ABSTRACT
Using classical and quantile regression analyses, we investigated whether predictor variables for early reading comprehension differed depending on language background and ability level in a mixed group of 161 monolingual (L1) and bilingual (L2) children in second grade (6–8 years). Although L2 readers scored lower on oral language skills and reading comprehension, the prediction of reading comprehension was similar for L1 and L2 readers. Classical regression identified decoding, vocabulary, and morphosyntactic knowledge as unique predictors with no interaction with language background. Quantile regression demonstrated that the prediction of reading comprehension differed across ability levels; decoding and morphosyntactic knowledge were consistently unique predictors, but vocabulary was uniquely related only for poor comprehenders and working memory only for good comprehenders. In both types of analysis, language background did not explain unique variance, indicating that individual differences in the predictor variables explained the L1–L2 performance gap in early reading comprehension across the ability range.
Given its utmost societal relevance, it is important to gain insight into the factors that predict reading comprehension performance. Reading comprehension has been associated with linguistic diversity, with second language (L2) readers generally falling behind their native language (L1) peers (Melby-Lervåg & Lervåg, Citation2014), although there may be L2 readers who do not struggle with reading comprehension and L1 readers who do. In studies investigating the factors accounting for variation in reading comprehension, children usually have been split up into L1 and L2 readers. Mixed results have been reported; some studies have shown that oral language skills are more important for L2 readers compared to L1 readers (Babayiğit, Citation2014; Droop & Verhoeven, Citation2003; Geva & Farnia, Citation2012; Lervåg & Aukrust, Citation2010), whereas other studies have found no differences (Lesaux, Lipka, & Siegel, Citation2006; Verhoeven & Van Leeuwe, Citation2012). These studies, however, did not attempt to disentangle language background from ability level when investigating the explanatory factors of reading comprehension. Furthermore, most of the studies were focused on upper primary grades, whereas comprehension difficulties can already be observed in the early stages of reading acquisition (Verhoeven, Citation2000; Verhoeven, Voeten, & Vermeer, Citation2018). In the present study, we therefore combined classical and quantile regression techniques to gain insight into individual variation in early reading comprehension performance in a mixed group of L1 and L2 readers. First, we used classical regression analysis to investigate whether key predictors of reading comprehension (determined on the basis of prominent theories) differed depending on children’s language background (L1 vs. L2). As a next step, we used quantile regression analysis to investigate whether the key predictors differed depending on children’s ability level (poor vs. average vs. good). Together, these two complementary approaches shed light on the role of linguistic diversity in the prediction of early reading comprehension across ability levels.
Individual variation in reading comprehension
Reading comprehension is a complex activity involving a range of cognitive and linguistic factors (Stanovich, Citation1980). According to the Simple View of Reading (Gough & Tunmer, Citation1986; Hoover & Gough, Citation1990), there are two central components of reading comprehension: word reading skills and language comprehension. More recently, Perfetti and Stafura (Citation2014) aimed to capture the complexity of reading comprehension in the Reading Systems Framework. According to this theory, three knowledge sources (i.e., linguistic, orthographic, and general knowledge) are involved in the reading process, which takes place within a cognitive system with limited processing resources. The Reading Systems Framework can be used to identify pressure points (i.e., candidates for a cause of reading comprehension difficulty) in the reading system. The Lexical Quality Hypothesis (Perfetti, Citation2007) assumes that one of those pressure points is the lexicon, which can be defined as knowledge of written word forms and word meanings, often referred to as vocabulary knowledge. The lexicon connects the word identification system with the comprehension system. First, linguistic and orthographic information is needed for rapid word identification (i.e., word decoding). Then, the comprehension system requires input from the word identification system to build meaning units. For integrating information at the sentence level, morphology (i.e., rules about the structure of words) and syntax (i.e., rules about the structure of sentences) play an important role (Perfetti & Stafura, Citation2014), also called morphosyntactic knowledge. Furthermore, the cognitive capacities that underlie the process of reading are crucial. Working memory capacity (i.e., temporary storage and manipulation of information; Baddeley, Citation2003) is required for higher level comprehension processes, such as integrating information, inference making, and comprehension monitoring (Kendeou, Van Den Broek, Helder, & Karlsson, Citation2014; Oakhill, Hartt, & Samols, Citation2005).
Based on these three central theories, we have defined four factors as key predictors of reading comprehension for the present study: decoding skills, vocabulary knowledge, morphosyntactic knowledge, and working memory skills. Empirical studies have shown that word decoding skills and vocabulary knowledge predict reading comprehension, particularly in the early stages (Cain, Citation2010; Catts, Hogan, & Adlof, Citation2005). Furthermore, morphosyntactic knowledge (Muter, Hulme, Snowling, & Stevenson, Citation2004) and working memory also explain unique variance in young children’s reading comprehension (Cain, Oakhill, & Bryant, Citation2004; Seigneuric, Ehrlich, Oakhill, & Yuill, Citation2000). However, these studies included only monolingual children; little is known about early reading comprehension in L2 readers, despite the detection of a performance gap between L1 and L2 readers in the early stages of reading development (Verhoeven, Citation2000; Verhoeven et al., Citation2018).
Reading comprehension in L2 learners
At the start of primary education, L2 readers are confronted with the challenging task of learning to read in a language that is not their native language, which often results in falling behind in reading comprehension (Melby-Lervåg & Lervåg, Citation2014). In relation to the four key predictors, previous studies have provided some relevant insights into reading comprehension in L2 readers. Their comprehension difficulties are usually not a consequence of poor decoding skills; L2 readers’ decoding skills are found to be in the average range (Geva & Zadeh, Citation2006; Mancilla-Martinez & Lesaux, Citation2010), and they develop similar to L1 readers’ decoding skills (Verhoeven, Citation2000). The main source of their comprehension difficulties seems to be related to their oral language skills; L2 readers have lower vocabulary knowledge than L1 readers, and their morphosyntactic knowledge also falls behind (Melby-Lervåg & Lervåg, Citation2014). Some studies have shown that oral language skills are even more important factors for L2 reading comprehension (Babayiğit, Citation2014; Droop & Verhoeven, Citation2003; Geva & Farnia, Citation2012; Lervåg & Aukrust, Citation2010), whereas other studies report that those skills are equally important for L1 and L2 readers (Lesaux et al., Citation2006; Verhoeven & Van Leeuwe, Citation2012). Regarding their cognitive capacities, there is no reason to assume that L2 readers’ working memory capacity limits their reading comprehension. Although the results are mixed, recent findings have even suggested that bilinguals have larger working memory capacity than monolinguals because of experience with dual language management (Grundy & Timmer, Citation2017). This advantage has mostly been observed in bilingual children (Blom, Küntay, Messer, Verhagen, & Leseman, Citation2014; Morales, Calvo, & Bialystok, Citation2013) and might enable them to compensate for their limits in oral language skills during comprehension processes.
Reading comprehension across the distribution
To gain more insight into children’s reading comprehension difficulties, comparisons between good and poor comprehenders have often been made (e.g., Cain & Oakhill, Citation2006; Lesaux et al., Citation2006; Nation, Cocksey, Taylor, & Bishop, Citation2010). Those studies included primarily monolingual children who were split up into two subgroups. However, by dichotomizing a continuous variable, such as reading comprehension, information about the distribution of scores gets lost, resulting in a loss of power. The one study that did include children from diverse linguistic backgrounds (Lesaux et al., Citation2006) used percentile scores to classify children as poor or good comprehenders, resulting in a limited sample size and large discrepancies. Quantile regression is an analysis technique recently applied to reading research that does not require the sample to be split up but still allows for an investigation of (the strength of) the relation between predictor variables and the outcome variable across different points in the distribution of scores, so-called quantiles (Petscher & Logan, Citation2014).
By using a quantile regression approach, recent studies have revealed that explanatory factors can vary across the range of word reading ability (McIlraith & LRRC, Citation2016) and reading comprehension ability (Hua & Keenan; Citation2017; LRRC & Logan, Citation2017; Mancilla-Martinez & Lesaux, Citation2017; Tighe & Schatschneider, Citation2016). Hua and Keenan (Citation2017) showed that for several reading comprehension tests, the contribution of word decoding skills and listening comprehension varied as a function of reading comprehension ability in younger and older readers (8–18 years). Furthermore, a study with third-grade children and a broader range of predictor variables (Logan, Citation2017) showed that decoding skills were related to reading comprehension for poor and average comprehenders but not for good comprehenders, whereas vocabulary and morphosyntactic knowledge showed a similar relation across ability levels. It was concluded that quantile regression is a useful approach for the study of reading in both typical and atypical readers. With respect to the latter, there is one quantile regression study with only L2 readers showing that the relation between early language skills and later reading comprehension outcomes was stronger for L2 readers with higher ability levels (Mancilla-Martinez & Lesaux, Citation2017).
To our knowledge, there are no studies to date in which quantile regression analysis is used with a mixed group of L1 and L2 readers. Previous research has shown that L1 and L2 readers differ not only in reading comprehension ability but also in oral language skills (Melby-Lervåg & Lervåg, Citation2014), and mixed results have been reported about whether the relation between oral language skills and reading comprehension differs for L1 and L2 readers (Babayiğit, Citation2014; Droop & Verhoeven, Citation2003; Geva & Farnia, Citation2012; Lervåg & Aukrust, Citation2010; Lesaux et al., Citation2006; Verhoeven & Van Leeuwe, Citation2012). Instead of investigating whether the influence of key predictors differs because of children’s language background, quantile regression can be used to investigate whether the relation between key predictors and reading comprehension differs depending on children’s ability level. Children’s scores are placed along a continuum of reading comprehension ability regardless of their language background to do justice to individual variation in performance. Of particular interest are children at the lower end of the distribution, who should be considered for extra support. With quantile regression we can identify the key predictors for this particular group, which is essential for the identification and subsequent remediation of reading comprehension problems.
The present study
In the present study, we investigated the factors that explain individual variation in early reading comprehension performance in a mixed group of L1 and L2 readers of Dutch. Based on a variety of prominent theories, we defined decoding skills, vocabulary knowledge, morphosyntactic knowledge, and working memory skills as key predictors of reading comprehension. In contrast to most previous studies, we focused on beginning readers (second grade) based on the conviction that early intervention in reading comprehension problems is crucial for later school success. We applied two complementary approaches to answer our research questions. First, with classical regression, we investigated whether key predictors of reading comprehension differed depending on children’s language background (L1 vs. L2). Second, with quantile regression, we investigated whether key predictors of reading comprehension differed depending on children’s ability level (poor vs. average vs. good). In both types of analysis, we investigated whether language background explained unique variance over and above the key predictors. This allowed us to examine the role of linguistic diversity in the prediction of early reading comprehension performance across ability levels.
Regarding the first approach, we expected similar key predictors of reading comprehension for L1 and L2 readers, although we expected L2 readers to fall behind their L1 peers in terms of oral language skills and reading comprehension performance. We expected our second approach to be more relevant by showing that the relations between key predictors and reading comprehension vary across ability levels, as found in previous studies with monolingual or bilingual children. For children with lower ability levels, the relation with oral language skills was expected to be stronger; delays in vocabulary and morphosyntactic knowledge will hinder their comprehension processes. If differences in oral language skills are not sufficient to explain the L1–L2 performance gap in reading comprehension, this is most likely to be true at the lower ability level.
Method
Participants
The participants were 161 second-grade students (M age = 7.5 years, range = 81–105 months) from 13 primary schools in the Netherlands located in lower middle-class neighborhoods in urban areas. Prior to the study, ethical approval was obtained from our institution’s ethics committee, and parental (informed) consent was sought. Based on language background information provided by the teachers, children were selected to participate. Because of our specific selection criteria and the large variation in the children’s language background, only a limited number of children per class were eligible for participation. Children with only Dutch as their home language were classified as L1 readers (n = 83, 51% male), and children with Turkish as their home language were classified as L2 readers (n = 78, 64% male). Because Turkish is the largest linguistic minority (CBS, Citation2016) and the most widely spoken additional language among Dutch primary school children (Extra, Citation2002), only Turkish–Dutch children were selected as L2 readers to maximize homogeneity. From a typological perspective, there is a large distance between Dutch and Turkish, and Turkish has a more transparent orthography than Dutch (Extra & Verhoeven, Citation2011). The instructional language at all schools was Dutch, and from first grade onward all children received formal reading instruction in Dutch only with similar reading methods used across schools. A questionnaire was used to determine the children’s and their parents’ native country and their language usage at home (see ). All L2 readers indicated that they spoke Turkish at home, but there was some variation reflective of the population: For the majority (59%), Turkish was their main home language, whereas others spoke Dutch to a larger extent (33%) or reported equal use of both languages (8%). Most children indicated that they spoke primarily Turkish with at least one parent, whereas they heard their parents speaking mainly Turkish to each other (82%). The L2 readers’ Turkish language proficiency was confirmed by the fact that all L2 readers scored above chance level on a Turkish receptive vocabulary testFootnote1 consisting of 96 multiple-choice items (A–D; percentage correct: M = 61.7, SD = 10.6, range = 35.4–83.3). The L2 readers who had Turkish as their dominant home language scored higher on Turkish vocabulary than children who had Dutch as their dominant home language, t(70) = −2.60, p = .011, d = 0.62.
Table 1. Demographics for L1 Readers and L2 Readers.
The two language groups did not differ in age, t(159) = 0.41, p = .686, d = 0.06, and nonverbal reasoning (Raven, Citation1965), t(159) = 0.99, p = .325 d = 0.16. Considering their parents’ average level of education measured on a 4-point scale from 1 (primary school) to 4 (higher education/university), the L1 readers had a significantly higher socioeconomic status (SES) than the L2 readers (U = 2360.00, z = −3.04, p = .002, r = −.24) despite coming from the same classrooms. This corresponds to other studies with Dutch monolingual and Turkish–Dutch bilingual children (Blom et al., Citation2014; Droop & Verhoeven, Citation2003; Van den Bosch, Segers, & Verhoeven, Citation2018).
Measures
Except for working memory, all factors were assessed by at least two measures. Therefore, the average z score on both tests was calculated for all factors (based on the present sample), and these composite scores were used in the analyses. The z scores were calculated for working memory.
Decoding skills
The children’s decoding skills were assessed with a word reading test and a pseudoword reading test (Verhoeven & Keuning, Citation2017), each consisting of three lists with an increasing number of syllables (e.g., boek, blaas, dromer/laas, stoef, gluifel). For each list, the children were asked to read aloud the (pseudo)words as quickly and accurately as possible within 1 min. The children’s score was the total number of correctly read (pseudo)words across the three lists (Cronbach’s α = .95–.97; Verhoeven & Keuning, Citation2017). On average, the children read more words than pseudowords, t(160) = 22.32, p < .001, d = 1.76, but their scores on both tests were highly correlated (r = .95, p < .001).
Vocabulary knowledge
The children completed two standardized tests for receptive and expressive vocabulary (r = .68, p < .001 in the current study). The 64-item receptive vocabulary test (T-TOS2; Verhoeven, Keuning, Horsels, & Van Boxtel, Citation2013) was presented on a laptop. For each item, a word (noun, verb, or adjective) was presented auditorily along with four pictures on the screen (e.g., in Dutch: juggler, to sketch, muscular). The children had to select the picture that matched the spoken word. The test was terminated after eight consecutive mistakes. A child’s score was the number of items answered correctly (Cronbach’s α = .88, Verhoeven et al., Citation2013).
Expressive vocabulary was measured with the 35-item Word Knowledge Task of the Wechsler Intelligence Scale for Children–Third Edition, Dutch version (Kort et al., Citation2005). Each item consisted of a word that the children were asked to describe orally (e.g., in Dutch: “What is a fairy tale?”). The items increase in difficulty. The children’s answers were scored as 2 points (correct), 1 point (partly correct), or 0 points (incorrect or no answer). The test was terminated after four consecutive mistakes (0 points). A child’s score was the total number of points (Cronbach’s α = .83, Kort et al., Citation2005).
Morphosyntactic knowledge
The children completed two subtests (r = .58, p < .001, in the current study) from the T-TOSFootnote2 (Verhoeven et al., Citation2013). The morphology subtest includes 36 items assessing children’s ability to apply four morphological rules in Dutch: inflexion of plural form of nouns, comparative/superlative of adjectives, inflexion of past tense, and past participle. The items were orally presented supported by images, for example, in Dutch: “This bag is heavy, but that bag is even … (heavier), and that one is the … (heaviest).”
The receptive syntax subtest assesses children’s knowledge of syntactic patterns and elements that determine the meaning of a sentence: word order, prepositions, grammatical roles, pronouns, quantifiers, passive, postmodified subject, ellipsis, and relative clause. The task, presented on a laptop, consists of 33 items. For each item, four pictures were presented, and the children had to select the picture that corresponded with a sentence they heard (e.g., in Dutch: “The dog is bitten by the cat.”). For both subtests, the score was determined by the number of items answered correctly (Cronbach’s α = .87/.82, Verhoeven et al., Citation2013).
Working memory
The children completed the Digit Span Backwards (Wechsler Intelligence Scale for Children–Third Edition, Dutch version; Kort et al., Citation2005). In this standardized task, children first listen to a sequence of digits and then repeat the sequence backward (e.g., 3–8-6; 6–8-3). Each item consists of two sequences of the same length, starting with two-digit sequences. When a child answered at least one sequence of an item correctly, the next item was presented with a longer sequence (one extra digit). The task stopped when a child gave an incorrect answer to both sequences of one item. The score was determined by the number of sequences repeated correctly (Cronbach’s α = .64, Kort et al., Citation2005).
Reading comprehension
The children completed three standardized paper-and-pencil tests for reading comprehension (r = .46–.58, p < .001, in the current study). For children who completed only two out of three tests (n = 12; 7.5%), the composite score was based on two tests instead of three. Two tests specifically aimed at comprehension of semantic relations and anaphoric relations (Verhoeven, Citation1992) and consisted of 27 multiple-choice items (A–D) and 24 multiple-choice items (A–D), respectively. The children’s scores corresponded to the number of items answered correctly for each test (Cronbach’s α = .75 for both tests in the current study). The third test was the CITO test (Cito, Citation2014), which is a more general measure of reading comprehension. This standardized test is part of the Dutch national student monitoring system in primary education. This test consisted of 40 multiple-choice questions (A–D), including a variety of text and question types (Cronbach’s α = .86; Jolink, Tomesen, Hilte, Weekers, & Engelen, Citation2015). A child’s score was a norm-based score determined from the number of items answered correctly.
Procedure
The data were collected during the first half of the second-grade school year by the first author and three trained research assistants. The children performed several tasks in a fixed order during two individual sessions of 30 min each. The sessions occured at school during school hours. The reading comprehension tests were group administered in class by the teacher. The children completed these tests silently without a time limit.
Data analyses
First, we performed independent sample t tests to check for between-group differences with the linear step-up procedure (Benjamini & Hochberg, Citation1995) to control for multiple testing. To investigate whether key predictors of reading comprehension differed depending on the children’s language background, we performed an ordinary least squares (OLS) multiple hierarchical regression analysis, also referred to as classical regression. The variables were included stepwise: (a) four key predictors, (b) language background, and (c) interaction terms between the key predictors and language background. Although there was a relatively strong correlation between vocabulary and morphosyntactic knowledge (r = .78, p < .001), the assumption of no multicollinearity was not violated (largest variance inflation factor = 3.1, average variance inflation factor = 1.9, tolerance statistics > 0.3).
To investigate whether key predictors of reading comprehension differed depending on children’s ability, we performed quantile regression analyses across multiple points in the distribution. In addition to the four key predictors, language background was included as an extra predictor variable to investigate whether it was uniquely related to reading comprehension across the distribution. The relations between predictor variables and the outcome variable were estimated at specific points (i.e., quantiles) along the distribution of the outcome variable. Given that the scores for reading comprehension were not completely normally distributed but skewed to the left (−.66), quantile regression can provide more accurate statistical estimates than OLS regression. There was no loss of power; all data points contributed to the estimation of these quantiles based on the proximity of each data point by means of a weighting matrix (Petscher & Logan, Citation2014). Based on our sample size, the number of parameters and the distribution of the data (Cade & Noon, Citation2003), we selected three quantiles, 0.25, 0.50, and 0.75, as an indication of three ability levels, poor (P), average (A), and good (G) comprehenders, respectively. First, we conducted individual quantile regression analyses to investigate the relation across the three quantiles for each predictor variable separately. Second, we performed a multiple quantile regression analysis including all predictor variables simultaneously to investigate unique relations across the distribution. Quantile regression analyses were performed in the R computing environment (version 3.4.2; R Core Team, Citation2017) using the quantreg package (version 5.34; Koenker, Citation2017).
Results
Group differences for predictor variables and reading comprehension
The means and standard deviations for all measures (raw scores) and composite scores are shown in . There were no group differences for decoding skills or working memory, but the L2 readers scored significantly lower on vocabulary, morphosyntactic knowledge, and reading comprehension with medium to large effect sizes (Cohen, Citation1988). The L1–L2 performance gap in reading comprehension still existed after controlling for differences in SES, F(1, 158) = 22.58, p < .001, ηp2 = .13.
Table 2. Descriptive Statistics for All Measures and Composite Scores for L1 Readers and L2 Readers.
Prediction of reading comprehension for L1 and L2 raders
The correlations among the five factors are shown in . Reading comprehension was positively correlated with the other factors; there was a weak correlation with decoding and working memory and a moderate to strong correlation with vocabulary and morphosyntactic knowledge.
Next, we performed an OLS multiple hierarchical regression analysis to investigate the unique predictors of reading comprehension and to ascertain whether the influence of those predictors differed for L1 and L2 readers. The results (see ) showed that decoding skills, vocabulary, and morphosyntactic knowledge were uniquely related to reading comprehension, but working memory was not, F(4, 156) = 41.15, p < .001, R2 = .513. Language background (Step 2: ΔR2 = .000, p = .799) and the interaction terms (Step 3: ΔR2 = .019, p = .186) did not explain unique variance, indicating that the relation between key predictors and reading comprehension did not differ for the two groups. Adding SES as a control variable did not alter the results and SES did not explain unique variance in reading comprehension (β = .04, p = .579).
Table 3. Correlations Across Variables Based on Composite Scores.
Table 4. Ordinary Least Squares Multiple Regression Analysis for Early Reading Comprehension Performance.
Prediction of reading comprehension across the distribution
shows the distribution of reading comprehension scores split by language background. On average, L1 and L2 readers differed in reading comprehension performance (see ), but there was considerable variation within both groups (see ). Both L1 and L2 readers were represented in all four quartiles, with an overrepresentation of L2 readers in lower quartiles and an overrepresentation of L1 readers in higher quartiles.
Figure 1. Distribution of reading comprehension performance across quartiles (low = 0–25%, below average = 25–50%, above average = 50–75%, high = 75–100%) split by language background.
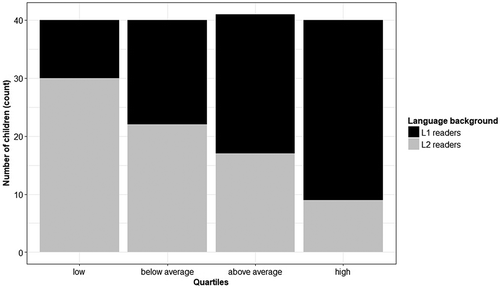
Individual quantile regression analyses
The results of the individual quantile regression analyses are shown in (columns 1–3). All estimates were statistically different from zero (i.e., the confidence intervals [CIs] did not include zero), apart from decoding skills for the higher quantile (0.75). This indicates that vocabulary, morphosyntactic knowledge, working memory, and language background were related to reading comprehension across the distribution. By contrast, decoding was related to reading comprehension for poor and average comprehenders but not for good comprehenders.
Table 5. Individual Quantile Regression Coefficients and Comparisons of Coefficients for Poor (P), Average (A), and Good (G) Comprehenders.
To ascertain whether the relation of each predictor was stronger at one point in the distribution than another, we calculated statistical significance tests of between-quantile slopes (see , columns 4–6). Again, we used the linear step-up procedure (Benjamini & Hochberg, Citation1995) to correct for multiple testing across quantiles. The comparisons showed that decoding was significantly stronger related to reading comprehension at the lower and mediate quantiles compared to the higher quantile. Furthermore, vocabulary knowledge was significantly stronger related to reading comprehension at the lower quantile compared to the mediate and higher quantiles.
A similar pattern was found for language background; however, the interpretation of a dichotomous predictor is substantially different from a continuous predictor (Petscher & Logan, Citation2014). The quantile regression coefficients (i.e., slope coefficients) are indicative of the performance gap in reading comprehension between L2 readers (dummy code = 1) as compared to L1 readers (dummy code = 0). The L1–L2 performance gap in reading comprehension performance was not uniform across the distribution: At the lower quantile, the performance gap was 0.99 and decreased to 0.56 at the mediate quantile and 0.38 at the higher quantile. The results of the between-quantile slope differences showed that the L1–L2 performance gap was significantly larger at the lower quantile compared to the mediate and higher quantiles. For morphosyntactic knowledge and working memory, no significant differences in slope coefficients were found between the three quantiles.
Multiple quantile regression analysis
Finally, we conducted a multiple quantile regression analysis because we were especially interested in how each variable was uniquely related to reading comprehension across the three quantiles after controlling for the other variables. The results of the multiple quantile regression analysis are shown in (columns 2–4), which can be compared to the regression coefficients of the OLS multiple regression analysis (column 1) retrieved from , Step 2. Decoding and morphosyntactic knowledge were uniquely related to reading comprehension across the distribution, indicating that these two constructs are important for reading comprehension regardless of ability level. By contrast, differential relations were found for vocabulary and working memory. Vocabulary knowledge was significant only at the lower quantile, whereas working memory was significant only at the higher quantile. Language background was not related to reading comprehension across the distribution after controlling for the other predictor variables. Again, adding SES showed no unique effect at any of the quantiles, and the results hardly changed; the confidence interval for working memory just cross the zero boundary, 95% CI [−0.01, 0.21], only at the higher quantile (0.75). This is probably due to limited power in the extreme of the distribution as working memory was still significant when the higher quantile was set to 0.70, 95% CI [0.01, 0.18]. To examine the explained variance across quantiles, we calculated pseudo R2 (Petscher, Logan, & Zhou, Citation2013). The amount of explained variance was largest at the lower quantile (37.2%) and somewhat lower at the mediate quantile (31.1%) and higher quantile (26.2%).
Table 6. Multiple OLS Regression and Multiple Quantile Regression Analyses for Poor (P), Average (A), and Good (G) Comprehenders.
Discussion
We aimed to gain insight into the factors that explain individual variation in early reading comprehension. We used two complementary approaches to investigate whether the key predictors of reading comprehension differed for L1 and L2 readers (considering the distribution of scores as a whole) and/or across ability levels (considering specific points across the distribution of scores). The main findings of the present study are discussed in relation to previous studies, and the theoretical and practical implications are described.
Early reading comprehension in L1 and L2 readers
First, our findings showed that even at the very start of reading acquisition, there was a performance gap in reading comprehension between L1 and L2 readers (Melby-Lervåg & Lervåg, Citation2014; Verhoeven, Citation2000; Verhoeven et al., Citation2018) and that L2 readers scored considerably lower on oral language skills (i.e., vocabulary and morphosyntactic knowledge), whereas their decoding skills and working memory capacity were comparable with L1 readers. A closer look at the distribution of the children’s reading comprehension scores revealed that there was considerable variation within both groups, and there was overlap between L1 and L2 readers’ score distribution; L1 and L2 readers were both represented in the lower and higher ends of the distribution. This finding corresponds with Lesaux et al. (Citation2006), who showed similar percentiles for L1 and L2 readers when classifying children as poor or good comprehenders. Moreover, it stresses the relevance of investigating the role of linguistic diversity across the distribution of reading comprehension performance.
Regarding the first approach, classical regression showed that the predictor variables for early reading comprehension performance were similar for L1 and L2 readers. Decoding skills, vocabulary, and morphosyntactic knowledge were identified as unique predictors of early reading comprehension performance, irrespective of language background. Considering the beta values, vocabulary and morphosyntactic knowledge were the most important factors for children’s reading comprehension, whereas decoding skills were less important. The latter is inconsistent with previous studies showing that early reading comprehension is strongly related to decoding skills for young monolingual readers of English (Cain, Citation2010; Catts et al., Citation2005; Lonigan & Burgess, Citation2017). One explanation for this discrepancy relates to differences in language transparency across studies. The children in our study read in Dutch, which is more transparent than English and may result in earlier mastery of decoding accuracy (Ellis et al., Citation2004; Verhoeven & Van Leeuwe, Citation2009). Consequently, children’s decoding skills may play a less prominent role in early reading comprehension for transparent orthographies, as found by Florit and Cain’s meta-analysis (Citation2011) on the Simple View of Reading in different types of orthographies. Another explanation relates to our mixed sample. Half of our sample consisted of L2 readers, who often have reading comprehension difficulties that probably stem from delays in oral language skills rather than poor decoding skills (Melby-Lervåg & Lervåg, Citation2014). In that respect, some studies have even shown that there is a stronger relation between oral language and reading comprehension for L2 readers as compared to L1 readers (Babayiğit, Citation2014; Droop & Verhoeven, Citation2003; Geva & Farnia, Citation2012; Lervåg & Aukrust, Citation2010). However, the current study is consistent with a more balanced view in which the importance of the underlying constructs is independent of children’s language background (Lesaux et al., Citation2006; Verhoeven & Van Leeuwe, Citation2012).
Early reading comprehension across ability levels
Regarding the second approach, quantile regression demonstrated that the relation between key predictors and reading comprehension differed depending on the children’s ability level as expected based on other recent studies using quantile regression (Hua & Keenan, Citation2017; Logan, Citation2017; Mancilla-Martinez & Lesaux, Citation2017; Tighe & Schatschneider, Citation2016). Our findings showed that decoding skills and morphosyntactic knowledge were uniquely related to reading comprehension across the ability range, but vocabulary knowledge was related to reading comprehension for poor comprehenders only, whereas working memory was related to reading comprehension for good comprehenders only. This suggests that poor comprehenders may have particular difficulty with lower level comprehension skills (i.e., vocabulary knowledge), which hinders their comprehension processes, whereas good comprehenders rely less on lower level skills and thus have more cognitive capacity available for higher level comprehension skills (i.e., skills requiring working memory), which benefits their comprehension processes.
In terms of age, it seems most relevant to compare our findings to the study of LRRC and Logan (Citation2017), who also used quantile regression to investigate pressure points in reading comprehension in young readers. Logan (Citation2017) found similar results for (morpho)syntactic knowledge (i.e., the strongest unique predictor across the distribution), but they showed reversed results for decoding skills and vocabulary; vocabulary knowledge was a consistently significant predictor across ability levels, whereas decoding was only a significant predictor at the lower level.
Two explanations can be proposed related to our sample. First, the children in our sample were, on average, one year younger and less experienced in reading. This may have led to a more prominent role for decoding skills across the distribution as shown by the multiple quantile regression analysis, although the individual quantile regression analysis showed that this relation was significantly weaker (and nonsignificant) for good comprehenders. This discrepancy between analyses may be due to the complex nature of unique and shared effects in multiple regression, where the magnitude of unique effects is influenced by the extraneous variance of the other predictor variables included (Lindenberger & Pötter, Citation1998). Because we were mainly interested in the unique effects of the key predictors, greater value was attached to multiple quantile regression for the interpretation of the results. Second, our sample included L1 readers as well as L2 readers, with an overrepresentation of L2 readers at the lower end of the distribution. Given that L2 readers often show delays in terms of vocabulary knowledge in the target language (Melby-Lervåg & Lervåg, Citation2014), this may explain the more prominent role of vocabulary knowledge at the lower ability level. In other words, our findings suggest that vocabulary knowledge is the main obstacle, or pressure point, for beginning readers with lower reading comprehension performance.
Apart from vocabulary knowledge, the relation between working memory and reading comprehension also differed across ability levels. In contrast to Cain et al. (Citation2004), who studied 8- to 11-year-olds, our classical regression analysis did not show a unique relation between working memory and reading comprehension. By contrast, our quantile regression analysis revealed that this relation seems to exist for higher performing children in our sample. We thus tentatively suggest that in the early stages of reading comprehension, particularly good comprehenders engage in higher level comprehension processes, as these require working memory (Kendeou et al., Citation2014). This also relates to the lower amount of explained variance at the higher level; possibly other higher order skills not included in the present study, such as inference making and comprehension monitoring, may be more important for good comprehenders (Cain et al., Citation2004).
The role of linguistic diversity in early reading comprehension
In both approaches, we also investigated whether children’s language background (L1 vs. L2) adds to the prediction of reading comprehension over and above the key predictors. Although language background was related to reading comprehension across the ability range (as shown by the individual quantile regression), in both the classical and quantile multiple regression analyses, language background did not explain additional variance over and above the variance accounted for by the key predictors. This was even the case at the lower ability level, where the performance gap between L1 and L2 readers was largest. Hence, the current study demonstrated that the prediction of reading comprehension is similar for beginning L1 and L2 readers because the key predictors (i.e., decoding skills, vocabulary, and morphosyntactic knowledge) were equally important for L1 and L2 readers, and individual differences in these skills were sufficient to explain the L1–L2 performance gap in early reading comprehension across the distribution. This contrasts with Lesaux et al. (Citation2006), who found that after controlling for several linguistic and cognitive predictors, children’s language status (ESL vs. EL1) still explained unique variance in fourth-grade reading comprehension performance. However, Lesaux et al. (Citation2006) did not control for decoding skills and vocabulary knowledge, which are two central components of reading comprehension. Not including vocabulary knowledge, which is one of the most prominent differences between monolingual and bilingual children (Bialystok, Luk, Peets, & Yang, Citation2010), is likely to account for the additional variance explained by language background in Lesaux et al. (Citation2006). In line with our findings, Babayiğit (Citation2014) also found that individual differences in oral language skills (i.e., vocabulary and morphosyntactic skills) explained the differences in fifth-grade reading comprehension between L1 and L2 readers of English.
Limitations and future directions
The present study has some limitations that require careful interpretation of the results. First, we included only L2 readers from the largest linguistic minority to maximize the homogeneity of the L2 group. Second, we were not able to include L2 readers’ native language skills as was done by Mancilla-Martinez and Lesaux (Citation2017), because we conducted our analyses with a mixed group of L1 and L2 readers. Nevertheless, in our study, L2 reading comprehension was not related to L1 vocabulary (r = .18, p = .118), and having either Turkish or Dutch as the dominant home language did not affect L2 reading comprehension scores, t(70) = 0.18, p = .861, d = 0.04. Third, we used cross-sectional data rather than longitudinal data, which means that we cannot draw conclusions about the prediction of reading comprehension over time. Nevertheless, the current study demonstrates the relevance of investigating key predictors of reading comprehension beyond the average relation and across the distribution of reading comprehension performance. In future research the use of a quantile regression approach should be extended in several ways: (a) by including another or more heterogeneous group of L2 readers; (b) by including a broader range of explanatory factors, such as sociolinguistic (e.g., quantity/quality of language usage) or metacognitive factors (e.g., comprehension monitoring); and (c) by including a larger sample to allow for latent variable modeling and reliable detection of interaction effects.
Conclusion and implications
Overall, this study has provided new insights into the factors that explain individual variation in early reading comprehension in a mixed group of L1 and L2 readers. By combining classical and quantile regression techniques, we demonstrated that the explanatory factors of reading comprehension are more likely to vary as a function of children’s ability level than their language background. By extending the use of quantile regression to a linguistically diverse group of beginning readers, this study adds to previous research showing that relations between key predictors and reading comprehension can vary across the distribution, even at the very beginning of reading acquisition, where intervention should ultimately start. Our findings revealed that children with lower vocabulary knowledge are particularly at risk for reading comprehension problems rather than L2 readers as a group. Furthermore, our study indicated that in the early stages of reading development, the key predictors of reading comprehension are similar for L1 and L2 readers, although, on average, L2 readers showed a delay with respect to reading comprehension and some of the key predictors, such as vocabulary and morphosyntactic knowledge. The conclusion that the prediction of reading comprehension is more universal than expected and that the role of linguistic diversity is less prominent than assumed has implications for educational practice. Educational professionals and teachers should be cautious about classifying or selecting children on the basis of their language background, because categorizing children as either monolingual or bilingual does not do justice to the individual variation across children (Luk & Bialystok, Citation2013) and may be off target when it comes to the differentiation or allocation of extra support in education. Instead, children’s language and literacy skills should guide teachers (and policy makers) to meet individual needs in the classroom.
Conflict of interest
The authors declared that there is no conflicts of interest.
Acknowledgments
We thank all schools and children for their participation, Dr. Louis ten Bosch for his helpful advice on the analyses, and the anonymous reviewers for their constructive comments.
Notes
1 The Turkish receptive vocabulary test is a translation of the Dutch version of the Peabody Picture Vocabulary Test (Dunn & Dunn, Citation2007) developed as part of the CoDEmBI project (https://www.uu.nl/onderzoek/education-for-learning-societies/projecten-resultaten/codembi). With the permission of the original publisher (i.e., Pearson), all items in sets 1 to 12 were translated. Turkish items were excluded if they were considered to be much easier or more difficult than the target words in the Dutch version or if they were cognates of the Dutch target words. This resulted in the removal of four items per set in the Turkish test with a remaining total of eight items per set for the first 12 sets. All 96 items were administered (Cronbach’s α = .859, in the current study).
2 The T-TOS test battery was primarily developed for diagnostic purposes, but it can also be used to assess linguistic skills in typically developing children. Norm-based standardized scores based on a representative sample across the age range (4–10 years old) are available for all subtests.
References
- Babayiğit, S. (2014). The role of oral language skills in reading and listening comprehension of a text: A comparison of monolingual (L1) and bilingual (L2) speakers of English Language. Journal of Research in Reading, 37, 22–47. doi:10.1111/j.1467-9817.2012.01538.x
- Baddeley, A. (2003). Working memory and language: An overview. Journal of Communication Disorders, 36, 189–208. doi:10.1016/S0021-9924(03)00019-4
- Benjamini, Y., & Hochberg, Y. (1995). Controlling for the false discovery rate: A practical and powerful approach to multiple testing. Journal of the Royal Statistics Society, 57, 289–300.
- Bialystok, E., Luk, G., Peets, K. F., & Yang, S. (2010). Receptive vocabulary differences in monolingual and bilingual children. Bilingualism: Language and Cognition, 13, 525–531. doi:10.1017/S1366728909990423
- Blom, E., Küntay, A. C., Messer, M., Verhagen, J., & Leseman, P. (2014). The benefits of being bilingual: Working memory in bilingual Turkish-Dutch children. Journal of Experimental Child Psychology, 128, 105–119. doi:10.1016/j.jecp.2014.06.007
- Cade, B. S., & Noon, B. R. (2003). A gentle introduction to quantile regression for ecologists. Frontiers in Ecology and the Environment, 1, 412–420. doi:10.1890/1540-9295(2003)001[0412:AGITQR]2.0.CO;2
- Cain, K. (2010). Reading development and difficulties. Chichester, UK: BPS Blackwell.
- Cain, K., & Oakhill, J. (2006). Profiles of children with specific reading comprehension difficulties. British Journal of Educational Psychology, 76, 683–696. doi:10.1348/000709905X67610
- Cain, K., Oakhill, J., & Bryant, P. (2004). Children’s reading comprehension ability: Concurrent prediction by working memory, verbal ability, and component skills. Journal of Educational Psychology, 96, 31–42. doi:10.1037/0022-0663.96.1.31
- Catts, H. W., Hogan, T. P., & Adlof, S. M. (2005). Developmental changes in reading and reading disabilities. In H. W. Catts & A. G. Kamhi (Eds.), The connections between language and reading disabilities (pp. 25–40). Mahwah, NJ: Lawrence Erlbaum Associates.
- CBS Statistics the Netherlands (2016). Bevolking naar migratieachtergrond [Population byimmigration background]. Retrieved from https://www.cbs.nl/nl-nl/achtergrond/2016/47/bevolking-naar-migratieachtergrond
- Cito, B. V. (2014). Begrijpend lezen 3.0 voor groep 4: M4-toets [Reading Comprehension 3.0 for second grade: M4 test]. Arnhem, the Netherlands: Cito B.V.
- Cohen, J. (1988). Statistical power analysis for the behavioral sciences (2nd edition). Hillsdale, NJ: Lawrence Earlbaum Associates.
- Droop, M., & Verhoeven, L. (2003). Language proficiency and reading ability in first- and second-language learners. Reading Research Quarterly, 38, 78–103. doi:10.1598/RRQ.38.1.4
- Dunn, D. M., & Dunn, L. M. (2007). Peabody Picture Vocabulary Test, Fourth Edition, manual. Minneapolis, MN: NCS Pearson, Inc.
- Ellis, N. C., Natsume, I., Stavropoulou, K., Hoxhallari, L., Van Daal, V. H. P., Polyzoe, N., … Petalas, M. (2004). The effects of the orthographic depth on learning to read alphabetic, syllabic, and logographic scripts. Reading Research Quarterly, 39, 438–468. doi:10.1598/RRQ.39.4.5
- Extra, G. (2002). De andere talen van Nederland: Thuis en op school. [The Netherlands’ other languages: At home and at school]. Bussum, the Netherlands: Coutinho.
- Extra, G., & Verhoeven, L. (2011). Processes of language change in a migration context. In G. Extra & L. Verhoeven (Eds.), Bilingualism and Migration (pp. 29–57). Berlin, Germany: Walter de Gruyter.
- Florit, E., & Cain, K. (2011). The Simple View of Reading: Is it valid for different types of alphabetic orthographies?. Educational Psychology Review, 23, 553–576. doi:10.1007/s10648-011-9175-6
- Geva, E., & Farnia, F. (2012). Developmental changes in the nature of language proficiency and reading fluency paint a more complex view of reading comprehension in ELL and EL1. Reading and Writing, 25, 1819–1845. doi:10.1007/s11145-011-9333-8
- Geva, E., & Zadeh, Z. Y. (2006). Reading efficiency in native English-speaking and English as-a-second-language children: The role of oral proficiency and underlying cognitive-linguistic processes. Scientific Studies of Reading, 10, 31–57. doi:10.1207/s1532799xssr1001_3
- Gough, P. B., & Tunmer, W. E. (1986). Decoding, reading and reading disability. Remedial and Special Education, 7, 6–10. doi:10.1177/074193258600700104
- Grundy, J. G., & Timmer, K. (2017). Bilingualism and working memory capacity: A comprehensive meta-analysis. Second Language Research, 33, 325–340. doi:10.1177/0267658316678286
- Hoover, W. A., & Gough, P. B. (1990). The simple view of reading. Reading and Writing, 2, 127–160. doi:10.1007/BF00401799
- Hua, A. N., & Keenan, J. M. (2017). Interpreting reading comprehension test results: Quantile regression shows that explanatory factors can vary with performance level. Scientific Studies of Reading, 21, 225–238. doi:10.1080/10888438.2017.1280675
- Jolink, A., Tomesen, M., Hilte, M., Weekers, A., & Engelen, R. (2015). Wetenschappelijke verantwoording Begrijpend lezen 3.0 voor groep 4 [Scientific justification for reading comprehension test 3.0 for second grade]. Arnhem, the Netherlands: Cito B.V.
- Kendeou, P., Van Den Broek, P., Helder, A., & Karlsson, J. (2014). A cognitive view of reading comprehension: Implications for reading difficulties. Learning Disabilities Research & Practice, 29, 10–16. doi:10.1111/ldrp.12025
- Koenker, R. (2017). Quantreg: Quantile regression. R package version 5.34. Retrieved from https://CRAN.R-project.org/package=quantreg
- Kort, W., Schittekatte, M., Dekker, P. H., Verhaeghe, P., Compaan, E. L., Bosmans, M., & Vermeir, G. (2005). WISC-III NL. Handleiding en Verantwoording. In D. Wechsler (Ed.), [Manual and Justification]. London, UK: The Psychological Corporation.
- Lervåg, A., & Aukrust, V. G. (2010). Vocabulary knowledge is a critical determinant of the difference in reading comprehension growth between first and second language learners. Journal of Child Psychology and Psychiatry, 51, 612–620. doi:10.1111/j.1469-7610.2009.02185.x
- Lesaux, N. K., Lipka, O., & Siegel, L. S. (2006). Investigating cognitive and linguistic abilities that influence the reading comprehension skills of children from diverse linguistic backgrounds. Reading and Writing, 19, 99–131. doi:10.1007/s11145-005-4713-6
- Lindenberger, U., & Pötter, U. (1998). The complex nature of unique and shared effects in hierarchical linear regression: Implications for developmental psychology. Psychological Methods, 3, 218–230. doi:10.1037/1082-989X.3.2.218
- Logan, J.; Language and Reading Research Consortium (LRRC). (2017). Pressure points in reading comprehension: A quantile multiple regression analysis. Journal of Educational Psychology, 109, 451–464. doi:10.1037/edu0000150
- Lonigan, C. J., & Burgess, S. R. (2017). Dimensionality of reading skills with elementary-school-aged children. Scientific Studies of Reading, 21, 239–253. doi:10.1080/10888438.2017.1285918
- Luk, G., & Bialystok, E. (2013). Bilingualism is not a categorical variable: Interaction between language proficiency and usage. Journal of Cognitive Psychology, 25, 605–621. doi:10.1080/20445911.2013.795574
- Mancilla-Martinez, J., & Lesaux, N. K. (2010). Predictors of reading comprehension for struggling readers: The case of Spanish-speaking language minority children. Journal of Educational Psychology, 102, 701–711. doi:10.1037/a0019135
- Mancilla-Martinez, J., & Lesaux, N. K. (2017). Early indicators of later English reading comprehension outcomes among children from Spanish-speaking homes. Scientific Studies of Reading, 21, 428–448. doi:10.1080/10888438.2017.1320402
- McIlraith, A. L., Language and Reading Research Consortium (LRRC). (2016). Predicting word reading ability: A quantile regression study. Journal of Research in Reading, doi:10.1111/1467-9817.12089
- Melby-Lervåg, M., & Lervåg, A. (2014). Reading comprehension and its underlying components in second-language learners: A meta-analysis of studies comparing first-and second-language learners. Psychological Bulletin, 140, 409–433. doi:10.1037/a0033890
- Morales, J., Calvo, A., & Bialystok, E. (2013). Working memory development in monolingual and bilingual children. Journal of Experimental Child Psychology, 114, 187–202. doi:10.1016/j.jecp.2012.09.002
- Muter, V., Hulme, C., Snowling, M. J., & Stevenson, J. (2004). Phonemes, rimes, vocabulary, and grammatical skills as founding of early reading development: Evidence from a longitudinal study. Developmental Psychology, 40, 663–681. doi:10.1037/0012-1649.40.5.665
- Nation, K., Cocksey, J., Taylor, J., & Bishop, D. (2010). A longitudinal investigation of the early language and reading skills in children with reading comprehension impairment. Journal of Child Psychology & Psychiatry, 51, 1031–1039. doi:10.1111/j.1469-7610.2010.02254.x
- Oakhill, J., Hartt, J., & Samols, D. (2005). Levels of comprehension monitoring and working memory in good and poor comprehenders. Reading and Writing, 18, 657–686. doi:10.1007/s11145-005-3355-z
- Perfetti, C. (2007). Reading ability: Lexical quality to comprehension. Scientific Studies of Reading, 11, 357–383. doi:10.1080/10888430701530730
- Perfetti, C., & Stafura, J. (2014). Word knowledge in a theory of reading comprehension. Scientific Studies of Reading, 18, 22–37. doi:10.1080/10888438.2013.827687
- Petscher, Y., & Logan, J. A. R. (2014). Quantile regression in the study of developmental sciences. Child Development, 85, 861–881. doi:10.1111/cdev.12190
- Petscher, Y., Logan, J. A. R., & Zhou, C. (2013). Extending conditional means modeling: An introduction to quantile regression. In Y. Petscher, C. Schatschneider, & D. L. Compton (Eds.), Applied quantitative analysis in education and social sciences (pp. 3–33). New York, NY: Routledge.
- R Core Team (2017). R: A language and environment for statistical computing. R Foundation for Statistical Computing, Vienna, Austria. Retrieved from https://www.R-project.org/
- Raven, J. C. (1965). Guide to using coloured progressive matrices, Sets A, AB and B. London, UK: BHK Levis & Co.
- Seigneuric, A., Ehrlich, M. F., Oakhill, J. V., & Yuill, N. M. (2000). Working memory and children’s reading comprehension. Reading and Writing, 13, 81–103. doi:10.1023/A:1008088230941
- Stanovich, K. E. (1980). Toward an interactive-compensatory model of individual differences in the development of reading fluency. Reading Research Quarterly, 16, 32–71. doi:10.2307/747348
- Tighe, E. L., & Schatschneider, C. (2016). A quantile regression approach to understanding the relations among morphological awareness, vocabulary and reading comprehension in adult basic education students. Journal of Learning Disabilities, 49, 424–436. doi:10.1177/0022219414556771
- Van den Bosch, L. J., Segers, E., & Verhoeven, L. (2018). Online processing of causal relations in beginning first and second language readers. Learning and Individual Differences, 61, 59–67. doi:10.1016/j.lindif.2017.11.007
- Verhoeven, L. (1992). Lezen met Begrip 1. In L. Verhoeven (Ed.), [Reading for Understanding 1]. Arnhem, the Netherlands: Cito.
- Verhoeven, L. (2000). Components in early second language reading and spelling. Scientific Studies of Reading, 4, 313–330. doi:10.1207/S1532799XSSR0404_4
- Verhoeven, L., & Keuning, J. (2017). The nature of developmental dyslexia in a transparent orthography. Scientific Studies of Reading, 1–17. doi:10.1080/10888438.2017.1317780
- Verhoeven, L., & Van Leeuwe, J. (2009). Modeling the growth of word-decoding skills: Evidence from Dutch. Scientific Studies of Reading, 13, 205–223. doi:10.1080/10888430902851356
- Verhoeven, L., & Van Leeuwe, J. (2012). The simple view of second language reading throughout the primary grades. Reading and Writing, 25, 1805–1818. doi:10.1007/s11145-011-9346-3
- Verhoeven, L., Voeten, M., & Vermeer, A. (2018). Beyond the simple view of early first and second language reading: The impact of lexical quality. Journal of Neurolinguistics. doi:10.1016/j.jneuroling.2018.03.002
- Verhoeven, L. T. W., Keuning, J., Horsels, L., & Van Boxtel, H. (2013). Testinstrumentarium Taalontwikkelingsstoornissen T-TOS. In Cito B.V. (Ed.), [Set of Instruments Developmental Language Disorder]. Arnhem, the Netherlands: Cito.