ABSTRACT
In today’s world shaped by technology and the natural sciences, knowledge and skills related to experimentation are crucial, especially given growing public debates about science-related topics. Despite a strong emphasis on experimentation in science curricula worldwide, learners still encounter diverse challenges when experimenting. This paper provides a systematic review of empirical research on learners’ challenges during the following inquiry phases: stating research questions, generating hypotheses, planning and conducting an experiment, analysing data and drawing conclusions. A database search and an analysis of two prior narrative literature reviews identified 368 studies, of which 66 were used for further analyses after screening for eligibility using specific inclusion criteria. The analyses revealed 43 challenges at the conceptual, procedural and epistemic level that not only elementary but even university students face during experimentation. Additionally, cognitive biases and preconceptions are identified as a source of such challenges. Overall, the findings demonstrate a lack of in-depth research on stating research questions despite its importance for experimentation, whilst learners’ abilities in the other inquiry phases have been intensively investigated. The results offer valuable information for science education research and provide a basis for tailored scaffolding in the science classroom or the design of effective instructional interventions.
1. Introduction
To cope with the demands of the twenty-first century and become more critical citizens, learners need to be able to use their understanding of science to contribute to public debate and develop informed opinions on science- and technology-based questions (OECD, Citation2018). Underpinning this important goal is the notion of scientific literacy (Bybee, Citation1997). Scientific literacy allows people to address, make decisions and take action on complex problems in a value-driven, scientifically knowledgeable and empowered manner (Abd-El-Khalick et al., Citation2004; Adams et al., Citation2018). Engaging learners in activities in which they apply scientific methods similarly to professional scientists (Keselman, Citation2003) is seen as one important way to foster scientific literacy and has therefore become a fundamental component of science teaching and learning (Rönnebeck et al., Citation2016). Conducting experiments is considered a particularly central investigative activity within scientific methods (Pedaste et al., Citation2015). Consequently, science standards and curricula worldwide emphasise the importance of experiments in the science classroom and seek to systematically foster knowledge and skills necessary for learners to understand and perform experiments (e.g., DfES & QCA, Citation2004; KMK, Citation2005a, Citation2005b, Citation2005c; NRC, Citation1996, Citation2000, Citation2012, Citation2013). One prominent science education strategy to engage learners in experimentation is inquiry-based learning (IBL; Rönnebeck et al., Citation2016). By directing their own investigative activities in the form of experiments, learners can gain insights into how scientific knowledge is produced (Arnold et al., Citation2014) and how scientists establish the credibility of the claims they advance (Osborne, Citation2014). However, while experimentation opens up rewarding learning opportunities, it also presents great challengesFootnote1 to learners. In this regard, numerous studies show that experimentation is a complex task, and learners’ abilities to state research questions, generate hypotheses, plan and conduct experiments, analyse data and draw conclusions are critical components for successful IBL (De Jong & van Joolingen, Citation1998; Zimmerman, Citation2007).
Identifying and analysing learners’ challenges will not only render learners’ implicit conceptions or errors explicit, but also allow these difficulties to be directly addressed in order to challenge and transform them, while acknowledging them as an important antecedent for learning (Siler & Klahr, Citation2012). This can be achieved if challenges are used to derive potential from them: learners’ challenges can potentially serve as a template for creating effective guidance and scaffolding and later for adapted lesson planning (Baur, Citation2015; Van Uum et al., Citation2017). Moreover, deeper knowledge of learners’ challenges is crucial not only in science education, but in science education research as well. Identifying and analysing learners’ challenges makes it possible to examine the reasons for their existence in greater detail. In this way, tailored support methods can be developed and empirically tested for their effectiveness in overcoming specific challenges in order to develop successful IBL concepts and interventions. In addition, challenges can potentially be linked to specific knowledge and skills needed in the investigative process.
Thus, the objective of this systematic literature review is to summarise and describe empirical research findings on learners’ challenges with respect to understanding and performing experiments. For this purpose, the article first presents theoretical background information on IBL and experiments. Next, it identifies knowledge and skills required for experimentation, which serves as a crucial baseline for analysing related challenges. Afterwards, the methodology and results of the newly conducted review are presented. Finally, the results are discussed and implications are derived.
2. Inquiry-based learning (IBL) and experiments
IBL has been considered as an essential component of science education for more than 50 years (Stender et al., Citation2018). Due to its long-lasting importance and the extensive literature on the topic, the term IBL is accompanied by a variety of descriptions and connotations (Abrams et al., Citation2008; Anderson, Citation2002; Blanchard et al., Citation2010). In this regard, Rönnebeck et al. (Citation2016) argues that inquiry is not only understood as a way for individuals to learn science and scientific ways of obtaining knowledge through different contents and materials but also as an instructional approach (Bybee, Citation2006; Furtak et al., Citation2012). Inquiry as an instructional approach can be defined along different forms of instruction (Blanchard et al., Citation2010; Furtak et al., Citation2012; Hmelo-Silver et al., Citation2007), ranging from minimally guided, discovery-oriented approaches in which learners engage in hands-on activities (e.g., Kirschner et al., Citation2006) to more structured forms, like traditional (verification) laboratory instruction, where a distinctive list of activities is predefined (Blanchard et al., Citation2010). The National Research Council (NRC, Citation1996) defines IBL as activities in which learners develop knowledge and understanding of scientific ideas, as well as an understanding of how scientists study the natural world and propose explanations based on the evidence derived from their work. This definition focuses on aspects from the learner’s point of view, not only on acquiring knowledge, but also on developing ways of scientific thinking and working as well as gaining an understanding of those methods. Furthermore, for this review we define IBL in terms of three dimensions (Duschl, Citation2008; Furtak et al., Citation2012): the conceptual, epistemic and procedural domain. In the PISA assessment, it is referred to as content, epistemic and procedural knowledge (OECD, Citation2019). The conceptual domain contains declarative knowledge, also referred to as substantive knowledge, consisting of learners understanding facts, theories, and principles of science, meaning science as a body of knowledge. If investigation methods are explicit learning content (gaining an understanding of those methods), then the concepts of these methods (such as: ”What exactly is an experiment?” or ”What is a research question?”) can also be understood as a part of declarative knowledge and must therefore be seen as a part of this specific content domain. The epistemic domain is based upon learners’ comprehension of how scientific knowledge is generated, meaning an understanding of the role of specific constructs and defining features essential to the process of building scientific knowledge (Duschl, Citation2008). ‘Epistemic knowledge includes an understanding of the function that questions, observations, theories, hypotheses, models and arguments play in science’ (OECD, Citation2019, p. 100). Furthermore, it describes an understanding of the rationale for the common practices of scientific inquiry, the status of claims that are generated and the meaning of foundational terms such as hypothesis, variable and theory (OECD, Citation2019). The focus lies on learning to develop explanations for phenomena and to understand that gained scientific knowledge is subject to change in the face of new evidence or new interpretations of old evidence, similar to the practice of professional scientists. In relation to IBL, in the literature procedural knowledge is referred not only to the knowledge of how to do something, of techniques and specific methods of a discipline, but also to the knowledge of scientific processes used to establish scientific knowledge (e.g., Gott & Duggan, Citation1995; Roberts et al., Citation2010). Hence, in this interpretation, it includes the knowledge of the practices and concepts on which empirical inquiry is based (see, Millar & Lubben, Citation1996). In this literature review, we define experimentation as a learning content and therefore focus mainly on the practices, the – procedural knowledge – in the procedural dimension. The procedural domain focuses in this review especially on hands-on performances and practically performing the scientific investigation.
As already explained above, a common and important type of scientific investigation in IBL settings is the experimental method (Osborne et al., Citation2003). The term experiment is often used as a synonym for other scientific investigations because it can include methods such as describing, observing, comparing or classifying (Mayer & Ziemek, Citation2006). However, an experiment goes beyond observation for example, as an experimenter controls the artificially altered conditions and intentionally intervenes in the process. Thus, although the term ‘experiment’ is used for different types of investigations (Arnold et al., Citation2014), it is generally considered the method of choice for investigating causal relationships between dependent and independent variables (Wellnitz & Mayer, Citation2011). This is also referred to as ‘fair testing’ (Gott et al., Citation2008).
Experimentation is often described as a problem-solving process (e.g., Klahr & Dunbar, Citation1988) in which ideas are tested (Osborne et al., Citation2003) to draw valid inferences about causal hypotheses based on the evidence derived (NRC, Citation2013). Depending on the goals, content, as well as the institutional setting, different types of experiments can be distinguished (e.g., Mayer & Ziemek, Citation2006; Roberts & Gott, Citation2006). The experiment is subject to the quality criteria of scientific work: objectivity, reliability and validity. The objectivity of an experiment ensures intersubjective comprehensibility, reliability ensures the measurement accuracy and reliable repeatability of an experiment, and validity ensures that the experiment really measures what it is intended to measure (Gott et al., Citation2008). The experimental process follows a hypothetico-deductive method (Popper, Citation1966) that typically consists of several logically connected phases, also known as inquiry phases. From a pedagogical point of view they guide students through the experiment as they subsequently organise the inquiry process and draw attention to important features of scientific thinking (Pedaste et al., Citation2015). These inquiry phases require learners to actively participate in inquiry activities; coordinating related knowledge and skill simultaneously (NRC, Citation2013). Although inquiry phases are logically connected, inquiry cannot be seen as a uniform linear process, but rather as a cycle in which multiple links, overlaps and interconnections between phases are possible (Pedaste et al., Citation2015; Rönnebeck et al., Citation2016). Descriptions of inquiry cycles by different researchers sometimes use different terms for phases that are essentially the same (Rönnebeck et al., Citation2016). Nevertheless, the successful application of inquiry in different settings suggests an underlying commonality that is at the core of IBL (Pedaste et al., Citation2015).
As our focus in this review lies on experimentation, the goal was to cover the main phases of this kind of scientific investigation. Thus, a conceptualisation was needed which targeted key components of experimentation instead of more broader concepts such as scientific inquiry or inquiry in general (e.g., Pedaste et al., Citation2015; Rönnebeck et al., Citation2016). The model used in this review is a synthesis of existing activity-based conceptualisations, which have lead to four main phases and related activities of IBL through experimentation: 1) stating scientific questions, 2) generating hypotheses 3) planning and conducting an experiment, and 4) analysing data and drawing conclusions (Arnold et al., Citation2014; Bruckermann et al., Citation2017; Hofstein et al., Citation2005; Kremer et al., Citation2013, Citation2019; Mayer, Citation2007; Mayer et al., Citation2008; Meier & Mayer, Citation2012). These inquiry phases can be found in many other conceptualisations (e.g., Bybee, Citation2006; Pedaste et al., Citation2015; Rönnebeck et al., Citation2016). Furthermore, this conceptualisation was selected because it is applicable to the conceptualisations used by empirical studies found in the data search from different disciplines covering IBL and experimentation in particular. Because of the overlaps with many other conceptualisations and its interdisciplinary nature, the conceptualisation seemed like an adequate basis for this review. To carry out each of these inquiry phases, learners need a variety of skills and knowledge during experimentation, which are described in the following paragraphs:
Stating scientific questions
Formulating research questions is important in problem-solving procedures (Cuccio-Schirripa & Steiner, Citation2000), because it usually represents the start of the research process (Bell et al., Citation2005), the pursuit of which advances and extends knowledge on a certain topic (Chin & Osborne, Citation2008; White & Frederiksen, Citation1998). The research question underlying an experiment focuses on the cause of an observed phenomenon (Pedaste et al., Citation2015) and creates a link to relevant prior knowledge. As an experiment investigates causal relationships between the dependent and independent variable(s), research questions ask about those causal relationships and can then be answered through the subsequent experimental process.
Generating hypotheses
Hypotheses provide plausible, justified answers to research questions (Eastwell, Citation2014). Hypotheses seeking to explain the investigated phenomenon are consistent with prior knowledge, theories or principles (Arnold et al., Citation2018) and align with the stated research question (Klahr & Dunbar, Citation1988). Accordingly, hypotheses are predictions about a presumed relationship between an independent variable (or variables) and its effect on a dependent variable (Gijlers & de Jong, Citation2005). Therefore, in order to formulate a hypothesis, the dependent and independent variable must first be identified by observing a phenomenon (Pedaste et al., Citation2015). Moreover, hypotheses are formulated as testable conclusions to inform the subsequent research process (Kremer et al., Citation2019).
Planning and conducting an experiment
Planning and conducting an experiment is done to answer the stated research question and test the generated hypotheses (Arnold et al., Citation2018). Setting up an experiment includes choosing the object of investigation and specifying the sample by determining the characteristics to be measured (Pedaste et al., Citation2012). This inquiry phase also includes determining the time periods, number of measurements and repetitions to be conducted (Germann et al., Citation1996). To ensure that the experimental results are comparable, test and control conditions (Germann et al., Citation1996) or a series of measurements need to be included. After methods and procedures are logically outlined, proper measurement equipment needs to be set up, safety precautions followed, and ultimately a sufficient number of trials to validate the results must be considered (Chang et al., Citation2011). When carrying out the experiment, it is crucial to manipulate variables in a scientific manner: whilst the independent variable is systematically varied to investigate its potential effect on the dependent variable, all other potential variables need to be held constant or ‘controlled’ across experimental conditions. This principle is often referred to as the control-of-variables strategy (Chen & Klahr, Citation1999). Furthermore, the experiment is recorded in the form of a laboratory report, documenting how the experiment was planned and conducted (Garcia-Mila et al., Citation2011).
Analysing data and drawing conclusions
The gathered data is analysed to establish evidence and build a link between the evidence and the conclusion through logical thinking, ultimately forming a model or an explanation (Chang et al., Citation2011). A first step in this process is to present the data gathered during the experiment in a clear manner by converting it into visualisations such as tables or graphs (Croner, Citation2003). Furthermore, the data quality is examined to assess certainty and limitations before determining to what extent the data supports the hypothesised relations (Millar & Lubben, Citation1996). Only then, it is possible to synthesise the results correctly and find meaningful patterns. Afterwards, the findings can be placed in relation to the previously stated research questions in order to evaluate the hypotheses regarding the causal relationship between the dependent and independent variables. Drawing inferences about causal hypotheses can contribute to explaining the cause of a phenomenon (Klayman & Ha, Citation1989). The data analysis also often leads to new research questions or modifications to the original hypotheses, which must then be tested again (Arnold et al., Citation2014), meaning that the inquiry cycle might begin anew.
3. Research goals
The sustained attention paid to IBL and experimentation in science education research over the past decades is reflected in two prior narrative reviews on a similar topic (De Jong & van Joolingen, Citation1998; Zimmerman, Citation2007). Whilst both have experimentation as their area of focus, their areas of application differ: De Jong and van Joolingen’s review (De Jong & van Joolingen, Citation1998) sought to determine the effectiveness and efficiency of discovery learning in simulation environments and identify problems learners encounter during experimentation in this specific kind of learning setting. Zimmerman’s (Citation2007) literature review sought to describe the development of scientific thinking and scientific inquiry, mainly in elementary and middle school, including experimental design, evaluating evidence and drawing inferences. Neither of the earlier reviews specifically and solely focuses on challenges learners face when experimenting, but both have overlapping content in this regard. Another reason for completing a new review is that the inquiry phase ‘state research question’ was not included in either of the previous reviews. We therefore conducted a new systematic review with three goals in mind. First, given the different priorities of the previous narrative reviews and when they were carried out (15 and 24 years ago), the current systematic review aims to not only provide a more up to date overview but also expand and deepen knowledge of learners’ challenges during experimentation, making it the primary focus of the review. Second, the present systematic review analyses issues of both theoretical (conceptualisation of challenges related to specific inquiry phases) and practical relevance (e.g., information about assessment type, context domain), resulting in a systematical overview that captures the challenging components of each of the aforementioned inquiry phases during experimentation. Third, the results should be valuable for future research, as they can reveal current areas of emphasis and outstanding desiderata to inform the development of effective learning interventions and scaffolds. Likewise, the findings can be valuable for practical and conceptual work in schools, as they should provide detailed insights into IBL processes and experimentation in particular, which can be used to conceptualise effective lessons and tailored scaffolds to support learners.
4. Method
For the reasons explained above, we conducted a new systematic literature review by searching four databases representing different disciplines of interest (e.g., education, psychology, social sciences), the two previous literature reviews (De Jong & van Joolingen, Citation1998; Zimmerman, Citation2007) and analysing the resulting data set. As systematic reviews aim to make sense of large quantities of information by identifying salient themes and gaps in existing knowledge (Littell et al., Citation2008), we used Petticrew and Roberts (Citation2006) method of review for the social sciences to systematically review the literature on learners’ challenges in understanding and performing experiments. Thus, we documented the research process in detail, including the definition of keywords, the selection of papers for analysis, the definition of inclusion criteria to refine the search, and the analysis of the data itself (see, ), which ensures transparency and replicability, as illustrated in other reviews (e.g., Rönnebeck et al., Citation2016). All publications included in the data analysis were read several times by the first and second authors and reviewed with respect to the inclusion criteria. Finally, descriptions of the reported challenges were extracted from the original studies. The identified challenges and their assignment to the different inquiry phases was verified via expert ratings and their interrater reliability. Study properties were coded in accordance with a coding scheme; interrater reliability was calculated here as well.
Figure 1. Flowchart of the search procedure and data analysis used for the systematic literature review, following PRISMA guidelines (Page et al., Citation2021) .
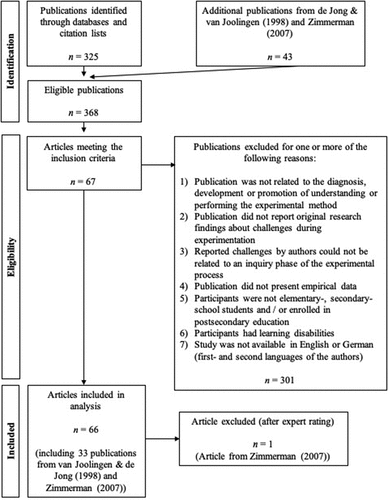
4.1 Search procedure
Initially, all studies analysed by De Jong and van Joolingen (Citation1998; n = 21) and Zimmerman (Citation2007; n = 26) describing learners’ challenges during experimentation were included in our sample of potentially relevant studies. Three duplicates were excluded. Another publication (from De Jong & van Joolingen, Citation1998) was excluded because the study was not available. The remaining 43 studies were added to a dataset. For the database search, we selected databases representing different disciplines that address IBL, science education and psychology: Education Resource Centre (ERIC) by the US Department of Education (educational science), Fachportal Pädagogik (a German educational science database) by the Leibniz Institute for Research and Information in Education, Google Scholar (interdisciplinary, including the educational, social, and natural sciences, humanities and psychology) and Web of Science by Thomson Reuters (social and natural sciences, humanities). To obtain relevant articles, the following criteria were used to formalise the search procedure: (1) relevant search terms (keywords) were used; (2) the publications must have been peer reviewed; (3) the publications had to be journal articles; (4) the articles had to have been published after 1998 (earliest option in the database (ERIC)). Keywords for the database search were defined in order to include all potentially relevant articles for the literature review. Therefore, in choosing keywords, we focused both on broader terms related to inquiry and experimentation (e.g., ‘experimentation’ or ‘scientific experiment’) as well as more specific terms describing the different inquiry phases (e.g., ‘planning an experiment’) or combinations of these (e.g., ‘stating research question’ AND ‘experiment’). The keywords chosen for the literature search were: scientific experiment, scientific investigation, scientific inquiry, experiment, state research questions, generate hypotheses, plan an experiment, conduct an experiment, data analysis, draw conclusions, control of variables strategy, confounded experiments and variations of these. The queries were altered or translated depending on the database language.
4.2 Data analysis
After checking titles and abstracts, we found 325 studies that fit our search procedure criteria and added these to the database. Next, all peer-reviewed full-text articles, totalling 368, (including 43 studies by De Jong and van Joolingen (Citation1998) and Zimmerman (Citation2007)) were examined in order to select eligible articles based on the following inclusion criteria:
The article was related to the diagnosis, development or promotion of learners’ understanding and/or ability to perform experiments.
The article reported original research findings regarding challenges during experimentation. Studies describing only participants’ general learning performance or the effects of intervention studies were excluded, as they do not fall within the objectives of this literature review. A reported finding was noted as a challenge of interest for this work if it compromises at least one of the inquiry phases mentioned above (see, Section 2).
At least one of the described challenges could be related to one or more of the four inquiry phases used to conceptualise this paper (see, Section 2).
The article presented empirical data. Theoretical papers or descriptions of activities were excluded.
The study participants were elementary and/or secondary school students or enrolled in post-secondary education (e.g., university students).
The participants were learners without learning disabilities.
Of the 368 articles assessed for eligibility, 67 articles fulfilled all of the inclusion criteria, including 12 articles analysed by De Jong and van Joolingen (Citation1998), 18 analysed by Zimmerman (Citation2007), and three articles that were part of both reviews. Articles presented in the literature reviews by De Jong and van Joolingen (Citation1998) and Zimmerman (Citation2007) were integrated into the analysis and marked as such. A summary of the study selection procedure is presented in .
All articles included in the analysis were read several times and reviewed with respect to the reported challenges. Afterwards, descriptions of the challenges mentioned in the original articles were extracted. Overall, 52 challenges were identified. The identified challenges and their assignment to the different inquiry phases were then verified via expert ratings. The selected experts are all characterised by considerable experience in experimentation and/or IBL (as both practitioners and researchers) and stem from different disciplines of science education (biology, chemistry, and physics education) to represent the range of approaches to IBL and experimentation in science education. In addition, the goal was to achieve a compilation of international experts that would lead to an assessment that is as broadly applicable as possible, for example, not tied to a specific national educational framework. Following these selection criteria, 17 international experts (from Austria, Cyprus, Finland, Germany, and Switzerland) with large experience in the field of experimentation and/or IBL stemming from various fields of science education were asked to assign the 52 identified challenges to the four inquiry phases. The experts achieved a mean agreement of 87% (median of Krippendorff’s alpha = 0.85). Based on the experts’ modal response each challenge was then assigned to one of the four inquiry phases. Acknowledging, however, that there are not always sharp distinctions and these inquiry phases are often very closely related in practice (NRC, Citation2013), multiple responses by individual experts for each challenge were accepted. In the case of low interrater reliability (Krippendorff’s alpha < 0.60), a discussion between the first and second author took place. In such cases, the expert response patterns (see Appendix) and experts’ comments were taken into consideration to ensure the consistent application of the inclusion criteria. Based on this process, it was determined that eight challenges with low interrater reliability could be related to at least two inquiry phases; they were therefore assigned to the category ‘multiple inquiry phases’. Another eight challenges were excluded because the experts did not classify them as a challenge or the description (definition) seemed unclear. As a result, one study from Zimmerman’s review was excluded because none of its identified challenges made the final cut (n = 66). In total, 43 challenges could be assigned to the phases of stating research questions, generating hypotheses, planning and conducting an experiment, analysing data and drawing conclusions, or multiple inquiry phases. Based on the results of the expert ratings and authors’ discussions, eleven of the 43 identified challenges were classified as specific learners’ approaches (marked with an asterisk in the results section). In line with Baur (Citation2018, Citation2021) these approaches are understood as procedures that do not contradict the experimentation process or compromise a particular inquiry phase or the experimental results in general. However, they provide the opportunity to make the experimental process more difficult or could lead to complications in the long run. Hence, specific learners’ approaches do not represent errors, but may impede the further development of competencies for experimentation. An example of a specific learners’ approach categorised as such by the experts is ‘learners working without a hypothesis’ (H2*; e.g., Dunbar & Klahr, Citation1989). Although this approach comprises core activities falling within the inquiry phase of generating hypotheses, it is still possible to conduct an experiment without stating a hypothesis and does not automatically compromise the outcome of the experiment per se. Nevertheless, not generating hypotheses makes it hard to coordinate theory (hypotheses) with the experimental results in order to solve the problem, which is why in educational settings attention needs to be drawn to this kind of learner approach.
The 66 studies reporting on learners’ challenges during experimentation were further analysed by the authors with regard to assessment type(s), context domain(s), sample size(s), grade level(s) and target group(s). The first author and a trained research assistant both coded 14 studies, or about 20% of the total. They reached a mean level of agreement of 97%, with a median Cohen’s Kappa of 1.0.
5. Results
The review of 368 articles led to 66 incorporated studies and 43 challenges learners encounter during the respective inquiry phases (see, ). Eleven of the 43 identified challenges are classified as specific learners’ approaches (marked with an asterisk; see, Section 4.2). The analysed studies were published between 1960 and 2018. The main target populations were K-12 students (n = 43) in secondary schools (n = 14), elementary schools (n = 15) or other educational institutions (n = 14), as well as university students (n = 19). Three studies compared school or university students with non-student populations. The sample sizes varied, with studies ranging from 4 to 1006 participants; about half of studies had 50 or fewer participants. All studies were published in English except for four studies that were only available in German. The analysis results are presented in five sections, relating to each of the four inquiry phases as well as challenges related to multiple inquiry phases. When contextualising the results, it is important to keep in mind that studies can address multiple target groups and context domains and include different types of assessments.
Figure 2. Number of challenges during the inquiry phases and number of studies reporting each challenge (studies can report more than one challenge).
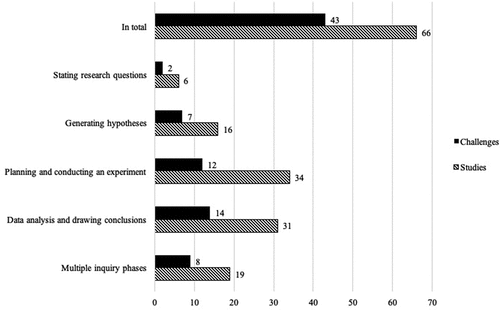
5.1 Stating research questions
Five articles addressed two challenges learners can have when stating research questions (Q1 – Q2*; see, and ). All five studies were part of the database search conducted for the current review. No studies included in De Jong and van Joolingen (Citation1998) or Zimmerman’s (Citation2007) literature review addressed challenges during the inquiry phase of stating research questions. Four of the five studies reported that learners do not state causal research questions (Q1) and therefore ask more factual type questions. Factual questions cannot be investigated in an experiment, as experiments generally investigate potential causal relationships between variables. An example of a factual question would be: ‘How is a heart constructed?’ or ‘What is in this lotion?’. The sample sizes of the studies in this category ranged from 53 to 181 participants. They all examined secondary school students in grades 7, 10 and 12 and conducted paper-pencil tests. Whilst Cuccio-Schirripa and Steiner (Citation2000) examined several context domains (biology, earth and space science and physics), the other three studies (Hofstein et al., Citation2005; Neber & Anton, Citation2008a, Citation2008b) used phenomena from the discipline of chemistry. The second challenge identified was reported by Hofstein et al. (Citation2004), who noted that learners state questions that are qualitative in nature (Q2*). An example of a qualitative type question would be: ‘Does a plant produce oxygen with the help of sunlight?’. However, while Hofstein et al. (Citation2004) consider qualitative questions to be lower-level research questions, qualitative questions are not necessarily unscientific or untestable, as experimentation can also investigate qualitative relationships. Particularly, if little is known about the context being investigated, an exploratory approach can provide valuable insights and information about a system. However, if learners only state research questions that are qualitative in nature, this could reflect that they have difficulty proposing quantitative and therefore higher-level research questions (Hofstein et al., Citation2004). An example of a quantitative question would be: ‘What effect does the intensity of sunlight have on the production of oxygen in a plant?’. Ideally, research goal and type of research question should match. Hofstein et al. (Citation2004) observed this challenge when examining 25 groups in grades 11 and 12 of secondary school. They assessed the inquiry phases by analysing laboratory reports produced during experimentation and by observing learners in experimental settings in the context domain of chemistry.
Table 1. Overview of empirical studies reporting about learners’ challenges when stating research questions during experimentation and selected properties.
5.2 Generating hypotheses
A total of 16 studies reported seven challenges learners encounter when generating hypotheses (H1 – H7*; see, and ). Seven studies originate from the current review, one from De Jong and van Joolingen (Citation1998), seven from Zimmerman (Citation2007), and one from both literature reviews. Sample sizes ranged from 6 to 498 participants, and grade levels ranged from third to eighth grade. Ten studies took place at the school level, meaning in secondary (n = 4) and elementary school (n = 4) as well as not further specified school levels (n = 2). Four studies were conducted with university students, whilst two studies compared children with university students and adults. Four studies describe challenges that children and even university students still have when generating hypotheses: they do not know what a hypothesis is (H1; Njoo & de Jong, Citation1993), they work without a hypothesis (H2*; Park, Citation2006), they operate abductively (H3; García-Carmona et al., Citation2017), and they only generate hypotheses that seem plausible to them (H6*; Penner & Klahr, Citation1996b). Two studies compared different target groups, such as adults and secondary school students (Klahr et al., Citation1993; Schauble, Citation1996). These studies applied various assessment types, including paper-pencil tests, in which, for example, elementary school students had to predict the effects of differences in the speed of a swing, which presumably relates to simple pendulum motion (Kwon et al., Citation2006). Also video-recorded laboratory sessions were used as assessment tools, where two practical experiments with a similar structure, a covariation and a non-covariation case, were conducted (Kanari & Millar, Citation2004). One of these was also a pendulum task, in which students were asked to investigate the relation between the length and weight of a pendulum and its swing time. In the other experiment, students were asked to investigate the relation between the mass of a small box and the area of its bottom surface on the one hand and the force needed to pull it along a level surface on the other. The students could choose among a predetermined set of hypotheses to investigate. Interviews were conducted afterwards, which suggested that students only selected hypotheses predicting the independent variable to have an influence on the dependent variable (H4*), which is also supported by other studies (Klahr et al., Citation2007; Schauble, Citation1990, Citation1996). Overall, the following assessment types were used: audio recordings of laboratory sessions (n = 2), computer simulations (n = 5), interviews (n = 8), laboratory reports produced during experimentation (n = 8), observations of learners in experimental settings (n = 3), paper-pencil tests (n = 7) and video recordings of laboratory sessions (n = 4). Not surprisingly, most test instruments concerned specific science contexts: physics (n = 7), biology (n = 2), logic (n = 2), the natural sciences (n = 1), everyday life (n = 1), earth and space science (n = 1), and mechanical engineering (n = 1). One study did not explicitly mention a specific context domain. Most studies (n = 6) reported that learners only generate hypotheses that seem plausible to them based on expected data to avoid choosing hypotheses with a high chance of being rejected (also known as fear of rejection; H6*; e.g., Echevarria, Citation2003). In such cases, preconceptions or beliefs have a strong influence on what kinds of hypotheses are formulated. When this phenomenon arises in a single experiment, it does not necessarily mean that the hypothesis is not testable or the outcome of the experiment is compromised, but it could affect whether learners seek out further evidence or only confirmatory evidence. The same is true of learners’ working without a hypothesis (H2*; e.g., Darus & Saat, Citation2014): learners can still experimentally investigate the relationship between at least two variables in such a case, but it could also indicate a lack of awareness that hypotheses or predictions are valuable and often part of the experimental process, as they give a reason to tie predictions back to theory or previous experimental results. Likewise, only selecting hypotheses predicting the independent variable will have an influence on the dependent variable and therefore not selecting hypotheses that predict no effect (H4*; Kanari & Millar, Citation2004), could still lead to a successful experimental outcome in any individual case. However, it might also indicate that one presumes an effect always exists or that a relationship just needs to be proven by an experiment, which could affect reasoning strategies further along in the experimental process. In addition, the following specific learner approaches could be identified as well: generating hypotheses that include more than one independent variable (H5*; e.g., Valanides et al., Citation2013) and learners not being aware that more than one hypothesis is possible (H7*; e.g., Klahr et al., Citation1993).
Table 2. Overview of empirical studies reporting about learners’ challenges generating hypotheses during experimentation and selected properties.
5.3 Planning and conducting an experiment
Overall, out of the 34 studies addressing challenges while planning and conducting an experiment, 16 studies originate from the current review, seven are part of De Jong and van Joolingen (Citation1998) literature review, ten stem from Zimmerman’s (Citation2007) literature review, and a single study was reported by both of them. At 34, most of the studies analysed in this review relate to challenges in the inquiry phase of planning and conducting an experiment, although only 12 unique challenges in this inquiry phase were identified (P1 – P12; see, and ). This is because the present literature intensively describes two challenges: learners not controlling variables (PC3; n = 17) and the engineering approach (PC7; n = 11). An analysis of the studies’ target groups shows that learners struggle with controlling variables from early elementary school (e.g., Chen & Klahr, Citation1999) up to the university level (e.g., Dasgupta et al., Citation2016) and into adulthood (e.g., Shute & Glaser, Citation1990). This challenge has been assessed with a range of different assessment types and seems to be a domain-general difficulty. The other well-documented challenge from early childhood on (third grade), through to secondary school (5th and 6th grade) and into university concerns the engineering approach (PC7), in which learners create an effect as an outcome of an experiment instead of investigating a presumed relationship between variables (e.g., Carey et al., Citation1989; García-Carmona et al., Citation2017; Schauble et al., Citation1995). In this sense, the engineering approach has the (implicit) goal of creating a desired outcome rather than investigating the origin of an observed phenomenon. Because the scientific and engineering approach have different objectives, they follow different processes: The scientific approach takes for example, previous experimental results into account when making subsequent predictions, or considers certain experimental variations as more informative in a particular situation than others, whilst the engineering approach follows the goal of a creativity-based engineering design process (e.g., Schauble et al., Citation1991b). This concept is discussed in eleven studies, mostly among school students (n = 5) but also at the university level (n = 3) or in comparisons of different education levels (n = 3).
Table 3. Overview of empirical studies reporting about learners’ challenges planning and conducting an experiment and selected properties.
Overall, the sample sizes ranged from 4 to 1006 participants, and the students participating in the studies ranged from second up to twelve graders. 21 studies took place among K-12 school students, seven studies involved university students. There were also five studies comparing school students’ ability to plan and conduct an experiment with that of adults and university students (Klahr et al., Citation1993; Kuhn et al., Citation1995; Schauble, Citation1996; Schrempp & Sodian, Citation1999; Tschirgi, Citation1980). A single study compared university students and adults, who were not enrolled at university (Shute & Glaser, Citation1990). Similarly to the previous inquiry phases, most studies identified challenges when learners plan and conduct experiments using paper-pencil tests (n = 17). Even though this inquiry phase has more procedural components than the others, only two studies used performance assessments in which learners were actually required to practically conduct an experiment (Klahr & Nigam, Citation2004; Siegler & Liebert, Citation1975). In a similar vein, one study focused on students’ cognitive and manual abilities in video-recorded laboratory sessions (Baur, Citation2018). It was found that students handle laboratory materials and substances indiscriminately, as students did not consider the comparability of substances or materials (e.g., use of test tubes with different volumes) across different conditions (PC8).
Altogether, participants were interviewed in 18 studies, while 12 studies used computer simulations. Laboratory reports from experiments were analysed in eleven studies, followed by audio recordings (n = 5), videos of laboratory sessions (n = 4), and observations of learners in experimental settings (n = 4). The context domains were mostly physics (n = 12), biology (n = 6), the natural sciences (n = 5), earth and space sciences (n = 5) and everyday life contexts (n = 4). The other context domains were chemistry (n = 1), logic (n = 2), mechanical engineering (n = 1) and the social sciences (n = 1).
5.4 Data analysis and drawing conclusions
Fourteen challenges with data analysis and drawing conclusions were reported by 31 studies (DC1 – DC14*; see, and ).16 of the 31 studies originate from the current review, seven from De Jong and van Joolingen (Citation1998) and eight from Zimmerman’s (Citation2007) literature review. Three of the 14 challenges in this category were considered to be specific learner approaches: The first such approach is that learners become uncertain in drawing conclusions when confronted with variability in the measured data, such as that resulting from large sample sizes in repeated measurements (DC2*; Masnick & Morris, Citation2008). The second learner approach identified and labelled DC9* is similar in nature. In sum, the findings categorised as DC9* show that anomalous data lead learners to assume that there are flaws in the experimental procedure. For example, in the study by Schauble (Citation1996), children and adults generated quantitative data by exploring tasks involving hydrodynamics and hydrostatics. Repeated experimental trials led to variation in the measured data. Because these were duplicate trials, learners were uncertain about what caused the differences in the data. In this case, students had to decide which differences were ‘real’ and which represented data variability. It could be shown that prior expectations about the experimental results strongly influenced learners’ interpretations. Different outcomes in duplicate trials were interpreted as indicating an effect if that effect was expected, but interpreted as measurement error if an effect was not expected (Schauble, Citation1996). On the one hand, this might be seen as a facet of confirmation bias (Wason, Citation1960); on the other hand, it could indicate that learners are willing to reflect on experimental procedures and therefore look for potential flaws, which is in line with the scientific principles underlying experimentation. The third specific approach, based on the expert ratings, is that learners do not connect their hypotheses to previous results of an experiment (DC14*). In this case, learners do not state hypotheses on the basis of data gathered and do not correct or adapt their hypotheses even if data contradicts it (Klahr & Dunbar, Citation1988). Overall, the sample sizes of studies reporting challenges with data analysis and drawing conclusions ranged from 4 to 1006 participants. Most studies took place at the school level (n = 19), but challenges with data analysis and drawing conclusions were also reported among university students (n = 6). Seven studies compared children to university students or adults regarding this inquiry phase and reported the challenges each group encountered (e.g., Aoki, Citation1991; Masnick & Morris, Citation2008). The students examined in these studies stemmed from second to eighth grade, with most in elementary school (n = 8). No studies presented challenges for children in higher grades. The assessment types used were mostly interviews (n = 16), followed by paper-pencil tests (n = 14), laboratory reports produced during experimentation (n = 10), computer simulations (n = 7), video recordings (n = 7), audio recordings of laboratory sessions (n = 3) and observations of experimental settings (n = 3). Not surprisingly, all of the studies investigated this inquiry phase with respect to specific context domains, namely physics (n = 10), followed by biology (n = 8) and logic (n = 4). The following context domains were examined less frequently: everyday life (n = 4), earth and space sciences (n = 2), the natural sciences (n = 2), chemistry (n = 1) and the social sciences (n = 1).
Table 4. Overview of empirical studies reporting about learners’ challenges analysing and drawing conclusions during experimentation and selected properties.
5.5 Multiple inquiry phases
19 studies in total described the eight challenges assigned to this category (see, and ). These varied in their degree of abstraction: from learners not being able to identify variables (M2), which is not only needed when generating hypotheses about potential causal relationships between variables, but is also important when planning and conducting an experiment; to learners not being aware of the concept of measurement error (M6). Two challenges in this category were labelled specific learner approaches, as they do not necessarily compromise the outcome of an experiment in all cases. The first of these is changing hypotheses during an ongoing experiment (M3*). Baur (Citation2018) notes that students change their hypotheses during the experimental process before obtaining a result. After changing their hypothesis, however, students do not throw out the previously established experimental setting, but expand it with additional structures. Changing hypotheses without obtaining a result that disconfirms or confirms one’s earlier hypothesis can lead learners to overlook causality. In addition, broadening the set-up of an experiment that has already begun can make experiments unsystematic. The second specific learner approach is not constructing tables (M7*), which may help learners illustrate and present the data they have obtained, but it is not always a prerequisite for scientific experimentation, because not every experiment requires data to be organised in tables.
Table 5. Overview of empirical studies reporting about learners’ challenges related to multiple inquiry phases and selected properties.
6. Discussion
In summary, the current literature review includes 66 studies, 13 of which were published between 1999 and 2007 and not included in the reviews by De Jong and van Joolingen (Citation1998) or Zimmerman (Citation2007). The current review also includes 20 studies that were not in the two previous reviews because they were published after 2007. By analysing all of these studies, this review provides an extended and up-to-date systematic overview of empirical research on 43 challenges encountered during the four inquiry phases of ‘stating a research question’, ‘generating hypotheses’, ‘planning and conducting experiments’, and ‘data analysis and drawing conclusions’. From our review, it is clear that an increasing number of published studies are investigating challenges related to planning and conducting an experiment (for example, the following studies: Klahr & Nigam, Citation2004; Tairab, Citation2015; White, Citation1993) as well as data analysis and drawing conclusions (for example, the following studies: Aoki, Citation1991; Klahr & Dunbar, Citation1988; Masnick & Morris, Citation2008). Research on learners’ challenges in stating research questions is quite young, as we only found the following studies by Cuccio-Schirripa & Steiner (Citation2000), Hofstein et al.,(Citation2005, Citation2004) and Neber & Anton (Citation2008a, Citation2008b). All studies were done on or after the turn of the millennium. In contrast, research on learners seeking out evidence confirming their hypotheses (confirmation bias) leads back to a study by Wason (Citation1960) sixty years ago. Overall, the findings reveal an intensive research focus on neglect of the control-of-variables strategy (for example, the following studies: Chen & Klahr, Citation1999; Dasgupta et al., Citation2016) and the engineering approach (for example, the following studies: García-Carmona et al., Citation2017; Schauble et al., Citation1991b), both of which are not only found among younger students at the elementary school level (e.g., Erdosne Toth et al., Citation2000), but remain challenging in adulthood (e.g., Dasgupta et al., Citation2016). This review’s findings demonstrate that empirical evidence on challenges during experimentation exists in different fields, including science education, psychology and education research, highlighting not only the importance of this topic across disciplines but also the interdisciplinary and domain-general nature of the identified challenges during experimentation.
Although numerous studies have identified a variety of challenges in learning to experiment, their analyses show that these difficulties share common origins. Their different facets and manifestations can be described in line with the three dimensions of knowledge: (1) the conceptual, (2) epistemic and (3) procedural domain (Duschl, Citation2008; Furtak et al., Citation2012; OECD, Citation2019). In the following, we describe the identified challenges along these different facets and manifestations of IBL and therefore condensed into the following key statements:
(1) In some cases, learners lack conceptual knowledge about the scientific concepts underlying the specific inquiry phases and activities of an experiment. Some types of challenges could be related to an inadequate concept about experiments and experimentation. In our opinion challenges in this vein include: ‘Not knowing what a hypothesis is’ (H1), ‘Not controlling variables’ (PC3), ‘Working without a control condition’ (PC5), ‘Confusing observation and interpretation of data’ (DC1) and ‘Learners make no exclusion inferences’ (DC12). (2) Learners also demonstrate a lack of epistemic knowledge related to how scientific knowledge is generated, meaning an understanding of the role of specific constructs and defining features essential to the process of building scientific knowledge. Challenges related to the epistemic domain reflect difficulties in understanding the rationale of experimentation and developing explanations for phenomena under the circumstances that scientific knowledge is subject to change in the face of new evidence or new interpretations of old evidence. Furthermore, the understanding of the function that questions, hypotheses, interpretation and conclusion play in science is affected. Based on this description, the following challenges load into the epistemic domain: ‘Lack of repetition of measurement’ (PC10), ‘No awareness of measurement error’ (M6) and ‘Not connecting hypotheses to previous results’ (DC14*). (3) Some of the challenges show that learners do not know how to conduct scientific investigations and therefore lack procedural skills. Through real-life handling of experimental objects such as equipment, chemicals or, in biology, living organisms, learners can gain practical experience. Challenges of this sort occur in hands-on performances and practically performing the experiment. Challenges revealing this kind of deficit are in our opinion: ‘Handling material and substances indiscriminately’ (PC8), ‘Planning and conducting the same trials several times (without the aim of repeated measurements)’, (PC11), ‘Do not involve all test trials while conducting their experiment’ (PC12). However, there is only limited evidence related to learners’ procedural challenges based on the relatively low occurrences of these kinds of challenges in the current review (PC8-PC12). Although, as just shown, challenges can be distinguished along the three IBL domains (conceptual, procedural, epistemic), scientific literacy requires all three forms of scientific knowledge and skills (OECD, Citation2018). This also suggests that not every challenge can always be clearly assigned to a single domain, as its characterisation encompasses difficulties in more than one domain. However, the central commonality of the identified challenges across the domains reflects their similarity, which can be helpful, for example, in aligning the goals of IBL and scaffolds in science education settings.
(4) In addition to these three domains there are challenges that stem from cognitive biases and preconceptions that develop early in students’ school careers (for example, the following studies: Carey et al., Citation1989; Valanides et al., Citation2013; Wu & Wu, Citation2011). Cognitive biases and preconceptions impact the conceptual, epistemic and procedural domains of an investigation. Therefore, often not only a single phase is affected, but rather multiple phases of inquiry (for example, as reported in the following studies: Dunbar & Klahr, Citation1989; Schauble et al., Citation1991a; Citation1993). Challenges demonstrating this include ‘Only generating hypotheses that are plausible and predict expected data (fear of rejection)’ (H6), ‘Producing desired experimental results’ (PC6), or ‘Ignoring anomalous data’ (DC8). Learners are guided by preconceptions and assumptions, not by evidence, in planning and conducting experiments and drawing conclusions. In this regard, Zimmerman (Citation2007) points out that learners’ assumptions and beliefs about the goals of experimentation influence how they learn in myriad ways: prior knowledge not only influences their understanding of the nature of science and what experimentation is on a meta level, but might also explain more specific approaches such as the tendency to focus on desirable effects and neglect undesirable ones.
In a broader context, those challenges indicate that there are many obstacles for learners on the way to engage not only in experiments, but with science-related issues that experiments can focus on. In this regard, experimentation relies not only on teaching content knowledge and facts, as these can hardly embrace the character of science (Arnold et al., Citation2014). In addition, an individual’s understanding of scientific concepts, phenomena and processes, and one’s ability to apply this knowledge to new and, at times, non-scientific situations can promote learners’ scientific literacy (OECD, Citation2019). By enabling learners to critically examine experiments and their limitations, science education can contribute to an understanding of the characteristics of science and the ability to think scientifically as a prerequisite for critical participation in the public debate. This is especially important at this place in time, where many science-related topics, such as anthropogenic climate crisis, require a certain degree of agreement or consensus in society about scientific evidence (Kranz et al, Citation2022; Sharon & Baram‐Tsabari, Citation2020). These complex public discourses are not necessarily a new phenomenon, but they have become especially demanding as science-related topics these days do not only influence everyday life decisions but will also impact personal living circumstances of individuals and entire societies in the near and far future (Lee & Brown, Citation2018). These topics often elicit persistent public controversy, not uncommonly because many people are misinformed about scientific facts or not able to distinguish between scientific and non-scientific information (Winter et al., Citation2022 Sharon & Baram‐Tsabari, Citation2020). Hence, to cope with these demands of the twenty-first century, learners should be able to acquire and then use their developed understanding of science, where experiments play a pivotal role, to contribute to public debate and form informed opinions on science- and technology-based questions in order to become more critical citizens (OECD, Citation2018).
6.1 Implications for educators and researchers
With regard to the challenges learners encounter when experimenting in IBL settings, a more challenge-oriented approach involving scaffolding measures (e.g., Hmelo-Silver et al., Citation2007; Sawyer, Citation2006) has been proven to be effective for overcoming these hurdles (Furtak et al., Citation2012). The characteristic feature of scaffolding measures is that they enable learners to successfully complete tasks that otherwise – without appropriate assistance – would not be manageable for them (Hmelo-Silver et al., Citation2007). The results of this review can be used to design specific scaffolds that address the challenges learners face. In that sense, formative assessments that point directly to students’ challenges could also be helpful for fostering learners’ skills and knowledge in experimentation or inquiry-based learning. Addressing the presented challenges in everyday teaching – giving more explanations or offering special instruction on challenges or specific learner’s approaches – could be also very beneficial. For example, if an IBL unit is being implemented to teach concrete scientific concepts (e.g., control-of-variables strategy, Chen & Klahr, Citation1999) it is advisable to use fully pre-structured tasks at the beginning: providing a pre-specified research question or guidelines for planning and documenting an investigation. This reduces requirements in the procedural and epistemic domains, allowing learners to focus on the subject content. This can ensure that the targeted scientific concept is actually addressed (e.g., Abrams et al., Citation2008; Blanchard et al., Citation2010; Vorholzer & von Aufschnaiter, Citation2019). In contrast, tasks that give learners greater responsibility are suitable when focusing on the application of procedural and epistemic competencies (e.g., Abels, Citation2015; Fang et al., Citation2016). Thus, IBL can take on numerous forms along a broad spectrum depending on the instructional objectives and learners’ competence. However, if learners are not given opportunities to experience inquiry activities as comprehensive procedures, they will not be able to fully comprehend scientific methods nor fully appreciate the nature of scientific knowledge itself (NGSS Lead States, Citation2013). Likewise, our analysis shows that it is not enough to only consider research results on learners’ conceptual and epistemic abilities if the goal is to identify learners’ understanding of and ability to perform experiments. Thus, more research is needed on learners’ procedural knowledge and skills (e.g., handling laboratory materials, chemicals, etc.) and associated challenges when actually conducting experiments in practice. In that sense, practical performance assessments, which are already used to some extent in international school assessment (e.g., Harmon et al., Citation1997), could provide insights into students’ development of manual skills and abilities. However, 64 out of 66 studies examined in this review used observations, video analyses or other non-practical oriented formats. These types of assessments (non-performance tests) might simplify or omit certain inquiry activities (Ludwig et al., Citation2018; Schecker & Parchmann, Citation2021; Shavelson et al., Citation1999), providing an incomplete picture of experimentation.
In sum, the results reveal an imbalance in the number of studies reporting on learners’ challenges during the different inquiry phases. Many more studies examine challenges learners face when generating hypotheses, planning and conducting an experiment or analysing data and drawing conclusions (n = 61) compared to stating research questions. Until 2008, only five studies had investigated children’s ability to state research questions. This could lead to two conclusions: on the one hand, this finding could illustrate that formulating research questions is not such a demanding research phase as to pose major challenges to learners, or on the other hand, there could be a lack of in-depth research on learners’ understanding of and ability to actually state research questions despite its importance in the inquiry process (Cuccio-Schirripa & Steiner, Citation2000). The latter would also align with the fact that research questions in IBL settings are often predefined in order to not overburden learners with a too-open research setting (Blanchard et al., Citation2010) and to ensure that different learners make comparable progress in the inquiry process. However, gaining more insight into how learners can successfully generate an experimentally verifiable research question – instead of leaving it as a blind spot that is largely set by default – should be a focus of future research.
6.2 Limitations
Literature reviews are generally limited by the spectrum of literature they examine based on their database search and subsequent selection procedure. For this literature review, we decided to limit the search to peer-reviewed articles in research journals indexed in any of the four databases used (ERIC, FIS, Web of Science and Google Scholar). We are aware that this decision may have limited the available literature (i.e., if articles are not indexed in the respective databases) and forced us to exclude certain publications (e.g., doctoral dissertations, research reports) that may have provided valuable insights into learners’ challenges when experimenting. However, by searching in four databases that index some of the most visible journals, we aimed to find the majority of important contributions to this research field. Moreover, given the relatively large number of articles included in the current review, a review of all inquiry-related publications might not have been feasible. Thus, we elected to only incorporate peer-reviewed articles to ensure that the reviewed contributions attained a certain level of quality by relying on the journals’ policy to ensure a rigorous peer review process.
Another limiting aspect of our review is the decision to limit the literature search to contributions published in the last 24 years (after 1998). To reduce the possibility of missing relevant contributions published before 1998, we consulted two prior literature reviews with a similar objective (De Jong & van Joolingen, Citation1998; Zimmerman, Citation2007), which consider relevant research dating back to before 1998. Thus, these two reviews seemed like an appropriate foundation for the current review’s literature search.
6.3 Conclusion
Overall, the results confirm that the experimental method in general and within IBL in particular represents a complex task for learners. In that sense, the effectiveness of IBL has been questioned in the past (Kirschner et al., Citation2006; Klahr & Nigam, Citation2004). Along these lines, recent reviews and meta-analyses of intervention studies contrasting IBL and more explicit or guided teaching methods have found that IBL has no overall positive benefit on student achievement. In fact, the degree of guidance (or openness; Author, Citation2020; Bell et al., Citation2005) does influence learning (Alfieri et al., Citation2011; Lazonder & Harmsen, Citation2016; Minner et al., Citation2010): Whilst unguided inquiry learning settings are unlikely to be effective because they neglect for example, the limitations of working memory and executive control (Kirschner et al., Citation2006), there is also considerable evidence that IBL can indeed improve cognitive achievement, critical thinking and attitudes towards science (e.g., Anderson, Citation2002; Haury, Citation1993; Minner et al., Citation2010; Osborne & Dillon, Citation2008; Schroeder et al., Citation2007) and improve learners’ ability to understand and perform experiments (e.g., Blanchard et al., Citation2010; Carey et al., Citation1989; Chen & Klahr, Citation1999; Furtak et al., Citation2012; Hofstein et al., Citation2005; Kuhn & Dean, Citation2005; Schwichow et al., Citation2016). In this regard, stringently designed and activity-centred learning environments have been proved as particularly effective (Blanchard et al., Citation2010; Bybee et al., Citation2006; Minner et al., Citation2010). According to Kirschner et al. (Citation2006) guidance like this can lower the high demands on working memory and executive control that are required and thus enable learners to encode and store novel information in long-term memory. In light of this debate, the challenges identified in this review could serve as groundwork for the development of scaffolds to support learners’ transition from closed to more open-ended IBL approaches. In a next step, these tailored learning approaches can be empirically investigated, for example, in the form of experimental intervention studies. In our view exploring both – learners’ abilities and challenges – is critical not only for developing IBL interventions to better inform research approaches in science education, but also for their application and implementation by practitioners in science classrooms. In sum, learners’ challenges during the inquiry phases of an experiment identified and analysed in the current review can be viewed as springboards for further learning progress to contribute to critical citizens in a scientific laden world.Citation2018
Acknowledgments
We would like to acknowledge the following experts who contributed to developing the framework for assigning challenges to specific inquiry phases and identifying their relations to the inquiry-based learning process through their ratings, comments and discussions: Till Bruckermann, Markus Emden, Katharina Groß, Marcus Hammann, Kerstin Kremer, Anja Lembens, Antti Lehtinen, Jürgen Mayer, Susanne Metzger, Pasi Nieminen, Marios Papaevripidou, Markus Rehm, Iris Schiffl, Martin Schwichow, Andreas Vorholzer, Nikoletta Xenofontos, Zacharias Zacharia. We also thank Marko Lüftenegger for his valuable feedback on earlier versions of this manuscript.
Disclosure statement
No potential conflict of interest was reported by the author(s).
Additional information
Notes on contributors
Johanna Kranz
Johanna Kranz is a Post-Doc-researcher at the Rhineland-Palatinate Competence Center for Climate Change Impacts at the Forest Ecology and Forestry Research Institute (Germany). Her main research interests are inquiry-based learning with a focus on experimentation and the control-of-variables strategy. She also engages in research on climate change education.
Armin Baur
Armin Baur is Professor for Biology and Biology Education at the University of Education in Heidelberg (Germany). His main research interests are inquiry-based learning, experimentation and teachers’ professional development.
Andrea Möller
Andrea Möller heads the Austrian Educational Competence Centre for Biology (AECC Biology) and is professor for Biology and Biology Education at the University of Vienna (Austria). Her main research interests are inquiry-based learning with a focus on experimentation. She also engages in research on environmental education and climate change education.
Notes
1. While other articles use terms such as problems, difficulties or errors (e. g., De Jong & van Joolingen, Citation1998; Zimmerman, Citation2007) to describe aspects that comprise the experimentation process, we follow Glazer (Citation2011) and use the term ‘challenge’ to emphasise the potential that arises from working through a challenging issue, rather than simply pointing out deficits and errors (Steuer et al., Citation2013; Tulis et al., Citation2016).
References
- Abd-El-Khalick, F., BouJaoude, S., Duschl, R., Lederman, N. G., Mamlok-Naaman, R., Hofstein, A., Niaz, M., Treagust, D., & Tuan, H.-L. (2004). Inquiry in science education: International perspectives. Science Education, 88(3), 397–419. https://doi.org/10.1002/sce.10118
- Abels, S. (2015). Scaffolding inquiry-based science and chemistry education in inclusive classrooms. In N. L. Yates (Ed.), New developments in science education research (pp. 77–96). Nova Science Publishers.
- Abrams, E., Southerland, S. A., & Evans, C. A. (2008). Introduction: Inquiry in the Classroom: Identifying necessary components of a useful definition. In E. Abrams, S. A. Southerland, & P. C. Silva (Eds.), Inquiry in the Classroom: Realities and opportunities (pp. xi–xlii). Information Age Publishing, Inc.
- Adams, J. D., Avraamidou, L., Bayram-Jacobs, D., Boujaoude, S., Bryan, L., Christodoulou, A., & Zembal-Saul, C. (2018). The role of science education in a changing world. Technical Report. Lorentz Centre.
- Alfieri, L., Brooks, P. J., Aldrich, N. J., & Tenenbaum, H. R. (2011). Does discovery-based instruction enhance learning? Journal of Educational Psychology, 103(1), 1–18. https://doi.org/10.1037/a0021017
- Amsel, E., & Brock, S. (1996). The development of evidence evaluation skills. Cognitive Development, 11, 523–550. https://doi.org/10.1016/S0885-2014(96)90016-7
- Anderson, R. D. (2002). Reforming science teaching: What research says about inquiry. Journal of Science Teacher Education, 13(1), 1–12 doi:https://doi.org/10.1023/A:1015171124982.
- Aoki, T. (1991). The relation between two kinds of U-shaped growth curves: Balance-scale and weight-addition tasks. The Journal of General Psychology, 118, 251–261. https://doi.org/10.1080/00221309.1991.9917784
- Arnold, J., Boone, W., Kremer, K., & Mayer, J. (2018). Assessment of competencies in scientific inquiry through the application of rasch measurement techniques. Education Sciences, 8(4). https://doi.org/10.3390/educsci8040184
- Arnold, J., Kremer, K., & Mayer, J. (2014). Understanding students’ experiments—What kind of support do they need in inquiry tasks? International Journal of Science Education, 36(16), 2719–2749. https://doi.org/10.1080/09500693.2014.930209
- Baur, A. (2015). Inwieweit eignen sich bisherige Diagnoseverfahren des Bereichs Experimentieren für die Schulpraxis? [To what extent are previous diagnostic procedures in the field of experimentation suitable for school practice?. Zeitschrift für Didaktik der Biologie - Biologie Lehren und Lernen, 19, 1.
- Baur, A. (2018). Fehler, Fehlkonzepte und spezifische Vorgehensweisen von Schülerinnen und Schülern beim Experimentieren [Students' errors, misconceptions and specific approaches during experimentation. 24, 115–129. https://doi.org/10.1007/s40573-018-0078-7
- Baur, A. (2021). Errors made by 5th-, 6th-, and 9th-graders when planning and performing experiments: Results of video-based comparisons. Zeitschrift für Didaktik Der Biologie (ZDB) - Biologie Lehren Und Lernen, 25, 45–63. https://doi.org/10.11576/zdb-3576
- Baur, A. (2021). Errors made by 5th-, 6th-, and 9th-graders when planning and performing experiments: Results of video-based comparisons. Zeitschrift für Didaktik Der Biologie (ZDB) - Biologie Lehren Und Lernen, 25, 45–63. https://archiv.ipn.uni-kiel.de/zfdn/pdf/003_12.pdf
- Baur, A., & Emden, M. (2020). How to open inquiry teaching? An alternative teaching scaffold to foster students’ inquiry skills. Chemistry Teacher International, 3, 1. https://doi.org/10.1515/cti-2019-0013
- Bell, R. L., Smetana, L., & Binns, I. (2005). Simplifying inquiry instruction. Science Teacher, 72(7), 30–33.
- Blanchard, M. R., Southerland, S. A., Osborne, J. W., Sampson, V. D., Annetta, L. A., & Granger, E. M. (2010). Is inquiry possible in light of accountability? A quantitative comparison of the relative effectiveness of guided inquiry and verification laboratory instruction. Science Education, 94(4), 577–616. https://doi.org/10.1002/sce.20390
- Boaventura, D., Faria, C., Chagas, I., & Galvão, C. (2013). Promoting science outdoor activities for elementary school children: Contributions from a research laboratory. International Journal of Science Education, 35(5), 796–814. https://doi.org/10.1080/09500693.2011.583292
- Bruckermann, T., Arnold, J., Kremer, K., & Schlüter, K. (2017). Forschendes Lernen in der Biologie. In T. Bruckermann & K. Schlüter (Eds.), Forschendes Lernen im Experimentalpraktikum Biologie [Inquiry-based learning in experimental biology]. Berlin, Heidelberg: Springer Spektrum. doi:10.1007/978-3-662-53308-6_2
- Bybee, R. W. (1997). Toward an understanding of scientific literacy. In W. Gräber & C. Bolte (Eds.), Scientific literacy. An international Symposium (pp. 37–68). IPN.
- Bybee, R. W. (2006). Teaching science as inquiry. In J. Minstrell & E. H. van Zee (Eds.), Inquiring into inquiry learning and teaching in science (pp. 21–46). American Association for the Advancement of Science.
- Bybee, R. W., Taylor, J. A., Gardner, A., van Scotter, P., Powell, J. C., Westbrook, A., & Landes, N. (2006). The BSCS 5E instructional model: Origins and effectiveness. BSCS. http://bscs.org/sites/default/files/_media/about/downloads/BSCS_5E_Full_Report.pdf
- Carey, S., Evans, R., Honda, M., Jay, E., & Unger, C. (1989). An experiment is when you try it and see if it works’: A study of grade 7 students’ understanding of the construction of scientific knowledge. International Journal of Science Education, 11, 514–529. https://doi.org/10.1080/0950069890110504
- Chang, H. P., Chen, C. C., Guo, G. J., Cheng, Y.-J., Lin, C., & Jen, T. (2011). The development of a competence scale for learning science: Inquiry and communication. International Journal of Science and Mathematics Education, 9, 1213–1233. http://dx.doi.org/10.1007/s10763-010-9256-x
- Chen, Z., & Klahr, D. (1999). All other things being equal: Acquisition and transfer of control of variables strategy. Child Development, 70(5), 1098–1120. https://doi.org/10.1111/1467-8624.00081
- Chin, C., & Osborne, J. (2008). Students’ questions: A potential resource for teaching and learning science. Studies in Science Education, 44(1), 1–39. https://doi.org/10.1080/03057260701828101
- Croker, S., & Buchanan, H. (2011). Scientific reasoning in a real-world context: The effect of prior belief and outcome on children’s hypothesis-testing strategies. The British Journal of Developmental Psychology, 29(3), 409–424. https://doi.org/10.1348/026151010X496906
- Croner, P. (2003). Developing critical thinking skills through the use of guided laboratory activities. The Science Education Review, 2(2), 1–13. https://files.eric.ed.gov/fulltext/EJ1058493.pdf
- Cuccio-Schirripa, S., & Steiner, H. E. (2000). Enhancement and analysis of science question level for middle school students. Journal of Research in Science Teaching, 37(2), 210–224. http://dx.doi.org/10.1002/(SICI)1098-2736(200002)37:2%3C210::AID-TEA7%3E3.0.CO;2-I
- Darus, F. B., & Saat, R. M. (2014). How Do Primary School Students Acquire the Skill of Making Hypothesis? The Malaysian Online Journal of Educational Science, 2(2), 20–26. https://files.eric.ed.gov/fulltext/EJ1086198.pdf
- Dasgupta, A. P., Anderson, T. R., & Pelaez, N. J. (2016). Development of the neuron assessment for measuring biology students’ use of experimental design concepts and representations. CBE Life Science Education, 15(2), 1–21. https://doi.org/10.1187/cbe.15-03-0077
- de Jong, T., & van Joolingen, W. R. (1998). Scientific discovery learning with computer simulations of conceptual domains. Review of Educational Research, 68(2), 179–201. https://doi.org/10.3102/00346543068002179
- DfES & QCA/Department for Education and Skills/Qualification and Curriculum Authority. (2004). Science—the national curriculum for England. HMSO.
- Duggan, S., & Gott, R. (2000). Intermediate general national vocational qualification (GNVQ) Science: A missed opportunity for a focus on procedural understanding? Research in Science & Technological Education, 18(2), 201–214. https://doi.org/10.1080/713694978
- Dunbar, K. (1993). Concept discovery in a scientific domain. Cognitive Science, 17, 397–434. https://doi.org/10.1207/s15516709cog1703_3
- Dunbar, K., & Klahr, D. (1989). Developmental differences in scientific discovery processes. In D. Klahr (Ed.), Complex information processing. The impact of Herbert A. Simon (pp. 109–143). Hillsdale, NJ: Erlbaum.
- Durmaz, H., & Mutlu, S. (2017). The effect of an instructional intervention on elementary students’ science process skills. The Journal of Educational Research, 110(4), 433–445. https://doi.org/10.1080/00220671.2015.1118003
- Duschl, R. (2008). Science education in three-part harmony: Balancing conceptual, epistemic, and social learning goals. Review of Research in Education, 32(1), 268–291. https://doi.org/10.3102/0091732X07309371
- Eastwell, P. (2014). Understanding hypotheses, predictions, laws and theories. Science Education Review, 13(1), 16–21. https://files.eric.ed.gov/fulltext/EJ1057150.pdf
- Echevarria, M. (2003). Anomalies as a catalyst for middle school students’ knowledge construction and scientific reasoning during science inquiry. Journal of Educational Psychology, 95(2), 357–374. https://doi.org/10.1037/0022-0663.95.2.357
- Erdosne Toth, E., Klahr, D., & Chen, Z. (2000). Bridging research and practice: A cognitively based classroom intervention for teaching experimentation skills to elementary school children. Cognition and Instruction, 18(4), 423–459. http://dx.doi.org/10.1207/S1532690XCI1804_1
- Fang, S.-C., Hsu, Y.-S., Chang, H.-Y., Chang, W.-H., Wu, H.-K., & Chen, C.-M. (2016). Investigating the effects of structured and guided inquiry on students’ development of conceptual knowledge and inquiry abilities: A case study in Taiwan. International Journal of Science Education, 38(12), 1945–1971. https://doi.org/10.1080/09500693.2016.1220688
- Furtak, E. M., Seidel, T., Iverson, H., & Briggs, D. C. (2012). Experimental and quasi-experimental studies of inquiry-based science teaching. Review of Educational Research, 82(3), 300–329. https://doi.org/10.3102/0034654312457206
- García-Carmona, A., Criado, A. M., & Cruz-Guzmán, M. (2017). Primary pre-service teachers’ skills in planning a guided scientific inquiry. Research in Science Education, 47(5), 989–1010. https://doi.org/10.1007/s11165-016-9536-8
- Garcia-Mila, M., & Andersen, C. (2007). Developmental change in notetaking during scientific inquiry. International Journal of Science Education, 29(8), 1035–1058. https://doi.org/10.1080/09500690600931103
- Garcia-Mila, M., Andersen, C., & Rojo, N. E. (2011). Elementary students’ laboratory record keeping during scientific inquiry. International Journal of Science Education, 33(7), 915–942. https://doi.org/10.1080/09500693.2010.48098
- Germann, P. J., Aram, R., & Burke, G. (1996). Identifying patterns and relationships among the responses of seventh-grade students to the science process skill of designing experiments. Journal of Research in Science Teaching, 33(1), 79–99. https://doi.org/10.1002/(SICI)1098-2736(199601)33:179::AID-TEA53.0.CO;2-M
- Gijlers, H., & de Jong, T. (2005). The relation between prior knowledge and students’ collaborative discovery learning processes. Journal of Research in Science Teaching, 42(3), 264–282. https://doi.org/10.1002/tea.20056
- Glazer, N. (2011). Challenges with graph interpretation: A review of the literature. Studies in Science Education, 47(2), 183–210. https://doi.org/10.1080/03057267.2011.605307
- Gott, R., & Duggan, S. (1995). Investigative work in the science curriculum. Open University Press.
- Gott, R., Duggan, S., & Roberts, R. (2008). Concepts of evidence and their role in open-ended practical investigations and scientific literacy. https://community.dur.ac.uk/rosalyn.roberts/Evidence/Gott%20&%20Roberts%20(2008)%20Research%20Report.pdf
- Greenhoot, A. F., Semb, G., Colombo, J., & Schreiber, T. (2004). Prior beliefs and methodological concepts in scientific reasoning. Applied Cognitive Psychology, 18(2), 203–221. https://doi.org/10.1002/acp.959
- Hammann, M., Phan, T. T. H., Ehmer, M., & Grimm, T. (2010). Assessing pupils’ skills in experimentation. Journal of Biological Education, 42(2), 66–72. https://doi.org/10.1080/00219266.2008.9656113
- Harmon, M., Smith, T. A., Martin, M. O., Kelly, D. L., Beaton, A. E., Mullis, I. V. S., Gonzalez, E. J., & Orpwood, G. (1997). Performance assessment in IEA’s third international mathematics and science study (TIMMS). Center for the Study of Testing, Evaluation, and Educational Policy, Boston College. https://timss.bc.edu/timss1995i/TIMSSPDF/PAreport.pdf
- Haury, D. L., ERIC, The Educational Resources Inforamtion Center. (1993). Teaching science through inquiry. ERIC/CSMEE Digest. http://files.eric.ed.gov/fulltext/ED359048.pdf
- Hmelo-Silver, C. E., Duncan, R. G., & Chinn, C. A. (2007). Scaffolding and achievement in problem-based and inquiry learning: A response to Kirschner, Sweller, and Clark. Educational Psychologist, 42(2), 99–107. https://doi.org/10.1080/00461520701263368
- Hofstein, A., Navon, O., Kipnis, M., & Mamlok-Naaman, R. (2005). Developing students’ ability to ask more and better questions resulting from inquiry-type chemistry laboratories. Journal of Research in Science Teaching, 42(7), 791–806. https://doi.org/10.1002/tea.20072
- Hofstein, A., Shore, R., & Kipnis, M. (2004). Providing high school chemistry students with opportunities to develop learning skills in an inquiry-type laboratory: A case study. International Journal of Science Education, 26(1), 47–62. https://doi.org/10.1080/0950069032000070342
- Kanari, Z., & Millar, R. (2004). Reasoning from data: How students collect and interpret data in science investigations. Journal of Research in Science Teaching, 41, 748–769. https://doi.org/10.1002/tea.20020
- Keselman, A. (2003). Supporting inquiry learning by promoting normative understanding of multivariable causality. Journal of Research in Science Teaching, 40(9), 898–921. https://doi.org/10.1002/tea.10115
- Kirschner, P. A., Sweller, J., & Clark, R. E. (2006). Why minimal guidance during instruction does not work: An analysis of the failure of constructivist, discovery, problem-based, experiential, and inquiry-based teaching. Educational Psychologist, 41(2), 75–86. https://doi.org/10.1207/s15326985ep4102_1
- Klahr, D., & Dunbar, K. (1988). Dual space search during scientific reasoning. Cognitive Science, 12(1), 1–48. https://doi.org/10.1207/s15516709cog1201_1
- Klahr, D., Fay, A., & Dunbar, K. (1993). Heuristics for scientific experimentation: A developmental study. Cognitive Psychology, 25, 111–146. https://doi.org/10.1006/cogp.1993.1003
- Klahr, D., & Nigam, M. (2004). The equivalence of learning. Path in early science instruction. Psychological Science, 15(10), 661–667. https://doi.org/10.1002/tea.20152
- Klahr, D., Triona, L. M., & Williams, C. (2007). Hands on what? The relative effectiveness of physical vs. virtual materials in an engineering design project by middle school students. Journal of Research in Science Teaching, 44, 183–203. https://doi.org/10.1002/tea.20152
- Klayman, J., & Ha, Y.-W. (1989). Hypothesis testing in rule discovery: Strategy, structure, and content. Journal of Experimental Psychology. Learning, Memory, and Cognition, 15(4), 596–604. https://doi.org/10.1037/0278-7393.15.4.596
- KMK/Sekretariat der Ständigen Konferenz der Kultusminister der Länder in der Bundesrepublik Deutschland. (2005a). Beschlüsse der Kultusministerkonferenz—Bildungsstandards im Fach Biologie für den Mittleren Schulabschluss [decisions of the assembly of German Ministers of Education—Educational standards in biology for lower secondary school]. Luchterhand.
- KMK/Sekretariat der Ständigen Konferenz der Kultusminister der Länder in der Bundesrepublik Deutschland. (2005b). Beschlüsse der Kultusministerkonferenz—Bildungsstandards im Fach Chemie für den Mittleren Schulabschluss [decisions of the assembly of German Ministers of Education—Educational standards in chemistry for lower secondary school]. Luchterhand.
- KMK/Sekretariat der Ständigen Konferenz der Kultusminister der Länder in der Bundesrepublik Deutschland. (2005c). Beschlüsse der Kultusministerkonferenz—Bildungsstandards im Fach Physik für den Mittleren Schulabschluss [decisions of the assembly of German Ministers of Education—Educational standards in physics for lower secondary school]. Luchterhand.
- Kranz, J., Schwichow, M., Breitenmoser, P., & Niebert, K. (2022). The (Un)political Perspective on Climate Change in Education—A Systematic Review. Sustainability. https://doi.org/10.3390/su14074194
- Kremer, K., Möller, A., Arnold, J., & Mayer, J. (2019). Kompetenzförderung beim Experimentieren [Promoting experimental competence]. In J. Groß, M. Hammann, P. Schmiemann, & J. Zabel (Eds.), Biologiedidaktische Forschung: Erträge für die Praxis (pp. 113–128). Springer Spektrum. https://doi.org/10.1007/978-3-662-58443-9_7.
- Kremer, K., Specht, C., Urhahne, D., & Mayer, J. (2013). The relationship in biology between the nature of science and scientific inquiry. Journal of Biological Education, 48(1), 1–8. https://doi.org/10.1080/00219266.2013.788541
- Kuhn, D. (2007). Reasoning about multiple variables: Control of variables is not the only challenge. Science Education, 91(5), 710–726. https://doi.org/10.1111/j.1467-9280.2005.01628
- Kuhn, D., & Dean, D. Jr. (2005). Is developing scientific thinking all about learning to control variables? Psychological Science, 16, 866–870. https://doi.org/10.1111/j.1467-9280.2005.01628
- Kuhn, D., Garcia-Mila, M., Zohar, A., Andersen, A., White, C., Sheldon, H., Klahr, D., Carver, D., & Sharon, M. (1995). Strategies of knowledge acquisition. Monographs of the Society for Research in Child Development, 60, 1–128. https://doi.org/10.2307/1166059
- Kuhn, D., Schauble, L., & Garcia-Mila, M. (1992). Cross-domain development of scientific reasoning. Cognition and Instruction, 9, 285–327. https://doi.org/10.1207/s1532690xci0904_1
- Kwon, Y.-J., Jeong, J.-S., & Park, Y.-B. (2006). Roles of abductive reasoning and prior belief in children’s generation of hypotheses about pendulum motion. Science & Education, 15(6), 643–656. https://doi.org/10.1007/s11191-004-6407-x
- Lazonder, A. W., & Harmsen, R. (2016). Meta-analysis of inquiry-based learning: Effects of guidance. Review of Educational Research, 86(3), 681–718. https://doi.org/10.3102/0034654315627366
- Lee, E. A., & Brown, M. J. (2018). Connecting inquiry and values in science education. Science & Education, 27(1–2), 63–79. https://doi.org/10.1007/s11191-017-9952-9
- Littell, J. H., Corcoran, J., & Pillai, V. (2008). Systematic reviews and meta-analysis. Oxford University Press.
- Ludwig, T., Priemer, B., & Lewalter, D. (2018). Decision-making in uncertainty-infused learning situations with experiments in physics classes. Looking back, looking forward. In M. A. Sorto, A. White, & L. Guyot (Eds.), Proceedings of the Tenth International Conference on Teaching Statistics, Kyoto, Japa, Voorburg, The Netherlands: International Statistical Institute.
- Masnick, A. M., & Klahr, D. (2003). Error matters: An initial exploration of elementary school children’s understanding of experimental error. Journal of Cognition and Development, 4(1), 67–98. https://doi.org/10.1207/S15327647JCD4,1-03
- Masnick, A. M., & Morris, B. J. (2008). Investigating the development of data evaluation: The role of data characteristics. Child Development, 79(4), 1032–1048. https://doi.org/10.1207/S15327647JCD4,1-03
- Mayer, J. (2007). Erkenntnisgewinnung als wissenschaftliches Problemlösen [Inquiry as scientific problem-solving]. In D. Krüger & H. Vogt (Eds.), Theorien in der biologiedidaktischen Forschung (pp. 177–186). Springer. https://doi.org/10.1007/978-3-540-68166-3_16.
- Mayer, J., Grube, C., & Möller, A. (2008). Kompetenzmodell naturwissenschaftlicher Erkenntnisgewinnung [Competence model of scientific inquiry]. In U. Harms & A. Sandmann (Eds.), Lehr- und Lernforschung in der Biologiedidaktik: Ausbildung und Professionalisierung von Lehrkräften (Vol. 3, pp. 63–79). StudienVerlag.
- Mayer, J., & Ziemek, H.-P. (2006). Offenes experimentieren. Forschendes Lernen im Biologieunterricht [Open experimentation. Inquiry-based learning in biology classes]. Unterricht Biologie, 317, 4–12.
- Meier, M., & Mayer, J. (2012). Experimentierkompetenz praktisch erfassen - Entwicklung und Validierung eines anwendungsbezogenen Aufgabendesigns [Practical assessment of experimental competence - development and validation of an application-oriented task design]. In U. Harms & F. X. Bogner (Eds.), Lehr- und Lernforschung in der Biologiedidaktik (Vol. 5, pp. 81–98). StudienVerlag.
- Millar, R., & Lubben, F. (1996). Investigative work in science. The role of prior expectations and evidence in shaping conclusions. Educational Research, 13(3), 28–34. https://doi.org/10.1080/03004279685200061
- Minner, D. D., Levy, A. J., & Century, J. (2010). Inquiry-based science instruction -what is it and does it matter? Results from a research synthesis years 1984 to 2002. Journal of Research in Science Teaching, 47(4), 474–496. https://doi.org/10.1002/tea.20347
- Mokros, J. R., & Tinker, R. F. (1987). The impact of microcomputer based labs on children’s ability to interpret graphs. Journal of Research in Science Teaching, 24, 369–383. https://doi.org/10.1002/tea.3660240408
- Neber, H., & Anton, M. A. (2008a). Förderung präexperimenteller epistemischer Aktivitäten im Chemieunterricht. [Promoting pre-experimental epistemic activities in chemistry education. Zeitschrift für Pädagogische Psychologie, 22(2), 143–150. https://doi.org/10.1024/1010-0652.22.2.143
- Neber, H., & Anton, M. A. (2008b). Promoting Pre-experimental activities in high‐school chemistry: Focusing on the role of students’ epistemic questions. International Journal of Science Education, 30(13), 1801–1821. https://doi.org/10.1080/09500690701579546
- NGSS Lead States. (2013). Next generation science standards: For states, by states. National Academies Press.
- Njoo, M., & de Jong, T. (1993). Exploratory learning with a computer simulation for control theory: Learning processes and instructional support. Journal of Research in Science Teaching, 30, 821–844. https://doi.org/10.1002/tea.3660300803
- NRC. (1996) . National science education standards. The National Academies Press.
- NRC. (2000) . Inquiry and the national science education standards. The National Academy Press.
- NRC. (2012) . A framework for K-12 science education: Practices, crosscutting concepts, and core ideas. The National Academies Press.
- NRC. (2013). Next generation science standards: For states, by states. The National Academies Press. https://www.nextgenscience.org/get-to-know
- OECD. (2018). The science of teaching science: An exploration of science teaching practices in PISA 2015. Paris: OECD Publishing.
- OECD. (2019). PISA 2018. Assessment and analytical framework. https://doi.org/10.1787/b25efab8-en
- Osborne, J. (2014). Teaching scientific practices: Meeting the challenge of change. Journal of Science Teacher Education, 25, 177–196. https://doi.org/10.1007/s10972-014-9384-1
- Osborne, J., Collins, S., Ratcliffe, M., Millar, R., & Duschl, R. (2003). What ‚ideas-about-science’ should be taught in school science? A delphi study of the expert community. Journal of Research in Science Teaching, 40(7), 692–720. https://doi.org/10.1002/tea.10105
- Osborne, J., & Dillon, J. (2008). Science education in Europe: Critical reflections. The Nuffield Foundation.
- Page, M. J., Moher, D., Bossuyt, P. M., Boutron, I., Hoffmann, T. C., Mulrow, C. D., Shamseer, L., Tetzlaff, J. M., Akl, E. A., Brennan, S. E., Chou, R., Glanville, J., Grimshaw, J. M., Hróbjartsson, A., Lalu, M. M., Li, T., Loder, E. W., Mayo-Wilson, E., McDonald, S., & McKenzie, J. E. (2021). PRISMA 2020 explanation and elaboration: Updated guidance and exemplars for reporting systematic reviews. BMJ, 372(n160). https://doi.org/10.1136/bmj.n160
- Park, J. (2006). Modelling analysis of students’ processes of generating scientific explanatory hypotheses. International Journal of Science Education, 28(5), 469–489. https://doi.org/10.1080/09500690500404540
- Pedaste, Margus, Mäeots, Mario, Leijen, Ääli, Sarapuu, Tago 2012 Improving Students’ Inquiry Skills through Reflection and Self-Regulation Scaffolds Tech., Inst., Cognition and Learning 9 81–95
- Pedaste, M., Mäeots, M., Leijen, Ä., & Sarapuu, T. (2012). Improving students’ inquiry skills through reflection and self-regulation scaffolds. Tech. Inst. Cognition and Learning, 9, 81–95. https://www.oldcitypublishing.com/journals/ticl-home/ticl-issue-contents/ticl-volume-9-number-1-2-2011/ticl-9-1-2-p-81-95/
- Pedaste, M., Mäeots, M., Siiman, L. A., de Jong, T., van Riesen, S. A. N., Kamp, E. T., Manoli, C. C., Zacharia, Z. C., & Tsourlidaki, E. (2015). Phases of inquiry-based learning: Definitions and the inquiry cycle. Educational Research Review, 14, 47–61. https://doi.org/10.1016/j.edurev.2015.02.003
- Penner, D. E., & Klahr, D. (1996a). The Interaction of Domain-Specific Knowledge and domain-general discovery strategies: A study with sinking objects. Child Development, 67(6), 2709–2727. https://doi.org/10.2307/1131748
- Penner, D. E., & Klahr, D. (1996b). When to trust the data: Further investigations of system error in a scientific reasoning task. Memory & Cognition, 24, 655–668. https://doi.org/10.3758/BF03201090
- Petticrew, M., & Roberts, H. (2006). Systematic reviews in the social sciences: A practical guide. Malden, MA, USA: Blackwell Publishing Ltd. https://doi.org/10.1002/9780470754887
- Popper, K. R. (1966). Logik der Forschung [The logic of scientific discovery]. J.C.B. Mohr.
- Quinn, J., & Alessi, S. (1994). The effects of simulation complexity and hypothesis generation strategy on learning. Journal of Research on Computing in Education, 27, 75–91. https://doi.org/10.1080/08886504.1994.10782117
- Ramnarain, U. (2012). Exploring the use of a cartoon as a learner scaffold in the planning of scientific investigations. Perspectives in Education, 30(2), 50–61. Retrieved July 9, 2021, from https://www.ajol.info/index.php/pie/article/view/81906
- Reimann, P. (1991). Detecting functional relations in a computerized discovery environment. Learning and Instruction, 1, 45–65. https://doi.org/10.1016/0959-4752(91)90018-4
- Roberts, R., & Gott, R. (2006). Assessment of biology investigations. Journal of Biological Education, 37(3), 114–121. https://doi.org/10.1080/00219266.2003.9655865
- Roberts, R., Gott, R., & Glaesser, J. (2010). Students’ approaches to open‐ended science investigation: The importance of substantive and procedural understanding. Research Papers in Education, 25, 377–407. https://doi.org/10.1080/02671520902980680
- Rönnebeck, S., Bernholt, S., & Ropohl, M. (2016). Searching for a common ground – A literature review of empirical research on scientific inquiry activities. Studies in Science Education, 52(2), 161–197. https://doi.org/10.1080/03057267.2016.1206351
- Sawyer, K. (2006). Introduction. In K. Sawyer (Ed.), The Cambridge handbook of the learning sciences (pp. 1–18). Cambridge University Press.
- Schauble, L. (1990). Belief revision in children: The role of prior knowledge and strategies for generating evidence. Journal of Experimental Child Psychology, 49, 31–57. https://doi.org/10.1016/0022-0965(90)90048-D
- Schauble, L. (1996). The development of scientific reasoning in knowledge-rich contexts. Developmental Psychology, 32, 102–119. https://doi.org/10.1037/0012-1649.32.1.102
- Schauble, L., Glaser, R., Duschl, R. A., Schulze, S., & John, J. (1995). Students’ understanding of the objectives and procedures of experimentation in the science classroom. The Journal of the Learning Sciences, 4, 131–166. https://doi.org/10.1207/s15327809jls0402_1
- Schauble, L., Glaser, R., Raghavan, K., & Reiner, M. (1991a). Causal models and experimentation strategies in scientific reasoning. The Journal of Learning Sciences, 1(2), 201–238. https://doi.org/10.1207/s15327809jls0102_3
- Schauble, L., Klopfer, L. E., & Raghavan, K. (1991b). Students’ transition from an engineering model to a science model of experimentation. Journal of Research in Science Teaching, 28, 859–882. https://doi.org/10.1002/tea.3660280910
- Schrempp, I., & Sodian, B. (1999). Wissenschaftliches Denken im Grundschulalter: Die Fähigkeit zur Hypothesenprüfung und Evidenzevaluation im Kontext der Attribution von Leistungsergebnissen [Scientific thinking at primary school age: The ability to test hypotheses and evaluate evidence in the context of attributing performance outcomes]. Zeitschrift für Entwicklungspsychologie und Pädagogische Psychologie, 31(2), 67–77. https://doi.org/10.1026//0049-8637.31.2.67
- Schroeder, C. M., Scott, T. P., Tolson, H., Huang, T.-Y., & Lee, Y.-H. (2007). A meta-analysis of national research: Effects of teaching strategies on student achievement in science in the United States. Journal of Research in Science Teaching, 44(10), 1436–1460. https://doi.org/10.1002/tea.20212
- Schwichow, M., Croker, S., Zimmerman, C., Höffler, T., & Härtig, H. (2016). Teaching the control-of-variables strategy: A meta-analysis. Developmental Review, 39, 37–63. https://doi.org/10.1016/j.dr.2015.12.001
- Sharon, A. J., & Baram‐Tsabari, A. (2020). Can science literacy help individuals identify misinformation in everyday life? Science Education, 104, 873–894. https://doi.org/10.1002/sce.21581
- Shavelson, R. J., Ruiz-Primo, M. A., & Wiley, E. W. (1999). Note on sources of sampling variability in science performance assessments. Journal of Educational Measurement, 36(1), 61–71. https://doi.org/10.1111/j.1745-3984.1999.tb00546.x
- Shute, V. J., & Glaser, R. (1990). A large-scale evaluation of an intelligent discovery world: Smithtown. Interactive Learning Environments, 1, 51–77. https://doi.org/10.1080/1049482900010104
- Siegler, R. S., & Liebert, R. M. (1975). Acquisition of formal scientific reasoning by 10- and 13-year-olds: Designing a factorial experiment. Developmental Psychology, 11, 401–402. https://doi.org/10.1037/h0076579
- Siler, S. A., & Klahr, D. (2012). Detecting, classifying, and remediating Children’s explicit and implicit misconceptions about experimental design. In R. W. Proctor & E. J. Capaldi (Eds.), Psychology of science (pp. 137–180). Oxford University Press.
- Stender, A., Schwichow, M., Zimmerman, C., & Härtig, H. (2018). Making inquiry-based science learning visible: The influence of CVS and cognitive skills on content knowledge learning in guided inquiry. International Journal of Science Education, 40(15), 1812–1831. https://doi.org/10.1080/09500693.2018.1504346
- Steuer, G., Rosentritt-Brunn, G., & Dresel, M. (2013). Dealing with errors in mathematics classrooms. Structure and relevance of perceived error climate. Contemporary Educational Psychology, 38(3), 196–210. https://doi.org/10.1016/j.cedpsych.2013.03.002
- Tairab, H. H. (2015). Assessing students’ understanding of control of variables across three grade levels and gender. International Education Studies, 9(1), 44–54. https://doi.org/10.5539/IES.V9N1P44
- Tschirgi, J. E. (1980). Sensible reasoning: A hypothesis about hypotheses. Child Development, 51, 1–10. https://doi.org/10.2307/1129583
- Tulis, M., Steuer, G., & Dresel, M. (2016). Learning from errors: A model of individual processes. Frontline Learning Research, 4(2), 12–26. http://dx.doi.org/10.14786/flr.v4i2.168
- Valanides, N., Papageorgiou, M., & Angeli, C. (2013). Scientific investigations of elementary school children. Journal of Science Education and Technology, 23(1), 26–36. https://doi.org/10.1007/s10956-013-9448-6
- van Joolingen, W. R., & de Jong, T. (1991). Supporting hypothesis generation by learners exploring an interactive computer simulation. Instructional Science, 20, 389–404. https://doi.org/10.1007/BF00116355
- van Uum, M. S. J., Verhoeff, R. P., & Peeters, M. (2017). Inquiry based science education: Scaffolding pupils’ self-directed learning in open inquiry. International Journal of Science Education, 39(18), 2461–2481. https://doi.org/10.1080/09500693.2017.1388940
- Vorholzer, A., & von Aufschnaiter, C. (2019). Guidance in inquiry-based instruction – An attempt to disentangle a manifold construct. International Journal of Science Education, 41(11), 1562–1577. https://doi.org/10.1080/09500693.2019.1616124
- Wahser, I., & Sumfleth, E. (2008). Training experimenteller Arbeitsweisen zur Unterstützung kooperativer Kleingruppenarbeit im Fach Chemie. [Training of experimental working methods to support cooperative work in small groups in the subject chemistry]. Zeitschrift für Didaktik der Naturwissenschaften, 14, 219–241. https://archiv.ipn.uni-kiel.de/zfdn/pdf/14_012_Wahser_Sumfleth.pdf
- Wason, P. C. (1960). On the failure to eliminate hypotheses in a conceptual task. Quarterly Journal of Experimental Psychology, 12(3), 129–140. https://doi.org/10.1080/17470216008416717
- Wellnitz, N., & Mayer, J. (2011). Modelling and assessing scientific methods. In the Proceedings of the Annual meeting of the National Association of Research in Science Teaching (NARST), Orlando, Florida, United States. NARST.
- White, B. Y. (1993). ThinkerTools: Causal models, conceptual change, and science education. Cognition and Instruction, 10, 1–100. https://doi.org/10.1207/s1532690xci1001_1
- White, B. Y., & Frederiksen, J. R. (1998). Inquiry, modeling, and metacognition: Making science accessible to all students. Cognition and Instruction, 16(1), 3–118. https://doi.org/10.1207/s1532690xci1601_2
- Winter, V., Kranz, J., & Möller, A. (2022). Climate Change Education Challenges from Two Different Perspectives of Change Agents: Perceptions of School Students and Pre-Service Teachers. Sustainability, 14(10). https://doi.org/10.3390/su14106081
- Wu, H.-K., & Wu, C.-L. (2011). Exploring the development of fifth graders’ practical epistemologies and explanation skills in inquiry-based learning classrooms. Research in Science Education, 41(3), 319–340. https://doi.org/10.1007/s11165-010-9167-4
- Zhai, J., Jocz, J. A., & Tan, A.-L. (2014). ‘Am I Like a scientist?’: Primary children’s images of doing science in school. International Journal of Science Education, 36(4), 553–574. https://doi.org/10.1080/09500693.2013.791958
- Zimmerman, C. (2007). The development of scientific thinking skills in elementary and middle school. Developmental Review, 27(2), 172–223. https://doi.org/10.1016/j.dr.2006.12.001
- Zimmerman, C., Raghavan, K., & Sartoris, M. L. (2003). The impact of the Mars curriculum on students’ ability to coordinate theory and evidence. International Journal of Science Education, 25, 1247–1271. https://doi.org/10.1080/0950069022000038303
Appendix
Results of the expert rating assigning learners challenges to the inquiry phases of an experiment
Note. Learners’ challenges and their descriptions identified in the original studies were assigned by experts (n = 17) to an inquiry phase (stating research questions, generating hypotheses, planning and conducting an experiment, data analysis and drawing conclusions) or to the category multiple inquiry phases of an experiment. The number of expert ratings for each challenge, the frequency of assigning the challenge to the respective inquiry phase in percent (based on the mode), the mean answers per rater (multiple answers were possible) and krippendorff’s alpha are depicted in the table below. In the category ‘multiple phases’ the mode is displayed in squared brackets (1 = stating research questions; 2 = generating hypotheses; 3 = planning and conducting an experiment and 4 = data analysis and drawing conclusions). Challenges marked with an asterisk are specific learner approaches that do not necessarily comprise the successful outcome of an inquiry phase or the outcome of an experiment in general but need to be reflected and addressed in the learning process to prevent subsequent difficulties.