Abstract
We comprised metabolites of gut microbiota (GM; endogenous species) and dietary plant-derived natural flavonoids (DPDNFs; exogenous species) were known as potent effectors against non-alcoholic fatty liver disease (NAFLD) via network pharmacology (NP). The crucial targets against NAFLD were identified via GM and DPDNFs. The protein interaction (PPI), bubble chart and networks of GM or natural products- metabolites-targets-key signalling (GNMTK) pathway were described via R Package. Furthermore, the molecular docking test (MDT) to verify the affinity was performed between metabolite(s) and target(s) on a key signalling pathway. On the networks of GNMTK, Enterococcus sp. 45, Escherichia sp.12, Escherichia sp.33 and Bacterium MRG-PMF-1 as key microbiota; flavonoid-rich products as key natural resources; luteolin and myricetin as key metabolites (or dietary flavonoids); AKT Serine/Threonine Kinase 1 (AKT1), CF Transmembrane conductance Regulator (CFTR) and PhosphoInositide-3-Kinase, Regulatory subunit 1 (PIK3R1) as key targets are promising components to treat NAFLD, by suppressing cyclic Adenosine MonoPhosphate (cAMP) signalling pathway. This study shows that components (microbiota, metabolites, targets and a key signalling pathway) and DPDNFs can exert combinatorial pharmacological effects against NAFLD. Overall, the integrated pharmacological approach sheds light on the relationships between GM and DPDNFs.
Introduction
Non-alcoholic fatty liver disease (NAFLD) is an initial stage to develop malignant cascade, which might lead to hepatocellular death as irreversible condition: cirrhosis [Citation1] and hepatocellular carcinoma (HCC) [Citation2]. These diseases represent chronic inflammation, including liver damage to be aggravated by NAFLD [Citation3,Citation4]. Moreover, alterations of gut microbiota (GM) have notable characteristics such as intestinal dysbacteriosis; enhancement of intestinal penetrability and disparity of endogenous compounds: bile acids, choline and free fatty acids [Citation5].
In the context of metabolites from GM, they play critical roles to modulate inflammatory level via gut-liver crosstalk, suggesting that metabolites from GM can traverse liver through portal vein [Citation6,Citation7]. Most recently, it has been suggested that equol converted from daidzein in the gut has potent antioxidative, antiandrogenic and oestrogenic effects in host system [Citation8,Citation9]. Interestingly, individual variation to produce equol depends on the diversity of GM, which is responsible for converting from precursor [Citation10]. It implies that GM might play important roles to produce valuable flavonoids against diseases. Furthermore, dietary plant-derived natural flavonoids (DPDNFs) and GM have interconnected intricately to biological benefits for human health [Citation11]. The DPDNFs in myriad plants, fruits and vegetables own the therapeutic efficacy such as antioxidant, anti-inflammatory, anticancer and antiviral qualities [Citation12].
An animal experiment indicated that myricetin in flavonoid species has alleviative effects by modulating hepatic lipid mechanism on NAFLD in obese mice [Citation13]. The spinach, peas, turnip, carrot, cauliflower and cranberry are in plentiful supply of myricetin, its key mechanism diminished the amount of triglycerides and cholesterol [Citation14]. Additionally, another animal test suggested that luteolin reduces inflammatory factors and alleviates dysbiosis by GM, which leads to the restoration in aggravated intestinal mucosal barrier [Citation15]. The peppers, carrots, broccoli, celery, cabbages and parsley are abundant providers of luteolin, which have been taken as alternatives for alleviation in hypertension, inflammatory diseases and cancer [Citation16].
Collectively, we investigated promising components to ameliorate NAFLD by comprising GM and DPDNFs. In parallel, we explored its interactive metabolites, targets and a key signalling pathway against NAFLD, via incorporating GM and DPDNFs.
Specifically, network pharmacology (NP) is an important methodology to elucidate the integrated biological information, including complex microbiome analysis [Citation17,Citation18]. To pioneer the pharmacological pathways and relevant mechanisms is significant processing to interpret the combinatorial effects via NP concept [Citation19]. Thus, we utilized NP-based analysis to expound the relationships between GM-metabolites-targets and a key signalling pathway with DPDNFs against NAFLD. From this holistic viewpoint, we needed to make a new methodology and concept to reveal the combinatorial effects on GM and DPDNFs. At present, four crucial components (GM, targets, metabolites and a key signalling pathway) and key DPDNFs have yet to be documented.
The aim of this study is to uncover the combinatorial value in microbiome components and DPDNFs against NAFLD. Firstly, we retrieved GM targets and key DPDNFs from public database. Then, we browsed flavonoids-related and NAFLD-related targets with bioinformatics database. Also, the identified crucial targets were utilized to analyse PPI and a bubble chart. Moreover, we utilized molecular docking test (MDT) to obtain affinity between the targets and molecules on a key signalling pathway against NAFLD. More importantly, we integrated relationships with GM or DPDNFs – metabolites – targets – a key signalling pathway. In the merged networks, it has been shown that GM or DPDNFs might exert combinatorial effects. Collectively, we interpret the medicinal value of GM as endogenous species, DPDNFs as exogenous species in integrated pharmacological viewpoints. The workflow of this study is displayed in .
Materials and methods
Database platforms and data analysis associated with NP
Biologically significant databases that give a large amount of data linked to the relationship between ligands and targets, which is enables the researcher to utilize NP as a powerful tool for drug development. The utilized databases in this study were profiled in Supplementary Table S1. All these web-based databases are accessible freely to users who retrieve valuable information, moreover, which are useful resources to employ microbiome and NP concept at the same time. In this study, we incorporated the connectivity of the GM and DPDNFs, based on public databases.
The selection of metabolites from GM and its targets
The metabolites from GM were identified by gutMGene as a collective database of GM’s targets and the metabolites (http://bio-annotation.cn/gutmgene) (accessed on 3 July 2022) [Citation20]. The targets were collected by the Similarity Ensemble Approach (SEA) (https://sea.bkslab.org/) (accessed on 3 July 2022) [Citation21] and SwissTargetPrediction (STP) (http://www.swisstargetprediction.ch/) (accessed on 4 July 2022) [Citation22] as cheminformatics databases to identify potential targets. Specially, we selected only overlapping targets from the two databases to achieve the rigorousness. The Venny 2.1 tool was utilized to select the overlapping targets between SEA and STP, which was visualized by Venn diagram plotter.
The collection of DPDNFs and identification of its targets
The DPDNFs were collected by literature and the drug-likeness qualities of them were evaluated by SwissADME platform based on Lipinski’s rule of five [Citation23]. With exactness and rigour, the targets responded to DPDNFs were retrieved by SEA and STP.
The retrieval of intersecting targets and NAFLD-related targets
The targets identified by metabolites from GM and DPDNFs-related targets were analysed by Venny 2.1. The targets linked directly to NAFLD were found in both DisGENet as a platform of target-disease correlation (https://www.disgenet.org/) (accessed on 5 July 2022) and Online Mendelian Inheritance in Man (OMIM) as a database of target-etiology association (accessed on 5 July 2022) (https://omim.org/).
The identification of crucial targets and its PPI networks
The overlapping targets between the intersecting targets and NAFLD-related targets were considered as crucial targets deeply associated with NAFLD, based on the GM metabolites’ targets and DPDNFs targets. The crucial targets were input into STRING database version 11.5 to construct PPI network (accessed on 7 July 2022) [Citation24], the result of which was obtained via Excel file. The Excel file was input into R Package to describe the connectivity between each target. The PPI network depicts the networks of protein interacted by biochemical responses and/or distinctive biological events in host system [Citation25]. Noticeably, we found two significant targets on PPI network analysis.
The construction of bubble chart to identify a key signalling pathway
We obtained crucial targets with combinatorial properties on the GM metabolites’ targets and DPDNFs targets. On the bubble chart, the rich factor (the number of targets in the pathway/number of all targets in the total target set) was considered as a key selective factor [Citation26]. Then, a signalling pathway with the lowest rich factor was chosen as the uppermost mechanism, indicating that the mechanism might function as a suppressor to ameliorate NAFLD.
The preparation of metabolites and targets for MDT
The metabolites (or flavonoids) associated with the key signalling pathway were changed .sdf format from PubChem into .pdb format using Pymol, and they were changed into .pdbqt format through Autodock tool. The three targets were linked to a key signalling pathway, ie AKT Serine/Threonine Kinase 1 (AKT1) (PDB ID: 3O96), PhosphoInositide-3-Kinase, Regulatory subunit 1 (PIK3R1) (PDB ID: 4JPS) and CF Transmembrane conductance Regulator (CFTR) (PDB ID: 6O1V) were extracted by STRING on RCSB PDB (https://www.rcsb.org/), (accessed 8 July 2022). Additionally, the PDB formats of proteins were converted into .pdbqt via Autodock tool to perform the MDT.
MDT of a key signalling pathway
Based on formatting 4 energy range and 8 exhaustiveness as default to identify 10 different poses of metabolites, we performed MDT between metabolites and targets [Citation27]. The centre of each target on the key signalling pathway was AKT1 (x = 6.313, y= −7.926, z = 17.198), PIK3R1 (x= −9.551, y= −24.547, z = 31.350), CFTR (x = 152.958, y = 156.025, z = 132.814). The definition of “center” is middle point in the target frame [Citation28]. The box size of active cube was x = 40 Å, y = 40 Å, z = 40 Å, setting up as automatic in protein-ligand complexes. The complex of metabolites (or flavonoids) – targets was conducted by AutoDock 1.5.6 for 3D diagram and Ligplot + 2.2 for 2D diagram [Citation29], including hydrophobic and hydrophilic interaction.
Also, each metabolite formed the most stable conformer on the key three targets (AKT1, PIK3R1 and CFTR) was considered as a crucial effector. In other words, a molecule with the lowest binding energy on each target is defined as the most stable effector [Citation30]. After MDT, the metabolites with the lowest binding energy were chosen to describe conformers on targets in Pymol.
Toxicological verification of two key metabolites
During drug development, the level of toxicity is an important factor to be successful therapeutic agents. The toxicity was verified by the following assays: hERG blockers [Citation31], Human Hepato-Toxicity (H-HT) [Citation32], Rat Oral Acute Toxicity [Citation33], Carcinogenicity [Citation34], Eye Corrosion [Citation35], Respiratory Toxicity [Citation36]. The toxicity parameters are assessed by using ADMETlab platform [Citation37].
The networks of GM or natural resources - metabolites-targets-key signalling pathway (GNMTK)
To describe a holistic viewpoint, we constructed the network of GM or natural resources- metabolites -targets-key signalling pathway (GNMTK) via R Package. The GNMTK was depicted as nodes in the network, and their correlations were indicated as edges [Citation38]. The GNMTK network was depicted on a size plot with the number of edges connected to each node. In the network diagram, yellow circle (node) represented GM; purple circle (node) stood for metabolites; orange circle (node) indicated targets and green circle (node) described a key signalling pathway. The merged network was visualized utilizing R Package.
Results
The identification of potential targets from GM
In the gutMGene, we identified a total of 208 metabolites from GM (Supplementary Table S2) and the metabolites were searched for its targets through SEA (1256 targets) and STP (947 targets) cheminformatics platforms (Supplementary Table S2). The number of 668 overlapping targets was identified between 1256 targets in SEA and 947 targets in STP (; Supplementary Table S2). The targets were considered as respondents modulated by metabolites from GM.
Figure 2. (A) The number of 668 overlapping targets between SEA (1256 targets) and STP (947 targets). (B) The number of 112 overlapping targets related to 14 flavonoids between SEA (173 targets) and STP (200 targets). (C) The number of 112 overlapping targets between (A) and (B). (D) The number of 47 crucial targets against NAFLD.
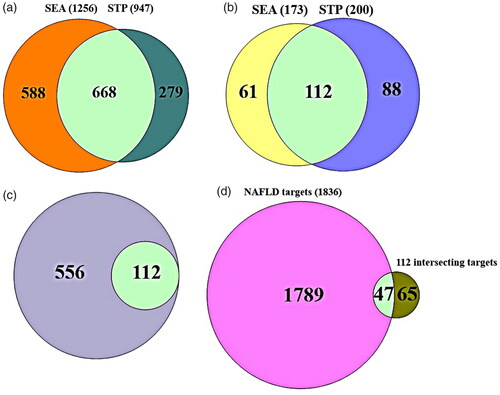
The DPDNFs’ mining and drug-likeness selection
The sixteen DPDNFs were selected through a literature study, and their chemical information was profiled in . The selected compounds’ physicochemical properties or drug-likeness qualities were assessed utilizing Lipinski’s rule of five, the parameters of which were confirmed by SwissADME web-based tool. Based on Lipinski’s rule of five, 14 out of 16 DPDNFs were accepted as drug-likeness molecules without violating of the criteria: (i) molecular weight ≤500; (ii) H-bond donors ≤5; (iii) H-bond acceptors ≤10; (iv) MLogP ≤4.15 1 (v) and Topological Polar Surface Area (TPSA) <140 (Å2) [Citation39,Citation40]. The violated compounds were Epigallocatechin-gallate and Procyanidins, which were excluded in the analysis.
Table 1. The physicochemical properties of the number of 16 dietary plant derived flavonoids.
The identification of crucial targets against NAFLD
The targets related to the fourteen DPDNFs were retrieved via SEA and STP databases, indicating 173 targets in SEA and 200 targets in STP (; Supplementary Table S3). The 112 intersecting targets were identified by the two databases (Supplementary Table S3). The 668 overlapping targets were associated with metabolites from GM, suggesting that the 112 intersecting targets were a subset of the 668 metabolites’ targets (). Coordinately, NAFLD-related targets were retrieved by DisGeNET, and OMIM online database, resulting in 1836 targets (Supplementary Table S3). Collectively, the number of 47 overlapping targets (Supplementary Table S3) was identified between NAFLD-related targets (1836) and the 112 intersecting targets (). The obtained 47 targets were considered as significant targets in determining integrated application of GM and DPDNFs against NAFLD.
PPI network construction
The STRING database was incorporated to construct PPI network on the crucial 47 targets, constructing via R Package. The 44 out of 47 targets were interacted closely with each other, however, the three targets (CA3, ST6GAL1 and ERN1) were not correlated with one another. The 44 targets were represented with 44 nodes and 241 edges (). In the PPI networks, the two targets with the highest degree value were AKT1 (31) and VEGFA (31) (). The two targets were considered as the most significant protein-coding gene in the entire PPI network.
Table 2. The degree of value in PPI network.
Bubble chart on signalling pathways against NAFLD
The results of Kyoto Encyclopaedia of Genes and Genomes (KEGG) pathway enrichment analysis indicated that the 47 targets were associated with 30 signalling pathways (False Discovery Rate < 0.05). The 30 signalling pathways were involved in occurrence and development of NAFLD, suggesting that the implicated pathways might be significant mechanisms against NAFLD. The description of the 30 signalling pathways was profiled in . Additionally, the bubble chart represented that cAMP (cyclic Adenosine MonoPhosphate) signalling pathway with the lowest rich factor might be a key mechanism against NAFLD ().
Table 3. The description of 30 signalling pathways against NAFLD.
MDT on cAMP signalling pathway
Through MDT analysis, it was uncovered that AKT1 (PDB ID: 3O96) was related to 21 metabolites: (1) Luteolin, (2) Myricetin, (3) Kaempferol, (4) Cyanidin, (5) Apigenin, (6) Diosmetin, (7) Delphinidin, (8) Pelargonidin, (9) Glycocholic acid, (10) Equol, (11) Naringenin chalcone, (12) Malvidin, (13) Quercetin, (14) Icaritin, (15) Eriodictyol, (16) Urolithin A, (17) Iuro-a, (18) Indole-3-lactic acid, (19) Lunularin, (20) 3-Indolepropionic acid and (21) Phenylalanine.
PIK3R1 (PDB ID: 4JPS) was associated with 20 metabolites: (1) Myricetin, (2) Quercetin, (3) Kaempferol, (4) Luteolin, (5) Ponciretin, (6) Apigenin, (7) Pelargonidin, (8) Acacetin, (9) 11-Methoxycurvularin, (10) Cyanidin, (11) Delphinidin, (12) Icaritin, (13) Diosmetin, (14) Malvidin, (15) Arctigenin, (16) Naringenin chalcone, (17) Secoisolariciresinol, (18) 1-Caffeoylglycerol, (19) DIF-3 and (20) 3-Hydroxyphenethyl alcohol.
CFTR (PDB ID: 6O1V) was connected with 19 metabolites: (1) Luteolin, (2) Icaritin, (3) Apigenin, (4) Kaempferol, (5) Baicalein, (6) 6,7,4′-Trihydroxyisoflavone, (7) Chrysin, (8) Genistein, (9) Diosmetin, (10) Acacetin, (11) Naringenin chalcone, (12) Indole-3-acrylic acid, (13) Indole-3-lactic acid, (14) 3-Indolepropionic acid, (15) Indoxyl sulphate, (16) Indole-3-carboxylic acid, (17) Dihydroisoferulic acid, (18) 3-Methylindole and (19) Indole-3-carboxaldehyde ().
Table 4. The binding energy and interactions of key metabolites and targets.
We observed that Luteolin could bind stably to AKT1 (−8.7 kcal/mol) (PDB ID: 3O96) and CFTR (−8.0 kcal/mol) (PDB ID: 6O1V), and Myricetin could form stable conformer on PIK3R1 (−9.3 kcal/mol) (PDB ID: 4JPS). It has been suggested that these metabolites could make strong affinity with the key targets. Its figures were displayed in .
Toxicological evaluation of two key metabolites in silico
The two metabolites (luteolin and myricetin) was assessed through ADMETlab web-based tool. The result has been shown that the two compounds had no noticeable toxic properties ().
Table 5. The verification of toxic parameters on two key metabolites.
The networks of microbiota or natural resources-metabolites-targets-key signalling pathway
The GM or natural resources-metabolites-targets-key signalling pathway (GNMTK) suggested that they have strong relationships to exert the therapeutic effects. Hence, GNMTK network represented that the orchestration of DPDNFs and favourable GM might have more beneficial effects against NAFLD. All in all, both Luteolin and Myricetin might bind stably to AKT1, PIK3R1 and CFTR to dampen cAMP signalling pathway, suggesting that the effectors are bona fide agents in DPDNFs and Enterococcus sp. 45, Escherichia sp.12, Escherichia sp.33 and Bacterium MRG-PMF-1 are critical GM in the treatment of NAFLD ().
Discussion
Our study suggested that combinatorial application with natural resources and beneficial GM might favourable pharmacological efficacy on NAFLD. Most recently, a report demonstrated that NP is an efficient methodology to elucidate active molecules in Silybum marianum against NAFLD [Citation41]. Similarly, another report has been shown that Xiaochaihu decoction plays an important role to ameliorate NAFLD via NP-based analysis [Citation42]. Certainly, we assembled the GM database on natural products database to unravel the relationships between GM and DPDNFs, in the pursuit of scaled-up approach. This methodology is needed to optimize key GM and metabolites against some diseases and would provide new insight into NAFLD therapeutics.
Accordingly, we employed the systemic analysis by integrating key components to be identified by data mining, thereby, luteolin (referred to as postbiotics or metabolites), myricetin (referred to as postbiotics or metabolites), AKT1 (target), CFTR (target), PIK3R1 (target), Enterococcus sp. 45 (probiotics), Escherichia sp.12 (probiotics), Escherichia sp.33 (probiotics) and Bacterium MRG-PMF-1 (probiotics) were associated with cAMP signalling pathway. In parallel, we surveyed luteolin-rich and myricetin-rich natural food resources. As a result, the pepper, carrot, broccoli, celery, cabbage and parsley are luteolin-rich natural resources, which are related deeply to AKT1 and CFTR. Likewise, Enterococcus sp. 45 (probiotics) can convert orientin (prebiotics) (flavone species) and cymaroside (prebiotics) (flavone species) into luteolin (postbiotics) [Citation43,Citation44].
In addition, the spinach, pea, turnip, carrot, cauliflower and cranberry are significant suppliers of myricetin, which are associated closely with PIK3R1. Similarly, Escherichia sp.12 (probiotics), Escherichia sp.33 (probiotics) and Bacterium MRG-PMF-1 (probiotics) can convert myricitrin (prebiotics) into myricetin (postbiotics) [Citation45].
An animal test demonstrated that cAMP level in NAFLD rats was elevated to as fivefold as control groups, conversely, the cAMP level had negative correlation with high density lipoprotein (HDL) cholesterol [Citation46]. Furthermore, cAMP triggers gluconeogenesis in NAFLD as well as causes insulin resistance [Citation47–49]. It has been suggested that inhibitors of cAMP signalling pathway might be important therapeutic agents in the treatment of NAFLD.
Both luteolin and myricetin are secondary metabolites in natural resources or postbiotics in specific GM. The luteolin improves sensitivity level of insulin as an antidiabetic agent, including antioxidant and anti-inflammatory properties [Citation50]. Likewise, myricetin has not only potent stimulatory effect of insulin, but accelerates lipogenesis in rat adipocytes [Citation51]. The MK-2206 as an AKT inhibitor reached at a phase II clinical trial suppressed the progression of NAFLD [Citation52]. Uncommonly, there was no relationship between CFTR and hepatic steatosis in the development of NAFLD [Citation53]. Wortmannin as PIK3R1 (PI3K) inhibitor diminished the expression level of interleukin 1β (IL-1β) and interleukin 18 (IL-18), which leads to protecting mice from NAFLD [Citation54]. The results suggested that targets (AKT1, PIK3R1) and effectors (luteolin, myricetin) might be critical elements to alleviate NAFLD on a pharmacological viewpoint. Thus, our innovative methodology gives significant clues to clarify the nuanced relationships between GM and DPDNFs.
The top 10 signalling pathways related to NAFLD were briefly discussed as follows, the selective criteria of which was based on false discovery rate (FDR< 0.05). cAMP signalling pathway: Metformin with AMPK (AMP-activated protein Kinase)-dependent mechanisms dampens mitochondrial respiratory system, activating AMPK and lowering cAMP and thereby enhancing liver enzymes and fat content in NAFLD [Citation55]. Interestingly, an animal test demonstrated that metformin as cAMP suppressor is associated with diversity of GM and reduced the production of bacterial endotoxin in NAFLD [Citation56]. It elicits that cAMP blocker might have synergistic effects via positive pharmacological mechanism and favourable GM composition on NAFLD.
Cyclic Guanosine MonoPhosphate Protein Kinase G (cGMP-PKG) signalling pathway: The Gene Expression Omnibus (GEO) database analysis shows that cyclic Guanosine MonoPhosphate Protein Kinase G (cGMP-PKG) signalling pathway is related directly to insulin resistance and gives rise to steatosis via aggravating cytotoxic cascades of NAFLD [Citation57]. JAnus Kinase-Signal Transducer and Activator of Transcription (JAK-STAT) signalling pathway: An animal experiment demonstrated that the deletion of JAK2 induces fatty liver, thereby elevating liver triglyceride as twenty times as normal group [Citation58]. Neurotrophin signalling pathway: A logistic regression study demonstrated that brain-derived neurotrophic factor (BDNF) has a negative independent factor with 0.041 (Odds ratio) in NAFLD patients, by comparison with control groups [Citation59]. T cell receptor signalling pathway: The recognition of antigen on T cell is an initial step to maintain the homeostasis of the GM, affecting liver immune system in gut-liver axis [Citation60]. NF-kappa B (NFKB) signalling pathway: O. indicum seed extract (OISE) with anti-inflammatory effects inhibits the activity of NFKB, leading to the development non-alcoholic steatohepatitis (NASH) [Citation61]. Chemokine signalling pathway: The concentrations of the C-C Motif Chemokine Ligand 2 (CCL2) and CXC Motif Ligand 9 (CXCL9) in NAFLD group were significantly greater than the control group [Citation62]. Oxytocin signalling pathway: Oxytocin is an accelerator to promote liver regeneration via autophagy, liver metabolic homeostasis [Citation63,Citation64]. Insulin signalling pathway: Insulin resistance a key risk factor to aggravate NAFLD, insulin sensitizers (thiazolidinediones) taken to ameliorate NAFLD might be critical therapeutic agents [Citation65,Citation66]. Thyroid hormone signalling pathway: The thyroid hormone can reduce the concentration of blood lipid and attenuate the progression of NAFLD [Citation67]. It implies that thyroid hormone can alleviate the hyperlipidaemia and NAFLD.
To sum up, we believe that the integrated analysis of prebiotics, probiotics, postbiotics and targets is an essential strategy to decode the complex microbiome system. In parallel, DPDNFs and GM play important role against NAFLD. Collectively, we indicate relationships of the key GM or natural resources, metabolites, targets and a key signalling pathway in the treatment of NAFLD. From a holistic perspective, we suggest the GM and DPDNFs components might exert the combinatorial effects on NAFLD. The key findings of this study were displayed in .
The pros and cons of this study
With the aid of NP, the connectivity of GM and DPDNFs is a strategy to decrypt novel uses for existing, validated, or known therapeutic ingredients that are outside extent of its real clinical features in microbiome research. This study is based on the scientific evidence that metabolites and DPDNFs from GM affect some key targets against NAFLD. At present, thanks to the development of bioinformatics, cheminformatics and microbiome databases, a great deal of biodata has been achieved. This enables to analyse the promising natural resources, GM, mechanisms, targets and metabolites against human diseases, including NAFLD. The aim of this applicability is to represent the utilization of these integrated pharmacological method to predict the relationships and to discover for new effectors in certain diseases. At this stage, the devised platform allows new data to input in the stylized format. Nevertheless, there are some limitations that our suggests are theoretical points and dependent on data-driven study. It is a challenge that we should overcome in further study.
Conclusion
Overall, this study indicated key probiotics (Enterococcus sp. 45, Escherichia sp.12, Escherichia sp.33 and Bacterium MRG-PMF-1) or natural resources, postbiotics (luteolin and myricetin) and targets (AKT1, PIK3R1 and CFTR) might block cAMP signalling pathway against NAFLD. In the scenario, we shed light on the significance of both luteolin and myricetin as metabolites from GM (Enterococcus sp. 45, Escherichia sp.12, Escherichia sp.33 and Bacterium MRG-PMF-1) and DPDNFs in the treatment of NAFLD. To conclude, we suggest that combinatorial application of favourable GM and DPDNFs might make positive efficacy in the alleviation of NAFLD.
Author contributions
Ki-Tae Suk, Dong Joon Kim and Ki-Kwang Oh had substantial contributions to the conception or design for the work. Ki-Tae Suk, Ki-Kwang Oh, Su-Been Lee, Min-Gi Cha, Goo-Hyun Kwon, Haripriya Gupta and Raja Ganesan were involved in the acquisition, or analysis for the work. Satya Priya Sharma, Sung-Min Won, Jin-Ju Jeong and Min-Kyo Jeong performed the interpretation of data for the work. Byeong-Hyun Min, Ji-Ye Hyun and Jung-A Eom performed draft of the figures. Hee-Jin Park, Sang-Jun Yoon and Mi-Ran Choi conducted the revising of the figures. All authors agree to be accountable for all aspects of the work in ensuring that questions related to the accuracy or integrity of any part of the work are appropriately investigated and resolved. Also, all authors confirmed the final approval of the version to be published.
Supplemental Material
Download MS Excel (37.6 KB)Supplemental Material
Download MS Excel (40.6 KB)Supplemental Material
Download MS Excel (11 KB)Acknowledgments
A preliminary version of this paper appeared as a Preprint [Citation68] which was reelaborated during authors’ stay at Center for Microbiome, Institute for Liver and Digestive Diseases in August 2022 and December 2022 respectively. The authors wish to thank the Hallym university for exceptional working conditions. This study has been implemented with the support of the Hallym University Research Fund, the Basic Science Research Program through the National Research Foundation of Korea, funded by the Ministry of Education, Science and Technology.
Disclosure statement
No potential conflict of interest was reported by the author(s).
Data availability statement
The authors confirm that the data supporting the findings of this study are available within the article [and/or] its supplementary materials.
Additional information
Funding
References
- Li B, Zhang C, Zhan YT. Nonalcoholic fatty liver disease cirrhosis: a review of its epidemiology, risk factors, clinical presentation, diagnosis, management, and prognosis. Can J Gastroenterol Hepatol. 2018;2018:2784537.
- Huang DQ, El-Serag HB, Loomba R. Global epidemiology of NAFLD-related HCC: trends, predictions, risk factors and prevention. Nat Rev Gastroenterol Hepatol. 2021;18(4):223–238.
- Ramakrishna G, Rastogi A, Trehanpati N, et al. From cirrhosis to hepatocellular carcinoma: new molecular insights on inflammation and cellular senescence. Liver Cancer. 2013;2(3-4):367–383.
- Bhandari P, Sapra A, Ajmeri MS, et al. Nonalcoholic fatty liver disease: could it be the next medical tsunami? Cureus. 2022;14 (4):1–10
- Giraud J, Saleh M. Host-microbiota interactions in liver inflammation and cancer. Cancers. 2021;13(17):4342.
- Zheng Z, Wang B. The gut-liver axis in health and disease: the role of gut Microbiota-Derived signals in liver injury and regeneration. Front Immunol. 2021;12:775526.
- Wang R, Tang R, Li B, et al. Gut microbiome, liver immunology, and liver diseases. Cell Mol Immunol. 2021;18(1):4–17.
- Setchell KDR, Brown NM, Lydeking-Olsen E. The clinical importance of the metabolite equol-a clue to the effectiveness of soy and its isoflavones. J Nutr. 2002;132(12):3577–3584.
- Atkinson C, Frankenfeld CL, Lampe JW. Gut bacterial metabolism of the soy isoflavone daidzein: exploring the relevance to human health. Exp Biol Med. 2005;230(3):155–170.
- Murota K, Nakamura Y, Uehara M. Flavonoid metabolism: the interaction of metabolites and gut microbiota. Biosci Biotechnol Biochem. 2018;82(4):600–610.
- Baky MH, Elshahed MS, Wessjohann LA, et al. Interactions between dietary flavonoids and the gut microbiome: a comprehensive review. Br J Nutr. 2022;128(4):577–591.
- Ullah A, Munir S, Badshah SL, et al. Important flavonoids and their role as a therapeutic agent. Molecules. 2020;25(22):5243.
- Zhu X, Bian H, Wang L, et al. Berberine attenuates nonalcoholic hepatic steatosis through the AMPK-SREBP-1c-SCD1 pathway. Free Radic Biol Med. 2019;141:192–204.
- Park KS, Chong Y, Kim MK. Myricetin: biological activity related to human health. Appl Biol Chem. 2016;59(2):259–269.
- Liu X, Sun R, Li Z, et al. Luteolin alleviates non-alcoholic fatty liver disease in rats via restoration of intestinal mucosal barrier damage and microbiota imbalance involving in gut-liver axis. Arch Biochem Biophys. 2021;711:109019.
- Lin Y, Shi R, Wang X, et al. Luteolin, a flavonoid with potential for cancer prevention and therapy. Curr Cancer Drug Targets. 2008;8(7):634–646.
- Chen X, Wang X, Ma L, et al. The network pharmacology integrated with pharmacokinetics to clarify the pharmacological mechanism of absorbed components from viticis fructus extract. J Ethnopharmacol. 2021;278:114336.
- Zhu H, Wang R, Hua H, et al. Network pharmacology exploration reveals gut microbiota modulation as a common therapeutic mechanism for anti-fatigue effect treated with maca compounds prescription. Nutrients. 2022;14(8):1533.
- Li S, Fan TP, Jia W, et al. Network pharmacology in traditional Chinese medicine. Evid Based Complement Alternat Med. 2014;2014:138460.
- Cheng L, Qi C, Yang H, et al. gutMGene: a comprehensive database for target genes of gut microbes and microbial metabolites. Nucleic Acids Res. 2022;50(D1):D795–D800.
- Keiser MJ, Roth BL, Armbruster BN, et al. Relating protein pharmacology by ligand chemistry. Nat Biotechnol. 2007;25(2):197–206.
- Daina A, Michielin O, Zoete V. SwissTargetPrediction: updated data and new features for efficient prediction of protein targets of small molecules. Nucleic Acids Res. 2019;47(W1):W357–W364.
- Chen X, Li H, Tian L, et al. Analysis of the physicochemical properties of acaricides based on Lipinski’s rule of five. J Comput Biol. 2020;27(9):1397–1406.
- Szklarczyk D, Gable AL, Nastou KC, et al. The STRING database in 2021: customizable protein-protein networks, and functional characterization of user-uploaded gene/measurement sets. Nucleic Acids Res. 2021;49(D1):D605–D612.
- Raykova D, Kermpatsou D, Malmqvist T, et al. A method for Boolean analysis of protein interactions at a molecular level. Nat Commun. 2022;13(1):4755.
- Guo L, Cui H, Zhao G, et al. Intramuscular preadipocytes impede differentiation and promote lipid deposition of muscle satellite cells in chickens. BMC Genomics. 2018;19(1):1–14.
- Khanal P, Patil BM, Chand J, et al. Anthraquinone derivatives as an immune booster and their therapeutic option against COVID-19. Nat Prod Bioprospect. 2020;10(5):325–335.
- Morris GM, Huey R, Olson AJ. Using autodock for ligand-receptor docking. Curr Protocols Bioinf. 2008;24(1):8.14.1–8.14.40.
- Laskowski RA, Swindells MB. LigPlot+: multiple ligand-protein interaction diagrams for drug discovery. J Chem Inf Model. 2011;51(10):2778–2786.
- Xu X, Yan C, Zou X. Improving binding mode and binding affinity predictions of docking by ligand-based search of protein conformations: evaluation in D3R grand challenge 2015. J Comput Aided Mol Des. 2017;31(8):689–699.
- Lamothe SM, Guo J, Li W, et al. The human ether-a-go-go-related gene (hERG) potassium channel represents an unusual target for protease-mediated damage. J Biol Chem. 2016;291(39):20387–20401.
- Mulliner D, Schmidt F, Stolte M, et al. Computational models for human and animal hepatotoxicity with a global application scope. Chem Res Toxicol. 2016;29(5):757–767.
- Karmaus AL, Mansouri K, To KT, et al. Evaluation of variability across rat acute oral systemic toxicity studies. Toxicol Sci. 2022;188(1):34–47.
- Madia F, Worth A, Whelan M, et al. Carcinogenicity assessment: addressing the challenges of cancer and chemicals in the environment. Environ Int. 2019;128:417–429.
- Wang Q, Li X, Yang H, et al. In silico prediction of serious eye irritation or corrosion potential of chemicals. RSC Adv. 2017;7(11):6697–6703.
- Da Silva E, Hickey C, Ellis G, et al. In vitro prediction of clinical signs of respiratory toxicity in rats following inhalation exposure. Curr Res Toxicol. 2021;2:204–209.
- Dong J, Wang NN, Yao ZJ, et al. Admetlab: a platform for systematic ADMET evaluation based on a comprehensively collected ADMET database. J Cheminform. 2018;10(1):11.
- Lee AY, Park W, Kang TW, et al. Network pharmacology-based prediction of active compounds and molecular targets in Yijin-Tang acting on hyperlipidaemia and atherosclerosis. J Ethnopharmacol. 2018;221:151–159.
- Oh KK, Adnan M, Cho DH. Network pharmacology-based study to identify the significant pathways of Lentinula edodes against cancer. J Food Biochem. 2022;46(9):e14258.
- Matsson PR, Kihlberg J. How big is too big for cell permeability? J Med Chem. 2017;60(5):1662–1664.
- Jiang G, Sun C, Wang X, et al. Hepatoprotective mechanism of Silybum marianum on nonalcoholic fatty liver disease based on network pharmacology and experimental verification. Bioengineered. 2022;13(3):5216–5235.
- Hu Q, Wei S, Wen J, et al. Network pharmacology reveals the multiple mechanisms of Xiaochaihu decoction in the treatment of non-alcoholic fatty liver disease. BioData Min. 2020;13:11.
- Xu J, Qian D, Jiang S, et al. Application of ultra-performance liquid chromatography coupled with quadrupole time-of-flight mass spectrometry to determine the metabolites of orientin produced by human intestinal bacteria. J Chromatogr B Analyt Technol Biomed Life Sci. 2014;944:123–127.
- Tao J, Hua Wang D, Geng Yang C, et al. Biotransformation of luteoloside by a newly isolated human intestinal bacterium using UHPLC-Q-TOF/MS. J Chromatogr B Analyt Technol Biomed Life Sci. 2015;991:1–8.
- Du LY, Zhao M, Xu J, et al. Identification of the metabolites of myricitrin produced by human intestinal bacteria in vitro using ultra-performance liquid chromatography/quadrupole time-of-flight mass spectrometry. Expert Opin Drug Metab Toxicol. 2014;10(7):921–931.
- Awaad AK, Kamel MA, Mohamed MM, et al. The role of hepatic transcription factor cAMP response element-binding protein (CREB) during the development of experimental nonalcoholic fatty liver: a biochemical and histomorphometric study. Egyptian Liver Journal. 2020;10:1–13.
- Wahlang B, McClain C, Barve S, et al. Role of cAMP and phosphodiesterase signaling in liver health and disease. Cell Signal. 2018;49:105–115.
- Lu Q, Tian X, Wu H, et al. Metabolic changes of hepatocytes in NAFLD. Front Physiol. 2021;12:710420.
- Hatting M, Tavares CDJ, Sharabi K, et al. Insulin regulation of gluconeogenesis. Ann N Y Acad Sci. 2018;1411(1):21–35.
- Sangeetha R. Luteolin in the management of type 2 diabetes mellitus. Curr Res Nutr Food Sci. 2019;7(2):393–398.
- Kandasamy N, Ashokkumar N. Myricetin modulates streptozotocin–cadmium induced oxidative stress in long term experimental diabetic nephrotoxic rats. Journal of Functional Foods. 2013;5(3):1466–1477.
- Jeong SH, Lim DS. Insulin receptor substrate 2: a bridge between hippo and AKT pathways. BMB Rep. 2018;51(5):209–210.
- Ayoub F, Trillo-Alvarez C, Morelli G, et al. Risk factors for hepatic steatosis in adults with cystic fibrosis: similarities to non-alcoholic fatty liver disease. World J Hepatol. 2018;10(1):34–40.
- Zhang W, Liu Y, Wu M, et al. PI3K inhibition protects mice from NAFLD by down-regulating CMKLR1 and NLRP3 in Kupffer cells. J Physiol Biochem. 2017;73(4):583–594.
- Kim KS, Lee BW. Beneficial effect of anti-diabetic drugs for nonalcoholic fatty liver disease. Clin Mol Hepatol. 2020;26(4):430–443.
- Brandt A, Hernández-Arriaga A, Kehm R, et al. Metformin attenuates the onset of non-alcoholic fatty liver disease and affects intestinal microbiota and barrier in small intestine. Sci Rep. 2019;9(1):1–14.
- Li L, Liu H, Hu X, et al. Identification of key genes in non-alcoholic fatty liver disease progression based on bioinformatics analysis. Mol Med Rep. 2018;17(6):7708–7720.
- Riordan JD, Nadeau JH. Modeling progressive non-alcoholic fatty liver disease in the laboratory mouse. Mamm Genome. 2014;25(9-10):473–486.
- Hashida R, Nakano D, Yamamura S, et al. Association between activity and brain-derived neurotrophic factor in patients with non-alcoholic fatty liver disease: a data-mining analysis. Life. 2021;11(8):799.
- Yang X, Lu D, Zhuo J, et al. The gut-liver axis in immune remodeling: new insight into liver diseases. Int J Biol Sci. 2020;16(13):2357–2366.
- Sun W, Liu P, Yang B, et al. A network pharmacology approach: inhibition of the NF-κB signaling pathway contributes to the NASH preventative effect of an oroxylum indicum seed extract in oleic acid-stimulated HepG2 cells and high-fat diet-fed rats. Phytomedicine. 2021;88:153498.
- Pan X, Chiwanda Kaminga A, Liu A, et al. Chemokines in non-alcoholic fatty liver disease: a systematic review and network meta-analysis. Front Immunol. 2020;11:1802.
- Luo D, Jin B, Zhai X, et al. Oxytocin promotes hepatic regeneration in elderly mice. IScience. 2021;24(2):102125.
- Yan S, Huda N, Khambu B, et al. Relevance of autophagy to fatty liver diseases and potential therapeutic applications. Amino Acids. 2017;49(12):1965–1979.
- Birkenfeld AL, Shulman GI. Non alcoholic fatty liver disease, hepatic insulin resistance and type 2 diabetes. Hepatology. 2014;59(2):713–723.
- Takahashi Y, Sugimoto K, Inui H, et al. Current pharmacological therapies for nonalcoholic fatty liver disease/nonalcoholic steatohepatitis. World J Gastroenterol. 2015;21(13):3777–3785.
- Zhang Y, Li J, Liu H. Correlation between the thyroid hormone levels and nonalcoholic fatty liver disease in type 2 diabetic patients with normal thyroid function. BMC Endocr Disord. 2022;22(1):1–11.
- Oh K-K, Gupta H, Ganesan R, et al. A network pharmacology study to determine the integrated application of dietary plant-derived natural flavonoids and gut microbiota against nonalcoholic fatty liver disease. 2022; https://doi.org/10.21203/rs.3.rs-1996432/v1