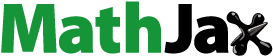
ABSTRACT
Assessment for learning (AfL) can facilitate students’ development of metacognition. However, teachers often struggle with the implementation of AfL in their classroom. The dynamic approach can help teachers develop professionally in the complex competency that is AfL. The dynamic approach is based on four principles: (a) addressing the professional needs of teachers, (b) integrating skills, (c) explaining underlying mechanisms, and (d) implementing support and feedback. In this experimental study, we investigated the effect on students’ metacognition of an AfL teacher professional development program for secondary mathematics teachers based on the dynamic approach (nteachers = 47, nstudents = 803). We found a statistically significant positive effect on students’ ability to predict (d = 0.24) and evaluate (d = 0.22), but not on students’ ability to plan.
Introduction
Metacognition is an important predictor of student achievement and student motivation (e.g., Desoete & De Craene, Citation2019). It literally means “thinking about thinking” (Flavell, Citation1976) and is commonly defined as “one’s knowledge concerning one’s own cognitive processes and products or anything related to them … [and] the active monitoring and consequent regulation and orchestration of these processes” (Flavell, Citation1976, p. 232). However, without instructional support for students, it is unlikely that they will develop their metacognition (Baars et al., Citation2020). One promising approach to help students to develop their metacognition is the implementation of assessment for learning (AfL) in the classroom (Nicol & MacFarlane-Dick, Citation2006). AfL is:
assessment to the extent that evidence about student achievement is elicited, interpreted, and used by teachers, learners, or their peers, to make decisions about the next steps in instruction that are likely to be better, or better founded, than the decisions they would have taken in the absence of the evidence that was elicited. (Wiliam & Leahy, Citation2015, p. 8)
However, the use of AfL in the classroom is not simple (Heitink et al., Citation2016; Kippers et al., Citation2018). Teachers are not only supposed to develop and share the learning goals with their students, to elicit, analyze, and interpret collected data, and to make decisions based on those data, but also to include students as active agents in their own and their peers’ learning processes. This complexity can make it difficult for teachers to implement AfL, and because of that, the potential benefits of AfL for students’ metacognitive, self-regulatory skills may not be realized.
Therefore, a teacher professional development program for teachers to facilitate their implementation of assessment for learning (Heitink et al., Citation2016) may be beneficial. The dynamic approach to teacher professional development could be appropriate for such a complex teacher skill. The dynamic approach takes the interrelatedness of the different teacher skills involved and the varying professional needs regarding AfL skills into account (e.g., Antoniou et al., Citation2015). Recognizing and addressing the different (levels of these) professional needs (a common feature of AfL) in a teacher professional development program that uses the dynamic approach makes it more likely that teachers can improve the skills necessary for AfL (Christoforidou et al., Citation2014).
In the present study, we assessed the impact on students’ metacognition of a teacher professional development program for AfL based on the dynamic approach. We expected that if teachers are supported in the implementation of AfL in their classrooms, their students’ use of metacognition may be facilitated, and through this, the students may show better metacognitive development compared to students of teachers without participation in a teacher professional development program, as we will further explain in the theoretical framework. The research question for this study was: “What is the effect on student metacognition of a teacher professional development program for assessment for learning based on the dynamic approach?”
Theoretical framework
Metacognition
Metacognition has two parts (Kyriakides et al., Citation2020): (a) metacognitive knowledge and (b) metacognitive skills. Metacognitive knowledge is “declarative knowledge stored in memory and comprises models of cognitive processes, such as language, memory, and so forth” (Efklides, Citation2008, p. 278). Students depend on metacognitive skills in order to use their metacognitive knowledge effectively (Desoete & De Craene, Citation2019). Metacognitive knowledge is updated continuously as new information coming from observing one’s actions and the consequences of these actions while working on a task is integrated. Moreover, the integration of new information can also result from communication with others, such as through feedback (Efklides, Citation2008). Metacognitive skills refers to “the deliberate use of strategies (i.e., procedural knowledge) in order to control cognition” (Efklides, Citation2008, p. 280). Students’ metacognitive skills typically develop as students age (Veenman et al., Citation2006); however, this development may also be influenced by external factors, such as opportunities to use feedback, and dialogue about feedback (Nicol & MacFarlane-Dick, Citation2006). Important metacognitive skills are (a) planning, (b) monitoring, and (c) evaluating (Desoete & De Craene, Citation2019; Kyriakides et al., Citation2020).
Planning
Prior to starting a task, students may begin by planning their learning strategies (how, what, and when to act) in order to accomplish the learning goals (Desoete & De Craene, Citation2019). This planning can involve activities such as determining the kind of problem. Moreover, planning requires making connections between prior and current knowledge and selecting appropriate strategies to solve the problem (Shilo & Kramarski, Citation2019).
Monitoring
After students have planned their learning strategies and started the assignment, they may engage in monitoring their progress towards achieving the learning goals. Students can ask themselves questions during tasks such as “Does the solution make sense?” (Desoete & De Craene, Citation2019). If the answer is no, this might mean asking extra, clarifying, questions or investigating models of how to solve the problem.
Monitoring their results can later help students to predict the difficulty of a task and their capacity to address a task successfully (Baars et al., Citation2020). For example, students may decide to spend more time on tasks with larger learning gaps. For students to be able to predict the difficulty of tasks correctly, it is necessary that they have some prior knowledge of the topic, or familiarity with the type of task, so that they can distinguish better between apparent and real difficulties (Desoete & De Craene, Citation2019).
Evaluating
When the task has been completed, students may evaluate the effectiveness of their learning strategies. Students can use information gained from the completed task to improve their understanding, which, in turn, can improve their explanations and their approach to their next task (Shilo & Kramarski, Citation2019). However, when external feedback is provided, the student might also diagnose an error in their work, which, in turn, can lead to a re-do, or an adjustment of their work and the use of alternate learning strategies.
Assessment for learning
Teachers can stimulate students to use metacognitive skills by making the formative AfL cycle of “Where is the learner going?”, “Where is the learner now?”, and “How is the learner going there?” part of the classroom routine. For example, having students formulate and share learning goals can influence their knowledge of course expectations, which in turn can influence their effective reception of feedback. Below we will further describe the three formative questions, and how these can support students’ metacognitive skills (Nicol & MacFarlane-Dick, Citation2006; Wiliam & Leahy, Citation2015).
Where is the learner going?
Answering this question involves clarifying, sharing, and understanding learning goals and success criteria. Learning goals are what a teacher wants the learner to learn. Success criteria are more concrete parameters that can identify precisely where students are in their learning process compared to the learning goals (Wiliam & Leahy, Citation2015). The teacher can share learning goals and success criteria in the form of dialogues in which both the teacher and student play a role. For example, students can compare and assess exemplars: “carefully chosen samples of student work which are used to illustrate dimensions of quality and clarify assessment expectations” (Carless & Chan, Citation2017, p. 930). If learning goals and success criteria are clarified for both students and teachers, teachers and students can elicit evidence in a more targeted manner. In addition, clarification of learning goals and success criteria can prompt students to better plan and further manage their learning strategies, as they may have a better sense of what is expected of them (Carless & Winstone, Citation2020; Winne, Citation2018).
Where is the learner now?
Answering this question involves engineering classroom discussions and other learning tasks in order to continually elicit evidence of student progress compared to the learning goals. There is a wide variety of qualitative and quantitative assessment techniques that can give both teacher and students insight into where the students are in the learning process (van der Kleij et al., Citation2015). For example, teachers can pose hinge questions, carefully designed multiple-choice questions that can indicate student misconceptions (Wiliam & Leahy, Citation2015). The evidence that results from such assessment techniques can serve as continual feedback for students, which they can use, in turn, to monitor and evaluate their learning progress (Nicol & MacFarlane-Dick, Citation2006). Related to this, students’ progress can also be self-assessed and peer assessed, for example, with the use of co-constructed success criteria. In this way, students can become more accurate at self-reflection, and their improvement efforts can be better directed (Carless & Winstone, Citation2020). When students are not involved in assessment, they usually find the assessment process incomprehensible, which can make it difficult for them to effectively predict, plan, monitor, and evaluate their learning (Carless & Winstone, Citation2020).
How is the learner going there?
Feedback based on the collected information, which can be given by both teacher and students, can be an effective way of improving students’ understanding and learning (Hattie & Timperley, Citation2007). The use of feedback may be the most complex strategy of all, as the effects of feedback on student achievement are inconsistent and not always positive (Brooks et al., Citation2021). Recent studies (Gulikers et al., Citation2021; Panadero et al., Citation2018) have suggested that student involvement in the other AfL(-related) strategies may be beneficial for students’ feedback use. Students can be stimulated to improve their own learning process based on feedback only when that feedback gives information on where students are compared to the learning goals and success criteria. Moreover, feedback must give enough information about how specific strategies can help students to improve (Nicol & MacFarlane-Dick, Citation2006). For teachers to be able to carry out such improvements, they can best give feedback during the learning process. Teachers often decide to give feedback only at the end of the task completion process, but it can be more effective when provided during the process (Carless & Winstone, Citation2020).
Not only can the elicited evidence be used as feedback for students, it can also give teachers insight into how to continue with their teaching efforts. Teachers may, for example, diagnose a misconception in some or all students’ knowledge of a topic, and decide to use an alternate instructional strategy, such as instructional methods that require active student thinking rather than passive listening to repeated explanations of the subject matter. The use of such adaptations to match students’ level of understanding is similar to the concept of differentiated instruction, when “teachers deliberately plan such adaptations to facilitate students’ learning and execute these adaptations during their lessons” (Smale-Jacobse et al., Citation2019, p. 1).
Professional development for assessment for learning
AfL moves teachers away from a teaching approach focused more on “transmission” (i.e., telling students what to do) to a way of teaching in which students take an active role (Brooks et al., Citation2021). When students take this active role, they have more ownership over their own learning progress, which may instigate the development of metacognition. Competencies for AfL include, for example, the ability of teachers to formulate appropriate learning goals and success criteria for their subject domain as well as the ability to share these meaningfully with their students. As every step of AfL depends on the others, AfL can be defined as a complex teacher competence.
Teacher professional development may be helpful to equip teachers for the implementation of AfL in their own classroom. The dynamic approach can be used for teacher professional development in AfL (Antoniou et al., Citation2015). The dynamic approach is characterized by the following core principles:
Addressing the professional needs of teachers. Teachers may vary greatly in their ability and their motivation to implement AfL in the classroom. Where some teachers may already coherently implement all strategies belonging to AfL, other teachers may still struggle with the first AfL strategy of formulating suitable learning goals for their target groups of students (Kippers et al., Citation2018). Teacher development is more likely to occur when teachers’ specific professional needs are taken into account and addressed in a teacher professional development (TPD) program (Christoforidou et al., Citation2014). In addition, when teachers’ needs are analyzed prior to (but also during) the TPD program, it is possible for the TPD program to better connect to teachers’ practices (Darling-Hammond et al., Citation2017). Such coherence between TPD and teaching practice increases relevance for teachers, which, in turn, can help make change happen (“coherence”; Desimone, Citation2009).
Integrating skills. As stated, each AfL strategy is dependent on the others (Wiliam & Leahy, Citation2015). An integrated approach to addressing these skills in teacher professional development is necessary in order to help teachers tackle the complexity of AfL and transfer the complex skills to their own classroom. This means, for example, that the TPD program should include not only discussing different forms of assessment techniques but also explaining the relation of these assessment techniques with success criteria, and the timing of using assessment (results). In addition, not only do the AfL skills depend on each other during their development, the development of these skills also depends on teachers’ development of knowledge and beliefs regarding AfL (Heitink et al., Citation2016). For example, when teachers have a more positive attitude towards AfL, they can also feel greater appreciation when they are given feedback on their implementation of AfL.
Explaining underlying mechanisms. For teachers to recognize the potential benefits of AfL for student learning in their own classrooms, it is helpful that they understand why AfL can improve students’ metacognition. For example, an AfL mechanism might be that sharing learning goals can create transparency on what is expected from students, which can lead to increased student motivation (Carless & Winstone, Citation2020). Not only will it be helpful for teachers to have such mechanisms explained and demonstrated, ideally, teachers can also experience these mechanisms themselves. Through teachers’ use of action plans, they can create their own path for experimentation with respect to the implementation of AfL in their own classrooms (“active learning”; Desimone, Citation2009).
Implementation support and feedback. Teachers need help not only in the form of explanations and demonstrations but also in the form of real-time, individual, feedback from experts on the topic of AfL. Such feedback, as well as the explanations and demonstrations, can be especially supportive if the expert is also knowledgeable in the teacher’s specific subject area (“content”; Desimone, Citation2009). When teachers are experimenting with AfL in their classrooms, these experts can monitor and elicit evidence on whether teachers are able to transformatively apply TPD content to their lessons, and are not just layering new techniques on top of their current practice (Darling-Hammond et al., Citation2017). A potential way to monitor this effectively is through reflective discussion during the teacher professional development program (Korthagen, Citation2017). In addition, the reflective discussions, facilitated by knowledgeable experts, may also provide the needed scaffolding for teachers to take actual steps to transform their lessons (Darling-Hammond et al., Citation2017; Desimone, Citation2009). Experts in AfL can help to identify teachers’ underlying beliefs and misconceptions regarding AfL that may restrict their professional development (Timperley, Citation2008).
Method
Context
This study, which took place in the Netherlands, was part of the Erasmus project “Promoting Formative Assessment: From Theory to Practice to Policy” (FORMAS). One aim of this project was to investigate the impact on student achievement and metacognition of a teacher professional development program on AfL for secondary math teachers in four countries: Belgium, Cyprus, Greece, and the Netherlands.
Participants
The participants in this study were recruited via convenience sampling. Approximately all 462 secondary school locations in the Netherlands with the necessary school levels (i.e., general higher education and pre-university levels) of the lower grades (i.e., ages 12–14), were approached for participation in this study via email. In addition, calls for participation were published in professional magazines and a mathematics newsletter. A total of 33 schools decided to participate, with one to four mathematics teachers per school (N = 75). These teachers were asked to participate in this study with only one class of students, and were randomly assigned to either the experimental (n = 36) or the control group (n = 39). As we randomized at the school level, this could have caused the slightly uneven initial group sizes. Unfortunately, 27 teachers dropped out during our study (i.e., they did not participate in both measurements). The main reason for dropout was a shift in priorities, which was caused by extra workload or, especially during the posttest, the COVID-19 pandemic. Ultimately, 26 teachers in the experimental group and 22 teachers in the control group participated in both the pre- and posttests (see ).
Table 1. Characteristics of the teachers in the experimental and the control groups.
We therefore ended up with a total data set of 803 students (43.1% in the experimental group and 70.2% in the control group) who completed both the pre- and the posttest, out of the original 1,443 students. The gender distribution of the participating students did not differ significantly between the experimental and the control groups: χ²(1, N = 803) = 0.854, p = .36. Unfortunately, the dropout caused by COVID-19 led to a statistically significant difference for grade between these groups: χ²(2, N = 803) = 228.40, p = .00 (see for the characteristics of the participating students).
Table 2. Characteristics of the students who participated in both the pretest and the posttest.
Instruments
The metacognitive test
We developed four metacognitive mathematical tests, originally in English and for this study translated into and validated in Dutch. These four tests served as pre- and posttests for the different grade levels (see ), which included tasks in which students could show their metacognitive knowledge and skills (cf. Magno, Citation2009).
Table 3. Order of administration of the metacognitive tests.
Each test consisted of five exercises with a similar format, addressing declarative and procedural knowledge, planning, predicting, and evaluating (see the scales below). When we developed the exercises, it proved to be impossible to develop exercises for the monitoring and evaluating scales that were distinctive enough to measure these constructs separately. We therefore had to exclude the monitoring scale from the instrument. In addition, we had to combine declarative and procedural knowledge into one scale.
Declarative and procedural knowledge (Exercises 2 and 3): Students were asked to evaluate several solutions to a proposed problem and to indicate which solution was best. An example item can be found in .
Planning (Exercise 4): Students were asked to evaluate and indicate which strategies were needed to solve a specific problem. An example can be found in .
Predicting (Exercise 1): Students were asked to predict their ability to solve a problem on a 4-point Likert scale (4 = sure I cannot to 1 = sure I can). An example is shown in .
Evaluating. (Exercise 5): Students were asked to solve the five problems posed in Exercise 1, and to indicate how sure they were about the correctness of their solution on a 4-point Likert scale (4 = sure it is wrong to 1 = sure it is correct). An example can be found in .
The teacher questionnaire
The self-perception questionnaire on teacher assessment skills, developed by Christoforidou et al. (Citation2014), was used to evaluate teachers’ professional needs at the start (and end) of the teacher professional development program. This teacher questionnaire, translated into Dutch for our study, included 115 items focusing on the phase of assessment (i.e., constructing, administering, recording, analyzing, and reporting), assessment techniques (i.e., oral, written, or performance assessment), and the assessor (i.e., teacher, student, peer). In addition, these items were measured in relation to various aspects of assessment: (a) frequency: the number of assessment moments in a classroom, (b) focus: use of multiple assessment moments to inform decision(s), (c) stage: the timing of the assessment moments, (d) quality: construct validity of assessment, and (e) differentiation: adapting assessment and/or feedback to student. Most items were measured on a 5-point Likert scale (1 = never to 5 = every lesson).
Rasch’s extended logistic model (Andrich, Citation1988) was used to identify a hierarchy of difficulty in items, which were consequently clustered into levels of difficulty that may be taken to stand for types of teacher behavior that move from relatively easy to more difficult (cf. Christoforidou et al., Citation2014). Three teacher developmental stages were distinguished prior to the professional development program:
Group A (“beginner”): seven teachers, who mainly used written assessments to measure student achievement in mathematics for summative purposes.
Group B (“advanced”): 13 teachers, who used different assessment techniques to measure achievement in mathematics, but without defining appropriate success criteria or providing constructive feedback.
Group C (“expert”): six teachers, who used assessment techniques to measure more complex educational objectives to provide constructive feedback, but yet without involving students in the assessment process and without differentiating their assessment practice.
Procedure
The teacher professional development program
The teachers in the experimental group participated in the teacher professional development program that was scheduled to last 1 school year and consisted of five sessions, each session emphasizing one or more of the formative AfL questions. In the first, introductory session, the teacher questionnaire was also administered.
We had planned different activities for each group of teachers, based on their developmental stage. However, after the first session we noted that teachers in Group A felt that their topics were less related to their specific subject or not advanced enough, and therefore less relevant to them. We changed our approach from differentiation between groups (different topics and activities per group) to differentiation within groups (same topics and activities, emphasis on developmental stage within individual feedback) on the basis of these reactions. The teacher professional development program included several recurring activities, in the following order:
Experimentation and reflective inquiry. Between sessions, teachers were asked to try to implement the content learned, and/or apply the developed tools in their own lessons. In this way, we could start the next session with reflective inquiry regarding the successfulness of this implementation, with questions such as “Why did you implement the AfL strategy in this way in your classroom?” and “What effect of using the AfL strategy did you notice?” Teachers’ reflection on their own teaching practice could be stimulated through such inquiry. In addition, the reflection gave the AfL trainers the opportunity to fight remaining misconceptions about AfL, such as the idea that the implementation of AfL is too time intensive, when teachers see it as an “extra” element on top of their current teaching.
Explanation and demonstration. Teachers were given an explanation of the definition of each AfL strategy and why the AfL strategy could be used to improve AfL in their classrooms. These explanations were often additionally substantiated by video examples of teachers using the AfL strategy in their classroom.
Hands-on activity. The largest part of each session was spent on a hands-on activity related to the AfL strategies discussed in that session. For example, the session on “where is the learner going” was mostly spent on developing and formulating learning goals and criteria for success for the next chapter or marking period together. Moreover, teachers were asked to think about ways to share the learning goals and criteria for success meaningfully with their students. Other examples of hands-on activities included developing rubrics (i.e., a collection of learning goals and success criteria, ranked from less to more advanced) and developing hinge questions.
Developing a lesson plan. Teachers were asked to develop a plan for a lesson in which they could integrate all of the discussed content from this session and previous sessions to answer all formative questions: “Where is the learner going?”, Where is the learner now?”, and “How is the learner going there?” They were supported in this process by each other and the AfL expert. After the session, teachers were also given the opportunity to send the lesson plan to the AfL expert for extra feedback.
The data collection
The metacognitive test was scheduled to be administered to students between the first and the second session (pre), and after the fifth session (post). This was done so that teachers could administer the test themselves. Unfortunately, due to the outbreak of COVID-19, schools closed after our fourth session. We rescheduled the fifth session to September of the next school year. The post-measurement was done before the summer break and thus also before the fifth session. Students in the Netherlands often change teachers after the summer holiday, which could have impeded our administration of the posttest. After schools changed to online lessons because of COVID-19, we changed from in-school administration during the pretest to digital administration during the posttest. Teachers were all asked to administer the tests themselves during an online lesson.
Analysis
Most of the items were rated on a 4-point Likert scale. Other items were scored based on an answer key and added to the data file. We checked whether the groups were comparable by comparing the students’ metacognitive pretest scores. There was no significant difference for predicting [t(795) = 2.806, p = .25] and evaluating [t(799) = 1.919, p = .55] across the experimental and the control groups. This was not the case for planning [t(801) = 2.806, p = 0.01]. However, the mean difference (ΔM = 0.19) in pretest scores for planning between these two groups, in favor of the control group, was within the boundaries for comparison (What Works Clearinghouse, Citation2020). Moreover, there was no interaction effect between dropout and group (experimental-control) on the pretest scores for any of the metacognitive variables, which indicates that there was no relation between a student in a particular group dropping out and their pretest score. Due to the COVID-19 pandemic, this study had a high dropout level, which decreased its statistical power. For this reason, we decided to equate the scores of the three grades. This was done by using item response theory techniques that are similar to the techniques used by the Programme for International Student Assessment (Organisation for Economic Co-operation and Development, Citation2017).
As the data were nested (Level 1: students and Level 2: teachers), we decided to conduct multilevel analyses to assess the impact of the teacher professional development program on the students’ metacognitive skills. The high dropout rate meant that the statistical power was insufficient to conduct a multivariate multilevel analysis. Multilevel analyses were conducted per metacognitive skill: planning, predicting, and evaluating. These analyses were carried out with MLwiN software (Charlton et al., Citation2020). We analyzed an empty model (i.e., without any explanatory variables added) to investigate the variance in the scores across teachers and students:
In Model 1, the explanatory variables of prior achievement and gender were added to the empty model:
To be able to assess the effectiveness of the teacher professional development program, we added the exploratory variable of group (0 = control, 1 = experimental) in Model 2:
We also evaluated the improvement of (self-reported) assessment skills by teachers, in order to validate that the changes at the student level were indeed caused by changes at the teacher level. We found an effect size of 0.88 (see ).
Table 4. Standardized means and SD of pre- and posttest of teacher assessment skills per group, and the corresponding t test values.
Results
Descriptive statistics
The pre- and posttest scores on the metacognitive tests per construct can be found in . As can be observed, the scores of the students in the control group are almost the same for both measurement points, whereas students in the experimental group scored higher on the posttest.
Table 5. Standardized pretest and posttest scores per group.
Planning
The results of the multilevel analysis for planning can be found in . Contrary to our expectations, the second model showed that the teacher professional development program did not have a statistically significant effect on the planning ability of students, β = 0.12, p < .10.
Table 6. Parameter estimates and standard errors for planning (students within teachers).
Predicting
The results of the multilevel analysis for predicting can be found in . The second model revealed that the teacher professional development program had a statistically significant positive effect on students’ ability to predict their own mathematical skills, β = 0.23, p < .05. The standardized effect size was d = 0.24, which is considerable (Lipsey et al., Citation2012).
Table 7. Parameter estimates and standard errors for predicting (students within teachers).
Evaluating
The results of the multilevel analysis for evaluating can be found in . The second model revealed that the teacher professional development program had a statistically significant positive effect on students’ ability to predict their own mathematical skills, β = 0.22, p < .05. The standardized effect size was d = 0.22, which is considerable.
Table 8. Parameter estimates and standard errors for evaluating (students within teachers).
Discussion
The aim of this study was to investigate whether teacher professional development for AfL based on the dynamic approach would be beneficial for students’ development of metacognition. The results showed that predicting and evaluating, two of the three measured metacognitive skills, improved considerably for students whose teacher participated in our teacher professional development program. The four dynamic-approach principles likely helped teachers to integrate AfL in their practice in various ways.
First, we addressed teachers’ professional needs in a differentiated manner, which may have allowed us to adapt to teachers’ zones of proximal development and connect more closely to their daily practice (Darling-Hammond et al., Citation2017; Timperley, Citation2008). We did not offer the initially intended between-group form of differentiation because many of the teachers in the “beginner” group were not content to be in that group. In their opinion, that would not benefit them as much, and they were more interested in the topics of the other groups, which included more applied activities for AfL such as developing rubrics and hinge questions.
Second, AfL was taught and practiced as a whole task, meaning that the focus throughout the program was on the coherent implementation of the set of AfL strategies. In the first session, for example, teachers were asked to formulate appropriate learning goals for their class. In the second session, teachers were asked to discuss what questions could help them elicit evidence as to where students were in their progress towards the previously formulated learning goals. Still, during each session, we had to put more emphasis on one particular AfL strategy to be able to discuss that one more extensively. The notion of the importance of coherence between all AfL strategies, especially the more difficult AfL strategies, such as the involvement of students in assessment (Christoforidou et al., Citation2014), could have been lost for some of the teachers.
Third, as a way to incorporate active learning (e.g., Desimone, Citation2009), teachers were not only “told” that AfL works but were also stimulated to ask and explain how AfL could benefit their teaching practice. For example, teachers were shown video examples of other teachers implementing AfL with varying degrees of success, and also asked to compare the video examples with their own standards for AfL. In this way, teachers could better understand why AfL can help to improve teaching quality.
Fourth, teachers were supported by each other and by knowledgeable AfL experts in their endeavor to integrate AfL in their teaching practice. As most teachers were new to the concept of AfL and some teachers had misconceptions regarding AfL (“I do not have time to take extra follow-up steps after having assessed my student’s knowledge”), it may have been beneficial that they received external feedback. This support is in line with guidelines by Timperley (Citation2008) and Darling-Hammond et al. (Citation2017), who mentioned that an external expert not only challenges assumptions but can also scaffold teachers to implement practices that will make a difference for student learning.
Our use of these principles as guides meant that teachers appeared to have been supported effectively to implement AfL in their own classroom, and this, in turn, appeared to have facilitated students’ development of two components of metacognition. It is interesting that this effect occurred without specific attention to the concept of metacognition during the intervention. It may indeed be possible that just the structure that AfL provides, such as clear expectations, frequent assessments, and feedback, helped to develop students’ metacognitive skills (Nicol & MacFarlane-Dick, Citation2006). Our findings could also demonstrate the proposed overlap between activities related to AfL and metacognition, such as working towards specific goals and monitoring the progress towards these goals (Gulikers et al., Citation2021; Panadero et al., Citation2018).
Limitations
It is difficult for us to explain why the metacognitive skills of predicting and evaluating showed statistically significant improvement, whereas planning did not. It is likely that this difference in effects for the different metacognitive skills was due to the way metacognition was measured. A limitation is that we used a proxy for planning that referred to being able to recognize the necessary solutions for problems. Other elements of planning, such as personal preferences regarding goal setting, as well as higher executive skills such as foresight and impulse control (Magno, Citation2009), need to be measured to improve the construct validity of planning. However, these elements are difficult to measure quantitatively, as they are often heavily based on recognition, like rank ordering of goals and strategy selection (Shilo & Kramarski, Citation2019).
The difficulty of measuring metacognition by means of tests alone was also apparent in the fact that we were unable to measure metacognitive knowledge validly. It is likely that instead of measuring metacognitive knowledge, we actually measured mathematical knowledge. Still, the operationalization of metacognitive knowledge used in this study is worth exploring further, as there are indications that such an assessment can be more accurate than a self-report questionnaire on metacognition (e.g., Craig et al., Citation2020).
Another limitation of this study is that it was carried out partly during the COVID-19 pandemic. Due to this, teachers had to switch from in-school lessons to online lessons approximately 2 months prior to the posttests. It is unlikely that teachers implemented AfL in their lessons with the same effectiveness as would have been the case if the lessons had been in person, as the focus of the teacher professional development was not on online lessons. This could have negatively influenced the outcome of this study.
The COVID-19 pandemic also caused differential attrition in our sample. This resulted in some unevenness between the experimental and control groups in their background characteristics. For example, we found that more teachers with more years of experience participated in the experimental group compared to the control group (not statistically significant). Fortunately, our analytical sample remained comparable for the purposes of this study, as students’ pretest scores on the metacognitive skills did not differ statistically significantly between the experimental and control groups. Still, the decrease in the number of students caused a decrease in the power, which made it impossible to use multivariate multilevel analyses, which could have revealed more about the relationship between teacher and student characteristics and the improvement of students’ metacognition.
Recommendations
This relatively large-scale study has shown the potential of teacher professional development for AfL based on the dynamic approach, as improvement in two of students’ metacognitive skills were observed. Still, further investigation into the potential of the dynamic approach for this purpose is necessary, as the metacognitive skill of planning did not improve and two metacognitive components could not be measured. A possibility would be to include open-ended questions with more options in order to capture more detailed elements of metacognition, such as goal setting and effort allocation (Baars et al., Citation2020). As this would, however, result in large amounts of data (e.g., 803 students in our sample), it would be necessary to code all data using artificial intelligence, which has already proven useful in other fields (e.g., peer feedback; Gardner et al., Citation2021).
Moreover, it will be worthwhile to repeat the study in times without a pandemic outbreak to ensure the reliability of the effectiveness of this TPD program. A larger data set could also allow for multivariate multilevel analyses, revealing more about the links between all background variables and the student outcomes with greater certainty. In addition, if this study were implemented and evaluated at a larger scale, this would also make it possible to have a more representative sampling procedure, as opposed to the convenience sampling used in this study. More and stronger variation between participating teachers, such as teachers from different subjects and with and without a preference for AfL, would also make it possible to investigate in greater depth the link between teachers’ attitude, knowledge, and skills on the one hand, and student outcomes on the other.
Disclosure statement
No potential conflict of interest was reported by the authors.
Additional information
Funding
Notes on contributors
Jitske A. de Vries
Jitske A. de Vries, MSc, is a PhD candidate at ELAN Teacher Development, University of Twente, the Netherlands. She is involved in projects on teacher professional development for assessment for learning and has conducted multiple impact studies of teacher professional development programs.
Andria Dimosthenous
Andria Dimosthenous was a postdoctoral researcher at the Department of Education at the University of Cyprus. Currently, she is Assistant Professor of Educational Evaluation at the Department of Preschool Education at the University of Crete. She has participated in several international projects on educational effectiveness and school improvement. Her research interests involve parameters such as the home learning environment that affect student achievement gains.
Kim Schildkamp
Kim Schildkamp is a full professor at ELAN Teacher Development, University of Twente, the Netherlands. Her research focuses on formative assessment (data-based decision making, assessment for learning, and diagnostic testing), the use of research in schools, and big data in education. One of her central questions is how to support schools in the use of data to improve education. She is also the initiator and project leader of the data team® procedure project.
Adrie J. Visscher
Adrie J. Visscher is a full professor at ELAN Teacher Development, University of Twente, the Netherlands. In his research he investigates how the provision of various types of feedback to students, teachers, and schools can support the optimization of the quality of classroom teaching and student learning. His research also focuses on the characteristics of effective teacher professionalization.
References
- Allal, L. (2020). Assessment and the co-regulation of learning in the classroom. Assessment in Education: Principles, Policy & Practice, 27(4), 332–349. https://doi.org/10.1080/0969594X.2019.1609411
- Andrich, D. (1988). A general form of Rasch’s extended logistic model for partial credit scoring. Applied Measurement in Education, 1(4), 363–378. https://doi.org/10.1207/s15324818ame0104_7
- Antoniou, P., Kyriakides, L., & Creemers, B. P. M. (2015). The Dynamic Integrated Approach to teacher professional development: Rationale and main characteristics. Teacher Development, 19(4), 535–552. https://doi.org/10.1080/13664530.2015.1079550
- Baars, M., Wijnia, L., de Bruin, A., & Paas, F. (2020). The relation between students’ effort and monitoring judgments during learning: A meta-analysis. Educational Psychology Review, 32(4), 979–1002. https://doi.org/10.1007/s10648-020-09569-3
- Brooks, C., Burton, R., van der Kleij, F., Ablaza, C., Carroll, A., Hattie, J., & Neill, S. (2021). Teachers activating learners: The effects of a student-centred feedback approach on writing achievement. Teaching and Teacher Education, 105, Article 103387. https://doi.org/10.1016/j.tate.2021.103387
- Carless, D., & Chan, K. K. H. (2017). Managing dialogic use of exemplars. Assessment & Evaluation in Higher Education, 42(6), 930–941. https://doi.org/10.1080/02602938.2016.1211246
- Carless, D., & Winstone, N. (2020). Teacher feedback literacy and its interplay with student feedback literacy. Teaching in Higher Education. Advance online publication. https://doi.org/10.1080/13562517.2020.1782372
- Charlton, C., Rasbash, J., Browne, W. J., Healy, M., & Cameron, B. (2020). MLwiN Version 3.05. Centre for Multilevel Modelling, University of Bristol.
- Christoforidou, M., Kyriakides, L., Antoniou, P., & Creemers, B. P. M. (2014). Searching for stages of teacher’s skills in assessment. Studies in Educational Evaluation, 40, 1–11. https://doi.org/10.1016/j.stueduc.2013.11.006
- Craig, K., Hale, D., Grainger, C., & Stewart, M. E. (2020). Evaluating metacognitive self-reports: Systematic reviews of the value of self-report in metacognitive research. Metacognition and Learning, 15(2), 155–213. https://doi.org/10.1007/s11409-020-09222-y
- Darling-Hammond, L., Hyler, M. E., & Gardner, M. (2017). Effective teacher professional development. Learning Policy Institute.
- Desimone, L. M. (2009). Improving impact studies of teachers’ professional development: Toward better conceptualizations and measures. Educational Researcher, 38(3), 181–199. https://doi.org/10.3102/0013189X08331140
- Desoete, A., & De Craene, B. (2019). Metacognition and mathematics education: An overview. ZDM Mathematics Education, 51(4), 565–575. https://doi.org/10.1007/s11858-019-01060-w
- Efklides, A. (2008). Metacognition: Defining its facets and levels of functioning in relation to self-regulation and co-regulation. European Psychologist, 13(4), 277–287. https://doi.org/10.1027/1016-9040.13.4.277
- Flavell, J. H. (1976). Metacognitive aspects of problem solving. In L. B. Resnick (Ed.), The nature of intelligence (pp. 231–235). Lawrence Erlbaum.
- Gardner, J., O’Leary, M., & Yuan, L. (2021). Artificial intelligence in educational assessment: “Breakthrough? Or buncombe and ballyhoo?”. Journal of Computer Assisted Learning, 37(5), 1207–1216. https://doi.org/10.1111/jcal.12577
- Gulikers, J., Veugen, M., & Baartman, L. (2021). What are we really aiming for? Identifying concrete student behavior in co-regulatory formative assessment processes in the classroom. Frontiers in Education, 6, Article 750281. https://doi.org/10.3389/feduc.2021.750281
- Hattie, J., & Timperley, H. (2007). The power of feedback. Review of Educational Research, 77(1), 81–112. https://doi.org/10.3102/003465430298487
- Heitink, M. C., van der Kleij, F. M., Veldkamp, B. P., Schildkamp, K., & Kippers, W. B. (2016). A systematic review of prerequisites for implementing assessment for learning in classroom practice. Educational Research Review, 17, 50–62. https://doi.org/10.1016/j.edurev.2015.12.002
- Kippers, W. B., Wolterinck, C. H. D., Schildkamp, K., Poortman, C. L., & Visscher, A. J. (2018). Teachers’ views on the use of assessment for learning and data-based decision making in classroom practice. Teaching and Teacher Education, 75, 199–213. https://doi.org/10.1016/j.tate.2018.06.015
- Korthagen, F. (2017). Inconvenient truths about teacher learning: Towards professional development 3.0. Teachers and Teaching, 23(4), 387–405. https://doi.org/10.1080/13540602.2016.1211523
- Kyriakides, L., Anthimou, M., & Panayiotou, A. (2020). Searching for the impact of teacher behavior on promoting students’ cognitive and metacognitive skills. Studies in Educational Evaluation, 64, Article 100810. https://doi.org/10.1016/j.stueduc.2019.100810
- Lipsey, M. W., Puzio, K., Yun, C., Hebert, M. A., Steinka-Fry, K., Cole, M. W., Roberts, M., Anthony, K. S., & Busick, M. D. (2012). Translating the statistical representation of the effects of education interventions into more readily interpretable forms (NCSER 2013-3000). National Center for Special Education Research, Institute of Education Sciences, U.S. Department of Education. https://ies.ed.gov/ncser/pubs/20133000/pdf/20133000.pdf
- Magno, C. (2009). Developing and assessing self-regulated learning. The Assessment Handbook: Continuing Education Program, 1, 26–42.
- Nicol, D. J., & Macfarlane-Dick, D. (2006). Formative assessment and self-regulated learning: A model and seven principles of good feedback practice. Studies in Higher Education, 31(2), 199–218. https://doi.org/10.1080/03075070600572090
- Organisation for Economic Co-operation and Development. (2017). PISA 2015 technical report. https://www.oecd.org/pisa/data/2015-technical-report/
- Panadero, E., Andrade, H., & Brookhart, S. (2018). Fusing self-regulated learning and formative assessment: A roadmap of where we are, how we got here, and where we are going. The Australian Educational Researcher, 45(1), 13–31. https://doi.org/10.1007/s13384-018-0258-y
- Shilo, A., & Kramarski, B. (2019). Mathematical-metacognitive discourse: How can it be developed among teachers and their students? Empirical evidence from a videotaped lesson and two case studies. ZDM Mathematics Education, 51(4), 625–640. https://doi.org/10.1007/s11858-018-01016-6
- Smale-Jacobse, A. E., Meijer, A., Helms-Lorenz, M., & Maulana, R. (2019). Differentiated instruction in secondary education: A systematic review of research evidence. Frontiers in Psychology, 10, Article 2366. https://doi.org/10.3389/fpsyg.2019.02366
- Timperley, H. (2008). Teacher professional learning and development (Educational Practices Series No. 18). UNESCO International Bureau of Education. http://www.ibe.unesco.org/sites/default/files/resources/edu-practices_18_eng.pdf
- van der Kleij, F. M., Vermeulen, J. A., Schildkamp, K., & Eggen, T. J. H. M. (2015). Integrating data-based decision making, Assessment for Learning and diagnostic testing in formative assessment. Assessment in Education: Principles, Policy & Practice, 22(3), 324–343. https://doi.org/10.1080/0969594X.2014.999024
- Veenman, M. V. J., van Hout-Wolters, B. H. A. M., & Afflerbach, P. (2006). Metacognition and learning: Conceptual and methodological considerations. Metacognition and Learning, 1(1), 3–14. https://doi.org/10.1007/s11409-006-6893-0
- What Works Clearinghouse. (2020). What Works Clearinghouse standards handbook, Version 4.1. U.S. Department of Education, Institute of Education Sciences, National Center for Education Evaluation and Regional Assistance. https://ies.ed.gov/ncee/wwc/handbooks
- Wiliam, D., & Leahy, S. (2015). Embedding formative assessment: Practical techniques for K–12 classrooms. Learning Sciences International.
- Winne, P. H. (2018). Cognition and metacognition within self-regulated learning. In D. H. Schunk & J. A. Greene (Eds.), Handbook of self-regulation of learning and performance (2nd ed., pp. 36–48). Routledge.