Figures & data
Figure 1. (lhs) Conditional Gaussian densities for
; (middle) conditional probability
as a function of
; (rhs) densities of claims Y for age X = 40 and genders D = 0, 1.
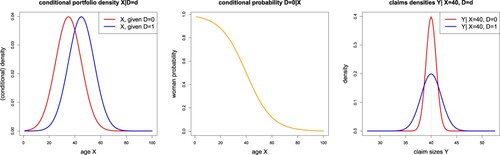
Figure 3. Average excess premium for women D = 0 compared to men D = 1, in Example 2.14, as a function of . The dashed vertical line corresponds to the baseline scenario of
,
.
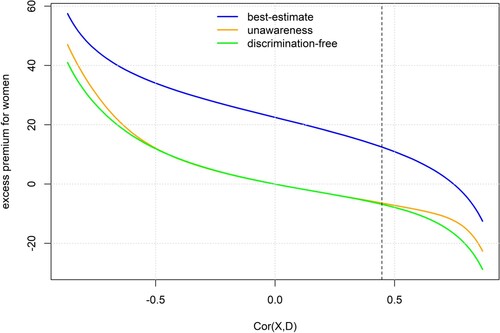
Table 1. MSEs and average prediction of the different prices in Example 2.14.
Figure 4. Example 2.14, revisited: conditional densities , for
, and two different choices for
,
; for a formal definition we refer to (Equation31
(31)
(31) )–(Equation32
(32)
(32) ).
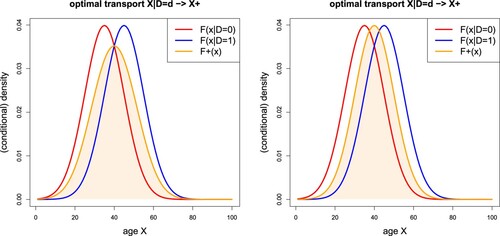
Table 2. Wasserstein distances for the two examples (Equation31
(31)
(31) )–(Equation32
(32)
(32) ) for
.
Figure 5. OT maps for examples (Equation31
(31)
(31) )–(Equation32
(32)
(32) ) of
with the original age X on the x-axis and the transformed ages
on the y-axis; the black dotted line is the 45
diagonal.
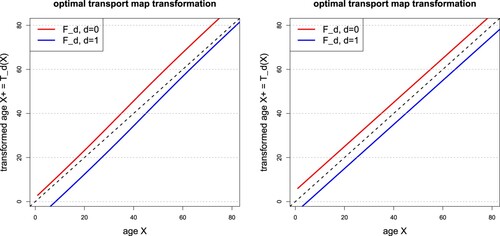
Figure 6. OT input transformed model prices for examples (Equation31
(31)
(31) )–(Equation32
(32)
(32) ) of
.
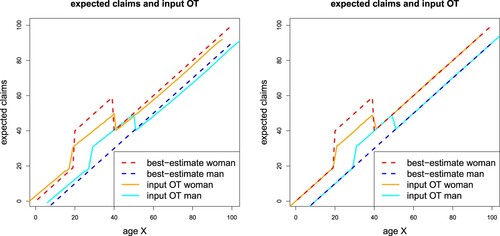
Table 3. MSEs and average prediction of the different prices in Example 2.14.
Figure 7. Changed age profiles with (women) and
(men): (lhs) conditional probability
as a function of
; (middle) best-estimate, unawareness and discrimination-free insurance prices; (rhs) OT input transformed model prices
for example (Equation32
(32)
(32) ) of
.
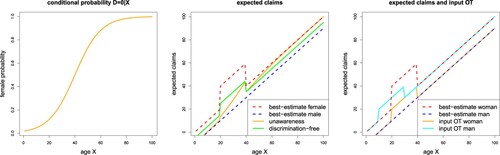
Table 4. Changed role of ages of women and men, setting and
.
Figure 9. (Top) OT output post-processed prices expressed in their original features
and separated by gender
, see (Equation38
(38)
(38) ); (bottom-lhs) OT input pre-processing taken from Figure ; (bottom-rhs) unawareness price and DFIP taken from Figure .
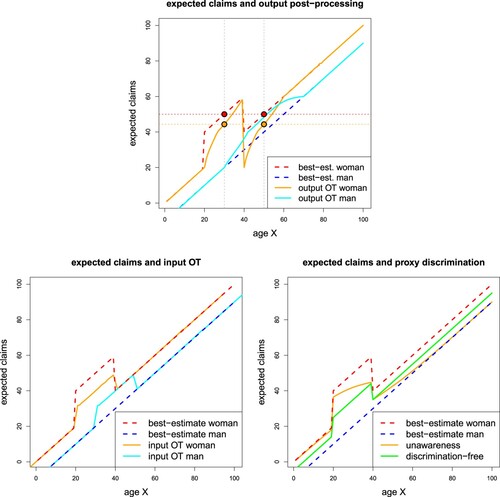
Table 5. MSEs and average prediction of the different prices in Example 2.14.
Table