have been carried out in the same laboratory.
3D-QSAR is widely used in the lead optimization stage and provides valuable insights about ligand–protein interactions. In order to establish a relationship between the spatial 3D pharmacophoric features of quinoline-3-carbonitrile-type Tpl2 kinase inhibitors and their activity, a 3D-QSAR analysis was carried out on the combined datasetCitation25–27.
Methods and materials
Data set
A set of 38 quinoline-3-carbonitrile derivatives with well-defined Tpl2 kinase inhibitory activity was used for the QSAR analysisCitation18. In vitro inhibitory concentrations (IC50) of the molecules against Tpl2 kinase were converted into corresponding pIC50 [-log(IC50)] and were used as dependent variables in the QSAR calculations ().
Table 1. Tpl2 kinase inhibition data of quinoline-3-carbonitrile and their analogues.
Molecular modelling
PHASE 3.0 module of the Schrodinger molecular modelling software was used to generate pharmacophore modelsCitation26,Citation27. In the QSAR studies, appropriate conformation (lowest energy) of the compound is required for accurate calculation of 3D descriptors. All the 38 compounds were sketched by Maestro. Further, geometry optimization was carried out using the semi empirical OPLS_2005 force field. All the molecules were divided into a training set (28) and a test set (11) to maintain the structure and activity diversity in both sets for the QSAR modelling and pharmacophore generation and validation.
PHASE incorporates a structure cleaning step utilizing LigPrep, which attaches hydrogens, converts 2D structures to 3D, generates stereoisomers and neutralizes charged structures or determines the most probable ionization state at a user-defined pH. It also allows for the importation of 3D structures prepared outside its own workflow. Because one does not generally know the structure that a given molecule will adopt if and when it binds to a target protein, it is customary to represent each molecule as a series of 3D structures that sample the thermally accessible conformational states. For purposes of pharmacophore model -development, PHASE provides two built-in approaches, both of which employ the MacroModel conformational search engineCitation27,Citation29. The first approach involves a rapid torsion angle search followed by minimization of each generated structure using OPLS_2005 force field, with implicit GB/SA or distance dependent dielectric solvent model. The torsion search sample’s ring conformations, invertible pseudo-chiral nitrogens and all rotatable bonds within a core region, which includes everything from the centre of a molecule out to, but not including, the last rotatable bond along each path. Torsion angles for these terminal groups are varied either one at-a-time or simultaneously, according to the user’s preference. As torsions are sampled, a truncated force field is applied to identify dihedral minima, and the overall energy of each structure is estimated by combining dihedral potentials with internal ring energies. Structures with high estimated energies are eliminated, as are structures with close non-bonded contacts.
As an alternative to the rapid torsion search, conformational space may be explored through a combination of Monte–Carlo multiple minimum (MCMM) sampling and low mode (LMOD) conformational searchingCitation27,Citation29. MC sampling provides excellent global coverage of the potential energy surface, while low mode searching facilitates effective treatment of local regions with complex and/or problematic characteristics, such as saddle points. Although more computationally demanding, the mixed MCMM/LMOD approach is among the most powerful and generally applicable conformational searching methods currently available.
In the present study, the mixed MCMM/LMOD approach was employed with a defined energetic window (10 kcal/mol) to increase the chances of finding the representative conformer close to the bound structure. The ligands were assigned as actives and inactives by giving an appropriate activity threshold value (pIC50). The threshold value for active ligands was set to 7, whereas it was 4.5 for inactive ligands (pharma set). The activity threshold value was selected on the basis of dataset activity distribution (4.389–8.523) and the active ligands were chosen to derive a set of suitable pharmacophores. The prepared ligands were used for generating common pharmacophore and QSAR model building.
Creation of pharmacophore sites
The chemical features of all ligands were defined by six pharmacophoric features: H-bond acceptor (A), H-bond donor (D), hydrophobic group (H), negatively charged group (N), positively charged group (P) and aromatic ring (R). An active analog approach was used to identify common pharmacophore hypotheses (CPHs), in which common pharmacophores were culled from the conformations of the set of active ligands using a tree-based partitioning technique that groups together similar pharmacophores according to their intersite distances. A tree depth of five with an initial box size of 25.6 Å and a final box size of 0.8 Å was usedCitation27.
Scoring pharmacophores with respect to actives and inactives
The resulting pharmacophores were then scored and ranked. The scoring was done to identify the best candidate hypothesis, which provided an overall ranking of all the hypotheses. The scoring algorithm included the contributions from the alignment of site points and vectors, volume overlap, selectivity, number of ligands matched, relative conformational energy and activityCitation29.
Perceiving common pharmacophores
After careful analysis of the scores and alignment of active ligands to the generated hypotheses, a best pharmacophore hypothesis ADRRR () was selected for further studies (). The selected 3D pharmacophore hypothesis encompassed the following features: one H-bond acceptor (A) (pink sphere with one arrow), one H-bond donor (D) (blue sphere with single arrow), three aromatic rings (R) (grey circle). The 2D representation of the pharmacophore ADRRR shows the carbonitrile group (pink sphere with one arrow) hydrogen bond acceptor (A), the secondary amine group depicting a blue sphere with a single arrow as H-bond donor (D) and rings R9, R10 and R11 as the key pharmacophoric elements present in the selected pharmacophore.
Figure 1. Common pharmacophore for active ligands (three aromatic rings (grey circle)), one H-donor (blue sphere with single arrow) and one acceptor (pink sphere with one arrow).
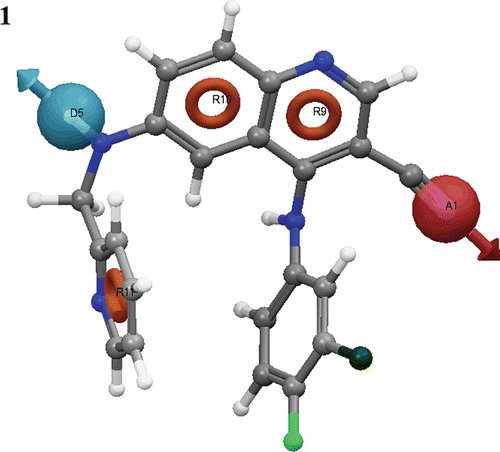
Table 2. The scores of different parameters of the ADRRR hypothesis.
Building 3D-QSAR models
QSAR modelling was carried out using the selected hypothesis by dividing the dataset into a training set (70%) and a test set (30%) in a random manner. PHASE presents two options for alignment of 3D structure of molecules; the pharmacophore based alignment and the atom-based alignmentCitation27,Citation28. In this study, we have used an atom-based QSAR model, which is more useful in explaining the structure-activity relationship. In atom-based QSAR, a molecule is treated as a set of overlapping van der Waals spheres. Each atom (and hence each sphere) is placed into one of six categories according to a simple set of rules: hydrogens attached to polar atoms are classified as hydrogen bond donors (D); carbons, halogens, and C–H hydrogens are classified as hydrophobic/non-polar (H); atoms with an explicit negative ionic charge are classified as negative ionic (N); atoms with an explicit positive ionic charge are classified as positive ionic (P); non-ionic nitrogens and oxygens are classified as electron withdrawing (W); and all other types of atoms are classified as miscellaneous (X).
Negative and positive ionizable sites were modelled as a single point situated on a formally charged atom, or at the centroid of a group of atoms over which the ionic charge was shared. Because most commonly occurring ionizable centres are automatically recognized by PHASE, the structures provided need not be ionized explicitlyCitation29.
For the purpose of QSAR development, van der Waals models of the aligned training set molecules were placed in a regular grid of cubes, with each cube allotted zero or more ‘bits’ to account for different types of atoms in the training set that occupy the cube. This representation gives rise to binary-valued occupation patterns that can be used as independent variables to create partial least-squares (PLS) QSAR models. Atom-based QSAR models were generated for the selected hypothesis using the 27-member training set using a grid spacing of 1.0 Å. The best QSAR model was validated by predicting activities of the 11 test set compounds. A four component (PLS factor) model with good statistics was obtained for the dataset whereas the maximum number of PLS factors in each model can be one-fifth of the total number of training set molecules. Further increase in the number of PLS factors did not improve the model statistics or predictive abilityCitation25.
Pharmacophore model validation
One efficient approach in drug discovery is the virtual screening of the molecular libraries. However, the developed model needs to be validated before going for virtual screening. The validation of the ADRRR model was carried out by the receiver operating characteristic (ROC) curve calculation. The ROC was calculated by screening 100 compound database containing 34 known inhibitorCitation24 and 66 decoys from the ADRRR model.
The ROC curves were used to measure the prediction rate of actives vs. inactives. Two variables, i.e., true positive fraction (sensitivity) and false positive fraction (1–specificity), were calculated and the graph was plotted. The ROC area is a single quantitative measure of the predictive ability and varies from 0.5 for random prediction to 1.0 for perfect predictionCitation30.
Database screening
The validated pharmacophore hypothesis (ADRRR) was used as a 3D query for screening Asinex database. The purpose of this screening was to retrieve novel and potential leads suitable for further development. Conformers were generated for each molecule in the database using PHASE. The database screening was carried out using PHASE virtual screening protocol implemented in Schrödinger with best/flexible search optionCitation29. The retrieved compounds were filtered by fitness value (can be maximum 3).
Asinex database was filtered through the common pharmacophore (ADRRR) using the following running conditions: (a) search in the conformers database, (b) do not score in place the conformers into the structure-based common pharmacophore and refine (i.e., allow reorientation of the conformers to determine if they match the pharmacophore or not), (c) match at least four out of the five sites of the quantitative common pharmacophore, (d) do not consider any site as mandatory and (e) do not prefer partial matches involving more sites. The rest of the options and parameters used during the search were the default values.
Results and discussion
The primary objective of the present study was to elucidate the 3D structural features of quinoline-3-carbonitrile-type Tpl2 kinase inhibitors crucial for binding, by generating an atom-based 3D-QSAR model. The generated pharmacophore hypothesis (ADRRR) conveyed the relative binding confirmation of the ligands to the active site. Therefore, the ADRRR hypothesis was used for generating a 3D-QSAR model to identify overall aspects of molecular structure of the ligands, which govern their Tpl2 inhibitory activity (pIC50).
For the generation of pharmacophore model, we have considered 9 compounds having activity >7 against Tpl2 kinase as active, as they contain important structural features crucial for binding to the active site. We have used 4 minimum sites and 5 maximum sites in order to have optimum combination of sites or features common to the most active compounds. A total of 626 common pharmacophore models were generated with different combination of variants (supplementary data). Among 626 pharmacophores, only those models which are showing superior alignment with the active compounds were identified by calculating the survival scoreCitation29. The survival scoring function of the PHASE module identifies the best candidate hypothesis from generated models and provides an overall ranking of all the hypotheses. The scoring algorithm includes contributions from the alignment of site points and vectors, volume overlap, selectivity, number of ligands matched, relative conformational energy and activity ()Citation29.
The PHASE module of Schrodinger has special feature to differentiate between the active (most active) and inactive (less active) molecules. To identify the pharmacophore models with more active and less inactive features, all models were mapped to inactive compounds and scored. If inactives score well, the hypothesis could be invalid because it does not discriminate between actives and inactives. Therefore, adjusted survival score was calculated by subtracting the inactive score from the survival score of these pharmacophores (supplementary data). Finally, the models with maximum adjusted survival score and lowest relative conformational energy were selected for generating pharmacophore (atom) based alignment of Tpl2 kinase inhibitors. Further, the ADRRR model was selected since it rendered the good predictive power over other models (). As depicted in the figure, out of three aromatic ring features, two were mapped to the quinoline ring of all nine active inhibitors. The other aromatic ring feature was mapped to the substituted phenyl group at the sixth position of quinoline ring. The H-bond donor feature was mapped to the NH group, substituted at the sixth position of quinoline ring. Further, the acceptor feature was mapped to the electron rich nitrogen of CN group, substituted at the third position of quinoline ring.
For generating an atom-based 3D-QSAR hypothesis, a dataset of 29 training-set compounds having inhibitory activity against Tpl2 kinase was used. The model was validated using 9 test-set compounds, which covered a wide range of Tpl2 kinase inhibitory activity. The alignment generated by the ADRRR hypothesis was used for QSAR model generation (). A four PLS factor model with good statistics and predictive ability was generated for the dataset (). The incremental increase in the statistical significance and predictivity was observed for each incremental increase in the incorporated PLS factors up to four (). The model expressed 96% variance exhibited by quinoline-3-carbonitrile derivatives, which was near to one, signifying a close agreement of fitting points on the regression line for the observed and PHASE predicted activity ( and ).
Table 3. PLS statistical parameters of the selected 3D-QSAR model.
Table 4. Fitness and predicted activity data for the test and training set of compounds.
Figure 4. Fitness graph between observed activity vs. Phase predicted activity for training and test set compounds.
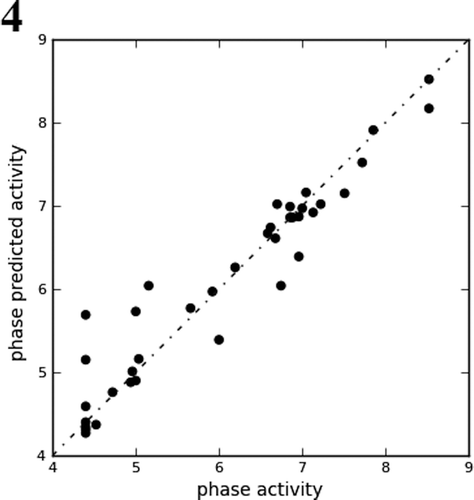
A 3D-QSAR analysis was performed on the series of quinoline-3-carbonitrile derivatives to understand the effect of spatial arrangement of structural features on their Tpl2 kinase inhibition. The large value of F (131.9) suggested a statistically significant regression model, which was further supported by the small value of the variance ratio (P), an indication of a high degree of confidence. The small value of standard deviation (0.28) and root-mean-square error (RMSE = 0.58) established that the data used for model generation was best for the QSAR analysis. The validity of the model was further demonstrated by the cross validated correlation coefficient (q2 = 0.79). The q2 is more reliable and robust statistical parameter than r2 because it is obtained by external validation (test set). depicted the good alignment of the active ligands and scattered alignment of the inactive ligands to the developed ADRRR model.
The contribution maps obtained from model ADRRR show how 3D-QSAR methods can identify features important for the interaction between ligands and their target protein. Such maps allow identification of those positions that require a particular physicochemical property to enhance the bioactivity of a ligand. The QSAR model displays 3D characteristics as cubes and the blue cubes indicate positive coefficients which are favourable while red cubes indicate negative coefficients which are unfavourable regions for activity (). This might give a clue to what functional groups are desirable or undesirable at certain positions in a molecule.
Figure 5. QSAR visualization of various substituents effect: (A) Electron withdrawing feature, (B) Hydrogen-bond donor, (C) Hydrophobic features, (D) Positive ionic and (E) Negative ionic.
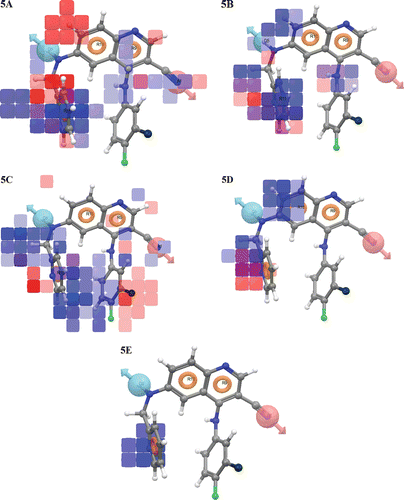
Visual analysis of the demonstrates that the throng of the blue cubes at the ring R11 and amino group in R9 ring site is pointing out the positive potential of electron withdrawing characteristic of the molecules and is prerequisite for the activity at this particular place. It can be suggested that the addition of appropriate electron withdrawing groups at the ring R11 and in R9 ring site will increase the Tpl2 kinase inhibition. However, the addition of electron withdrawing groups at ring R10 position will contribute to decreased receptor binding which in turn will result in lower potency of compounds since the red colour cubes exhibit negative effect of electron withdrawing group. depicts that H-donor characteristic is necessary at D5 (NH2 group), whereas the red cubes at ring R11 represent a negative potential for the compounds. It can be deduced from the that hydrophobic groups are well tolerated from D5 to R11 side (blue cubes), while the substitution of hydrophobic groups above ring R9 site (where benzene ring is positioned) are unfavourable (red cubes) or may hinder the binding of the molecules to the active site. Further, exhibits that the presence of positive ionic group at position R10 will have the positive effect (blue cubes) while it will contribute negatively (red cube) at R11 ring. However, the insertion of negative ionic group at ring R11 will increase the Tpl2 kinase inhibition ().
Virtual screening aims at the maximum enrichment of active compounds in a hit list. Therefore, such methods are usually validated by evaluating the accuracy of discrimination between actives and decoys. A good pharmacophore model will be able to distinguish a significant portion of known active compounds and as few decoys as possible. After QSAR modelling, our ADRRR hypothesis was used for the validation and virtual screening. Our model has detected 33 true positives and 65 true negatives with 98% accuracy and 98.5% specificity. A ROC curve is a true positive fraction vs. false positive fraction plot and the area under ROC was 0.98 which is near to one, gives accuracy of the model ( and ). Further, the validated ADRRR pharmacophore hypothesis was used as a 3D structural query for retrieving compounds from the Asinex database. The hits were selected based on their good fitness value to the pharmacophoric site and good predicted activity (pIC50). After considering all these factors, we selected six molecules as potential hits (), which can be further modified to increase their efficacy.
Table 5. The ROC and a few performance measures derived from the number of true positives, true negatives, false positives and false negatives by confusion matrix.
Figure 7. The structure of six potential Tpl2 kinase inhibitors with their Asinex ID, fitness value and predicted activity.
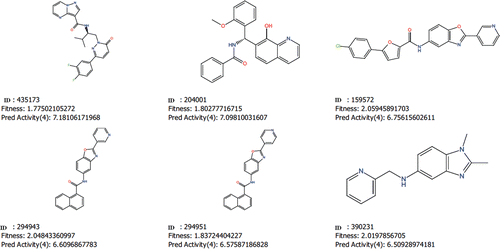
In summary, a ligand based pharmacophore model was generated for the series of quinoline-3-carbonitrile-type Tpl2 kinase inhibitors to reveal the structural features responsible for their biological activity. With the help of pharmacophore based alignment, a meaningful 3D-QSAR was derived to identify how three dimensional arrangements of various substituents will affect the Tpl2 kinase inhibition. The selected model as shown by the correlation statistics and predictive statistics is very much significant to draw unambiguous inferences. Further, the generated 3D-QSAR model also explains how and at what extent electron withdrawing, hydrophobic and H-donor moieties should be modified to achieve better Tpl2 inhibition. Finally, six potential hits with good fitness value and predicted activity were identified by virtual screening, whose activity can be further improved by the help of this QSAR model. The present study provides a set of guidelines which will greatly help in designing the newer and more potent quinoline-3-carbonitrile-type Tpl2 kinase inhibitors and novel scaffolds.
Supplementary Material
Download PDF (37.7 KB)Acknowledgement
We gratefully acknowledge Dr. Ravikumar Muttneni, Application Scientist, Schrodinger Inc., Bangalore, India for his valuable suggestions to carry out this work.
Declaration of interest
The authors report no conflicts of interest.
References
- Das S, Cho J, Lambertz I, Kelliher MA, Eliopoulos AG, Du K et al. Tpl2/cot signals activate ERK, JNK, and NF-kappaB in a cell-type and stimulus-specific manner. J Biol Chem 2005;280:23748–23757.
- Dumitru CD, Ceci JD, Tsatsanis C, Kontoyiannis D, Stamatakis K, Lin JH et al. TNF-alpha induction by LPS is regulated posttranscriptionally via a Tpl2/ERK-dependent pathway. Cell 2000;103:1071–1083.
- Eliopoulos AG, Dumitru CD, Wang CC, Cho J, Tsichlis PN. Induction of COX-2 by LPS in macrophages is regulated by Tpl2-dependent CREB activation signals. Embo J 2002;21:4831–4840.
- Eliopoulos AG, Wang CC, Dumitru CD, Tsichlis PN. Tpl2 transduces CD40 and TNF signals that activate ERK and regulates IgE induction by CD40. Embo J 2003;22:3855–3864.
- Kontoyiannis D, Boulougouris G, Manoloukos M, Armaka M, Apostolaki M, Pizarro T et al. Genetic dissection of the cellular pathways and signaling mechanisms in modeled tumor necrosis factor-induced Crohn’s-like inflammatory bowel disease. J Exp Med 2002;196:1563–1574.
- Rodríguez C, Pozo M, Nieto E, Fernández M, Alemany S. TRAF6 and Src kinase activity regulates Cot activation by IL-1. Cell Signal 2006;18:1376–1385.
- Stafford MJ, Morrice NA, Peggie MW, Cohen P. Interleukin-1 stimulated activation of the COT catalytic subunit through the phosphorylation of Thr290 and Ser62. FEBS Lett 2006;580:4010–4014.
- Sugimoto K, Ohata M, Miyoshi J, Ishizaki H, Tsuboi N, Masuda A et al. A serine/threonine kinase, Cot/Tpl2, modulates bacterial DNA-induced IL-12 production and Th cell differentiation. J Clin Invest 2004;114:857–866.
- Choy EH, Panayi GS. Cytokine pathways and joint inflammation in rheumatoid arthritis. N Engl J Med 2001;344:907–916.
- Aggarwal BB, Samanta A, Feldmann MA. Compendium of Cytokines and Other Mediators of Host Defense. Academic Press, San Diego 2001: pp. 413–434.
- Feldmann M, Maini RN. Anti-TNF alpha therapy of rheumatoid arthritis: What have we learned? Annu Rev Immunol 2001;19:163–196.
- Schottelius AJ, Moldawer LL, Dinarello CA, Asadullah K, Sterry W, Edwards CK 3rd. Biology of tumor necrosis factor-alpha- implications for psoriasis. Exp Dermatol 2004;13:193–222.
- Simmons DL. What makes a good anti-inflammatory drug target? Drug Discov Today 2006;11:210–219.
- Doran MF, Pond GR, Crowson CS, O’Fallon WM, Gabriel SE. Trends in incidence and mortality in rheumatoid arthritis in Rochester, Minnesota, over a forty-year period. Arthritis Rheum 2002;46:625–631.
- Smyth A, Houlihan DD, Tuite H, Fleming C, O’Gorman TA. Necrotising fasciitis of the shoulder in association with rheumatoid arthritis treated with etanercept: A case report. J Med Case Reports 2010;4:367.
- Gutierrez M, Becciolini A, Bertolazzi C, Di Geso L, Tardella M, Ariani A et al. [Polyarthritis flare in patient with ankylosing spondylitis treated with infliximab]. Reumatismo 2010;62:221–224.
- Shenoi S, Wallace CA. Tumor necrosis factor inhibitors in the management of juvenile idiopathic arthritis: An evidence-based review. Paediatr Drugs 2010;12:367–377.
- Hu Y, Green N, Gavrin LK, Janz K, Kaila N, Li HQ et al. Inhibition of Tpl2 kinase and TNFalpha production with quinoline-3-carbonitriles for the treatment of rheumatoid arthritis. Bioorg Med Chem Lett 2006;16:6067–6072.
- Hall JP, Kurdi Y, Hsu S, Cuozzo J, Liu J, Telliez JB et al. Pharmacologic inhibition of tpl2 blocks inflammatory responses in primary human monocytes, synoviocytes, and blood. J Biol Chem 2007;282:33295–33304.
- Green N, Hu Y, Janz K, Li HQ, Kaila N, Guler S et al. Inhibitors of tumor progression loci-2 (Tpl2) kinase and tumor necrosis factor alpha (TNF-alpha) production: Selectivity and in vivo antiinflammatory activity of novel 8-substituted-4-anilino-6-aminoquinoline-3-carbonitriles. J Med Chem 2007;50:4728–4745.
- Caivano M, Rodriguez C, Cohen P, Alemany S. 15-Deoxy-Delta12,14-prostaglandin J2 regulates endogenous Cot MAPK kinase kinase 1 activity induced by lipopolysaccharide. J Biol Chem 2003;278:52124–52130.
- Gavrin LK, Green N, Hu Y, Janz K, Kaila N, Li HQ et al. Inhibition of Tpl2 kinase and TNF-alpha production with 1,7-naphthyridine-3-carbonitriles: Synthesis and structure-activity relationships. Bioorg Med Chem Lett 2005;15:5288–5292.
- Kaila N, Green N, Li HQ, Hu Y, Janz K, Gavrin LK et al. Identification of a novel class of selective Tpl2 kinase inhibitors: 4-Alkylamino-[1,7]naphthyridine-3-carbonitriles. Bioorg Med Chem 2007;15:6425–6442.
- Wu J, Green N, Hotchandani R, Hu Y, Condon J, Huang A et al. Selective inhibitors of tumor progression loci-2 (Tpl2) kinase with potent inhibition of TNF-alpha production in human whole blood. Bioorg Med Chem Lett 2009;19:3485–3488.
- Mahipal Tanwar, OP, Karthikeyan C, Moorthy NS, Trivedi P. 3D QSAR of Aminophenyl Benzamide Derivatives as Histone Deacetylase Inhibitors. Med Chem 2010;6:287–285.
- Samantha L, Cesare M, Aleksey K, Mattia S, Stefano M, Elena C, Dorotea R, Alessandro C. SAR and QSAR study on 2-aminothiazole derivatives, modulators of transcriptional repression in Huntington’s disease. Bioorg Med Chem Lett 2008;16:5695–5703.
- User manual Phase, version 3.0, Schrödinger, LLC, New York, NY, 2008.
- Shah UA, Deokar HS, Kadam SS, Kulkarni VM. Pharmacophore generation and atom-based 3D-QSAR of novel 2-(4-methylsulfonylphenyl)pyrimidines as COX-2 inhibitors. Mol Divers 2010;14:559–568.
- Dixon SL, Smondyrev AM, Knoll EH, Rao SN, Shaw DE, Friesner RA. PHASE: A new engine for pharmacophore perception, 3D QSAR model development, and 3D database screening: 1. Methodology and preliminary results. J Comput Aided Mol Des 2006;20:647–671.
- Eng J. Receiver operating characteristic analysis: A primer. Acad Radiol 2005;12:909–916.