Technology for Teaching and Learning
AR: Thanks very much, Hollylynne, for taking the time to be interviewed for the Journal of Statistics and Data Science Education. Let me jump right in and ask: What are the primary questions that have driven your research in the past, and what are some questions driving your current projects?
HL: I have always been very interested in the role that technology plays in teaching and learning. That’s always driven everything that I have focused on. When I was in graduate school, an elementary school teacher asked if I would design some probability lessons for those young learners. That opportunity sparked the questions around the beginning of my career: how to engage learners in understanding how data are generated from random models. I wanted to use different dynamic representations to support this thinking, but this was not possible in the current state of software at this time.
AR: What software tools were you using at this point?
HL: I developed my own. I had developed a piece of software called Probability Explorer as part of my dissertation work, and then I tested it out with fourth-grade students. One of the next big studies that I did, as an early assistant professor, was taking that software into a middle-grades classroom and designing an entire curriculum unit around the use of that tool. I worked with James Tarr to study how students’ interactions with different dynamic representations influenced their understanding of probability. Because this was a simulation- and data-based approach, this led me to broaden my career into thinking about statistics and statistics education.
Because I am also a teacher educator, my research questions have spanned teachers’ learning as well as students’ learning in K-12 spaces. I study how I can help teachers think about doing something different with technology around probability data, and statistics. Many of my questions examine the interplay of learning tasks, software tools, student thinking, and teacher questioning. I also study how those things lead to opportunities or conundrums for students in the classroom.
AR: What grades are you focusing on?
HL: The grade band tends to be middle school to high school. I have done a couple of studies looking at introductory statistics at the college level, but I primarily focus on middle and high school. I also study those grade levels with respect to teacher preparation, as well.
In one of my current projects, I am working with Gemma Mojica and Emily Thrasher to design online spaces to help teachers learn to teach statistics and data science in asynchronous ways. We are looking at how to build an environment that allows us to pick and choose to meet teachers where they are, and to take them along their own professional journey in a kind of a design-your-own adventure approach. We’re building recommendation engines into our online learning platform to try to learn what teachers do, and also to try to suggest different learning materials that we think would be helpful for those teachers.
AR: Are you essentially building your own learning management system?
HL: Yes, we’re working with RTI International to build our own platform. It’s called http://instepwithdata.org. Our first set of about 90 teachers pilot-tested it in Fall 2022. The platform is free to use and has been open to the public since March 2023.
AR: What are some things you’ve learned in your research that are guiding your implementation of this project?
HL: Back in 2015 I had an opportunity to start doing massive open online courses for teachers, and that was a whole new world to me. My dirty little secret is that I taught online professional learning to teachers all over the world before I ever taught an online class at N.C. State.
In that work, I really learned a lot about how to connect teachers. We know a lot about general research on teachers’ learning. For example, teachers learn best whenever the learning is connected to their teaching practice as much as possible. So, we try to structure activities in an online environment that connect to their teaching, such as analyzing different statistics tasks, and then deciding and thinking about what opportunities students have to learn when you give them these tasks. Then we show videos of students working on different statistics and data ideas, and we ask teachers to reflect on those lessons.
We know that teachers learn how to teach statistics and data science through doing statistics and data science. We are always looking for ways to build those opportunities, structure them, and scaffold them in a way that motivates teachers to engage in these activities on their own. We also provide spaces to talk with their peers, in order to improve their own learning and comfort level for doing statistics. I find this aspect to be the hardest thing to achieve online.
AR: What else do you find challenging about teaching how to do statistics?
HL: We try to engage teachers in an entire data investigation. We want to put a new tool in front of them and have them think about different and larger datasets that they might analyze and also different questions that might arise. We don’t want to make it too structured, like saying “do this, then do this, then do this.” Providing growth opportunities where teachers come up with their own questions from the data is hard, but we have found evidence that if you scaffold enough and provide spaces for them to create a short video or to create screenshots of what they’ve done, and then plop those artifacts into a discussion forum, then teachers will have helpful conversations about each other’s work.
AR: How do you attract the teachers to engage in the program? Are they doing it? What are their motivations?
HL: Most of this is self-motivation. We do offer certificates of completion that they can use for continuing education units (CEUs) in their own states and local districts, so they can get a certificate that attests they’ve done a certain amount of professional development. Some of our work is developed through research grants, through which we’re able to provide stipends to try to attract teachers into working with us. We’re trying to work more toward models that allow us to partner directly with local school districts, and then the districts can provide that local structure and incentive, while using our online spaces to provide structure for what the teachers engage in.
AR: About the software tools that your teachers are learning to use—are these tools that you have developed, or commercially available ones, or what?
HL: We’ve used a variety of different tools. With the work that we did with the MOOCs from 2015 to 2020, we used tools such as CODAP, TinkerPlots, and Tuva. We made sure that teachers had access to a datafile for all of the activities, and we also provided csv files. We also had a partnership with StatCrunch and JMP where teachers could get licenses to use those.
In our current work with the InSTEP platform, we’re primarily using CODAP. We are also building broader things like in our technology modules. We’re introducing them to different applets, like the Rossman-Chance collection, and many different kinds of mini-tools that are available out there. We try to privilege open-access tools, because I think K-12 schools have become tired of buying very specific software. They’ll invest in larger software packages but not a whole lot of individual ones.
Preparing Pre-Service Teachers
AR: What would you say is the biggest challenge in preparing teachers of statistics and data science? I’m thinking now about pre-service now as opposed to in-service.
HL: Current pre-service teachers have had widely varying experiences of learning statistics in their own K-12 education. Some have had really rich opportunities, but others ended up in schools where teachers always skipped the unit on data and statistics.
It takes a lot of coordination between education departments and mathematics/statistics departments to think about and coordinate course experiences that happen on the college campus. This is hard because of the life of a university, and how different faculty rotate through teaching different courses. This makes progress hard to sustain.
I think about the Statistical Education of Teachers (SET) document that came out in 2015 (Franklin et al. Citation2015). At the time I supervised a doctoral student, Jennifer Lovett, who did a national survey on pre-service teachers’ understanding of statistics and confidence to teach statistics. Not surprisingly, the findings were pretty dismal. The pre-service students weren’t very confident, and they struggled very much with content knowledge based on the LOCUS assessments of middle-school and high-school statistics content (Lovett and Lee Citation2018). I have used the results from Jen’s dissertation to structure a lot of my research since that time, identifying the kinds of topics that we need to focus on. For example, understanding statistical inference and using simulation-based approaches were real weaknesses. Understanding association, particularly association with categorical variables, was also a major weakness in both content and preparation to teach it. Some of the materials that we’ve developed for pre-service education have focused on trying to fill those gaps.
AR: Is most of your time spent on pre-service or in-service teachers?
HL: I would say it’s probably 50–50. From 2016 until this past year, I worked with Bill Finzer through CODAP, Stephanie Casey at Eastern Michigan, Rick Hudson at the University of Southern Indiana and Gemma Mojica at NC State to develop modules to try to tackle this undergraduate teacher preparation problem (http://go.ncsu.edu/esteem). One of the other major challenges is that teacher preparation looks different across states and universities. Some universities have just one teaching methods course in which they have to cover the entire gamut of how to teach all of high school math. So, we tried to create what we call the Foundation Module, in which our approach is: “If you can only spend two weeks getting your teachers ready to tackle something related to statistics in their teaching, then use this module. If you have more time, you know, here’s an entire module on the topic of association, and here’s a complete module on statistical inference.” Those materials have been used in over seventy institutions. In Fall 2022 we started Phase Two of our efforts to extend this project, because we are trying to tackle the long overdue problem of revolutionizing undergraduate teacher preparation in statistics education.
There are several pockets of people doing good work on this issue all over the place. But we need more coordinated efforts to help programs of different sizes and structures to think about how to best coordinate across their departments in order to build courses that are effective and long-lasting. The ESTEEM project is bringing many partners together to work together toward this goal (https://fi.ncsu.edu/projects/esteem).
AR: What are some challenges, and what are some joys, of working with the two different groups: pre-service teachers and in-service teachers?
HL: One of the joys is that most pre-service and in-service teachers tend to be incredibly excited about learning, and many recognize that they need better preparation. Once they get past any of their initial fears, helping them to realize that statistics is not all about formulas (because their perceptions of what statistics is tend not to be what we want them to be learning and teaching) opens up their very joyful curiosity. Many pre-service teachers and in-service teachers will say that they’ve never looked at a dataset that had more than thirty cases or more than two variables When they start seeing that data is a way of making sense of phenomena, then they become incredibly curious about how we can use statistics and data to make sense of the world around us. They get excited about that, but then they become nervous about how to do that in their classroom, because that opens up the conversation spaces in ways that they may not feel ready to have with a group of thirty students. So, that’s a challenge of getting them into that comfort zone of “you can do this,” and it’s actually okay to make sense of these ideas along with your students.
AR: I wonder if that reluctance is mostly about having a more open environment in their classroom at all, or if the reluctance is about this particular topic (data and statistics) that they are just starting to learn themselves.
HL: I think it’s a bit of both. Stephanie and Rick led a small study of pre-service teachers after they had used some of our materials to examine how they then designed their own tasks for students. We found strengths and weaknesses in how they designed their lesson plans. Some choose datasets that had good contextual meanings, but they weren’t always great at setting up the lesson so their students would understand the context of the data in order to actually make sense of it. We saw wide variability, with some pre-service teachers creating good lesson plans that used a more open environment and asked good questions, while others were just creating worksheets that did not ask very meaningful questions.
Changes over Decades
AR: Looking back over your career, how would you say that teacher preparation, with regard to statistics and data, has changed, and how has it not changed, either for the better or for the worse?
HL: I’m going to start with how it has not changed. There are still many programs that don’t even require teachers to take a statistics class. And with programs that do require a statistics course, especially for high school teachers, it’s often a calculus-based statistics class. That’s the wrong class. That’s not true in all institutions, but it’s still pretty widespread.
We also have mathematics teacher educators who were not exposed to much statistics in their own schooling, so they feel less prepared to help prepare teachers for statistics and data. Just like K-12 teachers will often cut out the unit that they feel the least comfortable with, teacher preparation faculty also cut out the unit that they feel at least comfortable with. I think that has not changed.
I think what has changed is that there is more attention to the fact that we need data and statistics as part of mathematics teacher preparation. I think our voices are starting to be heard, whether it’s through ASA or NCTM, and especially when those organizations work together to make joint statements. As more math education faculty have become involved with statistics education, whether it’s through their research or practice, they’ve become part of that community. I think we’re seeing a bigger marriage between the two, and the marriage between the best of what people understand about teacher education in general and math teachers specifically, and what we know about good statistics education practice. So, I think that there are good things ahead. But we have to move quickly because we’ve been moving much too slowly.
AR: Do you think that more and better statistics is taught in K-12 now compared to, say, three decades ago, or is that not a true statement?
HL: I think the more part is probably true, but I’m not convinced that the better part is true. The fact that students have the opportunity to take AP Statistics absolutely has changed things. But students see data in their science classes much more than in their math classes, which provides opportunities for us to think differently about how doing things with data is not just a math curriculum thing.
AP Statistics Teachers
AR: You recently did a study of AP Statistics, teachers, and their practices and knowledge. What were some of your findings?
HL: We did a large national survey, and then we followed it up with interviews. We ended up conducting about 20 interviews. In the aggregate, AP Statistics teachers tend to be incredibly experienced. Of course, this could have been due to sampling bias, because experienced teachers were probably much more likely to fill out the survey.
The AP Statistics teachers reported on the kinds of practices that they use; lecturing, going over homework, and conducting assessments that are similar to the AP exam were pretty dominant practices. Their use of technology was primarily the graphing calculator, while some used spreadsheets and a few used applets. Usually, only the teacher has control over the spreadsheets and applets. Hardly any of them reported using more dynamic kinds of software like TinkerPlots, Fathom, or CODAP. I think that has implications for the AP exam. If they’re going to continue to only allow graphing calculators, the teachers are going to continue to privilege that tool out of necessity. The AP Statistics teachers also tended to overemphasize statistical inference.
AR: Can you elaborate on that over-emphasis?
HL: When we asked them to report what percentage of their course they spend on each of the four different areas of the AP Stats curriculum, they were spending much more time on statistical inference than what aligned with the proportion on the AP Exam; I think the mean proportion of their course was somewhere around 50%. They also under-did it with ideas around sampling and experimental design.
AR: Did you ask teachers about their perceptions of their content knowledge or about their confidence levels?
HL: We did not. We did ask them where they got some of their professional learning and the kinds of journals they read or professional conferences they went to. They go to math teacher stuff. They read things in math teacher journals. Most of them had never heard of some of the resources specific around teaching statistics. They had never heard of USCOTS and had never gone to a conference that was specific to statistics. I think this means that we still need NCTM, and we need organizations that conduct conferences and publish journals for math teachers in general to make sure that they’re getting a good heavy dose of statistics in those venues, because that’s where math teachers are going. For more, see Lee and Harrison (Citation2021).
Data Science in Schools
AR: How do you define data science? Is it different than statistics? What are the implications for trying to infuse data science into the K-12 curriculum?
HL: I first started thinking about the term “data science” when I was designing my first MOOC. We were trying to come up with the title of the MOOC, and someone suggested that I put “data science” in the title. My reaction was: There is no way I am putting that term in the title! This was 2014, and I was adamant that data science was not listed specifically in any standard, and so I knew that teachers would not attend a course called “Data Science.” So, we tried to do a play on words in order to get the idea of data science in the title, which became “Teaching Statistics Through Data Investigations.” That title led with the word that math teachers were going to see in their curriculum but also brought in the idea of teaching statistics through deep exploration of data. Andy Zieffler and I had a conversation at USCOTS many years ago about the term “data science.” Andy said: “Isn’t data science what good statistics educators already do?” and I replied that I think it is, because good statistics educators use bigger data, and they think not only about investigations where you start with a question and design your data collection, but also about questions for which data are already available from another source. I think that’s one of the key aspects of a data science approach, where the data might already exist but needs to be scraped from the web or obtained from other sources. For something to really be “data science,” I think it has to have a component of dealing with data for making sense of some phenomenon. Whether you’re doing that from asking your own question first and then gathering your data, or whether you’re doing it from getting available data and then unpacking where the data came from, what the measurements were, how someone decided what measurement to use, and what biases might have been present. If we don’t consider such issues, then we can’t actually make sense of the phenomenon. Another aspect of data science is that a lot more processing of the data must come into play, because data don’t just come to you in an easy-to-use format. Understanding how to transform data into more usable formats, and how to decide which data visualizations are going to be informative for the type of data that you have and the questions you are addressing, are the kinds of decisions that make things much more data science-y. My colleagues and I have a 2022 paper in SERJ on “Thinking like a data scientist” (Lee et al. Citation2022) where we really unpack this.
AR: Do you think the emergence of data science, both as a term and as a field, is going to be helpful for infusing more and better statistics into K-12?
HL: I think this does have promise, as long as we realize that data science in K-12 should not just be the “mini-me” of data science in industry. At the K-12 level, we should focus first and foremost on data literacy. We need to make sure students understand the very basics of how data come to be, why data is useful, how we can use data, how we can work with data, how we can be persuaded by data, and how to question whether to believe data that we see and represented in different ways in the media. Those are primary goals that we must achieve for all students in K-12.
But we also know that so many careers of the future, across a wide spectrum, rely on data. One example is data journalism. If we want citizens to be better at interpreting data, we certainly want journalists to be better at representing data in the media. And, of course, you can’t pursue any science career without dealing with data. Understanding history and social implications, and making political and socio-political decisions, whether for yourself or your community or society at large, also should rely on analyzing relevant data.
AR: What do you think of efforts from folks like Stephen Levitt and Jo Boaler to infuse data literacy/data skills in K-12?
HL: They’re certainly not the only ones in that space, but the initial podcast that they did (https://freakonomics.com/podcast/americas-math-curriculum-doesnt-add-up/) gave a wide-scale public platform that sparked a lot of questions at a national level about “what is data science, and should this be in K-12?” There were undercurrents of that already happening, but this podcast and the reaction to it raised awareness, including a workshop through the National Academies of Sciences (National Academies of Sciences, Engineering, and Medicine Citation2023).
I go back and forth in my thinking about this. I have concerns about creating pathways in data science within the mathematics curriculum at high school level, because there are so many structural issues, the whole way through college admissions that depend on what kids take in high school math. Adding new pathways can provide really rich and engaging opportunities, but I worry that they might create more inequities than they might solve. I also worry about potential unintentional consequences that might come from developing data science pathways. So we need to be thoughtful in these designs and implementation.
On the other hand, if we just take the statistics and probability standards that are already in place for middle school and high school, and concentrate on making them better, so all students enjoy a better statistics and probability and data science-y experience, that might provide an opportunity to reexamine the math curriculum and perhaps get rid of some mathematical ideas that don’t need to be there anymore, like synthetic division.
AR: How much of the challenge of making the current curriculum better is dependent on teacher preparation?
HL: It’s 100% dependent on that! If we’re going to create Introduction to Data Science courses in high school, they’ll be part of the math curriculum, so they’ll be assigned to be taught by math teachers. But we already have math teachers who say “I don’t feel very comfortable with data.” So, I feel like the train is leaving the station, and that’s why I think the teacher preparation problem has got to move quicker and get ahead of this. I don’t know if we’re ever going to get ahead of it, but we at least need to catch up.
I think the most long-standing curriculum for Introduction to Data Science at K-12 is the Mobilize project out of UCLA (https://www.mobilizingcs.org/about) (see Gould et al. Citation2016) They have used that curriculum with many teachers across several years, and they’ve learned a lot about what works. I think we still have a lot to learn as these new curricular ideas are being pushed out. But I’m not sure that we have the research capacity to rigorously study all of this and learn from it. We don’t have enough researchers who can shift their research agenda on a dime and examine these new ideas that are rolling out. We need to study these innovations thoroughly, not just at a level of how many students passed and what courses did they take afterward. Those are important questions, but we also need to study the differences in student learning that are happening in those contexts.
Beginnings, Belatedly
AR: Let me take a step back and ask you to tell us about your career path to statistics education research? What came before your graduate study in math education?
HL: I was a middle school teacher. I went to Penn State to be a high school math teacher, and my first job was teaching middle school. I got some summer experiences teaching high school, but I was primarily a very traditional algebra teacher. I started teaching when graphing calculators were first being rolled out in the early 1990s. I was handed a graphing calculator and told that I must teach with it, although I had never seen such a device.
I distinctly remember teaching out of a textbook that had boxplots. This was an algebra textbook that had a unit on statistics and a lesson about making sense of boxplots. But I had never seen a boxplot in my life up to that point, not even in my statistics class in college. I remember thinking that I had no idea about how to make sense of boxplots or why anyone would construct boxplots. All I taught my kids was how to enter the data and push the buttons to make the boxplot on the graphing calculator, and then tell what Q1, Q3, and median were. That was the extent of the wonderful statistics teacher that I was!
Then, when I first started my graduate degree, I was very interested in the role of the graphing calculator. What’s funny is that I don’t even pick up a graphing calculator anymore—seriously I have many different models and they all collect dust in a display in my office. At that time the graphing calculator was becoming a ubiquitous tool in the classroom, so I really wanted to study how that was changing things. Then I had an experience in a first-grade classroom where the teacher said: “I want to teach a probability unit. Is there any software that you could use that could do some probability stuff with kids?” My response was: “What? Probability with first graders?” As I started to think about this, I noticed that she happened to have a set of dominoes on her desk. I picked them up and started playing with them, and my immediate thought was the combinatorial difference between dominoes and rolling two dice. That led me to create a unit called “Dominoes and Dice.” We used a piece of software called Tabletop Junior, which could simulate rolling dice. We compared how likely you were, when rolling two dice, to get the outcome of a domino that was, say, three-four versus a domino that was, say, six-six. In other words, we compared outcomes of a domino that was a double versus two unique numbers. The kids immediately started saying: “Well, it depends on how I hold my domino. Is it a three-four, or is it a four-three?” I replied: “What do you think?” So, this created immediate cognitive dissonance for them, and we built the whole learning around that difference between those two situations. Through running simulations, we saw that one of those dominoes (two unique numbers) happened a lot more than the other. That experience led me to design my own software tool to do it, and then the research questions that I studied emerged organically from the teaching situations that I found myself in.
AR: What made you give up your career as a middle school teacher and go to graduate school?
HL: In my first couple of years of teaching, I had a principal who really believed in me and gave me a lot of freedom and also encouragement to attend some professional development sessions. I was teaching in the Outer Banks of North Carolina, and there were not a lot of professional development opportunities there. So, I would drive into central North Carolina to attend professional development. I started to build a network of people outside of my little school where I taught. I became really curious about how students learned, and I realized that I didn’t pay as much attention in my undergraduate studies as I wish I had, so I didn’t know enough about learning processes and the impacts of tools and teachers. I became so curious about those questions that I decided to go back and get my Master’s degree. I had to move away from the Outer Banks to do that, because there was no institution within driving distance. I moved to Virginia and enrolled at the College of William and Mary, where I was paired with a faculty member as a teaching assistant. I got to help teach his secondary methods class and supervise student teachers, and I fell in love with that. Then part of my research assistantship was working with a group in the Special Education Department to help them edit a journal, and I fell in love with reading research papers. I wanted to do what those faculty did, so I decided to go to graduate school and learn how to be a math teacher educator. I enrolled at the University of Virginia and earned a Ph.D. in mathematics education.
Pop Quiz
AR: Now let me ask my “pop quiz” questions. First, please tell us about your family.
HL: I grew up in a small town called Mount Pleasant, near Pittsburgh in Pennsylvania. My mother and father were divorced when I was young, and both of them remarried. So, I had lots of people to love me, and I got to see different examples of different careers. I didn’t really know what I necessarily wanted to do when I grew up.
I have a brother who’s a little younger than me, who eventually landed in North Carolina with his family. My husband Todd is a mathematician at Elon University. We have done some joint work in thinking about statistics education. We have two children: Delta, because she is our major change in life, and Jonah Tycho, as in Tycho Brahe, and Tyche, the goddess of Chance and Luck.
AR: What are some of your hobbies?
HL: I love to read mysteries. I grew up a Nancy Drew girl, and I have never left that genre. I read anything having to do with lawyers, FBI, police mysteries, or murder mysteries. I have a running feed on my Kindle for that genre. I especially like to follow a character through several different books.
I also love amusement parks, especially roller coasters, so I am kind of a thrill seeker in that way. I love to plan vacations. Most of my life has been spent up and down the east coast, and it’s just in the last decade that I have begun to enjoy international travel.
AR: That’s a great lead-in to my next question. Make some recommendations, for pleasure rather than work: a book to read, a piece of music to listen to, a movie or TV show to watch, and a place to visit.
HL: I am going to start with a place to visit: Prague is one of my favorite cities. I have been there twice, and I would go back in a heartbeat. The architecture is absolutely phenomenal. I happened to be there once when they were doing an art lightshow all around town. It was such an interesting mix of old architecture with these amazingly modern light shows and light art everywhere. It was an amazing experience, and the food is just fantastic. It reminds me of the food that I grew up with in Pennsylvania—very rich meat and potatoes and a lot of gravies.
I read a lot of James Patterson, in particular the Alex Cross Series. I’ve gone the whole way through, I think a few times. I have also enjoyed the Harry Bosch series by Michael Connelly.
I’m a bit eclectic in my music tastes. I love the Dave Matthews Band, and my daughter is very into K-Pop, and so we listen to a lot of K-Pop in our house. That is a lot of fun, and it keeps me young to think about the music videos that go along with K-Pop.
One of my favorite movies that just makes me smile, and I have seen so many times that it’s ridiculous, is “Say Anything” with John Cusack. It’s a rom-com and really funny; he’s one of my favorite actors. Another favorite is “A Knight’s Tale” with Heath Ledger. It’s also a rom-com, but it’s musical, and it’s a fun, feel-good movie.
AR: Here’s a fanciful question. If you could travel in time where you get to observe the world for one day, but not change anything, would you go to the past or the future, and why?
HL: I would probably go to the past. I would love to be kind of a fly on the wall on a date that was special in my family’s history. Perhaps my parents’ wedding day, or even the day I was born. What the heck was going on in the world then? Or maybe I would choose the day that my grandmother was born, just to help with putting into perspective things that ended up influencing my life in ways that I never realized. If I went to the future, I would probably pick a date that, again, is related to my family. I’m an old parent, so it’s quite possible that I might not see some very special days of my children, so I would love to see their fiftieth birthday parties.
AR: I hope you’ll be able to attend those parties in real time, without the need for a time travel device. Now suppose that you’re offered dinner for four anywhere in the world. The only catch is that the conversation has to be about statistics and data science education. Who would you invite for your three companions, and where would you go?
HL: Let’s see, I’d want to fly everybody in to somewhere around water, because I have an affinity for water. I’ll select a location on a lake, or perhaps an island, where we can just sit and enjoy the scenery. I think I would pick people that I have not had the opportunity to actually sit and converse with. There are lots of folks that I have been fortunate to sit and pick their brain, but then I’ve had very few interactions with others. I’d love to have dinner with either Chris Wild or Maxine Pfannkuch, from the other side of the world in New Zealand, because I’ve had some small interactions with them but never extended conversations. Though I’ve had some opportunities to interact with Carmen Batanero from Spain, I would love to hear her perspectives on the data science movement. She’s had such a long career, and she brings a European perspective that not many of us often think through. Finally, I have not had many personal conversations with him, but I’ve been in the room with him a lot: Ron Wasserstein from the ASA. He knows so much history and has such a unique perspective that I would love him to join the conversation.
AR: Of your many accomplishments in statistics education, can you identify one of which you’re most proud?
HL: I’m most proud of being a Fellow of the American Statistical Association, because that was not my home academic community. I grew into becoming a part of that community and am incredibly proud of that honor.
Closing Advice
AR: For JSDSE readers who teach at the post-secondary level but want to see statistics and data science education succeed at the K-12 level, what advice do you have for how they can help?
HL: Find the time to get to know the faculty at your institution who are preparing pre-service teachers and working with in-service teachers. Work with those faculty on ways to help influence curricular decisions. I think we need more collaborations at the K-12 level that bring together people who think about statistics and data science at the post-secondary level and those who focus on learning at the K-12 level. I think some K-12 educators live in too much of a bubble, and think that they can’t learn from a discipline-based perspective. I think about the AP Statistics curriculum and how so many of the folks in post-secondary have been strong influencers of what happens not just in that curriculum but also in the professional learning of AP Statistics teachers.
AR: What advice do you have for statistics and data science education researchers who are near the beginning of their career?
HL: First, I think you have to read broadly to understand the issues. For instance, if you’re coming at this from a math education perspective, you can’t just read math education journals. Similarly, if you’re coming at things from a statistics education perspective, you can’t just read statistics education journals. In some of the early days, as the field of statistics education was emerging, we had to look to psychology and other fields that we were studying how students understood different probabilistic or statistical ideas, and we learned a lot from them. When you do a broad literature search, you find aspects of what scholars have learned about teaching and learning statistics and data science in social studies journals. You’re going to find more in educational psychology journals. You’re going to find valuable insights in journals that are much more interdisciplinary, and in journals that are thinking about social justice and critical equity perspectives. We’re going to learn from computer science education journals. We need to learn broadly, because data science is a multidisciplinary field. New researchers today need to do a much broader search to acquire background knowledge, because you want to build from what’s already been learned and then ask research questions that push us into the future so that we can build stronger momentum.
My other advice is that there are a lot of unanswered questions in this field, so you don’t have to look too far to generate at least the start of some questions based on things that you notice happening around you in the practice of teaching and learning statistics and data science.
Additional information
Notes on contributors
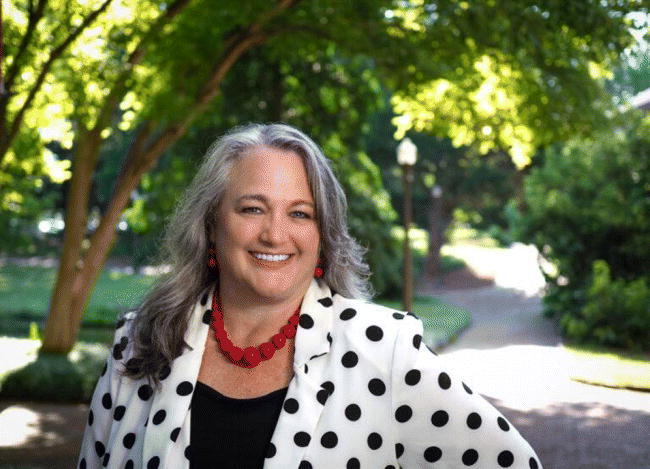
Hollylnne S. Lee
Hollylynne Stohl Lee is Distinguished University Professor of Mathematics and Statistics Education at North Carolina State University. She is also a Senior Faculty Fellow at the Friday Institute for Educational Innovation and director of the Hub for Innovation and Research in Statistics and Data Science Education. In 2020 she became a Fellow of the American Statistical Association. She received the 2022 Robert Foster Cherry Award for Great Teaching from Baylor University and the 2023 William D. Warde Statistics Education Award from the Mu Sigma Rho statistics honorary society.
References
- Franklin, C., Bargagliotti, A., Case, C., Kader, G., Scheaffer, R., and Spangler, D. (2015), SET: Statistics Education of Teachers, Alexandria, VA: American Statistical Association. Available at https://www.amstat.org/asa/files/pdfs/EDU-SET.pdf.
- Gould, R., Machado, S., Ong, C., Johnson, T., & Molyneux, J. (2016), “Teaching Data Science to Secondary Students: The Mobilize Introduction to Data Science Curriculum,” in Promoting Understanding of Statistics about Society. Proceedings of the Roundtable Conference of the International Association of Statistics Education (IASE), ed. J. Engel. Available at http://iase-web.org/documents/papers/rt2016/Gould.pdf DOI: 10.52041/SRAP.16402.
- Lee, H. S., and Harrison, T. (2021), “Trends in Teaching Advanced Placement Statistics: Results from a National Survey,” Journal of Statistics and Data Science Education, 29, 317–327. DOI: 10.1080/26939169.2021.1965509.
- Lee, H. S., Mojica, G. F., Thrasher, E. P., and Baumgartner, P. (2022), “Investigating Data like a Data Scientist: Key Practices and Processes,” Statistics Education Research Journal, 21, 3. DOI: 10.52041/serj.v21i2.4.
- Lovett, J., and Lee, H. S. (2018), “Preservice Secondary Mathematics Teachers’ Preparedness to Teach Statistics: A Call for Reform,” Journal of Statistics Education, 26, 214–222. DOI: 10.1080/10691898.2018.1496806.
- National Academies of Sciences, Engineering, and Medicine. (2023), Foundations of Data Science for Students in Grades K-12: Proceedings of a Workshop, Washington, DC: The National Academies Press. DOI: 10.17226/26852.