Figures & data
Figure 1. Location of the burned study area in Europe (top-left), in Portugal (bottom-left). The image on the right shows the overview of the study area (Sentinel-2 composite image based on the second-lowest NIR criterion, false-color composite SWIR-NIR-RED); the red areas represent the burned surfaces.
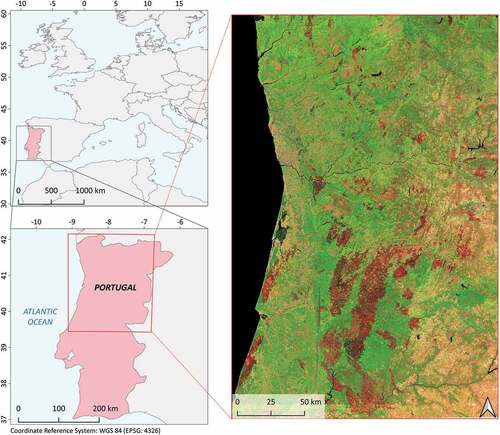
Table 1. Sentinel-2 (S2) and Sentinel-1 (S1) image mosaic dates used in this study (format: year/month/day), acquired before and during the fire season.
Figure 2. The flowchart illustrates the second-lowest NIR (secMinNIR) scheme, SAR criteria, and their placement within the workflow.
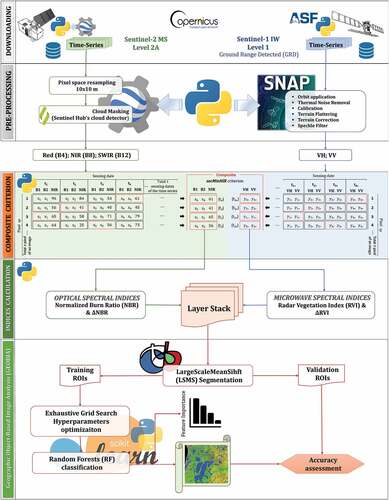
Table 2. Parameter values of the random forest (RF) classifier tested through an exhaustive grid search approach to set the optimal combination.
Figure 3. Multitemporal composite output relative to the optical part of the dataset (Sentinel-2) that resulted from processing fire season images (June-October). Five illustrative areas are shown on a more detailed scale beside the overview of the entire study area (top left). The images are shown in RGB combination (R = B12; G = B8; B = B4).
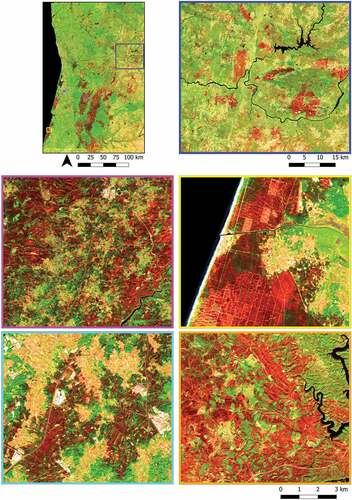
Figure 4. The Figure shows the ΔNBR index map calculated by applying the subtraction NBRpost-fire – NBRpre-fire. The darker areas correspond to lower index values, while the brighter pixel means represent higher values. The overview of the entire study area is shown on the top-left; five illustrative areas are shown on a more detailed scale.
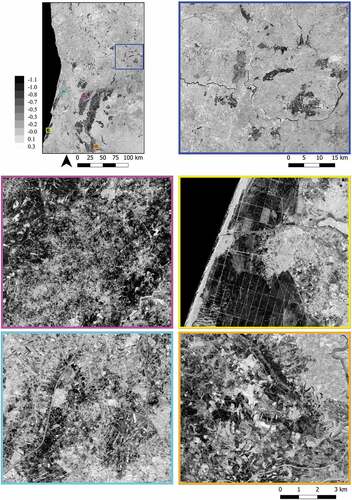
Figure 5. The ΔRVI index map, calculated by applying the subtraction RVIpost-fire – RVIpre-fire. The darker areas correspond to lower index values, while the brighter pixels represent higher values. The overview of the entire study area is shown on the top-left; five illustrative areas are shown on a more detailed scale.
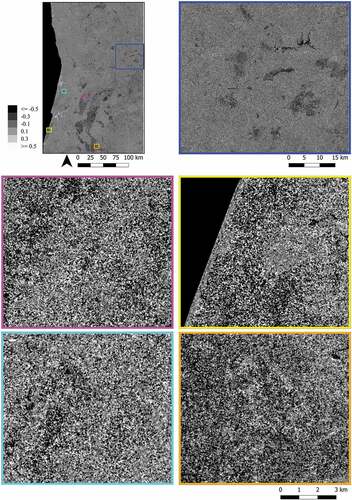
Figure 6. Segmentation output that resulted from applying the Large-Scale-Mean-Shift (LSMS) algorithm to the S1+ S2 dataset. The segments are presented in a blue border, while the base map is the S2 composite image based on the second-lowest NIR (SecMinNIR) criterion (false-color composite B12-B8-B4). The segmentation is shown for the entire study area (top left) and five detailed illustrative areas.
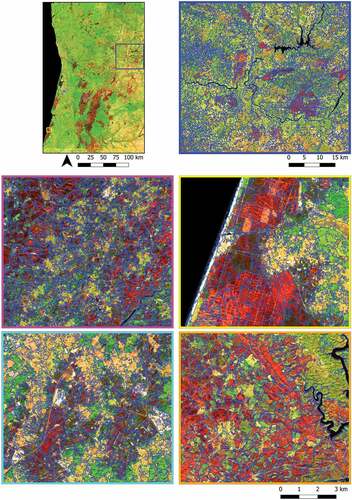
Figure 7. Segmentation output that resulted from applying the Large-Scale-Mean-Shift (LSMS) algorithm to the S2 dataset. The segments are presented in a blue border, while the base map is the S2 composite image based on the second-lowest NIR (SecMinNIR) criterion (false color composite B12-B8-B4). The segmentation is shown for the entire study area (top left) and five detailed illustrative areas.
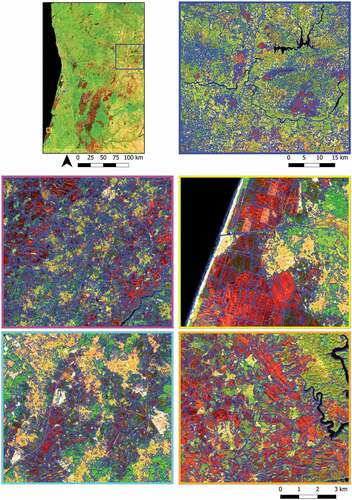
Table 3. The final combination of Random Forest (RF) parameter values that resulted from the exhaustive grid search-based test and was used in the classification approach for both datasets (S1+ S2 and S2).
Figure 8. Classification output, based on the S1+ S2 dataset. Segments showing only the burned class (blue) are overlaid on an S2 composite image based on the second-lowest NIR (SecMinNIR) criterion (false color composite B12-B8-B4). The classification is shown for the entire study area (top left) and five detailed illustrative areas.
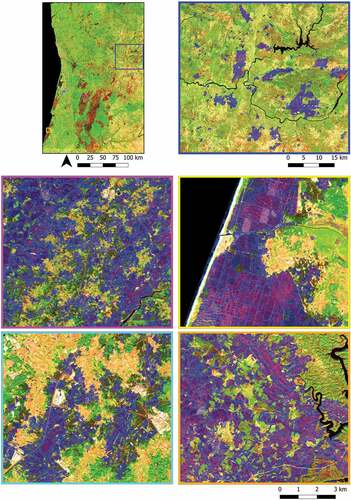
Figure 9. Classification output, based on the S2 dataset. Segments showing only the burned class (blue) are overlaid on an S2 composite image based on the second-lowest NIR (SecMinNIR) criterion (false color composite B12-B8-B4). The classification is shown for the entire study area (top left) and five detailed illustrative areas.
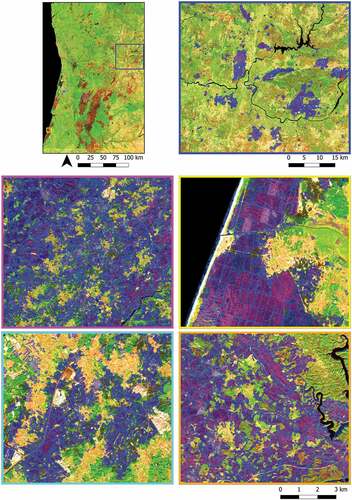
Figure 10. Feature importance for burned area mapping (Gini importance), expressed by each spectral feature (mean and variance) used in the classification process for both dataset combinations: S1+ S2 and S2.
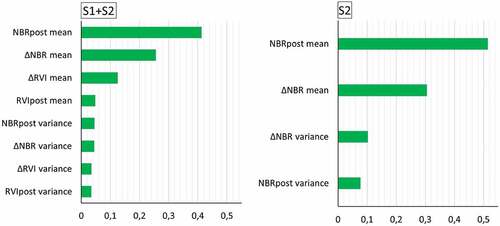
Table 4. Weighted descriptive statistics (average, µsw; standard deviation, σsw), computed using the values of the spectral features (mean, ms; variance, vs) of all the classified polygons of each S1+ S2 input layer (ΔNBR, NBR, ΔRVI, RVI), separately for burned and unburned classes.
Data availability statement
The data supporting this study’s findings are available from the corresponding author upon reasonable request. These data were derived from the following resources available in the public domain: ESA Sentinel Homepage (Citation2021).