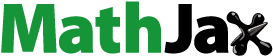
ABSTRACT
Expert opinion and judgment enter into the practice of statistical inference and decision-making in numerous ways. Indeed, there is essentially no aspect of scientific investigation in which judgment is not required. Judgment is necessarily subjective, but should be made as carefully, as objectively, and as scientifically as possible.
Elicitation of expert knowledge concerning an uncertain quantity expresses that knowledge in the form of a (subjective) probability distribution for the quantity. Such distributions play an important role in statistical inference (for example as prior distributions in a Bayesian analysis) and in evidence-based decision-making (for example as expressions of uncertainty regarding inputs to a decision model). This article sets out a number of practices through which elicitation can be made as rigorous and scientific as possible.
One such practice is to follow a recognized protocol that is designed to address and minimize the cognitive biases that experts are prone to when making probabilistic judgments. We review the leading protocols in the field, and contrast their different approaches to dealing with these biases through the medium of a detailed case study employing the SHELF protocol.
The article ends with discussion of how to elicit a joint probability distribution for multiple uncertain quantities, which is a challenge for all the leading protocols. Supplementary materials for this article are available online.
1 Introduction
This article is a result of a presentation I made to the October 2017 Symposium on Statistical Inference, organized and sponsored by the American Statistical Association, in a session devoted to the role of expert opinion and judgment in statistical inference and decision-making. From the presentations and discussions in that session, a position article by the convenor and speakers in the session presents their view that
We must accept that there is subjectivity in every stage of scientific inquiry, but objectivity is nevertheless the fundamental goal. Therefore, we should base judgments on evidence and careful reasoning, and seek wherever possible to eliminate potential sources of bias. (Brownstein et al. Citation2018)
One way in which expert opinion and judgment enters into statistical inference and decision-making is through expert knowledge elicitation. Elicitation in this context is the process of expressing expert knowledge in the form of probability distributions for uncertain quantities. It is increasingly used to quantify uncertainty about parameters in models that are employed across the whole spectrum of human endeavour. In the context of the Symposium on Statistical Inference, we can think of the elicited probability distributions as forming prior distributions for Bayesian statistical inference, or as directly informing decision-making under uncertainty.
The literature on elicitation is extensive. with important contributions made in fields as diverse as statistics, psychology, management science, economics, and environmental science. A review of this literature can be found in O’Hagan et al. (Citation2006), while Kurowicka and Cooke (Citation2006), O’Hagan (Citation2012), European Food Safety Authority (Citation2014), and Hanea et al. (Citation2018) provide entry points to some more recent developments. Even works that seek only to elicit estimates of uncertain quantities, rather than to characterize uncertainty in the form of a probability distribution, for instance Burgman (Citation2015), may offer much good advice about eliciting judgements from experts.
Eliciting expert knowledge carefully, and as scientifically as possible, is not simply a matter of sitting down with one or more experts and asking them to tell us what they think. In particular, psychologists have identified numerous ways in which naive questioning can engender cognitive biases in the experts’ judgements. To capture expert knowledge as objectively as possible in the form of a probability distribution, the elicitation needs to be structured so as to avoid, or at least to minimize, such biases. Research in the field has led to the development of carefully designed elicitation protocols, which set out procedures for the elicitation to follow.
This article begins by addressing these issues of cognitive biases and elicitation protocols. Section 2 introduces the psychological literature on heuristics and biases, highlighting those that are of particular relevance in eliciting probability distributions. In Section 3, issues around the use of multiple experts are considered, including further psychological hazards in elicitation from groups of experts. The leading protocols are introduced in Section 4. Section 5 presents a detailed case study, where judgments about the future incidence of long-term medical conditions among elderly people in the UK were elicited from a group of experts using the SHELF protocol. Each step in the protocol is discussed with particular emphasis on how it is designed to address and minimize the biases identified in Sections 2 and 3. At each step, differences between SHELF and the other leading protocols are also discussed. Section 6 considers elicitation of knowledge about two or more uncertain quantities, which is a challenge in elicitation that applies to all protocols. Finally, Section 7 reviews the principal ideas in this article.
2 Biases in Elicitation
Theories of subjective probability, in which probability is characterized as a personal degree of belief, date back at least to Ramsey (Citation1926), with important contributions by de Finetti (Citation1937), (1970) and Savage (1954). The idea of formally representing uncertainty using subjective probability judgments to inform decision-making began to be taken seriously in the 1960s and 1970s. However, at about the same time, psychologists were identifying problems in the ways that people make judgments. The ground-breaking research of Tversky and Kahneman (Citation1974) set in motion the heuristics and biases research program, the underlying principle of which is that people’s judgments are often made on the basis of heuristics, which are quick, short-cut reasoning processes. These heuristics have served us and our ancestors well in countless everyday situations where we do not have time or need for prolonged thought, but the instinctive application of heuristics can lead to systematic errors of judgment (biases) in more complex, less everyday tasks. The conclusion is that for serious decision-making we need to consciously slow down, taking time to think. Kahneman (Citation2011) expounds in detail this theory of fast and slow thinking.
Of the large number of heuristics that have been identified in the psychology literature, a few are of particular relevance to the judgment of probabilities, and hence to elicitation.
2.1 Anchoring
One of the original heuristics of Tversky and Kahneman (Citation1974) was ‘‘anchoring and adjustment’’: when asked to make a numerical judgment, people start with a readily available value and adjust from that point to assign a judgment. The initial value is called the anchor, because typically the adjustment is insufficient and so the resulting judgment is biased toward the anchor. The canonical case is when two successive judgments are made of related quantities; the first judgment acts as an anchor for the second. An example of this in the case of probability judgments is discussed in Section 2.4.
To illustrate a different form of anchoring in probability judgment, I have conducted an experiment on a sample of participants on courses I have given over the past four years. This is admittedly not a proper random sample from a well-defined population of subjects, but randomization was applied within each course.
The participants were asked to make judgments about the number of Muslims in England and Wales according to the UK census of 2011. They were given the following information:
In the 2011 United Kingdom Census, people were asked to specify their religion. Of the 52.0 million people in England and Wales who gave an answer (excluding 4 million non-respondents), 33.2 million stated that they were Christian (which includes Catholic and various Protestant denominations). Other possible answers (18.8 million people in total) were Buddhist, Hindu, Jewish, Muslim, Sikh, “Other religion”, “No religion”. In this exercise, you are asked to assess probabilities concerning the number, M, of Muslims amongst those 18.8 million.
They were asked to provide two probabilities, that M was more than 8 million and that it was more than 2 million. There were two versions of the exercise: in one version they were asked for first, and in the other version the first judgment was
. They did not see the second question until they had answered the first (and could not go back and revise their first answer). In each course, the allocation of versions to participants was randomized. I gave 10 courses over four years, to participants from widely varying backgrounds. For instance, in some cases, they were predominantly British, in two cases they were all from Nordic countries, and in two more mostly Italian. I have data from approximately 90 participants on each version of the exercise. Their average probabilities are given in .
Table 1 Average probability judgments in the Muslims exercise.
The effect of anchoring is seen clearly here. Participants who received the question first on average give higher probability judgments on both questions. Putting the number 8 million in their heads serves as an anchor, suggesting higher values for M than if the anchor is 2 million. Since the data are not from a proper random sample, any formal statistical analysis can only be indicative, but the differences are quite clear. In fact, the same feature, of higher average probabilities on both questions for participants who received the version with
first, was observed separately in every one of the 10 courses, with the sole exception of just one inversion in one course.
2.2 Availability
According to the availability heuristic, also originally identified in Tversky and Kahneman (Citation1974), an event is judged more probable if we can quickly bring to mind instances of it occurring. For instance, I will judge deaths from a particular disease to be more common if I know of people who suffer from, or have died from, this disease. Dramatic events are more memorable, and so are more easily brought to mind. Thus, aircraft and train crashes make headline news, and will influence a person’s judgment of risk with these modes of transport. Car crashes are, in contrast, far more common but are rarely newsworthy, so their probability tends to be underestimated.
More generally, when experts are asked about an uncertain quantity, their judgments will be more influenced by evidence that they can readily bring to mind. This will tend to be recent evidence and evidence that they have personally been involved in deriving. Less salient evidence is likely to be overlooked.
2.3 Range–Frequency
If we divide the possible values of an uncertain quantity into a number of categories, and ask experts to assign a probability to each category, then they will tend to spread the total probability more or less evenly between the categories. Their stated probability judgments are then a compromise between this even spread and their actual beliefs about how probable each category is. This range–frequency compromise is related to a more general heuristic of the same name (Parducci Citation1963), and leads to a bias that assigns less probability to the categories judged most likely, and more probability to the other categories. Furthermore, probability judgments are influenced by the choice of categories. In a seminal experiment, Fischhoff, Slovic, and Lichtenstein (Citation1978) asked subjects to assign probabilities to various reasons that a car might fail to start. One group were given reasons grouped into seven categories. The other group had just four categories, the first three of which were the same as for the first group, but the remaining category was” All other problems.” The second group assigned higher probabilities on average to the first three categories than the first group.
To illustrate this bias in the context of elicitation of an uncertain quantity, I carried out another experiment with participants in my most recent training course. They were asked to make judgments about the total number, C, of medals that China will win in the next summer Olympic Games. They were given a table of the number of medals won by China at all previous Summer Olympics, and they were asked to assign probabilities to C being ‘‘more than 119,’’ ‘‘between 110 and 119,’’ ‘‘between 100 and 109,’’ and so on. There were two versions of the exercises which again were randomly assigned to participants. One version had as its final category C being ‘‘less than 60.’’ while the other split this into two final categories, ‘‘between 50 and 59,’’ and ‘‘less than 50.’’ Thus, one version had eight categories, while the other had nine.
The range–frequency compromise suggests that the participants with the first version of the exercise will assign a smaller probability to the single category ‘‘less than 60’’ than the total probability given by participants with the second version to their final two categories combined. This is indeed what I found, with an average probability of 0.077 given to the single category ‘‘less than 60,’’ compared with an average total probability of 0.104 given to the split category. This was a small sample, and certainly does not prove that the range–frequency compromise is operating as expected in this example, but it is supportive of that principle and I hope to be able to establish it firmly in future courses.
2.4 Overconfidence
It is often said that experts are typically overconfident in their judgments. The evidence for this is primarily from studies in which subjects were asked to give an interval of values for an uncertain quantity with a specified probability, and where the frequency with which the true values fell within those intervals was less than the specified probability. For instance, in studies where subjects gave 95% probability intervals, fewer than 95%, and perhaps as few as 65% of those intervals were found to contain the corresponding true values. The intervals exhibited overconfidence.
A number of possible explanations can be advanced for this finding.
Although not a heuristic in its own right, overconfidence may be related to anchoring. Subjects are often asked for an interval after they have first given an estimate, which can serve as an anchor. Then, the interval being too narrow may be due to insufficient adjustment from the anchor.
For experiments, the true values of quantities must be known to the researchers but not to the subjects. Some studies have asked about ‘‘almanac’’ quantities, that is ones whose values might readily be found in a reference book or online, such as populations of cities, lengths of rivers, heights of buildings, etc. In selecting quantities, the researchers may unconsciously choose ones which are ‘‘more interesting.’’ which could equate to them having unexpected values. If so, the low coverage frequency of the subjects’ intervals may be quite reasonable, and not evidence of overconfidence.
When the subjects are presented as experts, they may feel under some pressure to demonstrate their expertise by giving narrower intervals than their knowledge would really justify. Equally, though, experts may give wider intervals when they fear there may be consequences to “getting it wrong,” thereby expressing underconfidence.
It has been suggested that expertise is associated with experts developing their own short-cut heuristics to reach quick answers to questions commonly arising in their field. An instinctive reliance on such specialist heuristics may manifest as overconfidence, because in an elicitation exercise they are likely to be asked about less routine quantities.
To assess a 95% interval, a subject must judge the probability of being outside that interval as only 5%, but it is difficult to judge small probabilities in this way.
The significance of the last point is that if an expert is asked to give a 95% interval, they simply think in terms of giving a range of values in which they judge the quantity of interest is very likely to lie. They would give the same range if asked for a 99% interval, because ‘‘very likely’’ is an imprecise term. To illustrate this, I conducted another experiment with my most recent course participants. In this exercise, they were given the following information:
You are a taxi driver and you need to drive a customer from the town of Great Missenden to Terminal 5 of London’s Heathrow Airport, to arrive no later than 17:45 on Friday evening. The journey comprises 11 miles on A roads, followed by 11 miles on motorways, including 5 miles on the M25. According to Google maps, the journey time in “usual traffic” should be 33 minutes, but you need to arrange to pick the customer up early enough so that there is very little risk of not arriving by 17:45. Let the journey time on Friday be T (minutes).
Participants were then asked to specify an upper 95% bound (a value they were 95% confident that T would be below) and an upper ‘‘plausible’’ bound (that they were ‘‘almost sure’’ T would be below). As in the other exercises, there were two versions in which either the 95% or plausible bound was requested first, and they did not see the second question until they had answered the first. shows the average values of their bounds.
Table 2 Average bounds in the taxi journey exercise.
Again, this is a single small sample, but it is striking that the participants gave an average of about 90 minutes for their first upper bound, whether they were asked first for a 95% bound or a plausible bound. If they had first given a 95% bound, to give a plausible bound they adjusted their first value upwards, whereas to go from a plausible to a 95% bound they adjusted downwards (adjustments which, because of anchoring, we may expect to have been inadequate!). I hope to be able to replicate this finding in future courses.
3 Multiple Experts
Expert knowledge elicitation is generally conducted by a facilitator, somebody who is knowledgeable about the process of elicitation, working with one or more experts. It may equally be a more informal, solo activity, in the sense that a scientist wishes to quantify his or her own knowledge about an uncertain quantity, for the purposes of some scientific endeavour. In that case, the scientist plays the role of both facilitator and expert, and although this may seem an artificial analogy it is useful; the scientist’s judgments should be made as carefully and objectively as reasonably possible, according to the principles identified in this article.
When formal expert knowledge elicitation is employed, it is usual to seek judgments from more than one expert. Nevertheless, we generally require the outcome to be a single probability distribution representing the combined knowledge of experts in the field. Resolving the experts’ judgments into a single distribution is known as the problem of aggregation. There are two principal approaches.
Mathematical aggregation. In this approach, also known as pooling, separate judgments are elicited from the experts and a probability distribution is fitted to each expert’s judgments. These are then combined into the aggregate distribution using a mathematical formula (a pooling rule).
Behavioral aggregation. In contrast, the behavioral approach asks the group of experts to discuss their knowledge and opinions, and to make group ‘‘consensus’’ judgments, to which an aggregate distribution is fitted.
Neither approach is without disadvantages. Mathematical aggregation requires a choice of pooling rule, and numerous such rules have been proposed. To limit the choice, we might ask for the rule to have desirable properties, but French (Citation1985) offers two reasonable consistency criteria and reports that no fixed pooling rule can satisfy both.
The behavioral approach has the difficulty of persuading experts with differing opinions to reach ‘‘consensus.’’ It is also open to various additional hazards that have been identified by psychologists. For instance, there are clearly problems associated with the personalities of those whose opinions may be sought as experts. More junior experts may defer to a senior person, and thereby fail to contribute their own expertise fully. Similarly, a strong personality may dominate the group discussion and consensus judgments, without necessarily meriting a dominant position by virtue of their knowledge and expertise. Even if one expert does not dominate the group, two or more like-minded experts may do so together. Conversely, the judgments of a quieter and less extrovert expert may be ignored or overlooked.
Another feature of expert groups, which might be called a group heuristic, is a tendency for discussion to be restricted to ideas that will be broadly acceptable to all the group members, a behavior known as groupthink (Janis Citation1972). In the context of behavioral aggregation in elicitation, this term describes a tendency for a consensus view to emerge that is overconfident, because of the very act of seeking consensus.
Mathematical aggregation does not have to face these additional, potentially biasing, psychological effects, a fact which is often presented as a reason for using protocols employing mathematical aggregation. The problems of personalities and groupthink are formally avoided by the experts not meeting, with all judgments being anonymized, but this is achieved at the cost of losing the opportunity for the experts to share and debate their opinions, which is the principal benefit of behavioral aggregation.
A technical question that can be directed to the aggregate distributions derived from all of these methods is, “Whose probability distribution is this?” The point of the question is that the reason for seeking an aggregate distribution is to represent uncertainty about the quantity of interest, and for this purpose it should be interpretable as an expression of a person’s subjective beliefs. It is clear that the result of mathematical aggregation is not the belief of any individual. It might be claimed that the result of a behavioral aggregation represents the beliefs of the group, but it is far from clear that the group has any such beliefs when the distribution is more the outcome of compromise than consensus.
4 Elicitation Protocols
In a substantial guidance document on eliciting expert knowledge, EFSA (the European Food Safety Authority Citation2014) recommends three protocols for elicitation with multiple experts.
The Cooke protocol (Cooke Citation1991) employs mathematical aggregation. Experts separately make judgments about the uncertain quantity or quantities of interest, but they also make judgments about a number of seed variables whose true values are known to the facilitator. The seed variables are chosen so that as far as possible they are similar to the quantities of interest (although they inevitably differ in the sense that the true values of the quantities of interest are not known). The view is taken that experts’ performance in making judgments about the seed variables will be predictive of how good their judgments will be about the quantities of interest. A special pooling rule (referred to as ‘‘the classical model’’) is used in which the experts are weighted according to their performance on the seed variables.
The Sheffield protocol in the EFSA guidance is a particular case of the SHELF protocol (Oakley and O’Hagan Citation2016; Gosling Citation2018), and employs behavioral aggregation. It is characterized by two rounds of judgments from the experts. In the first round, they make individual judgments privately. These are then revealed and discussed, with a view to sharing and understanding the reasons for differing opinions, before the second round in which the group agrees on ‘‘consensus’’ judgments. The ‘‘consensus’’ is according to the perspective of a rational impartial observer; see the discussion in Section 5.5. The SHELF protocol requires an experienced facilitator to manage the experts, and to address possible sources of bias in group interactions.
EFSA’s third protocol is the classic Delphi method (Rowe and Wright Citation1999), but adapted to elicit judgments of uncertainty rather than simply estimates. It is known as EFSA Delphi or probabilistic Delphi, and has features of both mathematical and behavioral aggregation. Experts make two or more rounds of judgments, with feedback being given between rounds summarizing the judgments of all the experts. Anonymity is maintained but there is some interaction and sharing of knowledge between experts, although the interaction is strictly limited. At the end, it is necessary to apply a pooling rule to aggregate across the experts’ final distributions. The IDEA protocol (Hemming et al. Hemming Citation2018; Hanea et al. Citation2018) is a version of Delphi with just two rounds, but with more emphasis on a facilitated discussion between experts after the first round. Published examples do not ask for formal probabilistic judgments, but the protocol is flexible enough to accommodate different forms of judgments, including probabilistic judgments as in the above three protocols, and can involve seed variables and weighted pooling.
It is not possible to say which of these leading protocols is best, in the sense of most accurately quantifying the experts’ knowledge and beliefs in the form of a probability distribution. The principal reason is what has been seen as the impracticality of conducting experiments in which genuine experts make judgments about uncertain quantities that are within their area of expertise. Even if the true values of these quantities were to become known subsequently, which is rarely the case, an experiment would need to draw on a large number of experts, allocate them randomly to different protocols, and do this on enough different quantities and occasions to constitute adequate replication. Nevertheless, this is a neglected area of research that would benefit from some innovative thinking.
Such experimental evidence as exists is much more limited and can at best be only indicative of good practice. For instance, much of the experimental evidence in psychology addresses a small part of an elicitation (such as the elicitation of single probability) and is based on nonexperts (often psychology students). Cooke and Goossens (Citation2008) and Cooke et al. (Citation2014) analyze applications of the Cooke method and show that the “classical model” weighted pooling performs better than equal-weighted pooling. These analyses perhaps come closest to the goal of studying entire elicitation protocols, but they rely on comparing predictions of some of the seed variables (with the weighting based on judgments of the other seed variables), not on predictions of the quantities of interest.
Protocols represent the judgments of their developers, based on the evidence and their own experience, as to the best way to ensure that the elicited distribution provides a careful, scientific synthesis of the experts’ knowledge. And the choice of a protocol for any given elicitation task is another judgment, in this case the judgment of the facilitator in collaboration with the client.
The above brief outlines of the leading protocols will be amplified through the case study in Section 5, where the differences between them are identified for each stage of the elicitation process.
5 Case Study
In July 2014, I facilitated an elicitation workshop as part of a large project managed by the Centre for Workforce Intelligence on behalf of and by commission from the UK Department of Health called “Horizon 2035” (H2035), the purpose of which was to advise government on the likely demand for health services in the year 2035 and improve system-wide workforce planning (CFWI Citation2015). The elicitation concerned the incidence of Long-Term Conditions (LTCs) in 2035. LTCs cover a variety of health conditions that require long-term care, such as diabetes, heart disease, and respiratory disorders. The term also includes mental disorders, but the elicitation concerned only physical LTCs. This case study focuses on the elicitation of the number of LTCs in 2035 among people aged 85 and above, which is the group with the highest rate of LTCs.
The elicitation was conducted according to the SHELF protocol, and involved a one day face-to-face meeting. In this section, I will describe all the steps of that elicitation, highlighting how the protocol is designed to aid with careful, scientific judgments, including the ways in which SHELF avoids or minimizes the various cognitive biases and challenges identified in Sections 2 and 3. Comparisons will be made throughout with the other leading protocols.
5.1 Preliminaries
Whatever protocol one is using, good elicitation requires extensive preparation. An important early step is the selection and recruitment of the experts. There is much useful guidance on this in European Food Safety Authority (Citation2014). For the SHELF protocol, some considerations are:
Aim for around four to eight experts. This is a large enough number generally to cover the range of opinion within the relevant community; more will usually extend the discussion unnecessarily without usefully adding further knowledge and opinion. For Cooke and Delphi protocols, four to eight also works well, although it is possible to accommodate larger numbers.
Choose experts who will listen to and take account of the opinions of the other experts. People whose opinions are too firmly held will make it difficult to achieve the kind of consensus required. Even if such a person is a very high-ranking expert, it may be better to invite them to submit their judgments in writing, for consideration by the other experts in the elicitation workshop, than to invite them to take part in the workshop. This advice is also relevant for the Delphi protocol, although it is not a concern for Cooke.
Don’t invite an expert who will naturally defer to another invited expert. Pushing that person to express their own opinions is unlikely to be profitable and may cause them discomfort. In the Cooke and Delphi protocols, where experts’ judgments are made anonymously or no interaction between experts is allowed, this advice does not apply.
For the H2035 case study, five experts were present—a statistician working in chronic disease modeling, a Director of Public Health, an Allied Health Professions Officer at the National Health Service, a health policy analyst and an epidemiologist.
Another important step before the elicitation workshop is the preparation of an evidence dossier. This assembles all the most relevant evidence into a single document in a format that is readily accessible during the workshop. It is reviewed at the start of the SHELF workshop, and during the discussion stage the experts are encouraged to explain their first round judgments by reference to specific evidence in the dossier. The evidence dossier is a key tool in combating the availability heuristic, by ensuring that all the evidence is fresh in the experts’ minds at all times. Once experts have been recruited for the elicitation, they are asked to read a first draft of the dossier (which is based on initial research by the client) and to submit any additional evidence that they may have or be aware of, in order for this to be included in the final draft for the benefit of all the experts. The SHELF package of documents (Oakley and O’Hagan Citation2016) includes advice on preparing the evidence dossier, together with an example dossier.
Reviewing the evidence is obviously also required in other protocols, although there is generally less emphasis on the formal preparation of a dossier.
Finally, experts need to be trained to make the necessary probabilistic judgments. In the H2035 case study, the experts were trained at the start of the workshop, and completed a practice elicitation to familiarize them with the process and the judgments required, and to identify and resolve any initial misunderstandings. Rather than take time during the workshop itself for training, there is now an online e-learning course available at http://www.tonyohagan.co.uk/shelf/ecourse.html, sponsored by the U.S. Office of Naval Research. This will familiarize the experts with making the necessary probabilistic judgments in advance, although it will still be necessary to run through a training exercise so that they understand the process of group discussion and judgments.
Training is important for other protocols, too. Indeed, the original impetus for the e-learning course was the failure of the first attempt by EFSA to use the probabilistic Delphi protocol. Experts generally do not meet in Delphi elicitation, and so face-to-face training is not feasible. In that EFSA elicitation, experts were sent detailed written instructions by email, but it was clear from their subsequent judgments that they had either not read or not fully understood the instructions. It is hoped that the e-learning course will lead to experts being better able to make judgments in a Delphi exercise. The specific judgments covered in the course are those required in the SHELF protocol, but could also be used in probabilistic Delphi, and they are readily adapted to judgments required in the Cooke protocol.
5.2 SHELF Templates
An elicitation according to the SHELF protocol follows a number of templates that organize the process of the elicitation though a predefined series of steps. Each template is a document, in which the conduct and outcome of each step are to be recorded. The completed templates form a record of the elicitation. A SHELF workshop begins with the completion of a SHELF1 template, which records housekeeping details such as the time and place of the elicitation, the names and areas of expertise of the participants (experts, facilitator, and any other persons present), any conflicts of interest, and the training given. Then, for each uncertain quantity of interest, a SHELF 2 template is completed, which records the elicitation of a probability distribution for that quantity. It is through the prescribed sequence of steps in each template that the SHELF protocol ensures careful and thoughtful consideration of all the required judgments, and avoids or minimizes sources of bias.
shows the first part of the SHELF2 record for the H2035 case study. The first three fields repeat information from the SHELF1 record, while the ‘‘Quantity’’ field states the uncertain quantity of interest to be elicited with this template. Note that in the ‘‘Definition’’ step, the quantity (now named as X) is defined more precisely. It is essential that the definition is unambiguous, since otherwise the experts may interpret it differently from each other, and differently from how it was intended. All experts are required to agree on the definition.
Table 3 H2035 SHELF2 record, preliminaries.
The ‘‘Anonymity’’ field is important. Although the participating experts are named in the SHELF1 record, details of discussions and judgments recorded in the SHELF2 record are anonymized, so that a reader will know what was said but not who said it.
Note that the ‘‘Start time’’ was 14:15 because the morning was taken up with preparation, including training, a practice elicitation, and reviewing the evidence dossier. (The dossier is reviewed again for each quantity of interest, identifying data particularly relevant to that quantity.)
The SHELF templates serve the dual role of prescribing a protocol to minimize cognitive biases and documenting the elicitation exercise. The completed SHELF1 and SHELF2 records provide a traceable account of how the final probability distributions were reached. Documentation like this represents good scientific practice, and allows any recipients of the elicited distributions to evaluate the extent to which they should adopt them for purposes of inference or decision-making.
Further excerpts from the H2035 SHELF2 record will be shown in subsequent sections of this case study. The full record is available in supplementary material. The SHELF package can be downloaded from http://tonyohagan.co.uk/shelf, and contains all the templates together with many documents, PowerPoint presentations and software to guide and assist the facilitator in organizing and conducting an elicitation according to the SHELF protocol.
5.3 Individual Judgments
The next steps comprise the SHELF protocol’s individual judgments round. Experts are first asked to write down privately their own plausible range for X, in the form of a lower bound L and an upper bound U, such that they would find it very surprising if the true value were found to lie outside the interval . It is not important whether the expert interprets this interval as being a 95% interval, a 99% interval or an ‘‘almost sure’’ interval. Its function is to establish that some values of X are judged by the expert to be simply not plausible. By beginning with this judgment, the expert is encouraged to think at the outset about the full range of possibility for X, which helps to counter overconfidence.
The facilitator encourages them to challenge their bounds by imagining that somebody reports to them that the true value of X has been determined and it falls just outside . Their reaction to such a claim should be that it was most likely wrong—either the method of determining the value was flawed or it has been reported incorrectly. If not, if a value outside their range might indeed be plausible, then they should either decrease L or increase U. The facilitator then seeks to determine the lowest value of L given by any of the experts and the highest value of U, pointing out that all judgments can now be confined to
. Experts are asked again to consider whether their own plausible range is wide enough, and to revise if necessary.
Following the judgments of plausible range, the individual judgments round is completed according to one of three SHELF methods. shows the relevant field of the H2035 record, where the quartile method was chosen. The following points are illustrated by .
Table 4 H2035 SHELF2 record, individual elicitation.
Median. The expert is asked to specify their median value M, such that in their judgment X is equally likely to lie above or below M. Notice that the only numbers that have been suggested have come from the expert, rather than being given to the expert in a way that might create anchors. In judging M, the expert has anchors at L and U, and their anchoring effects should tend to cancel out so as to have minimal impact on the expert’s judgment of M.
Quartiles. The expert then specifies their quartiles Q1 and Q3, such that the expert judges it to be equally likely for X to be below Q1, or between Q1 and M, and equally likely to be between M and Q3, or above Q3. Again, any anchoring effect on these judgments of the previously specified values L,
and M should be minimal.
Challenging the judgments. records some of the ways that experts are challenged to think about their judgments, and to revise them if necessary. The challenges are an important part of helping the experts to make their judgments carefully and thoughtfully.
Training. The judgments of median and quartiles are not explicitly judgments of probability but simply ask the expert to identify equally likely ranges of possible values of X. Nevertheless, they are not easy for experts who have not encountered these ideas before. Training and careful explanation are essential. For every judgment that experts are asked to make within the SHELF protocol, the SHELF package includes a PowerPoint slide set to guide the expert in making, challenging, and refining their judgments.
The SHELF tertile method simply replaces the judgment of quartiles with tertiles T1 and T2. They divide the range of possible values into three equally likely parts—below T1, between T1 and T2, and above T2. This method addresses overconfidence and anchoring in the same way as the quartile method.
The third SHELF method is known as the roulette method. After the first step of specifying the plausible range, the expert is then presented with a tableau comprising a series of N ‘‘bins,’’ where N is typically about 10, each representing a range of possible values of X so that the bins partition the plausible range into N parts. The expert places counters in the bins to show the relative probability that X will fall in each bin. For instance, if the expert places one counter in the first bin and three counters in the next, he or she is making the judgment that X is three times as likely to lie in the second bin compared to the first. This method has the advantage that the experts find it simple to use, particularly if they are familiar with the concept of a probability density function. However, some bias due to the range–frequency compromise may be expected, as shown for instance in the example in Section 2.3. Moreover, there is a temptation for experts to place their counters to achieve a nice shape, rather than encouraging them to think carefully about probabilities. For these reasons, although some practitioners of the SHELF protocol use the roulette method, the guidance in the SHELF package recommends the tertile or quartile methods.
The other leading protocols ask the experts to make similar judgements. In the Cooke protocol, they are usually asked for their median and 95% range. The use of a 95% range to characterize the expert’s uncertainty seems unwise in the light of evidence that experts do not judge such intervals well, and do not distinguish well between 95%, 99%, or ‘‘almost certain’’; see Section 2.4. SHELF asks for a plausible range, but for the purpose of countering over-confidence and anchoring, using instead the quartiles or tertiles to characterize uncertainty. The EFSA guidance specifies using the SHELF judgments of plausible range, median, and quartiles for the probabilistic Delphi protocol, although others have been used.
5.4 Facilitated Group Discussion
When judgments are elicited only from a single expert, or when an individual is simply using the framework of formal elicitation to express their own knowledge, then there is no need for aggregation; all protocols will use that expert’s judgments as the basis for a final fitted probability distribution. In the case of more than one expert, however, once the experts are happy with their individual judgments, which hitherto have been written down privately, then in the SHELF protocol all are revealed and the group discussion phase begins. Other protocols do not use behavioral aggregation. The probabilistic Delphi protocol allows for some limited exchange of information between experts, but then they are aggregated by a pooling rule such as an equally weighted average. The Cooke protocol does not incorporate any interaction between experts, and their individual judgments are aggregated by a weighted pool, the weights being determined from the experts’ judgments on the seed variables. For the SHELF protocol, however, the group discussion is an essential part. Ideally, the experts will all be present for face-to-face discussion in what is called a SHELF workshop. If that is not feasible, video-conferencing provides a reasonable alternative, although it becomes harder to manage the discussion so that every expert is able to participate fully.
The judgments of the five experts in the H2035 elicitation workshop are shown in , while plots probability distributions fitted to those judgments using the SHELF software, which was set to select for each expert the best-fitting distribution from a variety of standard families. Note that in fitting the distributions the experts’ plausible bounds are not used, except that and/or
may be used if bounded distributions are to be fitted (and this range is also used to display the fitted densities).
Table 5 Individual judgments in the H2035 elicitation.
In the legend for , Expert 1 is A in , Expert 2 is B, and so on. It is clear that the experts have a wide range of initial opinions, forming a natural basis to start the group discussion stage.
SHELF is based on behavioral aggregation because its developers believe it makes better use of the combined knowledge and expertise of the experts, but Section 3 has identified a number of additional challenges associated with the behavioral approach. For this reason, the SHELF workshop must be led by a facilitator who has expertise in the process of eliciting expert knowledge, and in particular is familiar with SHELF. The expertise of the facilitator in managing the SHELF protocol is as important as the experts’ expertise regarding the quantities of interest. The facilitator works with the experts to obtain accurate judgments of their knowledge and manages the group discussion.
The facilitator prompts the experts to explore their areas of disagreement. Experts are asked to explain the reasons for their judgments, with reference wherever possible to the evidence dossier. Long discussions often ensue, and can become heated if not managed carefully by the facilitator. An experienced facilitator will be familiar with the possible sources of bias in behavioral aggregation, and needs to be constantly alert. A checklist for managing the discussion would include the following.
Allow discussion all the while it seems to be developing ideas. Don’t let the experts keep repeating the same arguments.
Make sure all opinions are heard and properly considered. Keep bringing quieter members into the discussion, and curtail the more voluble experts if necessary.
Don’t allow arguments to be presented aggressively.
Listen carefully. Try to get a sense of the strengths of competing arguments. Two people giving the same argument doesn’t make it twice as valid.
Highlight and summarize significant points of contention or of agreement before moving on to the next area for discussion. It can be useful to write these on a whiteboard or flipchart. These summaries will be noted in the SHELF2 record, and so it is important for the experts to confirm that they are a fair representation of the discussion.
The facilitator’s role may also be found in other elicitation protocols, but it is particularly important in SHELF.
shows the H2035 SHELF2 record of the group discussion. This part of a SHELF elicitation can take a substantial amount of time, even running to hours in some cases. As the first bullet point above says, it is important to allow discussion, but only as long as it is yielding additional insight. In the H2035 case study, it was possible to bring the discussion to a close relatively quickly.
Table 6 H2035 SHELF2 record, group discussion.
5.5 RIO and Group Judgments
The final stage of the SHELF protocol is for the experts to make group judgments leading to a ‘consensus’, aggregate distribution. It is important to understand, and to make clear to the experts, the nature of this ‘‘consensus.’’ Even after discussing and debating, experts will not reach complete agreement (such that they now have the same knowledge and beliefs about an uncertain quantity, represented by the same probability distribution). Their opinions may be modified by the discussion, but they will inevitably leave the workshop with differing beliefs about the quantity of interest. If, during the workshop, they are coerced into an artificial consensus, it is unclear what the resulting distribution will mean, since it would not represent a true convergence of opinion.
In the SHELF method, the experts are asked to judge what a rational impartial observer, known informally as RIO, might reasonably believe, having seen their individual judgments and listened to their discussion. Experts are advised that RIO would not completely agree with any one expert, but would see merit in the opinions of all the experts. Some arguments will have been more persuasive in the discussion, so that RIO would give more weight to some opinions than others, and it is the experts themselves who are best able to judge this.
Perhaps surprisingly, by taking the perspective of RIO, in my experience experts have never failed to reach agreement on a distribution that they believe represents a rational impartial view of their combined knowledge. Although RIO is a fictional person, it is an abstraction that allows the SHELF protocol to answer the question, “Whose probability distribution is this?” It is, at least notionally, RIO’s distribution. Furthermore, it is this distribution, representing the beliefs of a rational impartial observer, taking into account the combined knowledge, expertise, and reasoning of the group, that in effect is being sought when we conduct an elicitation with multiple experts.
shows the group judgments part of the SHELF2 record in the H2035 case study. Whereas only three methods are permitted for the individual judgments, SHELF has a fourth method that is appropriate for the group discussion. The probabilities method requires the facilitator to choose three X values and to ask the experts to agree on probabilities that RIO might assign to X being above or below those values. This method is not used at the individual judgments stage because the chosen values of X will serve as anchors to bias the experts’ judgments. At the group stage, there have already been very many X values mentioned in various contexts, and introducing new ones is unlikely to cause bias. The quartile and tertile methods are still available for group judgments, but it is recommended to use different methods in the two judgment stages. The advantage of changing the method, and particularly of switching to the probabilities method, is that the experts have to consider their probabilities in a fresh way. Otherwise, for instance, if the experts are asked to assign RIO’s median value they are likely to focus on their individual medians and engage in negotiating a compromise value for RIO, instead of applying the definition of the median.
Table 7 H2035 SHELF2 record, group elicitation.
Table 8 Original and fitted probabilities in the H2035 case study.
The three X values are chosen based on the facilitator’s feelings of what the experts’ RIO judgments are likely to be. The lowest one is placed so that the facilitator thinks the experts will judge there to be a probability of 0.2 to 0.3 below this value, while the highest is placed where they might judge there to be a probability of 0.2 to 0.3 above. The middle one is aiming for a probability of about 0.4 or 0.6 below (but not 0.5, since experts may then find 0.5 an easy, lazy judgment). The objective is for the experts to make a set of judgments that they have to think about and which will span the most probable X values to facilitate fitting a distribution.
also shows how the facilitator acts “on behalf of RIO” in challenging the group judgements. The facilitator needs to feel that the final judgments are indeed reasonable for a rational impartial observer. The fact that SHELF has two rounds of judgments is valuable here, because the facilitator can see each expert’s initial judgments, as well as hearing all the group discussion. If the group judgments suggest less overall uncertainty than the variability in the individual beliefs, and if this does not seem to be justified by the intervening exchange of views, then groupthink may be at work.
It may be noted that the H2035 SHELF2 fails to record any challenge from the facilitator to the experts’ group judgment of . This is unfortunate because the probability of 0.2 seems small, when shows that only expert F gave less than 0.25 to this event in their individual judgments. In this case, the judgment was questioned and the experts had reasons to retain the probability of 0.2. But without that detail in the record, a reader will rightly wonder whether the final judgments are fully defensible. Even experienced facilitators are not infallible and each elicitation offers learning opportunities. Expertise in this, as in any other area, is hard won!
5.6 The Final Distribution
The group judgments are finally used as a basis for fitting a probability distribution. In the individual elicitation round it is not important how well the fitted distributions represent each expert’s initial opinions, because it is primarily their individual judgments that are used to start the group discussion. In contrast, the final fitted distribution is the outcome of the elicitation process, and so must be selected carefully and with full approval of the experts. The SHELF software allows a range of standard distributions to be fitted to the group judgments, including the normal, gamma, beta and lognormal families. In each case, the fit cannot be perfect because the software is trying to match three probabilities with just two adjustable parameters. In discussion with the experts, best-fitting distributions from several families may be considered. The facilitator will give feedback about relevant features of a fitted distribution and how it may fail to match what RIO might think. If necessary, the experts may revise one or more of their group judgments.
In the H2035 elicitation, a Student-t distribution was fitted first but rejected because it did not capture skewness. The final fitted distribution is shown in and compares the three group judgments with the probabilities implied by this distribution.
is a lognormal distribution with origin shifted to –2, and the facilitator pointed out that it implied zero probability for X to be less than –2%. Although this hard constraint might be strictly unrealistic, the experts thought that the fitted distribution was a sufficiently accurate representation of their group judgments, and that the possibility that X might be below –2% could be ignored (from the perspective of RIO). The full H2035 SHELF2 record in the Supplementary Material gives more detail on the process of reaching this final fitted distribution.
6 Multiple Quantities
Before we can conduct an elicitation, no matter which protocol one might favour, it is necessary to decide what quantity or quantities the experts will be asked to provide judgments for. Although this may seem a simple decision—just ask about the quantities that we need their judgments for — in practice, there may be different ways to achieve the study goals, and some may promote careful, scientific judgments better than others.
6.1 Independence and Elaboration
Having more than one uncertain quantity of interest is a common challenge. Even with just two quantities, say X and Y, it is not enough to just elicit a probability distribution for each quantity because the two marginal distributions do not imply the joint distribution of (X, Y). We need to consider how X and Y may be correlated.
To understand what correlation or dependence means in this context, it is important to remember that X and Y are invariably defined to have unique values. They are not repeatable; we cannot imagine plotting a sample of (X, Y) values as a scatter diagram; there will only ever be one point in that diagram, but its location is uncertain. Terms like correlation or independence are defined as properties of an expert’s subjective joint distribution for the location of that (X, Y) point. X and Y are independent if (and only if) the expert’s beliefs about one quantity would not change if new information emerged about the other. Independence is a subjective judgment. For instance, I am uncertain about both the date of the next general election in the UK and the date of the next total lunar eclipse that will be visible from my home. I could find the date of the next lunar eclipse by a simple internet search, but this information would not change my beliefs about the date of the next general election, nor would an announcement of that latter date by the government change my uncertainty about the next total lunar eclipse, so these two uncertain quantities are independent for me (and probably for all readers of this article). If X and Y are independent, then for a single expert it would be enough to elicit (marginal) distributions for them separately. With multiple experts, in the SHELF protocol we can elicit X and Y separately if they would be judged independent by RIO, so this is another question to ask the experts. For the protocols using mathematical aggregation, there is no individual, real or conceptual, whose judgments are represented by the aggregated distribution, so the decision to assume independence and elicit separately is imposed by the facilitator or client.
If X and Y are not independent, then the nature and magnitude of dependence between them needs to be elicited to construct a joint distribution. This requires more complex judgments from the experts. For instance, if we first elicit median and tertiles for X from an expert we could ask for the median of Y both marginally and then conditionally upon X equalling its median, its lower tertile and its upper tertile. Eliciting conditional probability judgments like this requires the expert to make judgments about one quantity if the true value of the other variable was found to be some specific number, or to lie in some specific range. The cognitive challenge here is appreciably more difficult for experts than judgments about a single quantity. Furthermore, there is essentially no research identifying which judgments about two quantities experts understand most clearly or make most reliably. We do not know what new biases may be induced by the forms of questions. With three or more uncertain quantities, these challenges are multiplied enormously.
Two approaches to eliciting a joint distribution for two or more dependent quantities are used in practice. The first is effectively the same as for eliciting a univariate distribution—elicit as few extra judgments as necessary and then fit a joint distribution from a standard multivariate family. SHELF offers templates for two such families. One is for a set of uncertain proportions that must sum to 1, and a Dirichlet distribution is assumed. In this case, beta distributions are elicited for each proportion separately, no additional judgements are made, and a Dirichlet distribution fitted whose beta marginal distributions come as close as possible to the elicited distributions. The other template fits a Gaussian copula, using the elicited marginal distributions and pairwise ‘‘concordance probabilities,’’ that is for each pair of quantities the expert gives their probability that both will be above or both below their separately elicited medians. The concordance probabilities imply correlations in the Gaussian copula, but with three or more quantities the set of such correlations may not correspond to a valid correlation matrix, in which case the facilitator has the challenge of helping the experts to reconcile this noncoherence. Kurowicka and Cooke (Citation2006) describe more complex ways to elicit joint distributions with copulas, but experts are required to make even more difficult judgements, such as partial rank correlation coefficients.
The other principal way to elicit dependence is to avoid it by transforming the quantities. The suggestion above that “there may be different ways to achieve the study goals, and some may promote careful, scientific judgments better than others” refers particularly to this approach. With two quantities, X and Y, if we can identify a one-to-one transformation to and
, such that the experts judge U and V to be independent, then separate, independent distributions can be elicited for U and V and the joint distribution of (X, Y) deduced by the inverse transformation of variables. This is known as elaboration. O’Hagan (Citation2012) discussed elaboration at length, and gives several examples of how elaboration can address different elicitation tasks, not only achieving independence of multiple quantities of interest but also such that the transformed quantities are simpler for the experts to think about individually.
In the H2035 project, the primary quantity of interest was the number of LTCs amongst people aged 85+, but the quantity X defined for elicitation in the case study was not this number but the percentage change in the rate of LTCs per thousand people. This was a result of elaborating the primary quantity of interest as the product of the elicited X, the rate per thousand in 2012 and the population aged 85+ in 2035. These were considered to be three independent quantities. To derive the distribution for the primary quantity of interest, distributions were needed for the other two quantities. There was quite good data available on the rate of LTCs per thousand in this age group in 2012, with well characterized uncertainty, so that a distribution could be derived without requiring expert elicitation. Similarly, population projections for the UK in 2035 gave an estimate for the 85+ age group, again with a well-understood uncertainty. This was an example of what O’Hagan (Citation2012) calls ‘‘elaboration by information sources,’’ to simplify the elicitation of a single quantity of interest by expressing it as a function of several independent quantities for which distributions could more easily be obtained.
6.2 Many Quantities
If the number of quantities of interest is very large, methods that are good for a small number of quantities may become infeasible. If the quantities are not independent, methods based on fitting a standard family of distributions are in my opinion unreliable with more than four or five quantities. Even with independent quantities (perhaps after an elaboration step), constraints on resources and the willingness of experts to give their own time will limit the number of quantities that can be the subject of full and careful elicitation, particularly when using the SHELF or Cooke protocols.
One solution to this problem is proposed in the EFSA Guidance (European Food Safety Authority Citation2014). The process begins with a quick and simple elicitation for each quantity, conducted by the elicitation team using a process of minimal assessment, in which each quantity is given a ‘‘best estimate’’ m and an uncertainty measure s such that the quantity is judged ‘likely’ to be in the range m – s to m + s. These terms are vague but adequate for purpose because they are used only to decide which quantities are most important to be elicited carefully. The next step is a simple sensitivity analysis in which the decision or risk model is run with every quantity set to its m, and then varying each one separately out to m – s and m + s, to see how much the model output changes. Quantities are prioritized for full elicitation according to their sensitivities. The Guidance says that assuming distributions, based on their minimal assessment, is then adequate for quantities for which the output does not vary materially.
An alternative is to apply full elicitation with the SHELF, or possibly Cooke, protocol to a selection of quantities and then to send the same experts a probabilistic Delphi questionnaire. This approach is based on the idea that the experts will first be fully trained to make the necessary probabilistic judgments, thereby mitigating the problem which arises with Delphi of experts not understanding the task properly.
Finally, elaboration can be used to reduce the number of quantities to be elicited if we are prepared to make some assumptions. An example in O’Hagan (Citation2012) is of eliciting judgments about a dose–response relationship. In principle, this means eliciting beliefs about every point on the dose–response curve, an infinite number of quantities. However, it would be natural to assume a standard sigmoid shape, such as a probit function. Then, it is necessary only to elicit judgments about the two parameters of the curve.
In the H2035 project, there was interest not just in the rate of LTCs in people over the age of 85 but in that rate for all age groups. In the SHELF workshop, three distributions were elicited—the change of rate for over 85s, for those between 40 and 65, and for those aged under 18. These were sufficiently separated that the experts felt it reasonable for RIO to judge them independent. Change of rate for intermediate age groups could then be obtained by a quadratic interpolation between these three points, an instance of using elaboration to break correlation and to reduce the number of quantities. All three elicitations in the SHELF workshop were made under conditions of ‘‘business as now,’’ but the H2035 project was also interested in how rates would change under a number of alternative scenarios. A few days after the SHELF workshop, the experts were sent a questionnaire to elicit their judgments about various scenarios, as the first step in a probabilistic Delphi elicitation.
7 Conclusions
Subjective expert judgments play a part in all areas of scientific activity, and should be made with the care, rigor, and honesty that science demands. One area where expert judgment is particularly prominent is the elicitation of expert knowledge in the form of probability distributions for uncertain quantities.
There are many aspects of elicitation where care is needed to avoid introducing biases to the experts’ judgments. Research in psychology has identified a number of cognitive biases to which expert probabilistic judgments may be subject, but formal elicitation protocols have been developed by practitioners to minimize bias and to encourage experts to make their judgments accurately. The three leading protocols are the Cooke, SHELF, and probabilistic Delphi protocols.
The protocols were contrasted in the context of a case study in which the SHELF method was used. The protocols differ primarily in the following respects.
In all protocols, the basic format for eliciting from a single expert is to ask for quantiles, to avoid one source of anchoring bias. The Cooke method typically asks for 5th, 50th and 95th percentiles, although in my opinion there is good experimental evidence to suggest that it is unwise to rely on judgments of 5th and 95th percentiles to characterize uncertainty. SHELF usually asks for median and quartiles or median and tertiles, and does so in a structured sequence of judgments designed to minimize other sources of anchoring bias. The probabilistic Delphi protocol, as defined originally in the European Food Safety Authority (Citation2014) guidance, employs the same sequence of judgments as SHELF, although the IDEA variant is less prescriptive and may use the same judgments as in the Cooke protocol.
Although these protocols provide important disciplines for eliciting judgments from a single expert, or even for a single scientist eliciting his or her own judgments, formal expert knowledge elicitation usually involves consulting multiple experts. It is here that the three protocols are most strongly differentiated.
When multiple experts are consulted, SHELF uses behavioral aggregation, with the experts meeting together and agreeing on a final probability distribution representing what a rational impartial observer (RIO) might believe. The other two methods rely on mathematical aggregation, in which a distribution is elicited from each expert and the distributions combined by a formula. In the Cooke protocol, this involves weighting the experts according to their performance on ‘‘seed variables.’’ The distributions resulting from a mathematical aggregation do not represent the belief of any person, real or conceptual, and so their status as subjective probability distributions is unclear.
All the leading protocols demand substantial input of resources, particularly the time of the experts, the facilitator and those organizing the elicitation. Elicitation should not be seen as a cheap option. However, the result is information, elicited from experts in a rigorous and scientific manner. To obtain experimental data of the same information content and quality will almost invariably require much more resources.
The purpose for which the information is sought may not require such high standards, and in that case it would be possible to reduce and simplify the process. Judgments may be sought from fewer experts, possibly just one, and the elicitation may be done without the aid of a trained facilitator. For instance, if the purpose is Bayesian statistical inference, if the elicitation is of prior distributions and if the data will provide strong information, then formal, rigorous elicitation may be needed for only a small number of parameters, and perhaps for none. Similarly, if the elicited distributions will be for inputs to a decision model, the decision may be insensitive to the uncertainty in some of those inputs, in which case it will not be necessary to elicit them so carefully and “minimal assessment” may be adequate.
Supplemental Material
Download PDF (173.2 KB)Acknowledgments
I am grateful to the Department of Health for permission to use the H2035 case study and in particular to Matt Edwards, the Department’s Head of Horizon Scanning and International Workforce Information and Analysis, for several helpful comments on this article. It should be noted that some details of the elicitation have been changed in this article, to more clearly illustrate some concepts but without affecting the work’s implications within the Horizon 2035 project.
I am also grateful to the reviewers for making many very useful suggestions that have greatly improved this article.
References
- Brownstein, N., Louis, T., O’Hagan, A., and Pendergast, J. (2018), “The Role of Expert Judgement in Statistical Inference and Evidence-Based Decision-Making,” The American Statistician, this issue, DOI: 10.1080/00031305.2018.1529623.
- Burgman, M. A. (2015), Trusting Judgements: How to Get the Best Out of Experts, Cambridge, UK: Cambridge University Press.
- CFWI (2015), Horizon 2035 – Future Demand for Skills: Initial results. National Archives, available at https://www.gov.uk/government/publications/horizon-2035-future-demand-for-skills-initial-results
- Cooke, R. M. (1991), Experts in Uncertainty: Opinion and Subjective Probability in Science, Oxford, UK: Oxford University Press.
- Cooke, R. M., and Goossens, L. H. J. (2008), “TU Delft Expert Judgement Database,” Reliability Engineering and System Safety, 93, 745–756. DOI: 10.1016/j.ress.2007.03.017.
- Cooke, R. M., Wittmann, M. E., Lodge, D. M., Rothlisberger, J. D., Rutherford, E. S., Zhang, H., and Mason, D. M. (2014), “Out-of-sample Validation for Structured Expert Judgement of Asian Carp Establishment in Lake Erie,” Integrated Environmental Assessment and Management, 10, 522–528. DOI: 10.1002/ieam.1559.
- de Finetti, B. (1937), “Foresight: Its Logical Laws, its Subjective Sources,” Reprinted (and translated from the original French) in Studies in Subjective Probability, eds. H. E. Kyburg, Jr. and H. E. Smokler, New York: Wiley, 1964.
- de Finetti, B. (1970), Theory of Probability: A Critical Introductory Treatment (translation by A. Machi and A. F. M. Smith, 1974–5), 2 volumes. Wiley.
- European Food Safety Authority (2014), “Guidance on Expert Knowledge Elicitation in Food and Feed Safety Risk Assessment,” EFSA Journal 2014, 12 (6), 3734.
- Fischhoff, B., Slovic, P., and Lichtenstein, S. (1978), “Fault Trees: Sensitivity of Estimated Failure Probabilities to Problem Representation,” Journal of Experimental Psychology: Human Perception and Performance, 4, 330–344.
- French, S. (1985), “Group Consensus Probability Distributions: A Critical Survey,” in Bayesian Statistics 2, J. M. Bernardo et al (eds.), Oxford, UK: Oxford University Press, pp. 183–202.
- Gosling, J. P. (2018), “SHELF: The Sheffield Elicitation Framework,” in Elicitation: The Science and Art of Structuring Judgement, eds. L. C. Dias, A. Morton and J. Quigley, Springer, pp. 61–93.
- Hanea, A. M., McBride, M. F., Burgman, M. A., and Wintle, B. C. (2018), “Classical Meets Modern in the IDEA Protocol for Structured Expert Judgement,” Journal of Risk Research, 4, 417–433. DOI: 10.1080/13669877.2016.1215346.
- Hemming, V., Burgman, M. A., Hanea, A. M., McBride, M. F., and Wintle, B. C. (2018), “A Practical Guide to Structured Expert Elicitation using the IDEA Protocol,” Methods in Ecology and Evolution, 9, 169–1980. DOI: 10.1111/2041-210X.12857.
- Janis, I. L. (1972), Victims of Groupthink: A Psychological Study of Foreign-Policy Decisions and Fiascoes, Boston, MA: Houghton Mifflin.
- Kahneman, D. (2011), Thinking, Fast and Slow, New York: Farrar, Straus and Giroux.
- Kurowicka, D., and Cooke, R. M. (2006), Uncertainty Analysis with High Dimensional Dependence Modelling, Chichester: Wiley.
- Oakley J. E., and O’Hagan, A. (2016), “SHELF: the Sheffield Elicitation Framework” (version 3.0), School of Mathematics and Statistics, University of Sheffield, UK, available at http://tonyohagan.co.uk/shelf.
- O’Hagan, A., Buck, C. E., Daneshkhah, A., Eiser, J. R., Garthwaite, P. H., Jenkinson, D. J., Oakley, J. E., and Rakow, T. (2006), Uncertain Judgements: Eliciting Expert Probabilities, Chichester, UK: Wiley.
- O’Hagan, A. (2012), “Probabilistic Uncertainty Specification: Overview, Elaboration Techniques and their Application to a Mechanistic Model of Carbon Flux,” Environmental Modelling and Software, 36, 35–48. DOI: 10.1016/j.envsoft.2011.03.003.
- Parducci, A. (1963), “The Range-Frequency Compromise in Judgment,” Psychological Monographs, 77, 1–50. DOI: 10.1037/h0093829.
- Ramsey, F. P. (1926), “Truth and Probability,” reprinted in Studies in Subjective Probability, eds. H. E. Kyburg, Jr. and H. E. Smokler, 2nd edition, New York: R. E. Krieger Publishing Company, pp. 23–52.
- Rowe, G., and Wright, G. (1999), “The Delphi Technique as a Forecasting Tool; Issues and Analysis,” International Journal of Forecasting, 15, 353–375. DOI: 10.1016/S0169-2070(99)00018-7.
- Savage, L. J. (1954), The Foundations of Statistics. New York: Wiley.
- Tversky, A., and Kahneman, D. (1974), “Judgments under Uncertainty: Heuristics and Biases,” Science, 185, 1124–1131. DOI: 10.1126/science.185.4157.1124.