ABSTRACT
The present article aimed to explore how the development of reading comprehension is affected when its cognitive basis is compromised. The simple view of reading was adopted as the theoretical framework. The study followed 76 children with mild intellectual disabilities (average IQ = 60.38, age 121 months) across a period of 3 years. The children were assessed for level of reading comprehension (outcome variable) and its precursors decoding and listening comprehension, in addition to linguistic skills (foundational literacy skills, rapid naming, phonological short-term memory, verbal working memory, vocabulary, and grammar) and non-linguistic skills (nonverbal reasoning and temporal processing). Reading comprehension was predicted by decoding and listening comprehension but also by foundational literacy skills and nonverbal reasoning. It is concluded that intellectual disabilities can affect the development of reading comprehension indirectly via linguistic skills but also directly via nonlinguistic nonverbal reasoning ability.
According to the simple view of reading, reading comprehension depends on the strength of two core skills: word decoding and listening comprehension (Hoover & Gough, Citation1990). Their relative contributions to reading comprehension have been found to change over grades (Ouellette & Beers, Citation2010; Vellutino, Tunmer, Jaccard, & Chen, Citation2007). Early reading comprehension is limited by what the child is able to decode, and word decoding is the dominant predictor for reading comprehension at this stage. Once word decoding skill is developed sufficiently, the level of reading comprehension will be more strongly linked to the level of listening comprehension (Gough & Tunmer, Citation1986; Landi, Frost, Mencl, Sandak, & Pugh, Citation2013). It is known that a limited general intelligence can severely affect the acquisition of decoding skill and reading fluency (Lemons et al., Citation2013), but its influence on the developmental pattern of reading comprehension is far from clear. The role of general cognitive ability in typical populations can be better understood while examining the development of reading comprehension in children with significant limitations in general intelligence. The present article uses a longitudinal design to investigate the development of reading comprehension and its precursors in a group of children with intellectual disabilities.
Precursors of reading comprehension
Within the simple view framework, longitudinal studies have shown a relationship between linguistic precursors in early primary school and reading comprehension in later primary school (Catts, Herrera, Nielsen, & Bridges, Citation2015; Muter, Hulme, Snowling, & Stevenson, Citation2004). To read in an alphabetic language, children must first be aware of the alphabetic principle; they must understand the phonological structure of the language and be able to match letters to their corresponding speech sounds. Phonological awareness and letter knowledge can thus be considered foundational literacy skills (Liberman, Shankweiler, & Liberman, Citation1989; Melby-Lervåg, Lyster, & Hulme, Citation2012). On top of such skills, rapid automatized naming (RAN), which reflects fluency in lexical retrieval, can be seen as an important predictor of decoding fluency (Moll et al., Citation2014; Norton & Wolf, Citation2012). Moreover, children’s vocabulary may facilitate word decoding in two ways: (a) bottom-up, because the phonological specificity of words increases as vocabulary grows, thus promoting phonological awareness, and (b) top-down, by facilitating the recognition of familiar words (Language and Reading Research Consortium, Citation2015; Muter et al., Citation2004; Perfetti & Hart, Citation2002).
Furthermore, listening comprehension involves the matching of words to plausible concepts and the subsequent integration of these concepts into a meaningful message. Listening comprehension thus requires not only word knowledge but also the understanding of spoken language, and is typically predicted by prior vocabulary and grammatical knowledge (Foorman, Herrera, Petscher, Mitchell, & Truckenmiller, Citation2015; Language and Reading Research Consortium, Citation2015).
In addition, several linguistic processing skills are necessary for the interpretation of literacy-related information. For example, phonological short-term memory is needed in early decoding to blend sequences of phonemes into words (Perez, Majerus, & Poncelet, Citation2012). Verbal working memory is known as a longitudinal precursor for word decoding but also for reading comprehension due to its role in word-to-text integration, drawing inferences, and implementing reading strategies (Cain, Oakhill, & Bryant, Citation2004; Moll et al., Citation2014; Seigneuric & Ehrlich, Citation2005). Higher level language comprehension skills, such as verbal inference making and comprehension monitoring, have been found to be strongly related to, and largely mediated by, listening comprehension (Kim, Citation2017; Oakhill & Cain, Citation2012).
Finally, a number of studies have addressed the influence of nonlinguistic abilities on the development of word decoding, listening comprehension, and reading comprehension. Abstract reasoning ability is used to complete gaps and solve ambiguities in the text. Nonverbal reasoning has been found to be a unique predictor of reading comprehension in children with typical development (Asbell, Donders, Van Tubbergen, & Warschausky, Citation2010) and in poor readers (Fuchs et al., Citation2012). The contribution of reasoning ability seems to be stronger in the higher levels of primary school when the call for inference making increases (Ribeiro, Cadime, Freitas, & Viana, Citation2016; Tighe & Schatschneider, Citation2014). In other studies, auditory temporal processing, often operationalized as rhythm perception, was related to decoding fluency (Huss, Verney, Fokser, Mead, & Goswami, Citation2011), and to grammar skills (Gordon, Jacobs, Schuele, & McAuley, Citation2015). Sensitivity for speech prosody, which involves sensitivity for rhythm, has also been connected to reading comprehension directly (Choi, Tong, & Cain, Citation2016; Miller & Schwanenflugel, Citation2008).
The role of general cognitive ability
A person’s general cognitive ability is reflected in learning ability, as well as working memory capacity and problem solving. Some studies have found only indirect relationships between cognitive abilities and reading comprehension through decoding and listening comprehension (Gustafson, Samuelsson, Johansson, & Wallmann, Citation2013; Kim, Citation2017). Other studies report a direct influence of precursors such as memory and reasoning skill on reading comprehension over and above the role of linguistic skills, albeit often in the higher grades of primary education (Cain et al., Citation2004; Oakhill & Cain, Citation2012; Ribeiro et al., Citation2016). The unique influence of cognitive abilities on reading comprehension may be more difficult to recognize in early readers than in older readers. More insight into the role of general cognitive ability can be gained by examining how the development of early reading comprehension is affected in children with intellectual disabilities.
Children with intellectual disabilities are known to experience memory deficits, reasoning deficits, and information-processing deficits (Bonifacci & Snowling, Citation2008; Goharpey, Crewther, & Crewther, Citation2013; Schuchardt, Gebhardt, & Mäehler, Citation2010). These children also show severe deficits with regard to decoding and reading fluency (Lemons et al., Citation2013). The precursors of decoding for children with intellectual disbilities correspond to those for children showing typical development (foundational literacy skills, RAN, and phonological short-term memory; Soltani & Roslan, Citation2013; Wise, Sevcik, Romski, & Morris, Citation2010). Nonverbal intelligence and temporal processing have also been found to predict decoding and foundational literacy skills in young children with intellectual disabilities (Van Tilborg, Segers, Van Balkom, & Verhoeven, Citation2014). Regarding reading comprehension, in a cross-sectional study of children with intellectual disabilities and decoding skills at Grade 1 level, decoding was the main precursor of reading comprehension after control for nonverbal reasoning (Van Wingerden, Segers, Van Balkom, & Verhoeven, Citation2014). In addition, a longitudinal study of children with intellectual disabilities found that foundational literacy skills were predictive of reading comprehension after control for IQ, age, vocabulary, prior reading comprehension, primary language, and the type of school attended. Decoding was not included as a predictor in this analysis (Dessemontet & De Chambrier, Citation2015). Furthermore, the reading comprehension of adolescents with Down Syndrome who had well-developed decoding skills was predicted by their level of listening comprehension (Nash & Heath, Citation2011; Roch, Florit, & Levorato, Citation2011). Finally, in a longitudinal study concerned with linguistic and nonlinguistic precursors for reading comprehension in adolescents with borderline intellectual functioning, several factors proved predictive next to decoding: attention, self-monitoring, and visuospatial working memory (Antshel, Hier, Fremont, Faraone, & Kates, Citation2014).
In sum, the precursors of reading comprehension in children with intellectual disabilities appear to correspond to the simple view of reading and its typical linguistic precursors. However, the few available studies concerning this population have primarily addressed the linguistic aspects of reading comprehension. Adding to this literature would also add in the understanding of the prerequisites for reading comprehension in the typical population.
Therefore, a cross-lagged longitudinal study was conducted to investigate the influence of intellectual disabilities on the development of reading comprehension and the respective roles of its linguistic and non-linguistic precursors. As part of the 3-year longitudinal study, the data from Wave 1 have been reported in Van Wingerden, Segers, Van Balkom, and Verhoeven (Citation2017). Wave 1 included 81 children with mild intellectual disability for whom the level of reading comprehension, word decoding, and listening comprehension was related to linguistic precursors (foundational literacy skills, RAN, vocabulary, grammar, phonological short-term memory, verbal working memory), and two plausible underlying nonlinguistic precursors (temporal processing, nonverbal reasoning). Reading comprehension in Wave 1 was associated with decoding, listening comprehension, foundational literacy skills, and temporal processing.
In the present article, the same variables were used including follow-up data on word decoding, listening comprehension, and reading comprehension 1 and 2 years later. Linguistic and nonlinguistic variables from Wave 1, together with decoding and listening comprehension from Wave 1 and 2, were used to construct a longitudinal model for the prediction of reading comprehension in Wave 2 and 3. Our basic research question was: Does the simple view of reading hold for children with intellectual disabilities, or do linguistic or nonlinguistic precursor variables need to be incorporated? We expected nonlinguistic precursors, particularly nonverbal reasoning, to be an additional predictor of the development of reading comprehension on top of children’s word decoding and listening comprehension.
Method
Participants
The study was conducted in 20 Dutch schools for special education. In the Netherlands, children are referred to these schools when their estimated IQ is below 70 points and they encounter both social and academic problems. Within these schools, children with an estimated IQ above 50 points were included to ensure that at least some capacity to read was present. Children were selected from the upper levels of primary school because previous studies have shown a relatively steep increase in decoding skill for children with intellectual disabilities between Grades 4 and 6, compared to their progress before and after that period (Lemons et al., Citation2013). Further inclusion criteria were an age between 108 and 138 months at Wave 1, normal hearing, eyesight that was normal or corrected to normal, and a monolingual Dutch-speaking home environment. There were no requirements with regard to initial reading level, and there was no referral rule about aetiology of the ID. No genetic syndromes were present in more than three of the children in the sample. The current sample can therefore be considered to be of mixed aetiologies.
The participants were tested on three occasions, with 1 year in between. At Wave 1, 81 participants (29 girls, 52 boys; MIQ = 60.38, SDIQ = 7.20) were tested with a mean age of 121 months (SD = 5.83). At Wave 3, 76 participants (94%) were still included (28 girls, 48 boys; MIQ = 60.51, SDIQ = 7.58) with a mean age of 145 months (SD = 5.80). The participation of three children was ended due to a change of schools; two children withdrew because of health reasons.
Measures
Reading comprehension, decoding, and listening comprehension were measured at Waves 1, 2, and 3. The remaining linguistic and nonlinguistic precursors were measured only at Wave 1. Unless specified otherwise, the reported test score is the number of correct responses on a given task.
Simple view related measures
Reading comprehension was the outcome variable. On all measurements, the test consisted of eight short stories with three multiple-choice questions appropriate for the first grade of regular education. Four of the stories focused on the use of anaphoric references to communicate explicit story content (from Verhoeven, Citation1992). The other four stories focused on the drawing of inferences from information in the text (from Aarnoutse, Citation1997). Questions concerned sentence meaning, explaining an event in the story, or the main topic of the story. Cronbach’s alphas as calculated at Wave 1 were .85 for the anaphora task and .75 for the inferences task.
Decoding was measured using word decoding and pseudoword decoding tasks. The word decoding task consisted of two word lists that contained 120 and 150 words, respectively. The second word list contained words of greater orthographic complexity than the first list. Participants read as many words as possible in 1 min from each card (Krom & Jongen, Citation2009). The Cronbach’s alpha is .86 and .94 for the two lists (Krom, Jongen, Verhelst, Kamphuis, & Kleintjes, Citation2010). The pseudoword decoding task involved a similar procedure except that lists of pseudowords instead of existing words were used and participants were given 2 min for each list. The Cronbach’s alpha is .85 for the two lists combined (Verhoeven, Citation2005).
Listening comprehension comprised three short stories (intended for 9- to 10-year-old children) that were read to the participants (Clinical Evaluation of Language Fundamentals–Dutch version; Kort, Compaan, Schittekatte, & Dekker, Citation2008). After each story, the child responded to five open-ended questions that either pertained directly to the content of the story or required additional reasoning. Cronbach’s alpha is .71 (Egberink, Vermeulen, & Frima, Citation2014).
Linguistic precursor measures
Foundational literacy skills were measured using a computer test (Vloedgraven, Keuning, & Verhoeven, Citation2009). Four competences were assessed: rhyme, blending, deletion, and sound-to-letter knowledge. In three tests, one out of three pictures had to be selected. For rhyme, participants selected the picture representing a word rhyming with an auditory stimulus. In the blending task, participants heard a string of phonemes that spelled the target word. In the deletion task, participants heard a word of which they had to delete a certain phoneme, which resulted in a new word. In the sound-to-letter task, participants had to select one out of four letters or digraphs corresponding to an auditory stimulus. The overall Cronbach’s alpha for the tasks combined is .78 (Egberink et al., Citation2014).
RAN included five different line drawings of familiar objects, presented in random order in four columns of 30 items viewed on a single page (Verhoeven, Citation2005). The participants named as many of the pictures in the order presented as possible in 1 min. The Cronbach’s alpha is .95 (Verhoeven, Citation2005).
Vocabulary was tested using the Dutch version of the PPVT (Schlichting, Citation2005). During each trial, the participant chose one out of four pictures that best represented a verbally presented word. Item difficulty increased with each set of 12 stimuli. The test was terminated after nine or more incorrect responses within a single set. The Lambda-2-coefficients for this test range from .93 to .97 (Schlichting, Citation2005).
Grammar comprehension was tested using a multiple-choice task involving spoken sentences that required understanding of meaning relations within sentences. Participants responded by selecting one of four images that best depicted the meaning of a sentence (Verhoeven & Vermeer, Citation2001). The Cronbach’s alpha is .81 (Verhoeven & Vermeer, Citation2006).
Phonological short-term memory was measured using pseudowords that were prerecorded and played one by one on a computer. The participant was asked to repeat each pseudoword (Verhoeven, Citation2005). The phonemic complexity of the pseudowords increased (from stult to bomarkietpartant). The test was terminated when five consecutive incorrect responses occurred. The Cronbach’s alpha is .94 (Verhoeven, Citation2005).
Verbal working memory was tested using a Digit Span test (Kort et al., Citation2005). Participants repeated series of digits increasing in length from two to nine items. Two series were included for each length. The test was terminated when the response for both series of a particular length was incorrect. First, the digits were repeated in the same order to measure verbal short-term memory, later the order had to be reversed which calls for an executive working memory component. The Cronbach’s alpha is .64 (Kort et al., Citation2005).
Nonlinguistic precursor measures
Nonverbal reasoning was tested using Raven’s Coloured Progressive Matrices (Raven, Citation1958). Participants chose one of six puzzle pieces to best complete a larger visual pattern. The split-half reliabilities in Dutch children vary from .82 to .87 (Van Bon, Citation1986; the stability of the norms for this test has been demonstrated by Cotton et al., Citation2005).
Temporal processing was measured using the Rhythm Test (Van Uden, Citation1983). Participants repeated rhythms of increasing complexity by tapping on the table with a pencil. They repeated each rhythm twice immediately after demonstration and then five times without further demonstration. The accuracy of the repetition was rated. The test continued until the participant failed both attempts at direct imitation for a particular rhythm. Cronbach’s alpha is .99 (Van Tilborg et al., Citation2014).
Procedure
The research was ethically approved by the National Board of Special Schools for children who are deaf or have other disabilities that hinder communication. All parents of the children involved in the study signed the required informed consent form. Participants were tested in 3 successive years, with 1 year intervening each measurement occasion. On all occasions, the participants were tested during school hours in a separate room.
Data analysis
Only those children participating in all three waves were included in the present analyses. Missing scores (< 0.01% in total) were estimated on the basis of previous or later scores from similar tasks coming from the larger longitudinal data set using multiple imputation. The pooled data set was used for further analysis (see also Van Wingerden et al., Citation2017). Z-scores were calculated for all variables to adjust for differences in the scales and distributions across variables.
To reduce the number of predictor variables in our analyses, we created composite scores based on confirmatory factor analyses by adding the Z-scores of related measures. Reading comprehension was composed of anaphoric and inferential reading comprehension; the variable foundational literacy skills was composed of rhyme, blending, deletion and sound-to-letter knowledge; and decoding was composed of word decoding and pseudoword decoding. The composite scores were then treated as measured variables in further analysis. Skewness (between −0.88 and 1.02) and kurtosis (between −1.28 and 1.06) were acceptable for all variables with the exception of temporal processing (skewness = 2.20, kurtosis = 5.45). A log transformation for this variable did not substantially alter the results of further analysis. In light of the consistency across variables we therefore report our findings on the untransformed data.
Decoding and listening comprehension were considered precursor variables for Wave 3 reading comprehension. Only the data from Waves 1 and 2 were therefore included in the analyses for these variables. In a similar vein, reading comprehension was considered the outcome variable, and only the reading comprehension scores for Waves 2 and 3 were included in further analyses. Pearson correlations, stepwise multiple linear regression analyses, and forward-selection linear regression analyses were conducted to identify significant predictors of reading comprehension, decoding, and listening comprehension at Wave 2 and 3. Structural equation modeling analyses were conducted in LISREL 8.80 to further evaluate the prediction of reading comprehension by the various precursors. Given the sample size, the complexity of the model was reduced by using composite scores instead of latent variables to model relationships between observed variables. Model fit was evaluated using six criteria: the χ2:df ratio should be lower than 2:1; the chi-square significance exceeding .05; the normed fit index, comparative fit index, goodness of fit index exceeding .95; and the root mean square error of approximation below .08 (Hooper, Coughlan, & Mullen, Citation2008).
Results
Descriptives
The means and standard deviations for all of the noncomposite scores are displayed in . Prior to answering our research question, we looked at the average levels of reading comprehension, word decoding, and listening comprehension in each year, because the skill level of decoding and listening comprehension determine their relative contributions to reading comprehension.
Table 1. Descriptive Statistics for Three Measurement Occasions
To evaluate the levels of reading comprehension, the following formula was used: chance level = np + (n – np)p, where n is the total number of items in the two reading comprehension tasks and p is the chance of randomly selecting the correct answer on any item. The percentage of participants that scored above chance level on the reading comprehension test was 40% at the time of Wave 1, 57.9% at Wave 2, and 67.1% at Wave 3. The scores for reading comprehension at Wave 1 were not included in further analysis. One-sample t tests showed that the average group performance was significantly above chance level at Wave 2, t(75) = 3.30, p = .002, Cohen’s d = 0.38, and at Wave 3, t(75) = 4.53, p < .001, Cohen’s d = 0.52. The increase in reading comprehension between Wave 2 and 3 was significant although the effect is small, t(75) = 2.27, p = .026, Cohen’s d = 0.17.
Regarding decoding, at the time of Wave 1, 48.7% of all participants were able to decode at or above a Grade 1 level according to the standards of the test (Jongen & Krom, Citation2009). At Wave 2, this percentage was 59.2% and at Wave 3 the percentage had increased to 71.1%. Dependent samples t tests revealed a significant increase in the raw scores from Wave 1 to Wave 2 for both word decoding, t(75) = 8.55, p < .001, Cohen’s d = 0.37, and pseudoword decoding, t(75) = 5.19, p < .001, Cohen’s d = 0.26. The increase from Wave 2 to Wave 3 was also significant for word decoding, t(75) = 7.76, p < .001, Cohen’s d = 0.31, and pseudoword decoding, t(75) = 6.13, p < .001, Cohen’s d = 0.27.
For listening comprehension, the test provided norms only for children ages 9 and 10 (Kort et al., Citation2008). However, at Wave 1 the average performance level on vocabulary was also known. Vocabulary was equivalent to that of of typically developing children age 6 (Schlichting, Citation2005). On listening comprehension at Wave 1, 50% of the group scored below the fifth percentile for 9-year-olds. At Wave 2, 50% of the group scored below the ninth percentile for children age 9. At Wave 3, 50% of the group scored below the 25th percentile compared to the norm for this age. The group average differed significantly between Wave 1 and 2, t(75) = 4.32, p < .001, Cohen’s d = 0.30, and from Wave 2 to Wave 3, t(75) = 3.30, p < .001, Cohen’s d = 0.23.
Correlations
Pearson correlations between precursor and outcome measures were computed while adopting a significance level of .01. presents a summary of the correlations between Wave 1 precursor variables and Wave 2 and 3 outcome variables. Reading comprehension at Wave 3 was moderately to strongly correlated with all precursor variables except for temporal processing. For Waves 2 and 3, strong correlations were observed for the outcome variables with their autoregressors. Decoding at Wave 2 correlated moderately with foundational literacy skills, RAN, and verbal working memory. Listening comprehension at Wave 2 correlated moderately with vocabulary and grammar.
Table 2. Correlations Between Predictor Variables at Wave 1 and Outcome Variables at Waves 2 and 3
Regressions
Next, we tested more explicitly whether the simple view of reading applied in this group of children. Two multiple regression analyses were performed with reading comprehension (at Waves 2 and 3) as the outcome variable, and decoding and listening comprehension (at Waves 1 and 2) as predictors. Results showed Wave 1 decoding (β = .60, p < .001) and listening comprehension (β = .18, p = .048) to predict reading comprehension at Wave 2, R2adj. = .44, F(2, 73) = 30.22, p < .001. Wave 2 decoding (β = .58, p < .001) and listening comprehension (β = .40, p < .001), similarly predicted reading comprehension at Wave 3 significantly, R2adj. = .56, F(2, 73) = 49.39, p < .001. For both Wave 2 and Wave 3 reading comprehension, decoding and listening comprehension in the previous wave were significant predictors.
Additional stepwise multiple regression analyses were conducted to identify any variables playing a role in predicting Wave 3 reading comprehension, in addition to Wave 2 decoding and listening comprehension. In the first step of each analysis, the Wave 2 autoregressor for reading comprehension was entered into the model (β = .78, p < .001), R2adj. = .61, F(1, 74) = 117.78, p < .001. In the second step, Wave 2 decoding (β = .24, p = .011) and Wave 2 listening comprehension (β = .28, p < .001) were added, R2adj. = .69, ΔR2 = .09, ΔF(2, 72) = 10.61, p < .001. In the third step, one of the Wave 1 precursor variables was entered into the model. Variables that significantly explained additional variance at this step in the regression analyses were foundational literacy skills (β = .32, p = .001), R2adj. = .73, ΔR2 = .05, ΔF(1, 71) = 12.84, p = .001; grammar (β = .28, p < .001), R2adj. = .75, ΔR2 = .06, ΔF(1, 71) = 17.38, p = .003; verbal working memory (β = .18, p = .033), R2adj. = .71, ΔR2 = .02, ΔF(1, 71) = 4.72, p < .001; and nonverbal reasoning (β = .88, R2adj. = .75, ΔR2 = .06), ΔF(1, 71) = 56.39, p < .001. In a final analysis, the four preceding variables were all entered in the third step of the regression together. A forward selection procedure was used to examine which of the four variables from Wave 1 contribute the most to predicting reading comprehension in Wave 3. After again controlling for the autoregressor and for Wave 2 decoding and listening comprehension, Wave 3 reading comprehension was further significantly predicted by Wave 1 foundational literacy skills (β = .23, p = .014) and nonverbal reasoning (β = .22, p = .002), R2adj. = .77, ΔR2 = .08, F(2, 70) = 12053, p < .001.
In addition, the main predictors for reading comprehension, decoding, and listening comprehension at Wave 2 were identified. To this end, all precursor variables from Wave 1 were entered into a forward selection linear regression for each Wave 2 outcome variable separately. For reading comprehension at Wave 2 the predictors were foundational literacy skills (β = .33, p = .002), R2adj. = .37, F(2, 73) = 22.97, p < .001, decoding (β = .37, p < .001), and grammar (β = .23, p = .025, R2adj. = .61), F(3, 72) = 39.35, p < .001. The final model for decoding at Wave 2 included foundational literacy skills (β = .27, p = .001) and RAN (β = .33, p = .002, R2adj. = .37), F(2, 73) = 22.97, p < .001. However, when the autoregressor was taken into account, decoding at Wave 1 was the only significant predictor for decoding at Wave 2 (β = .91, p < .001), R2adj. = .88, ΔR2 = .50, ΔF(1, 72) = 308.88, p < .001. Listening comprehension at Wave 2 was predicted only by vocabulary (β = .66, p < .001), R2adj. = .42, F(1, 74) = 56.64, p < .001. Inclusion of the autoregressor added significantly to the model (β = .67, p < .001), R2adj. = .69, ΔR2 = .27, ΔF(1, 73) = 65.53, p < .001, whereas vocabulary continued to play a significant role.
Structural Equation Modeling
Structural equation modeling was next applied to summarize the preceding findings and construct a framework for the prediction of reading comprehension in children with intellectual disabilities. All of the precursor measures shown to play a significant role in the regression analyses were included in this model. Similar to the studies by Muter et al. (Citation2004) and Oakhill and Cain (Citation2012), predictors within Waves 1 and 2 were allowed to correlate. Nonsignificant paths and correlations were then removed to produce a simplified model in which all remaining relationships were statistically significant. Based on the modification indices, a path from grammar to listening comprehension was added to improve the fit of the model. In the final model () reading comprehension (Wave 3) was predicted directly by prior reading comprehension, decoding, and listening comprehension (Wave 2), with foundational literacy skills and nonverbal reasoning (Wave 1) as additional direct predictors (R2 = .79). Reading comprehension at Wave 2 was predicted by decoding, foundational literacy skills, and grammar (R2 = .63). Decoding was predicted by only its autoregressor (R2 = .88) and listening comprehension was predicted by grammar and prior listening comprehension (R2 = .71). The proportions of explained variance in this model are consistent with the adjusted R2s found in the preceding regression analyses to predict the Wave 2 and 3 outcome measures. The fit of the final model was good, χ2(14) = 17.06, p = .253, normed fit index = 0.98, comparative fit index = .99, goodness of fit index = 0.95, and the root mean square error of approximation = .056.
Figure 1. Standardized parameter estimates for the final structural equation model predicting Wave 3 reading comprehension from Wave 2 decoding, listening comprehension, and reading comprehension and from Wave 1 linguistic and nonlinguistic precursors. Note. For correlations between variables within Wave 1 and Wave 2, see .
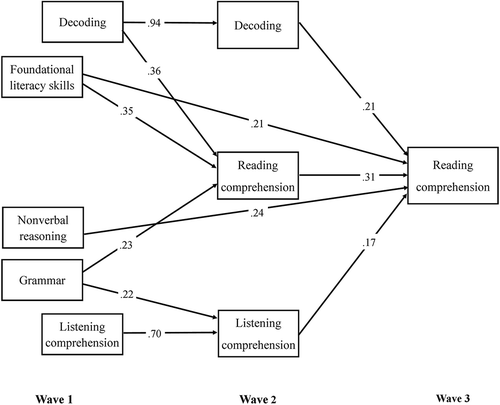
Discussion and conclusion
The present article addresses the development of reading comprehension in a group of children with mixed-aetiology intellectual disabilities. The average levels of reading comprehension, decoding, and listening comprehension that were observed in the participants at an age of 12 years were all comparable to the levels of typical children at an age of 6 years. Their scores on the precursor measures were also below those otherwise expected for children in this age range. The effects of the children’s cognitive limitations on skills related to reading comprehension are thus widespread. The low levels of overall performance that we found are in line with earlier findings (Lemons et al., Citation2013; Nash & Heath, Citation2011) and show that general cognitive limitations affect the acquisition and development of reading comprehension on many levels.
In line with the simple view of reading (Hoover & Gough, Citation1990), decoding and listening comprehension were the key precursors of reading comprehension and its development. The stronger contribution of decoding relative to listening comprehension among this group is consistent with the pattern found for early readers without disabilities (Ouellette & Beers, Citation2010; Vellutino et al., Citation2007). Classic linguistic precursors of Wave 2 decoding and Wave 2 listening comprehension were identified in the regression analyses (see also Catts et al., Citation2015; Muter et al., Citation2004), although the principal predictors were their autoregressors at Wave 1. Of interest, the prediction of Wave 2 and Wave 3 reading comprehension was not limited to decoding and listening comprehension.
For Wave 2 reading comprehension, correlations were found with all linguistic and nonlinguistic precursors, but only decoding, foundational literacy skills and grammar added significantly to the prediction of reading comprehension at Wave 2. For Wave 3 reading comprehension it was found that foundational literacy skills and nonverbal reasoning exerted an additional, direct effect on the longitudinal reading comprehension of children with intellectual disabilities, over and above decoding, listening comprehension, and prior reading comprehension. The final pattern is very similar to the long-term precursors for reading comprehension that have been found by Fuchs et al. (Citation2012) in children who were poor readers despite a normal intelligence. It seems that the pattern observed in the present analysis is not specific to children with low intelligence but can also be observed in other children who struggle to read.
A direct relationship between foundational literacy skills and reading comprehension has been observed for early readers with typical development in several studies (Engen & Høien, Citation2002; Ouellette & Beers, Citation2010), although others have found only indirect effects of these variables via decoding (Catts et al., Citation2015; Kjeldsen, Kärnä, Niemi, Olofsson, & Witting, Citation2014). Both Fuchs et al. (Citation2012) and Engen and Høien (Citation2002) emphasize that foundational literacy skills reflect—at least in part—the operation of higher level skills such as verbal working memory and metacognitive awareness. For our particular group of participants, the cognitive demands of the tasks that were used to measure phonological awareness may thus have led to an association with reading comprehension in addition to the linguistic contribution of these skills to decoding.
The direct association of nonverbal reasoning at Wave 1 with reading comprehension at Wave 3 implies that nonlinguistic cognitive skills are needed for reading comprehension in addition to word decoding and listening comprehension. One might argue that nonverbal reasoning represents higher level comprehension processes that were not included in the present study, such as inference making and comprehension monitoring. At the same time, from the perspective of the simple view of reading, the influence of higher level comprehension skills should be indirect via listening comprehension (Hoover & Gough, Citation1990; Kim, Citation2017), which is not the case here. Nonverbal reasoning explained 5% of additional variance on top of decoding, listening comprehension, and prior reading comprehension. This percentage is similar to what has been found for inference drawing in children without intellectual disabilities in longitudinal studies (Cain et al., Citation2004; Oakhill & Cain, Citation2012) and for verbal and nonverbal reasoning combined, found in early readers in cross-sectional studies (Ribeiro et al., Citation2016; Tighe & Schatschneider, Citation2014). The present finding adds to the notion that nonverbal reasoning is important for reading comprehension in its own right, most likely because it represents general intelligence.
In our previous analysis of the cross-sectional data from Wave 1 a different nonlinguistic precursor was found to predict Wave 1 reading comprehension, namely temporal processing (Van Wingerden et al., Citation2017). This shift may also be explained by the progress of the participants during the course of the longitudinal study, which can obviously alter the balance between the precursors to reading comprehension. The additional role of temporal processing in Wave 1 could have reflected a higher level of spoken language processing in addition to basic decoding skill that distinguished stronger readers from weaker readers. In a similar vein, a direct effect of nonverbal reasoning on reading comprehension was found only at Wave 3 and not at Wave 2. It may be the case that general cognitive ability is becoming a boundary condition as reading comprehension skills develop.
Study Strengths and Limitations
A comment should be made at this point with regard to the sampling used in the study that is described here. Our inclusion criteria did not specify children’s aetiology or their initial level of reading, which resulted in a heterogeneous sample of children with intellectual disabilities. The within-group diversity can be seen as a strength of the design because it gives us a representative sample of children with intellectual disabilities. However, the marked variation in their levels of literacy throughout the study may also have limited the interpretability of our findings. In addition, their reading level remained low on average. We cannot be certain that the present results are specific to children with low cognitive abilities, low reading levels, or possibly both. It is possible, for example, that the influence of foundational literacy skills would be considerably smaller in a sample with better word decoding skill.
Given the relatively small sample size, the present results must be regarded with caution. It should be noted that studies in this population are typically small and cross-sectional. We took this limitation into account in the use of observed, rather than latent variables in the longitudinal path model. In addition, the final model was based on the results of previous correlation and regression analysis, which are more resistant to small sample sizes. The consistency in our results throughout the analysis (correlations, hierarchical regressions, and the final structural model) provides confidence in the validity of our conclusions.
The explained variances by decoding and listening comprehension are lower than would be expected based on the existing literature regarding typical children. One explanation is that the cognitive factor puts constraints on the simple view of reading in this particular population and that other, cognitive factors are more decisive for the level of reading comprehension. However, several studies have pointed out that the predictive value of decoding and listening comprehension for reading comprehension can be influenced by the type of task that is used (Cutting & Scarborough, Citation2006; Lervåg, Hulme, & Melby-Lervåg, Citation2017). Further research must prove whether alternative tests for decoding, listening comprehension, and reading comprehension will yield the same pattern of results in children with intellectual disabilities.
The present analyses covered only a limited number of nonlinguistic precursors. Other indicators of general capacity such as attention, metacognitive ability, motivation, and inference skill were not included. In their cognitive view of reading comprehension, Kendeou, Van Den Broek, Helder, and Karlsson (Citation2014) identified inference making, executive functioning (working memory and inhibition), and the allocation of attention as core cognitive factors for the prediction of reading comprehension. Others have distinguished comprehension monitoring, inference making, knowledge of story structure, and use of story structure as critical factors for the prediction of reading comprehension (Cain et al., Citation2004; Oakhill & Cain, Citation2012). In light of the present results, the effects of these higher order abilities on reading comprehension certainly merit further investigation.
Conclusion
In line with the simple view of reading (Hoover & Gough, Citation1990), decoding and listening comprehension predicted reading comprehension in children with limitations in general cognition. The classic precursors for reading comprehension may not be sufficient, however, because foundational literacy skills and nonverbal reasoning significantly added to our longitudinal model to predict reading comprehension. General cognitive ability, reflected in nonverbal reasoning, may directly contribute to reading comprehension, at least in children with low cognitive abilities. General cognitive ability can thus be seen as a boundary condition for the development of reading comprehension. The longitudinal nature of this finding might indicate that the cognitive advantages and disadvantages that children experience continue to influence their reading development.
Additional information
Funding
References
- Aarnoutse, C. (1997). Begrijpend Leestest voor Groep 3 Basisonderwijs [Reading comprehension test for first grade]. Lisse, the Netherlands: Swets Test Publishers.
- Antshel, K., Hier, B., Fremont, W., Faraone, S. V., & Kates, W. (2014). Predicting reading comprehension academic achievement in late adolescents with velo-cardio-facial (22q11.2 deletion) dyndrome (VCFS): A longitudinal study. Journal of Intellectual Disability Research, 58, 926–939. doi:10.1111/jir.12134
- Asbell, S., Donders, J., Van Tubbergen, M., & Warschausky, S. (2010). Predictors of reading comprehension in children with cerebral palsy and typically developing children. Child Neuropsychology, 16, 313–325. doi:10.1080/09297041003733588
- Bonifacci, P., & Snowling, M. J. (2008). Speed of processing and reading disability: A cross-linguistic investigation of dyslexia and borderline intellectual functioning. Cognition, 107, 999–1017. doi:10.1016/j.cognition.2007.12.006
- Cain, K., Oakhill, J., & Bryant, P. (2004). Children’s reading comprehension ability: Concurrent prediction by working memory, verbal ability, and component skills. Journal of Educational Psychology, 96, 31–42. doi:10.1037/0022-0663.96.1.31
- Catts, H. W., Herrera, S., Nielsen, D. C., & Bridges, M. S. (2015). Early prediction of reading comprehension within the simple view framework. Reading and Writing, 28, 1407–1425. doi:10.1007/s11145-015-9576-x
- Choi, W., Tong, X., & Cain, K. (2016). Lexical prosody beyond first-language boundary: Chinese lexical tone sensitivity predicts English reading comprehension. Journal of Experiemental Child Psychology, 148, 70–86. doi:10.1016/j.jecp.2016.04.002
- Cotton, S. M., Kiely, P. M., Crewther, D. P., Thomson, B., Laycock, R., & Crewther, S. G. (2005). A normative and reliability study for the Raven’s coloured progressive matrices for primary school aged children from Victoria, Australia. Personality and Individual Differences, 39, 647–659. doi:10.1016/j.paid.2005.02.015
- Cutting, L. E., & Scarborough, H. S. (2006). Prediction of reading comprehension: Relative contributions of word recognition, language proficiency, and other cognitive skills can depend on how comprehension is measured. Scientific Studies of Reading, 10, 277–299. doi:10.1207/s1532799xssr1003_5
- Dessemontet, R. S., & De Chambrier, A. F. (2015). The role of phonological awareness and letter-sound knowledge in the reading development of children with intellectual disabilities. Research in Developmental Disabilities, 41, 1–12. doi:10.1016/j.ridd.2015.04.001
- Egberink, I. J. L., Vermeulen, C. S. M., & Frima, R. M. (2014). COTAN Documentatie [COTAN review of test instruments]. Amsterdam, the Netherlands: Boom Test Publishers.
- Engen, L., & Høien, T. (2002). Phonological skills and reading comprehension. Reading and Writing, 15, 613–631. doi:10.1023/A:1020958105218
- Foorman, B. R., Herrera, S., Petscher, Y., Mitchell, A., & Truckenmiller, A. (2015). The structure of oral language and reading and their relation to comprehension in Kindergarten through Grade 2. Reading and Writing, 28, 655–681. doi:10.1007/s11145-015-9544-5
- Fuchs, D., Compton, D. L., Fuchs, L. S., Bryant, V. J., Hamlett, C. L., & Lambert, W. (2012). First-grade cognitive abilities as long-term predictors of reading comprehension and disability status. Journal of Learning Disabilities, 45, 217–231. doi:10.1177/0022219412442154
- Goharpey, N., Crewther, D. P., & Crewther, S. G. (2013). Problem solving ability in children with intellectual disability as measured by the Raven’s colored progressive matrices. Research in Developmental Disabilities, 34, 4366–4374. doi:10.1016/j.ridd.2013.09.013
- Gordon, R. L., Jacobs, M. S., Schuele, C. M., & McAuley, J. D. (2015). Perspectives on the rhythm-grammar link and its implications for typical and atypical language development. Annals of the New York Academy of Sciences, 1337, 16–25. doi:10.1111/nyas.12683
- Gough, P. B., & Tunmer, W. E. (1986). Decoding, reading, and reading disability. Remedial and Special Education, 7, 6–10. doi:10.1177/074193258600700104
- Gustafson, S., Samuelsson, C., Johansson, E., & Wallmann, J. (2013). How simple is the simple view of reading? Scandinavian Journal of Educational Research, 57, 292–308. doi:10.1080/00313831.2012.656279
- Hooper, D., Coughlan, J., & Mullen, M. (2008). Structural equation modelling: Guidelines for determining model fit. Electronic Journal of Business Research Methods, 6, 53–60.
- Hoover, W., & Gough, P. (1990). The simple view of reading. Reading and Writing, 2, 127–160. doi:10.1007/bf00401799
- Huss, M., Verney, J. P., Fokser, T., Mead, N., & Goswami, U. (2011). Music, rhythm, rise time perception and developmental dyslexia: Perception of musical meter predicts reading and phonology. Cortex, 47, 647–689. doi:10.1016/j.cortex.2010.07.010
- Jongen, I., & Krom, R. (2009). Drie-minuten-toets (DMT): Handleiding [Three-Minute-Test: Instructions]. Arnhem, the Netherlands: Cito.
- Kendeou, P., Van Den Broek, P., Helder, A., & Karlsson, J. (2014). A cognitive view of reading comprehension: Implications for reading difficulties. Learning Disabilities Research & Practice, 29, 10–16. doi:10.1111/ldrp.12025
- Kim, Y. S. G. (2017). Why the simple view of reading is not simplistic: Unpacking component skills of reading using a direct and indirect effect model of reading (DIER). Scientific Studies of Reading, 21, 310–333. doi:10.1080/10888438.2017.1291643
- Kjeldsen, A. C., Kärnä, A., Niemi, P., Olofsson, Å., & Witting, K. (2014). Gains from training in phonological awareness in kindergarten predict reading comprehension in grade 9. Scientific Studies of Reading, 18, 452–467. doi:10.1080/10888438.2014.940080
- Kort, W., Compaan, E., Schittekatte, M., & Dekker, P. H. (2008). Clinical evaluation of language fundamentals–Dutch version. Amsterdam, the Netherlands: Pearson Assessment and Information.
- Kort, W., Schittekatte, M., Dekker, P. H., Verhaeghe, P., Compaan, E. L., Bosmans, M., & Vermeir, G. (2005). WISC-III-NL Wechsler intelligence scale for children. Amsterdam, the Netherlands: Harcourt Test Publishers.
- Krom, R., & Jongen, I. (2009). DMT en AVI. Arnhem, the Netherlands: Cito.
- Krom, R., Jongen, I., Verhelst, N., Kamphuis, F., & Kleintjes, F. (2010). Wetenschappelijke Verantwoording DMT en AVI [Scientific justification of DMT and AVI reading tests]. Arnhem, the Netherlands: Cito.
- Landi, N., Frost, S. J., Mencl, W. E., Sandak, R., & Pugh, K. R. (2013). Neurobiological bases of reading comprehension: Insights from neuroimaging studies of word-level and text-level processing in skilled and impaired readers. Reading & Writing Quarterly, 29, 145–167. doi:10.1080/10573569.2013.758566
- Language and Reading Research Consortium. (2015). Learning to read: Should we keep things simple? Reading Research Quarterly, 50, 151–169. doi:10.1002/rrq.99
- Lemons, C. J., Zigmond, N., Kloo, A. M., Hill, D. R., Mrachko, A. A., Paterra, M. F., … Davis, S. M. (2013). Performance of students with significant cognitive disabilities on early-grade curriculum-based measures of word and passage reading fluency. Exceptional Children, 79, 408–426. doi:10.1177/001440291307900402
- Lervåg, A., Hulme, C., & Melby-Lervåg, M. (2017). Unpicking the developmental relationship between oral language skills and reading comprehension: It’s simple, but complex. Child Development, Advance online publication. doi:10.1111/cdev.12861
- Liberman, I. Y., Shankweiler, D., & Liberman, A. M. (1989). The alphabetic principle and learning to read. In D. Shankweiler & I. Y. Liberman (Eds.), Phonology and reading disability: Solving the reading puzzle (pp. 1–33). Ann Arbor: University of Michigan Press.
- Melby-Lervåg, M., Lyster, S.-A. H., & Hulme, C. (2012). Phonological skills and their role in learning to read: A meta-analytic review. Psychological Bulletin, 138, 322–352. doi:10.1037/a0026744
- Miller, J., & Schwanenflugel, P. S. (2008). A longitudinal study of the development of reading prosody as a dimension of oral reading fluency in early elementary school children. Reading Research Quarterly, 43, 336–354. doi:10.1598/RRQ.43.4.2
- Moll, K., Ramus, F., Bartling, J., Bruder, J., Kunze, S., Neuhoff, N., … Tóth, D. (2014). Cognitive mechanisms underlying reading and spelling development in five European orthographies. Learning and Instruction, 29, 65–77. doi:10.1016/j.learninstruc.2013.09.003
- Muter, V., Hulme, C., Snowling, M. J., & Stevenson, J. (2004). Phonemes, rimes, vocabulary, and grammatical skills as foundations of early reading development: Evidence from a longitudinal study. Developmental Psychology, 40, 665–681. doi:10.1037/0012-1649.40.5.665
- Nash, H., & Heath, J. (2011). The role of vocabulary, working memory and inference making ability in reading comprehension in down syndrome. Research in Developmental Disabilities, 32, 1782–1791. doi:10.1016/j.ridd.2011.03.007
- Norton, E. S., & Wolf, M. (2012). Rapid automatized naming (RAN) and reading fluency: Implications for understanding and treatment of reading disabilities. Annual Review of Psychology, 63, 427–452. doi:10.1146/annurev-psych-120710-100431
- Oakhill, J. V., & Cain, K. (2012). The precursors of reading ability in young readers: Evidence from a four-year longitudinal study. Scientific Studies of Reading, 16, 91–121. doi:10.1080/10888438.2010.529219
- Ouellette, G., & Beers, A. (2010). A not-so-simple view of reading: How oral vocabulary and visual-word recognition complicate the story. Reading and Writing, 23, 189–208. doi:10.1007/s11145-008-9159-1
- Perez, T. M., Majerus, S., & Poncelet, M. (2012). The contribution of short-term memory for serial order to early reading acquisition: Evidence from a longitudinal study. Journal of Experimental Child Psychology, 111, 708–723. doi:10.1016/j.jecp.2011.11.007
- Perfetti, C., & Hart, L. (2002). The lexical quality hypothesis. In L. Verhoeven, C. Elbro, & P. Reitsma (Eds.), Precursors of functional literacy (pp. 189–214). Amsterdam, the Netherlands: John Benjamins.
- Raven, J. C. (1958). Guide to using the coloured progressive matrices. Oxford, England: H. K. Lewis.
- Ribeiro, I., Cadime, I., Freitas, T., & Viana, F. L. (2016). Beyond word recognition, fluency, and vocabulary: The influence of reasoning on reading comprehension. Australian Journal of Psychology, 68, 107–115. doi:10.1111/ajpy.12095
- Roch, M., Florit, E., & Levorato, C. (2011). Follow-up study on reading comprehension in down’s syndrome: The role of reading skills and listening comprehension. International Journal of Language & Communication Disorders, 46, 231–242. doi:10.3109/13682822.2010.487882
- Schlichting, L. (2005). Peabody picture vocabulary test-III-Dutch version (PPVT-III-NL). Amsterdam, the Netherlands: Hartcourt Test Publisher.
- Schuchardt, K., Gebhardt, M., & Mäehler, C. (2010). Working memory functions in children with different degrees of intellectual disability. Journal of Intellectual Disability Research, 54, 346–353. doi:10.1111/j.1365-2788.2010.01265.x
- Seigneuric, A., & Ehrlich, M. F. (2005). Contribution of working memory capacity to children’s reading comprehension: A longitudinal investigation. Reading and Writing, 18, 617–656. doi:10.1007/s11145-005-2038-0
- Soltani, A., & Roslan, S. (2013). Contributions of phonological awareness, phonological short-term memory, and rapid automated naming, toward decoding ability in students with mild intellectual disability. Research in Developmental Disabilities, 34, 1090–1099. doi:10.1016/j.ridd.2012.12.005
- Tighe, E. L., & Schatschneider, C. (2014). A dominance analysis approach to determining predictor importance in third, seventh, and tenth grade reading comprehension skills. Reading and Writing, 27, 101–127. doi:10.1007/s11145-013-9435-6
- Van Bon, W. H. J. (1986). Raven’s coloured progressive matrices: Dutch norms. Lisse, the Netherlands: Swets & Zeitlinger.
- Van Tilborg, A., Segers, E., Van Balkom, H., & Verhoeven, L. (2014). Predictors of early literacy skills in children with intellectual disabilities: A clinical perspective. Research in Developmental Disabilities, 35, 1674–1685. doi:10.1016/j.ridd.2014.03.025
- Van Uden, A. (1983). Diagnostic testing of deaf children, the syndrome of dyspraxia. Lisse, the Netherlands: Swets & Zeitlinger.
- Van Wingerden, E., Segers, E., Van Balkom, H., & Verhoeven, L. (2014). Cognitive and linguistic predictors of reading comprehension in children with intellectual disabilities. Research in Developmental Disabilities, 35, 3139–3147. doi:10.1016/j.ridd.2014.07.054
- Van Wingerden, E., Segers, E., Van Balkom, H., & Verhoeven, L. (2017). Foundations of reading comprehension in children with intellectual disabilities. Research in Developmental Disabilities, 60, 211–222. doi:10.1016/j.ridd.2016.10.015
- Vellutino, F. R., Tunmer, W. E., Jaccard, J. J., & Chen, R. S. (2007). Components of reading ability: Multivariate evidence for a convergent skills model of reading development. Scientific Studies of Reading, 11, 3–32. doi:10.1080/10888430709336632
- Verhoeven, L. (1992). LVS Lezen met begrip 1, groep 3-4: Schaal Verwijsrelaties [Reading with comprehension, first and second grade: Anaphora]. Arnhem, The Netherlands: Cito.
- Verhoeven, L. (2005). ESM-toets [Test for children with specific language impairment]. Arnhem, The Netherlands: Cito.
- Verhoeven, L., & Vermeer, A. (2001). Taaltoets Alle Kinderen [Language test for all children]. Arnhem, The Netherlands: Cito.
- Verhoeven, L., & Vermeer, A. (2006). Verantwoording Taaltoets Alle Kinderen [Justification of the language test for all children]. Arnhem, The Netherlands: Cito.
- Vloedgraven, J., Keuning, J., & Verhoeven, L. (2009). Screeningsinstrument beginnende geletterdheid [Diagnostic instrument for emergent literacy]. Arnhem, The Netherlands: Cito.
- Wise, J. C., Sevcik, R. A., Romski, M., & Morris, R. D. (2010). The relationship between phonological processing skills and word and nonword identification performance in children with mild intellectual disabilities. Research in Developmental Disabilities, 31, 1170–1175. doi:10.1016/j.ridd.2010.08.004