Abstract
Cognitive processes underlying a behavioural outcome (like reading ability) and the impact of familial risk (e.g., for dyslexia) have been studied in isolation. We present a novel design, linking the two avenues. How do familial influences impact on children’s cognitive skills, which subsequently underlie reading development? Participants from the Familial Influences on Literacy Abilities (FIOLA) Project included 373 children and their parents. We considered three causal routes from parental reading and children’s putative cognitive endophenotypes to children’s reading. Path analyses showed that half of parental effects on children’s reading bypassed and half operated through children’s cognitive underpinnings. Spousal correlation was small but significant. Findings do not support a strong hypothesis of cognitive endophenotypes with full mediation. Furthermore, we discuss the use of parental skills as a proxy for offspring’s liability. Finally, familial reading difficulties are not fully accounted for by known cognitive skills, which has implications for dyslexia diagnosis.
We can predict a child’s risk of dyslexia from two kinds of information: family history of reading problems and cognitive deficits in domains such as phonological awareness (PA), rapid naming (RAN), and visual attention span (VAS). What has remained unknown is the extent to which these cognitive deficits mediate familial risk. Here we address this question by considering relationships between data at three levels of analysis: parental phenotype, children’s cognitive skills, and children’s reading behaviour. Our approach is based on the intergenerational multiple deficit model (van Bergen, van der Leij, & de Jong, Citation2014), an extension of work by Pennington (Citation2006). According to this model, both parents pass on risk and protective factors via intertwined genetic and environmental pathways. These factors ultimately impact on behavioural disorders or traits via multiple intermediaries at the neural and cognitive levels.
It is increasingly realized that familial risk is a continuum and that both parents contribute to a child’s risk for dyslexia (Snowling, Gallagher, & Frith, Citation2003; van Bergen, van der Leij, et al., Citation2014). First, affected parents of affected children tend to be more severely dyslexic than those of unaffected children (Torppa, Eklund, van Bergen, & Lyytinen, Citation2011; van Bergen, de Jong, Plakas, Maassen, & van der Leij, Citation2012). Second, the literacy abilities of the spouse of the dyslexic parent add to the offspring’s liability (van Bergen, de Jong, Maassen, & van der Leij, Citation2014). However, studying parent–child resemblance for reading ability in a “classic” family-risk design is not ideal because of (a) a restriction-in-range problem when analysing the at-risk group, with the affected parents scoring all in the lower tail of the reading distribution, and (b) pooling across two different samples when analysing the at-risk and control groups together.
In the current study we looked at the whole range of reading ability in an unselected sample. This allowed us to move from a sole consideration of risk factors to look more broadly at familial effects including protective factors. Plomin’s group has shown that heritability is comparable for the lower tail (dyslexia) and the full range of the reading distribution (Harlaar, Spinath, Dale, & Plomin, Citation2005; Kovas, Haworth, Dale, & Plomin, Citation2007). This suggests that understanding the aetiology of individual differences across the range of an unselected population is likely to pertain to dyslexia as well.
We investigated three cognitive constructs in children as putative mediators of familial effects on children’s reading outcome: PA, RAN, and VAS. All three skills contribute to oral and silent reading (van den Boer, van Bergen, & de Jong, Citation2014) and are often impaired in children with dyslexia (Bosse, Tainturier, & Valdois, Citation2007; de Groot, van den Bos, Minnaert, & van der Meulen, Citation2015; de Jong & van der Leij, Citation2003). PA refers to the ability to identify and manipulate speech sounds (phonemes) in spoken words. In RAN the participant is required to name rapidly a matrix of stimuli that are repeated in random order. For VAS, participants are typically asked to report back a string of consonants (e.g., R H S D M) that is briefly flashed on a computer screen. VAS explains individual variation in reading ability, partly independently of phonological skills (Bosse et al., Citation2007). It is still debated whether the task mainly taps visual (Valdois, Lassus-Sangosse, & Lobier, Citation2012) or verbal skills (Ziegler, Pech-Georgel, Dufau, & Grainger, Citation2010). In this regard it is interesting to consider whether PA, RAN, and VAS have a similar mediational role in influencing parent–child relationships in reading skill.
Before considering how these cognitive skills mediate effects from parent to offspring, we first need to consider parent–child similarity for reading ability. Assuming the heritability to be comparable in both generations, the parent–offspring correlation would be half the heritability, because a parent passes on 50% of his or her genes. As genes account for roughly two thirds of individual differences in reading (Olson, Keenan, Byrne, & Samuelsson, Citation2014), parent–offspring correlation due to genetic transmission would be about .33 (= 2/3 × 1/2). Parent–child resemblance could, however, be increased by positive assortative mating (i.e., father–mother correlation), a shared environmental confound (an environmental factor influencing both generations), and cultural transmission (i.e., a direct environmental effect of parental reading ability and its associated traits on children’s reading ability). There is limited empirical evidence on parent–child similarity in reading: Two family-risk studies reported parent–offspring correlations (Snowling, Muter, & Carroll, Citation2007; van Bergen et al., Citation2012), but they suffer from the two issues mentioned earlier, that is, restriction-of-range and pooling. One study of an unselected sample by Wadsworth and colleagues (Citation2002) found parent–child correlations to be about .20. This is consistent with the rather low heritability in their sample of .49.
shows different routes linking reading ability across the generations and cognitive skills in the child. The first model, the additive factors model, treats parental reading ability and children’s cognitive skills as independent factors that additively impact on the child’s reading (Bishop, Hardiman, & Barry, Citation2012). Here, cognitive skills have a nonfamilial origin, like classroom effects. This model is unlikely given what we know about substantial familial effects on PA and RAN (Petrill et al., Citation2010; Willcutt et al., Citation2010). However, it could potentially apply to VAS, where nothing is yet known about familial effects.
FIGURE 1 Possible causal relationships between reading ability of parents on the one hand and reading-related cognitive skills and reading ability of children on the other.
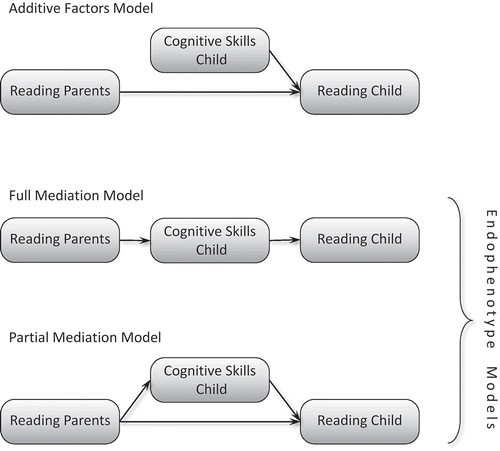
also shows two types of endophenotype model (Kendler & Neale, Citation2010). The full mediation model is a simple model in which the intergenerational association for reading ability is completely accounted for by children’s cognitive skills. Children’s cognitive skills are in this sense intermediate phenotypes, or cognitive endophenotypes, as they are thought to mediate between genetic variants and the ultimate phenotype (Moll, Loff, & Snowling, Citation2013). also shows a partial mediation model, where cognitive skills only partly mediate the parent–child reading resemblance. The additional direct effect from parent to child could arise if important underlying cognitive skills are left out or if reading ability has a unique aspect, not captured by any cognitive task.
We considered three related questions. First, are reading abilities of fathers and mothers associated (assortative mating) or not (random mating)? This is important because assortative mating underestimates heritability if not taken into account (Plomin, DeFries, McClearn, & McGuffin, Citation2008, p. 160). The spousal correlation for reading accuracy in Wadsworth et al.’s sample was .26. To our knowledge it has not been reported for other samples.
Second, does the parent–offspring correlation differ for fathers and mothers? van Bergen et al. (Citation2012) found that children’s reading ability was more strongly correlated with maternal reading ability. However, the one study based on an unselected sample (Wadsworth et al., Citation2002) found no difference. If all parents are truly biological parents, differences between the sexes could be due to differences in genetic mechanisms or cultural transmission (van Bergen, van der Leij, et al., Citation2014).
Third, how far does parental reading have its effect on the child’s reading via children’s cognitive skills? We tested parents on reading ability and their children on reading ability, PA, RAN, and VAS. We modelled the extent to which each of these cognitive abilities mediates between reading phenotypes in parent and child.
METHOD
Participants
Data were drawn from the Familial Influences on Literacy Abilities (FIOLA) Project. The FIOLA sample consists of families visiting the science museum in AmsterdamFootnote1 and willing to take part in the study with at least one parent and one child. Data collection took place during four school holidays over 2 consecutive years. In total, 1,235 people participated. Of those, 25 were excluded from the FIOLA data because they had not attended or did not currently attend Dutch mainstream education. For the current article, 18 family members were excluded because they did not fall in the biological parent or child category (e.g., grandparent or stepparent) and 11 “children” (age: M = 25.73, SD = 10.01) who participated with their parents were excluded because they were 17 or older, making the children’s age range too wide. If more than one child per family participated, the data of the oldest were selected (excluding 219 younger siblings) because this article focuses on father–mother–child triads. From the remaining 386 families, 13 were excluded because only parent data were present (excluding 7 fathers and 13 mothers). One more father and two mothers were excluded because one of the two reading test scores was missing (we used both scores for calculating a reading composite score). This yielded the final sample of 939 individuals from 373 families: 269 fathers (age: M = 43.64, SD = 4.24), 297 mothers (age: M = 42.26, SD = 3.97), and 373 children (age: M = 10.57, SD = 1.94, range = 6–16; 200 girls). All 373 children were at least in second grade and had completed at least 14 months of formal reading instruction (M = 48.21, SD = 19.36, range = 14–106). Of the 373 families, 200 had father–mother–child data, 69 father–child data, 97 mother–child data, and 7 had child data only. The latter children were included to help estimate relationships among child variables. Parent data were missing because of the exclusion reasons just given but mostly because parents did not participate. Of the nonparticipating parents, a minority did not want to participate and a majority was not in the museum. Parents who did and who did not participate did not differ on level of education, t(365) = −1.42, p = .156, for fathers and t(368) = 0.28, p = .780, for mothers.
We have two indicators of sample representativeness. First, level of education was rated on a scale ranging from 1 (primary school only) to 9 (PhD or equivalent). The average was 5.77 (SD = 1.93) for fathers and 5.52 (SD = 1.85) for mothers. Level of education was recoded to a 5-point scale, allowing comparison with data on the Dutch population of 35- to 55-year-olds (Statistics Netherlands, Citation2013). The FIOLA sample’s mean appeared to be 0.49 SD above average (see the appendix). The second indicator is children’s word reading level. For children attending Grade 2 to 6 (n = 321), national norms were available (M = 10, SD = 3), yielding a mean of 11.21 (SD = 3.49), which is 0.40 SD above average.
Measures
The FIOLA sample was assessed on several skills. Here we present data of parents’ and children’s reading fluency and children’s PA, RAN, and VAS.
Reading fluency
Fluency was tested for both words (Brus & Voeten, Citation1972) and pseudowords (van den Bos, Lutje Spelberg, Scheepstra, & De Vries, Citation1994). Parents and children were asked to correctly read as many (pseudo)words as possible within 1 min (word reading) or 2 min (pseudoword reading). The original test versions consist of a list of 116 (pseudo)words of increasing difficulty. To avoid a ceiling effect in adults, the lists were extended by adding the last column of its parallel test, resulting in a list of 145 (pseudo)words. Reliabilities for children of different ages range from .76 to .96 for word reading and from .89 to .95 for pseudoword reading (test manuals). To obtain reliability estimates for adults, we tested an independent sample (N = 50) on parallel forms of the reading tests. This yielded a correlation of .96 for word reading and .94 for pseudoword reading.
PA
PA was measured using our newly developed Amsterdam Phoneme Deletion Test. On each test item a phoneme (always a consonant) had to be deleted from a pseudoword, resulting in another pseudoword. The pseudowords were selected from the phoneme deletion test of de Jong and van der Leij (Citation2003). Twelve of 27 (four from each block) were selected, based on intermediate difficulty and good item–test correlation. For the current test, all items were digitally recorded. The item’s audio files were played from a laptop and presented via headphones. The child heard, for example, “tesp,” after which (s)he repeated the pseudoword back to the tester. This was repeated if the pseudoword was not pronounced correctly. Thereafter the child heard, “What is tesp without /p/?” [tes]. The to-be-deleted phoneme was presented as the letter sound, not letter name. The test consisted of two parts. The first part comprised four monosyllabic (Block 1) and four disyllabic pseudowords (Block 2). The second part consisted of four disyllabic pseudowords (Block 3), with the phoneme that had to be deleted occurring twice (e.g., “What is porsvust without /s/?” [porvut]). Both parts of the test started with two items (with feedback) for practice. The tester scored each test-item response as either correct or incorrect and measured the reaction time (RT). For measuring RTs, the timer started running after the audio file finished. The timer was stopped by the tester’s key press immediately after the child finished pronouncing the answer. The test was programmed and presented in Presentation® (Version 14.9, http://www.neurobs.com)
Cronbach’s alpha for the 12 accuracy scores is .71 and for the 12 RTs .89 (this sample). However, 15.4% scored at ceiling and RTs were skewed. To accommodate this and combine the two, we computed a fluency score. This score reflects the number of correct items per second, similarly to the number of correct items per 1/2 min in the reading fluency tests. The fluency score is based on the combination of accuracy and the total time required to finish all items. Accordingly, per participant all 12 RTs were used. A median RT for each of the three blocks of four items was calculated. These three median RTs were then averaged. The proportion of items correct was divided by this RT. The obtained fluency score reflects the number of phonological-awareness items correct per second.
Reliability was also calculated for an independent sample of 66 children ages 11;6 years (SD = 5 months) who attended Grade 5 (end) or Grade 6 (start). The Amsterdam Phoneme Deletion Test was administered twice with a 2-week interval. Test–retest reliabilities were .75 for accuracy, .74 for mean RT, and .81 for the fluency score.
RAN
RAN (van den Bos, Citation2003) was measured for digits (2, 4, 5, 8, and 9). Fifty randomly ordered digits arranged in five columns of 10 digits each. Children were instructed to name the digits column-wise as quickly as possible. The time to completion was transformed to number of digits per second to normalize the score distribution. The manual reports reliabilities ranging from .78 to .92 for children of different ages. In our independent sample of 66 children just mentioned, the test–retest reliability was .80.
VAS
The whole-report VAS task was administered (see Valdois et al., Citation2003). Participants were shortly presented with 10 five-letter strings (e.g., R H S D M) on a computer screen. Immediately after the presentation, they were asked to name as many letters as possible, in the correct order. Participants were asked to say “dot” if they missed a letter but had seen the letter(s) thereafter (e.g., R, H, S, dot, M), which would yield a score of 4. The strings were created from 10 consonants (B, D, F, H, L, M, P, R, S, and T), all presented once in each letter position. The task was programmed in E-prime version 1.0 (Schneider, Eschman, & Zuccolotto, Citation2002). To focus attention, a plus sign was presented in the middle of the screen for 1,000 ms. Subsequently, the letter string was flashed for 200 ms in the middle of the screen in bold 24-point black Arial font on a white background. The short presentation time prevents saccadic eye movements. The score consisted of the number of letters (from a total of 50) repeated correctly at the correct position. In our independent sample of 66 children mentioned previously, the test–retest reliability of the VAS scoring as used here was .88.
Procedure
All participants 18 years of age or older signed their own informed consent. Written informed consent of participants younger than 18 was given by parents. Ethical approval for this study was provided by the University of Amsterdam ethics committee, file number 2011-OWI-1882. Participants were tested individually by trained test assistants. Testing lasted 15 min and took place in the museum. The museum had two separate rooms dedicated to participant testing. The rooms had partial glass walls, so parents and children could see each other.
Data: Missingness and Preparation
VAS was administered only in the 2nd year. Children with (51%) and without VAS scores did not differ on reading ability, t(371) = −0.25, p = .80. PA was missing for 1% and RAN was complete. Before age corrections, the square root of VAS and PA was taken to equalize the variance across age. Subsequently, age and age-squared were regressed out of all child variables. This age correction was done on the full sample of children (N = 592), including siblings. Outliers (one for mothers’ and two for children’s word reading; two for RAN) were relocated to the tail of the distribution (± 3 SD). Distributions of all variables were close to normal. A reading composite was derived by summing z scores of word and pseudoword reading for fathers (r = .78), mothers (r = .64), and children (r = .83). All variables were standardized before analyses.
RESULTS
Descriptive statistics are provided in . For the VAS task the table gives the total score, which was used in subsequent analyses. The accuracy level at each of the five letter positions (from left to right) was almost at ceiling for the first two letters (showing that children were fine with reporting letters in itself) and then dropped. This pattern is very similar to that reported in previous studies using the global report version of the VAS task, showing that individual differences in VAS performance are mostly determined by differences in accuracy in letter positions three to five (Valdois et al., Citation2003; van den Boer, van Bergen, & de Jong, Citation2015).
TABLE 1 Descriptive Statistics
The correlations were estimated with the full information method, as implemented in Mplus version 6.11 (Muthén & Muthén, Citation2010), thereby taking the missingness of data into account. Children’s reading ability correlated .38 and .32 with father’s and mother’s reading ability, respectively, whereas the latter two—indexing assortative mating—correlated weakly (.16). Furthermore, children’s reading ability correlated .51 to .63 with their cognitive skills.
As the child sample draws from a wide age range, we inspected whether parent–child correlations differed across age. To this end, we split the sample in families with younger (6–10 years) and older children (11–16 years). As shown in , correlations did not differ significantly (ps > .22). Hence, age effects were not considered further.
TABLE 2 Parent–Child Correlations for Two Age Bands
To evaluate the sources of explained variance in the final structural equation model, we started with two separate multiple regression models (in Mplus) predicting children’s reading ability. On their own, fathers’ and mothers’ reading ability (in one step) accounted for 21.1% of the variance. In a second model, the three cognitive skills alone (in one step) accounted for 55.5%.
Given that children’s cognitive skills were not independent but correlated with parental reading, it could be concluded that the additive factors model in was not going to fit, as hypothesized. In the remaining models children’s cognitive correlates either fully or partially mediated the risk transmitted from parent to child.
Mediation was formally examined using structural equation modeling. Model fit was evaluated using chi-square, root mean square error of approximation (RMSEA), and comparative fit index (CFI). Close fit is indicated by an insignificant chi-square, RMSEA < .05, and CFI > .95. The difference in fit between two nested models was evaluated with the chi-square-difference test.
First, the baseline model was fit to the data, which is saturated (df = 0) and forms the starting point for hypothesis testing. This model is depicted in . The first question tested was whether children’s cognitive correlates fully mediate the risk passed on from parent to child. To this end, the direct path from father’s to children’s reading ability was dropped. This resulted in a significant drop in model fit, Δχ2(1) = 11.55, p < .001. Similarly, dropping the direct path from mother’s reading ability significantly deteriorated model fit compared to baseline, Δχ2(1) = 11.99, p < .001. Hence, these direct paths were needed, and so effects from parent to child reading were not fully mediated, as the full mediation model states.
FIGURE 2 Saturated structural equation model with standardized parameter estimates. Note. All parameter estimates are significant (ps < .03) except for VAS on mother’s reading. Correlations between unexplained variances in cognitive skills are omitted from the figure; values are PA-RAN .34, PA-VAS .37, RAN-VAS .26. Reading = word-level reading fluency; PA = phonological awareness; RAN = rapid naming; VAS = visual attention span.
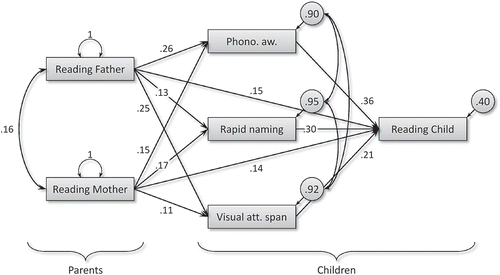
The second question was whether the effects of fathers and mothers are similar. In a series of models, equality constraints were imposed on analogous paths originating from both parents to reading child, PA, RAN, and VAS, respectively (see ). The fit of each of those four models was not significantly worse than the baseline model (ps > .22). Accordingly, the paths going from parents to the other variables did not differ significantly between the parents. Finally, a model was fitted including all four equality constraints. This final model (a partial mediation model) is depicted in and showed an excellent fit to the data: χ2(4, N = 373) = 3.25, p = .52; RMSEA < . 001, 90% confidence interval [.000, .071]; CFI = 1.000. All path estimates were significant (ps > .001).
FIGURE 3 Final structural equation model with standardized parameter estimates. Note. Arrows intersected by equal shapes are constrained to be equal. All path estimates have p values < .001; the parents’ reading correlation has p = .019. Correlations between unexplained variances in cognitive skills are omitted from the figure; values are: PA-RAN .33, PA-VAS .38, RAN-VAS .26. The model fit is χ2(4) = 3.25, p = .52; RMSEA < .001; N = 373 families (939 individuals). Reading = word-level reading fluency; PA = phonological awareness; RAN = rapid naming; VAS = visual attention span.
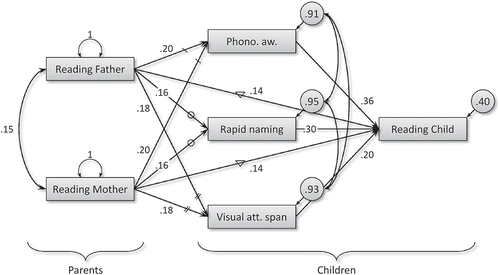
Apart from the two mentioned conclusions on partial mediation and equal parental effects, some other findings are noteworthy. The model explained 60% of the variance in children’s reading ability. Variance in children’s cognitive skills remained largely unexplained by parental reading skills. Furthermore, assortative mating was estimated at .15. The total effect of each of the parents’ reading ability to children’s reading ability was .30 (R2 = .302 = 9%), of which .14 was direct and .16 was indirect via the three underlying skills (i.e., .20 × .36 + .16 × .30 + .18 × .20 = .16).
DISCUSSION
Our work demonstrates that familial effects, as indicated by parental reading ability, influence children’s reading outcome partly via children’s cognitive intermediaries and partly directly. Assortative mating was low but significant, and reading ability of fathers and mothers had similar impact on children’s reading.
It should be noted that familial influences in the current study could reflect cultural transmission as well as genetic influences. A genetically sensitive family study could disentangle these effects (D’Onofrio, Lahey, Turkheimer, & Lichtenstein, Citation2013). Wadsworth et al. (Citation2002) showed that in adoptive families, parent–offspring resemblance for reading ability was absent. This is a direct estimate of cultural transmission. This suggests that in our FIOLA study, the observed parent–offspring correlation in biological families is largely due to genetic transmission. Indeed, the observed parent–offspring correlation for reading skills is in line with what we expected based on solely genetic transmission.
In the current study parents’ reading skills explained 21% of the variance in child reading. This is strikingly high relative to estimates of genetic effects from molecular genetic studies, where the largest effect of a single genetic variant on reading ability explains only 0.4% of individual differences (Luciano et al., Citation2013). The effect of all genetic variants associated with a phenotype can be combined in a polygenic score, but to date use of a polygenic score can account for only 2.5% of the variance in reading ability (de Zeeuw et al., Citation2014). There is evidence that genetic effects involve interactive as well as additive influences of many genes of small effect, meaning that genetic information would not be useful for predicting individual outcomes (Bishop, Citation2015). For heritable traits, at present parental abilities best index genetic liability.
We had anticipated that PA, RAN, and VAS might show different patterns of association with parental and child reading. However, the paths from parental reading to PA, RAN, and VAS were similar in magnitude, and although all captured unique variance in reading, their variances also partly overlapped, possibly due to shared phonological demands (van den Boer et al., Citation2014). For VAS this study provides the first piece of evidence that it is influenced by familial factors.
A complete-mediation model did not fit the data. It is possible that other, unmeasured cognitive endophenotypes would have accounted for additional variance. Nevertheless, it may be that all (reliable) variance in reading performance will never be completely captured by any set of cognitive skills. Explained variance would also have increased by using multiple indicators per cognitive construct (Protopapas, Citation2013). However, our set of tasks already explained more than half of the reading variance, and we do not expect a huge increase with multiple indicators as the reliability of our measures was high. More important, it is unlikely that the use of multiple indicators would have changed the balance between direct and indirect effects of parental on children’s reading skills.
Another concern with cognitive endophenotypes is that it is questionable whether they are indeed biologically less complex than the disorder itself (or its corresponding quantitative trait, like dyslexia vs. word-reading ability). To advance gene-finding studies, it was hoped to find genetically influenced traits that mediate between genetic susceptibility and disorder, which are themselves “closer to the DNA level” (Flint & Munafò, Citation2007; Kendler & Neale, Citation2010). From a molecular genetic perspective, PA, RAN, and VAS may not prove any less complex than word-reading itself. In that sense, they may be seen as phenotypes themselves.
What do the direct effects from parental reading to child reading tell us? Despite the substantial impact of children’s underlying skills on reading, parental skills explained additional variance (previously also found by Torppa et al., Citation2011; van Bergen et al., Citation2012; in family-risk studies). In fact, half of parents’ effects bypassed and half operated through children’s underlying skills. Longitudinal twin studies have shown that genetic influences on PA and RAN before learning to read partly overlap with genetic influences on later reading acquisition (Christopher et al., in press). The corollary is that reading is genetically partly distinct from PA and RAN. These new genetic influences kick in after the start of formal reading instruction (Byrne et al., Citation2009). A partly unique aetiology of reading contradicts a strong endophenotype hypothesis with complete mediation.
Our models assumed that correlations between cognitive skills and reading outcomes in children reflected a causal link from cognition to reading, but it is possible that some causal influence goes in the opposite direction. Alternatively, skills could mutually influence each other. Bidirectional influences between a cognitive correlate and reading acquisition have been shown for PA (Perfetti, Beck, Bell, & Hughes, Citation1987) but not for RAN (Lervåg & Hulme, Citation2009). Reciprocality still has to be studied for VAS. Determining the direction of causality within children’s traits would require longitudinal data. Nevertheless, inclusion of parental data in the model does constrain causal pathways to some extent: The direction of the arrow from parent to child skills is not ambiguous.
Our findings have general implications for educational policy, as they inform the debate about whether to include reading-related cognitive skills in the diagnosis of dyslexia (see Tannock, Citation2013). In dyslexia assessments it is common practice to review PA and RAN levels alongside literacy achievements. A group of 58 American scholars advocate the use of “processing weaknesses” in diagnosing dyslexia (Hale et al., Citation2010). In the Netherlands impairments in PA, RAN, or letter-sound integration is even a requirement for a diagnosis and government-funded treatment (Blomert, Citation2006). However, deficits in say PA or RAN are neither necessary nor sufficient for dyslexia (Pennington et al., Citation2012). The current study adds that familial reading difficulties are not totally accounted for by these underlying cognitive skills. The direct familial effect is not large at the group level but may be large for a particular child, who may well exhibit deficiencies in unmeasured cognitive processes. Our results therefore challenge the notion that deficits in PA and/or RAN should be requirements for a dyslexia diagnosis.
This is not to say that specifying a child’s cognitive profile serves no purpose. First, in prereading children it can help identify children at high risk of developing dyslexia, especially if combined with letter knowledge and family-risk status (Heath et al., Citation2014; van Bergen, de Jong, et al., Citation2014). Second, deficits in cognitive correlates of reading give a diagnostician more confidence in a dyslexia diagnosis (Pennington et al., Citation2012). Lack of such deficits combined with very poor reading might request further investigation but ought not to prevent a diagnosis and access to treatment (de Jong, Citation2014). Third, cognitive profiles would be useful if we would have evidence that profile-specific treatments are most effective. Strong evidence for this is currently lacking; most interventions combine training of several components of the reading system (de Jong, Citation2014).
The current study is an empirical demonstration based on the intergenerational multiple deficit model (van Bergen, van der Leij, et al., Citation2014). We showed that familial effects on children’s reading are nonexclusively mediated by cognitive underpinnings. Studying cognitive underpinnings of a behavioural outcome by employing parents’ characteristics is a novel approach. This approach can be applied to other traits and can be extended by including more outcomes, more time points, or more putative mediators, like environmental factors and brain measures.
ACKNOWLEDGMENTS
E. van Bergen, T. van Zuijen, and P.F. de Jong developed the FIOLA Project and E. van Bergen, D. Bishop, and P.F. de Jong this article’s study design. Testing and data collection were performed under the direction of E. van Bergen and T. van Zuijen. E. van Bergen and P.F. de Jong performed the data analyses. E. van Bergen drafted the manuscript, and P.F. de Jong and D. Bishop provided critical revisions. All authors approved the final version of the manuscript for submission. We are grateful to all participating families and to NEMO.
FUNDING
EvB is supported by a Rubicon Fellowship (grant no. 446-12-005) from the Netherlands Organisation for Scientific Research (NWO) and a Junior Research Fellowship at Oriel College, Oxford. DB is supported by a Wellcome Trust Principal Research Fellowship (grant no. 082498/Z/07/Z). TvZ is supported by the Dutch Dyslexia Programme (NWO grant no. 200-62-304). PdJ is supported by the Institute of Child Development and Education of the University of Amsterdam.
Additional information
Funding
Notes
1 This research is part of Science Live, the innovative research programme of Science Museum NEMO that enables scientists to carry out real, publishable, peer-reviewed research using NEMO visitors as volunteers.
REFERENCES
- Bishop, D. V. M. (2015). The interface between genetics and psychology: Lessons from developmental dyslexia. Proceedings of the Royal Society B: Biological Sciences, 282. doi:10.1098/rspb.2014.3139
- Bishop, D. V. M., Hardiman, M. J., & Barry, J. G. (2012). Auditory deficit as a consequence rather than endophenotype of specific language impairment: Electrophysiological evidence. PloS One, 7(5), e35851.
- Blomert, L. (2006). Protocol dyslexie diagnostiek en behandeling [Protocol for diagnosing and treating dyslexia]. Maastricht, The Netherlands: Universiteit Maastricht.
- Bosse, M., Tainturier, M. J., & Valdois, S. (2007). Developmental dyslexia: The visual attention span deficit hypothesis. Cognition, 104(2), 198–230. doi:10.1016/j.cognition.2006.05.009
- Brus, B. T., & Voeten, M. J. M. (1972). Eén-minuut-test [One-minute-test]. Lisse, The Netherlands: Swets & Zeitlinger.
- Byrne, B., Coventry, W. L., Olson, R. K., Samuelsson, S., Corley, R., Willcutt, E. G., … DeFries, J. C. (2009). Genetic and environmental influences on aspects of literacy and language in early childhood: Continuity and change from preschool to grade 2. Journal of Neurolinguistics, 22(3), 219–236. doi:10.1016/j.jneuroling.2008.09.003
- Christopher, M. E., Hulslander, J., Byrne, B., Samuelsson, S., Keenan, J. M., Pennington, B., … Olson, R. K. (2015). Genetic and environmental etiologies of the longitudinal relations between pre-reading skills and reading. Child Development, 86(2), 342–361. doi:10.1111/cdev.12295
- D’Onofrio, B. M., Lahey, B. B., Turkheimer, E., & Lichtenstein, P. (2013). Critical need for family-based, quasi-experimental designs in integrating genetic and social science research. American Journal of Public Health, 103(S1), S46–S55. doi:10.2105/AJPH.2013.301252
- de Groot, B. J. A., van den Bos, K. P., Minnaert, A. E. M. G., & van der Meulen, B. F. (2015). Phonological processing and word reading in typically developing and reading disabled children: Severity matters. Scientific Studies of Reading, 19(2), 166–181. doi:10.1080/10888438.2014.973028
- de Jong, P. F. (2014). Verklaringen van dyslexie: Implicaties voor de samenstelling van een dyslexie typerend profiel [Explanations of dyslexia: Implications for the composition of a typical dyslexia profile]. In L. Verhoeven, P. F. de Jong, & F. Wijnen (Eds.), Dyslexie 2.0: Update van het protocol dyslexie diagnostiek en behandeling [Dyslexia 2.0: Update of the protocol diagnosis and treatment of dyslexia] (pp. 37–53). Antwerp, Belgium: Garant.
- de Jong, P. F., & van der Leij, A. (2003). Developmental changes in the manifestation of a phonological deficit in dyslexic children learning to read a regular orthography. Journal of Educational Psychology, 95(1), 22–40. doi:10.1037/0022-0663.95.1.22
- de Zeeuw, E., van Beijsterveldt, C. E. M., Glasner, T., Ehli, E. A., Davies, G. E., … Boomsma, D. I. (2014). Polygenic scores associated with educational attainment in adults predict educational achievement and ADHD symptoms in children. American Journal of Medical Genetics Part B: Neuropsychiatric Genetics, 165(6), 510–520. doi:10.1002/ajmg.b.32254
- Flint, J., & Munafò, M. R. (2007). The endophenotype concept in psychiatric genetics. Psychological Medicine, 37(02), 163–180.
- Hale, J., Alfonso, V., Berninger, V., Bracken, B., Christo, C., Clark, E., & Yalof, J. (2010). Critical issues in response-to-intervention, comprehensive evaluation, and specific learning disabilities identification and intervention: An expert white paper consensus. Learning Disability Quarterly, 33(3), 223–236. doi:10.1177/073194871003300310
- Harlaar, N., Spinath, F. M., Dale, P. S., & Plomin, R. (2005). Genetic influences on early word recognition abilities and disabilities: A study of 7 year old twins. Journal of Child Psychology and Psychiatry, 46(4), 373–384. doi:10.1111/jcpp.2005.46.issue-4
- Heath, S. M., Bishop, D. V., Bloor, K. E., Boyle, G. L., Fletcher, J., Hogben, J. H., & Yeong, S. H. (2014). A spotlight on preschool: The influence of family factors on children’s early literacy skills. PloS One, 9(4), e95255.
- Kendler, K., & Neale, M. (2010). Endophenotype: A conceptual analysis. Molecular Psychiatry, 15(8), 789–797. doi:10.1038/mp.2010.8
- Kovas, Y., Haworth, C. M. A., Dale, P. S., & Plomin, R. (2007). The genetic and environmental origins of learning abilities and disabilities in the early school years. Monographs of the Society for Research in Child Development, 72(3), 1–160. doi:10.1111/j.1540-5834.2007.00453.x
- Lervåg, A., & Hulme, C. (2009). Rapid automatized naming (RAN) taps a mechanism that places constraints on the development of early reading fluency. Psychological Science, 20(8), 1040–1048. doi:10.1111/j.1467-9280.2009.02405.x
- Luciano, M., Evans, D., Hansell, N., Medland, S., Montgomery, G., Martin, N., … Bates, T. C. (2013). A genome wide association study for reading and language abilities in two population cohorts. Genes, Brain and Behavior, 12(6), 645–652. doi:10.1111/gbb.12053
- Moll, K., Loff, A., & Snowling, M. J. (2013). Cognitive endophenotypes of dyslexia. Scientific Studies of Reading, 17(6), 385–397. doi:10.1080/10888438.2012.736439
- Muthén, L. K., & Muthén, B. O. (2010). Mplus software ( version 6.11 ed.). Los Angelos, CA: Muthén & Muthén.
- Olson, R. K., Keenan, J. M., Byrne, B., & Samuelsson, S. (2014). Why do children differ in their development of reading and related skills? Scientific Studies of Reading, 18(1), 38–54. doi:10.1080/10888438.2013.800521
- Pennington, B. F. (2006). From single to multiple deficit models of developmental disorders. Cognition, 101(2), 385–413. doi:10.1016/j.cognition.2006.04.008
- Pennington, B. F., Santerre–Lemmon, L., Rosenberg, J., MacDonald, B., Boada, R., Friend, A., … Olson, R. K. (2012). Individual prediction of dyslexia by single versus multiple deficit models. Journal of Abnormal Psychology, 121(1), 212–224. doi:10.1037/a0025823
- Perfetti, C. A., Beck, I., Bell, L. C., & Hughes, C. (1987). Phonemic knowledge and learning to read are reciprocal: A longitudinal study of first grade children. Merrill-Palmer Quarterly, 33(3), 283–319.
- Petrill, S. A., Hart, S. A., Harlaar, N., Logan, J., Justice, L. M., Schatschneider, C., … Cutting, L. (2010). Genetic and environmental influences on the growth of early reading skills. Journal of Child Psychology and Psychiatry, 51(6), 660–667. doi:10.1111/j.1469-7610.2009.02204.x
- Plomin, R., DeFries, J. C., McClearn, G. E., & McGuffin, P. (2008). Behavioral genetics (5th ed.). New York, NY: Worth Publishers.
- Protopapas, A. (2013). From temporal processing to developmental language disorders: Mind the gap. Philosophical Transactions of the Royal Society of London.Series B, Biological Sciences, 369(1634), 20130090. doi:10.1098/rstb.2013.0090
- Schneider, W., Eschman, A., & Zuccolotto, A. (2002). E-prime computer software (version 1.0). Pittsburgh, PA: Psychology Software Tools.
- Snowling, M. J., Gallagher, A. M., & Frith, U. (2003). Family risk of dyslexia is continuous: Individual differences in the precursors of reading skill. Child Development, 74(2), 358–373. doi:10.1111/cdev.2003.74.issue-2
- Snowling, M. J., Muter, V., & Carroll, J. (2007). Children at family risk of dyslexia: A follow-up in early adolescence. Journal of Child Psychology and Psychiatry, 48(6), 609–618. doi:10.1111/j.1469-7610.2006.01725.x
- Statistics Netherlands. (2013). Beroepsbevolking; behaalde onderwijs [Population’s educational achievement]. The Hague, The Netherlands: CBS Statistics Netherlands.
- Tannock, R. (2013). Rethinking ADHD and LD in DSM-5: Proposed changes in diagnostic criteria. Journal of Learning Disabilities, 46(1), 5–25. doi:10.1177/0022219412464341
- Torppa, M., Eklund, K., van Bergen, E., & Lyytinen, H. (2011). Parental literacy predicts children’s literacy: A longitudinal family-risk study. Dyslexia, 17(4), 339–355. doi:10.1002/dys.437
- Valdois, S., Bosse, M., Ans, B., Carbonnel, S., Zorman, M., David, D., & Pellat, J. (2003). Phonological and visual processing deficits can dissociate in developmental dyslexia: Evidence from two case studies. Reading and Writing, 16(6), 541–572. doi:10.1023/A:1025501406971
- Valdois, S., Lassus-Sangosse, D., & Lobier, M. (2012). Impaired letter-string processing in developmental dyslexia: What visual-to-phonology code mapping disorder? Dyslexia, 18(2), 77–93. doi:10.1002/dys.1437
- van Bergen, E., de Jong, P. F., Maassen, B., & van der Leij, A. (2014). The effect of parents’ literacy skills and children’s preliteracy skills on the risk of dyslexia. Journal of Abnormal Child Psychology, 42(7), 1187–1200. doi:10.1007/s10802-014-9858-9
- van Bergen, E., de Jong, P. F., Plakas, A., Maassen, B., & van der Leij, A. (2012). Child and parental literacy levels within families with a history of dyslexia. Journal of Child Psychology and Psychiatry, 53(1), 28–36. doi:10.1111/j.1469-7610.2011.02418.x
- van Bergen, E., van der Leij, A., & de Jong, P. F. (2014). The intergenerational multiple deficit model and the case of dyslexia. Frontiers in Human Neuroscience, 8(346), 1–13. doi:10.3389/fnhum.2014.00346
- van den Boer, M., van Bergen, E., & de Jong, P. F. (2014). Underlying skills of oral and silent reading. Journal of Experimental Child Psychology, 128, 138–151. doi:10.1016/j.jecp.2014.07.008
- van den Boer, M., van Bergen, E., & de Jong, P. F. (2015). The specific relation of visual attention span with reading and spelling in dutch. Learning and Individual Differences, 39, 141–149. doi:10.1016/j.lindif.2015.03.017
- van den Bos, K. P. (2003). Serieel benoemen en woorden lezen [Serial naming and word reading]. Groningen, The Netherlands: Rijksunversiteit Groningen.
- van den Bos, K. P., Lutje Spelberg, H. C., Scheepstra, A. J. M., & De Vries, J. R. (1994). De klepel: Een test voor de leesvaardigheid van pseudowoorden [The klepel: A test for the reading skills of pseudowords]. Lisse, The Netherlands: Swets & Zeitlinger.
- Wadsworth, S., Corley, R., Hewitt, J., Plomin, R., & DeFries, J. (2002). Parent–offspring resemblance for reading performance at 7, 12 and 16 years of age in the colorado adoption project. Journal of Child Psychology and Psychiatry, 43(6), 769–774. doi:10.1111/1469-7610.00085
- Willcutt, E. G., Betjemann, R. S., McGrath, L. M., Chhabildas, N. A., Olson, R. K., DeFries, J. C., & Pennington, B. F. (2010). Etiology and neuropsychology of comorbidity between RD and ADHD: The case for multiple-deficit models. Cortex, 46(10), 1345–1361. doi:10.1016/j.cortex.2010.06.009
- Ziegler, J. C., Pech-Georgel, C., Dufau, S., & Grainger, J. (2010). Rapid processing of letters, digits and symbols: What purely visual-attentional deficit in developmental dyslexia? Developmental Science, 13(4), F8–F14. doi:10.1111/j.1467-7687.2010.00983.x
APPENDIX
TABLE A1 Level of Education on a 5-Point Scale, Including Percentages for the FIOLA Sample and the Dutch Population of 35- to 55-Year-Olds