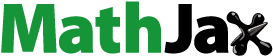
ABSTRACT
We examined whether word recognition accuracy and latency of words children encounter during primary school across the upper primary school grades can be predicted from word form (word length, mean Levenshtein distance, and mean frequency of neighbors), word meaning (free association network markers) and word exposure (corpus frequency and contextual diversity). As a measure of word recognition, 1454 children (M = 10.1 years, SD = 11.8 months, 52.4% girls) in grade 3, 4 and 5 of Dutch regular primary schools completed a lexical decision task. Confirmatory factor analyses showed that word characteristics could be reduced to latent constructs of form, meaning, and exposure. Structural equation models indicated that word form and exposure predicted word recognition accuracy, and that word recognition accuracy, word form, and word meaning predicted word recognition latency. The present study provided empirical evidence that word form, word meaning, and word exposure differentially predict word recognition accuracy and latency of words children encounter during primary school across the upper primary grades.
Introduction
Visual word recognition, or the ability to recall word representations from memory, is a cornerstone of reading and learning in school. When a child is presented with a string of letters, the first process is to decode the letters to the spoken form. If a word has been successfully decoded, it can be recognized as familiar (seen or heard before) with greater speed. Word recognition is possible without word identification (recall of meaning and encountered contexts, Perfetti, Citation2017), since word recognition can take place by recognizing trained pseudowords as familiar or by only having a vague sense of familiarity after having heard a word once or twice. However, studies have shown that word recognition ability is facilitated by semantic knowledge via semantic priming (Hoedemaker & Gordon, Citation2017) and semantic richness effects (Yap, Pexman, Wellsby, Hargreaves, & Huff, Citation2012). This indicates that word recognition is a proxy for deeper word knowledge. Word recognition ability grows incrementally through exposure (Reichle & Perfetti, Citation2003) and various word characteristics have been shown to predict word recognition accuracy and latency (see Yap & Balota, Citation2009). For instance, it has been posited that word form, which relates to both the phonological and orthographic representation of a word, is associated with measures such as word length and orthographic neighborhood size (for an overview see Rastle, Citation2016). Word meaning is predicted by semantic richness variables (for an overview see Pexman, Hargreaves, Siakaluk, Bodner, & Pope, Citation2008), and word exposure by word frequency measures (for a recent review see Brysbaert, Mandera, & Keuleers, Citation2018).
So-called “mega studies” have shown the unique predictive effects of a large collection of word characteristics (e.g., Yap & Balota, Citation2009) on a large collection of adult word recognition responses. However, no attempt has been made to reduce various word characteristics into these three overarching constructs of word form, word meaning and word exposure and to examine how these relate to children’s word recognition accuracy and latency in one and the same design. Throughout the primary grades, children are developing their word recognition skill and they need to reach a certain accuracy threshold before they can learn to speed up (Juul, Poulsen, & Elbro, Citation2014; Karageorgos, Müller, & Richter, Citation2019) in order to free resources for higher level processing (i.e., reading comprehension, Perfetti, Citation2017). However, it is still unclear how children’s accuracy and speed of word recognition can be predicted from differential word characteristics. Therefore, in the present study we examined to what extent the accuracy and latency of word recognition of a representative set of words in the upper primary grades can be predicted from form, meaning and exposure characteristics of these words.
Word recognition across the primary grades
A review by Castles, Rastle, and Nation (Citation2018) describes how children develop their visual word recognition skill from novices to experts. The first step is cracking the alphabetic code. Children first need to gain the insight that written symbols correspond to sounds and they can then become more and more skilled in phonological recoding (translating a written string to its spoken form). According to the self-teaching hypothesis (Share, Citation1995), this orthographic learning of novel words is a consequence of simply encountering them in everyday reading. Previous studies have shown that only a few encounters with novel words are sufficient for children and adults to acquire orthographical representations (Share, Citation2004). When children become more skilled at reading, they are able to retrieve a word’s meaning swiftly and automatically without phonological recoding (Castles & Nation, Citation2006; Nation & Castles, Citation2017). It is assumed that this process of acquiring a direct mapping between written form and meaning grows item-by-item. This means that a child may have acquired direct mappings from written form to meaning for some words, but still relies on phonological recoding to read other words. Exposure to printed words, i.e. repeatedly encountering written words in context, also leads to a strengthening of the lexical quality of the mental representations of words (Frishkoff, Perfetti, & Collins-Thompson, Citation2010). Consequently, exposure has repeatedly been shown to be a significant predictor of word recognition (Brysbaert & Cortese, Citation2011; Dufau et al., Citation2010; Herdağdelen & Marelli, Citation2017).
In the lexical quality framework (Perfetti, Citation2017), lexical quality is characterized by lexical representations that contain specific and redundant information about form (orthography and phonology), unification (morphology and constituent binding), and generalized context-tuned meaning. When a reader has acquired high lexical quality, cognitive resources are available for higher-level processes such as comprehension. As a consequence, children’s reading comprehension skills rely on their knowledge of each of the components of lexical quality (Richter, Isberner, Naumann, & Neeb, Citation2013). Although high lexical quality might not be necessary to recognize a word in isolation, many studies have shown that more familiar words with more elaborate representations are recognized more accurately and faster (for an overview, see Perfetti, Citation2017). Specific and redundant orthographic representations allow for finely tuned word recognition. The development of finely tuning orthographic representations was studied by Castles, Davis, Cavalot, and Forster (Citation2007) comparing beginning readers (grade 3 and 5) to skilled adult readers. Orthographic representations of grade 3 children were broadly tuned and became more precise in grade 5, but not as precise as compared to adult readers. As orthographic representations are still developing in the upper primary grades, the effects of orthographic neighborhood size and frequency that have often been shown in adult word recognition (e.g., Yap & Balota, Citation2009), may not generalize to children’s word recognition.
Words are not only connected in the mental lexicon based on form, but also on meaning characteristics. The mental lexicon is formed as an interrelated network where semantically related words are connected (Meara & Wolter, Citation2004), and thus closer together in the mental semantic space. As children develop from beginning to expert readers, reading experience plays a vital role. Upon each encounter of a word, the connections to other words may be updated, building the lexical legacy (Nation, Citation2017) of the word. The semantic distinctiveness model stipulates that the weights in semantic memory are updated most when words are encountered in diverse and meaningful contexts (Jones, Dye, & Johns, Citation2017), as the weights hardly need to be changed when a word is encountered in similar contexts. As a result, words that occur in (many) different contexts are more strongly coded in the mental lexicon, enhancing lexical quality and facilitating word processing. This is evidenced by semantic diversity effects, over and above the effect of frequency, on children’s word recognition accuracy and latency (Hsiao & Nation, Citation2018) and adult’s word learning (Johns, Dye, & Jones, Citation2016).
Different types of word characteristics
Many word characteristics, representing different aspects of words, have been shown to play a role in the recognition of words in isolation (for a large study with many word characteristics predicting visual word recognition, see Balota, Cortese, Sergent-Marshall, Spieler, & Yap, Citation2004). Perfetti (Citation2017) argued that word knowledge relies on knowledge of form (phonology and orthography) and meaning, which are incrementally built up through exposure (Reichle & Perfetti, Citation2003). Thus, as possible predictors of word recognition ability a distinction can be made between word characteristics based on form, meaning, and exposure.
Form related word characteristics at the lexical level (whole word) provide an indication of the orthographical and phonological complexity of words. Investigating the effect of word length provides insight in the shift from recognizing a word through an indirect, sub-lexical process to a direct, lexical search process as typically the effect of word length decreases with age and mainly predicts speed and not accuracy (Martens & De Jong, Citation2006; Schröter & Schroeder, Citation2018). New, Ferrand, Pallier, and Brysbaert (Citation2006) found a quadratic effect of word length on adult visual word recognition latencies: There was a facilitating effect of length on response latencies for words of 3–5 letters, no effect of length for words of 5–8 letters, and an inhibitory effect for words of 8–13 letters. This quadratic effect was also found in adults (e.g., Yap & Balota, Citation2009) and children (Schröter & Schroeder, Citation2018; Van den Boer, De Jong, & Haentjens-van Meeteren, Citation2012). Furthermore, the number of orthographic neighbors (neighborhood density, Coltheart’s N) has been shown to have a facilitatory effect on word recognition, and the mean frequency of these neighbors typically has an inhibitory effect on word recognition (for a review, see Andrews, Citation1997). An issue of using Coltheart’s N is that some, typically longer, words only have one neighbor or none at all. This can be resolved by measuring orthographic or phonologic similarity based on Levenshtein distance (Yarkoni, Balota, & Yap, Citation2008). Similarity between two letter strings is based on the smallest number of insertions, deletions and substitutions of letters necessary to transform one letter string into the other. The mean distance to the 20 nearest neighbors has been shown to have a similar, but stronger, effect on word recognition as Coltheart’s N and the mean frequency of these 20 nearest neighbors has a similar effect on word recognition as Coltheart’s N frequency (Yap et al., Citation2012; Yarkoni et al., Citation2008). In Dutch, words with a short mean distance to the 20 nearest neighbors are also processed faster than words with longer mean distances (Brysbaert, Stevens, Mandera, & Keuleers, Citation2016). When predicting Dutch children’s word processing performance, Marinus and De Jong (Citation2010) showed an inhibitory effect of a high-frequency neighbor on beginning and dyslexic readers’ word naming latency. In summary, word length, mean Levenshtein distance to the 20 nearest neighbors, and the frequency of these neighbors appear to be highly relevant form-related word characteristics when predicting (children’s) word recognition.
The effects of meaning related measures on word recognition have been divided into effects of semantic richness, semantic ambiguity, and valence in a review by Pexman (Citation2012). Semantic richness has a facilitatory effect on processing, i.e., when a word has a richer semantic representation, more semantic information comes to mind, and word recognition is facilitated. Typical semantic richness variables include imageability, number-of-features, semantic neighbors, number of associates, et cetera. What or how much “comes to mind” can be described using free association tasks where participants are presented with a word and are asked to list one or multiple words that were semantically related or associated to the cue word (Nelson, McEvoy, & Schreiber, Citation2004). With these responses, an associative network of the words can be created. The links between words in this network can be described using various metrics (e.g., centrality), and several of these metrics have been shown to predict word recognition. De Deyne, Navarro, and Storms (Citation2013) showed that centrality measures strongly predict adult word recognition and argue that the centrality measures relate to various other semantic richness effects. For example, semantic neighborhood size relates to the clustering coefficient that represents the incoming and outgoing nodes of the word and how connected the neighbors are. The effect of centrality metrics on children’s word recognition has not been studied.
Exposure related measures capturing the frequency or likeliness of encountering words in context have been repeatedly shown to be important predictors of word recognition. High frequency words are recognized faster, and by more people, compared to low frequency words (for a review, see Brysbaert et al., Citation2018). The interaction between word frequency and age on word recognition (from age 8–83) indicates that the word frequency effect is stronger for young readers, compared to adult readers (Davies et al., Citation2017). Adelman, Brown, and Quesada (Citation2006) found that contextual diversity is a stronger predictor of word recognition than word frequency, because frequency counts of some words can be affected too much by high frequency of occurrence within a specific context. Contextual diversity captures the probability of encountering a word in any context, and has been repeatedly shown to predict word recognition (Brysbaert & New, Citation2009; De Deyne et al., Citation2013; Keuleers, Brysbaert, & New, Citation2010). Contextual diversity also predicts children’s word recognition (Perea, Soares, & Comesaña, Citation2013). Other measures of exposure have been proposed, the most important ones being age of acquisition of words (Ghyselinck, Lewis, & Brysbaert, Citation2004) and the relatively new characteristic prevalence or subjective frequency of words (Brysbaert et al., Citation2016). Both age of acquisition and prevalence depend on human ratings of the words and may therefore capture a mixture of the raters’ familiarity with the form and the meaning of the word that reflects more than just exposure, whereas corpus frequency and contextual diversity do not. The fact that such rating variables are strong predictors of word recognition might be because they reflect the raters’ familiarity with words. However, they probably do not measure exposure as precisely as corpus-based metrics.
To conclude, many word characteristics, describing characteristics of the word’s form, meaning, and exposure, have been shown to predict word recognition performance. Investigating the effects of word characteristics, whilst controlling for the effect of others, is not trivial as many word characteristics correlate with each other. Therefore, in the present study, we aimed to reduce several observed word characteristics to latent constructs of word form, meaning and exposure.
The present study
Research so far has mainly focused on adult word recognition. Fewer studies have addressed word recognition in school children, and the effect of multiple word characteristics on children’s word recognition accuracy and speed has generally been ignored. Therefore, the present study aimed to investigate to what extent children’s word recognition accuracy (i.e., item difficulty) and speed (i.e., item latency) can be predicted from word form, word meaning, and word exposure characteristics. An attempt was made to find an answer to the following research questions: To what extent can item difficulty be explained from word form, word meaning, and word exposure characteristics? And to what extent can item latency be predicted from item difficulty, on the one hand, and word form, word meaning, and word exposure characteristics, on the other?
In order to address these research questions, we drew a random set of words from a large corpus of words derived from texts written for children across primary school grade levels (Basilex, Tellings, Hulsbosch, Vermeer, & Van den Bosch, Citation2014), and measured the word recognition performance (item difficulty and latency) of children in the upper primary school (grades 3, 4, and 5). Word characteristics were reduced to latent constructs of word form, meaning, and exposure, to be used as predictors of the latencies and item difficulties. In this study, measures of word form included word length, the average phonological Levenshtein distance of the word’s 20 nearest neighbors, and the average frequency of these nearest neighbors; word meaning measures included betweenness, in-degree, and clustering coefficient scores derived from a free association network; and word exposure measures included corpus frequency and contextual diversity.
We expected that item difficulty would be predicted by word form, word meaning, and word exposure; and that word recognition latency would be predicted by item difficulty, word form, word meaning, and word exposure. Juul et al. (Citation2014) have shown that accuracy precedes speed in word reading as reaction times of young children sped up once at least 70% accuracy was reached. Therefore, we hypothesized that, at the word level, item latency was predicted by item difficulty, and that the easier words were responded to faster than more difficult words. We also expected that word form would have an inhibitory effect on word recognition; and that shorter words with nearer, high-frequency neighbors would be read more accurately and have a shorter latency than long words with less near, low-frequency neighbors (consistent with the correlations reported by Yap & Balota, Citation2009). Furthermore, based on the findings by De Deyne et al. (Citation2013) we expected that words with a higher connectedness based on the word meaning measures would be less difficult and have a shorter latency than words with fewer connections. Finally, we expected to find the commonly reported effect that higher frequency words (word exposure) are less difficult and have a shorter latency than lower frequency words (see Brysbaert et al., Citation2018).
Method
Participants
Participants were 1,454 children in grade 3 (n = 456), 4 (n = 478) and 5 (n = 520) of 23 Dutch regular primary schools. The age of the children was between 8 and 13 years old (M = 10.1 years, SD = 11.8 months, 52.4% girls). All children had normal or corrected to normal vision.
Data was collected in Spring 2017; before European GDPR legislation became effective in 2018. At that time, approval of an ethical committee was not yet common practice. However, the current sample was treated in accordance with institutional guidelines and APA ethical standards. Data collection was noninvasive, since it was based on regular educational practices. Schools, parents, and children were informed about the purpose of the research, the expected durations of the tasks, and the procedures. They were informed about whom to contact for questions about the research. Schools gave active consent to participate in the study. Prior to testing, informed passive consent was obtained from the parents of all participating children. Both schools and parents were aware of their right to decline participation and to withdraw from the research any time before or during the research project.
Criterion variables: materials
Stimuli
A list of 300 target words was drawn from the 20,000 lemma list from the Dutch 11.5 million words corpus BasiLex (Tellings et al., Citation2014) that contains texts written for children (i.e., an input corpus). Words were drawn at random from grade-specific subcorpora (grades 2 to 6). The noun, verb, and adjective ratio was roughly the same as in the 20,000 lemma list, the list of 20,000 highest frequency words in the BasiLex corpus that roughly represents the words known at the end of grade 6 (i.e., 62% nouns, 25% verbs, and 13% adjectives; in the BasiLex 20,000 list the distribution is 67%, 20%, and 14%, respectively). The frequency distribution of the 300 target words ranged from 9 to 4,828 and the log transformed frequencies ranged from 0.95 to 3.68 (M = 2.03, SD = 0.64). The frequency distribution of the words indicates that the words represent a broad spectrum of very familiar to fairly unfamiliar words and reflect the variety of words children encounter during their primary school years.
Pseudowords were created by matching 300 other words from the 20,000 BasiLex lemma list to the list of target words based on frequency, word class, and length and altering 1 or 2 letters. This resulted in a list of phonologically and orthographically legal Dutch pseudowords.
Lexical decision task
A digital lexical decision task was created using PsyToolkit (Stoet, Citation2010, Citation2017). At the start of the task the screen entered full-screen mode and children were presented with a black screen. Each trial consisted of a fixation cross presented for 750 ms and after a delay of 750 ms the letter string (target word or pseudoword) appeared on the screen for 5000 ms. Children were instructed to press the letter “A” on the keyboard for a pseudoword and the letter “L” for an existing Dutch word. If a child would press another button or no button at all (within 5000 ms), the response was recorded as an incorrect response.
Procedure
The list of 300 target words (and matching pseudowords) was randomly split into three equally sized parts of 100 target words each, which resulted in three versions of the task. This data collection was part of a larger data collection, with two other word knowledge tasks: a context decision task and a definitional decision task, which measured different aspects of word knowledge of the same 300 target words (see Monster et al., Citation2021). The lexical decision task was the first task that all of the children completed. In order to reduce the burden for schools and children, children received one of these three lists of target words (i.e., a child was presented to the same 100 words in all three tasks). Each version was randomly split into two parts, since the data collection was divided into two sessions. The items presented in session 1 and 2 were counterbalanced across children that received the same version.Footnote1
In the first session, children received a short oral presentation in which the tasks were explained and some examples were given. At the start of the task children received short, written digital instructions and four practice items (two target words and two pseudowords) including feedback based on their response (during the task no feedback was given). The children were presented with new practice items at session 2. The lexical decision task took the children approximately 10– 15 minutes to complete. The full session took one hour to complete with a five-minute break after the second task.
Predictor variables: word characteristics
Form related measures
Word length, measured as the word’s number of letters, was included in the models as one of the form-related measures. Following the methodology of Yap and Balota (Citation2009), Levenshtein distances were computed between each of the target words and the BasiLex lexicon to compute the mean distance to the 20 nearest neighbors and the mean frequency of these nearest neighbors. Levenshtein distance represents the number of insertions, deletions and substitutions of e.g., letters to transform one word into the other. We chose to compute the Levenshtein distance between words using phonemes as the Dutch language contains many bigraphs that form a single phoneme. Dutch children are taught to decode such a bigraph as a single unit (e.g., the Dutch word for book is “boek” and children learn to decode this word as “b” “oe” “k”). To summarize, we computed for each target word the average phonological Levenshtein distance to the 20 nearest neighbors (PLD20) and the mean frequency of these 20 nearest neighbors (PLD20 NF). These measures are an indication of how distinct the phonological form of the word is. The phonological form of words was retrieved from the CELEX database (Baayen, Piepenbrock, & Van Rijn, Citation1993).
Meaning related measures
Using the free association responses by adults collected by De Deyne et al. (Citation2013) and the methodology described in their article, a word association network was created. This network used all three responses that over 70,000 adult participants gave to 12,571 cues in a free association task. In line with De Deyne et al. (Citation2013), a weighted, directed network was created, of which the largest weakly connected component was extracted. The weights between nodes of this network were computed using Maki’s gradient descent algorithm (Citation2008). Three centrality scores were computed from this network: betweenness, in-degree, and clustering coefficient. Betweenness represents the number of times a node is on the shortest paths between other nodes in the network and is computed using the normalized betweenness formula by Freeman (Citation1978).
where n denotes the number of nodes in the graph and B(i) denotes the raw betweenness of node i, the number of shortest paths from node h to j passing through i, divided by the number of shortest paths from h to j, summed for all possible pairs of h and j. According to De Deyne et al. (Citation2013) this measure accounts for age of acquisition effects, since words that are learned at a younger age form the foundations on which new word representations are built (and therefore are on the shortest paths between words). The in-degree measure is the sum of the weighted incoming vertices and has been shown to be the strongest predictor of adult word recognition latency in the study by De Deyne et al. (Citation2013). Clustering coefficient is considered to be a measure of semantic neighborhood size and also captures how tightly connected the neighbors of the node are. This measure was computed using the algorithm by Fagiolo (Citation2007):
where W represents the weighted adjacency matrix, d represents the degrees of the node (tot = all degrees, incoming and outgoing, bilat = the number of bilateral degrees so where there is an edge i → j and j → i).
Exposure related measures
We used the BasiLex (Tellings et al., Citation2014) frequency counts of the words as BasiLex is a corpus of Dutch texts for children and therefore provides a good estimate of word frequency for children. If a word had multiple parts-of-speech, like the word “jong” (young, which can be both a noun and an adjective), the summed frequency was taken. In some cases, word frequency can be influenced by high occurrences of some words in specific texts (Brysbaert & New, Citation2009). Therefore, we also included the SUBTLEX-NL contextual diversity measure from Keuleers et al. (Citation2010), which is a count of the number of films or tv shows the word appeared in.
Plan of analysis
The accuracy scores were analyzed using a Rasch model (Rasch, Citation1960) to assess the scalability of the lexical decision task accuracy responses. Marginal maximum likelihood was used to estimate the item parameters. Response latencies were corrected for the average latency of the child to control for individual differences at the child level as these analyses were conducted at the item level. Only response times to correctly answered items were used to compute the average response time for each item. All measures (dependent measures and word characteristics) were scaled and centered. All word characteristics were also log transformed.
A confirmatory factor analysis was conducted to test whether the eight word characteristics could be reduced to three latent variables of word form, word meaning, and word exposure. Structural equation models were then conducted to assess how these three latent variables predict word recognition item difficulty and item latency. Item difficulty was expressed by the item difficulty parameter from the Rasch model. Item latency was represented by the mean reaction time across all children per item. The confirmatory factor analysis and structural equation models were computed using lavaan package (Rosseel, Citation2012) in R (version 3.6.3, R Core Team, Citation2020).
Results
Descriptive statistics and preliminary analyses
The means and standard deviations of the accuracy and response times of the children on the lexical decision task are presented separately for each grade in .
Table 1. Means and standard deviations of accuracy (out of 100) and latency in milliseconds (max 5000) of responses to target words and pseudowords per grade.
To assess the scalability of word recognition accuracy, the lexical decision accuracy responses were also analyzed with a Rasch model (Rasch, Citation1960). We used a similar approach of assessing model fit as was used by Keuning and Verhoeven (Citation2008). Items with a negative item-rest correlation were removed from the dataset as a negative value is indicative of a bad fitting item. A total of six items were removed (innig, vod, prompt, sperwer, guur, and grimeur. English: intimate, rag, prompt, sparrow, bleak, and stage make-up artist) resulting in a set of 294 items. Model fit was evaluated using the item-specific S-tests and the overall R1c-test (Verhelst & Glas, Citation1995; Verhelst, Glas, & Verstralen, Citation1995). Both tests indicated acceptable fit of the Rasch model to the observed data. The distribution of the S-test p-values was approximately uniform at the interval [0,1] and the R1c contribution was acceptable in light of the number of degrees of freedom (R1c/df = 1.22). The fit of the model was further assessed by verifying that the data meets the properties of item response theory (Hambleton, Swaminathan, & Rogers, Citation1991). If the Rasch model fits the data, invariant item and ability parameter estimates should first be obtained. Invariance of ability parameters was assessed by estimating child ability from two random sets of 50 items. The bivariate plot for the ability estimates showed a linear association and the correlation between the two sets of ability estimates was high, r(1452) = .65, p < .001. Ability parameter invariance was thus obtained with the Rasch model. Invariance of item parameters was assessed by estimating them for each grade separately. presents the correlations between the item parameters for participants in each grade. Collectively, these correlations provide additional support that item parameter invariance was obtained with the Rasch model.
Table 2. Correlations between item parameters given each grade.
Lastly, the accuracy of the model predictions was investigated by analyzing the standardized item residuals. Note that the standardized item residual is the difference between the observed item performance for a group of children and their expected item performance. The expected distribution of standardized item residuals under the assumption of the Rasch model is unknown although one might expect a normal distribution, with mean of 0 and a standard deviation of 1. Rather than assuming a normal distribution, however, an empirical distribution of residuals was simulated to interpret the real distribution of the residuals. This was done by generating response data of 10,000 respondents with a similar ability distribution as our observed data and using the estimated item parameters. This generated data set holds similar properties as our observed data and fits the assumptions of the Rasch model perfectly. If the observed data fit the assumptions of the Rasch model, the real and simulated distributions of the standardized residuals should be highly similar (for more information about this approach, we refer to Keuning & Verhoeven, Citation2008). As can be seen in , the predictions of the Rasch model seem to be accurate.
Figure 1. Overlapping frequency distributions of the observed and simulated standardized item residuals.
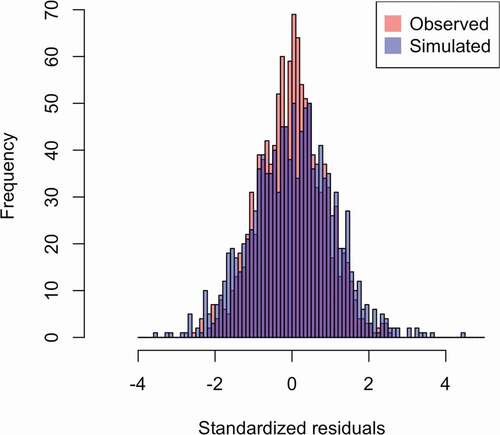
To visualize the (spread of) performance of the children across the grade levels on the lexical decision task, a Wright map was created using the scaled item difficulty and child ability parameters per grade (). As can be seen, children had rather high accuracy scores on the lexical decision task compared to the range of the item difficulty parameters (see also ), but there is a clear spread in scores and item difficulty.
Assessing relations between word characteristics
The lexical decision accuracy responses from children in grades 3, 4 and 5 fit the assumptions of the Rasch model which resulted in a unidimensional scale of item difficulty across the three grades. Therefore, in the following analyses we used the item difficulty parameters across all three grades as our dependent variable. In , descriptives of the two dependent measures (item difficulty and item latency) and the word characteristics are presented.
Table 3. Means, standard deviations and range of difficulty, latency (in milliseconds and corrected for mean latency of the child), and word characteristics.
In , the Rasch item parameter estimates and the latencies are related to the three different categories of word characteristics. From this point onwards, the scaled, centered, and log transformed scores of the word characteristics are used as well as the scaled and centered mean latency (the Rasch model yields scaled and centered item parameters, so item difficulty was not processed further). Additionally, the neighborhood frequency of the 20 nearest neighbors exhibited a negative correlation with the other two word form variables (word length and average phonological Levenshtein distance to the 20 nearest neighbors) and therefore the inverse of this measure was subsequently used (multiplied with −1). The correlations indicate that there are strong to very strong associations between word characteristics within each category (range .70-.90). Correlations between categories are weak (range .20-.38), except for the correlations between the word characteristics of word meaning and word exposure, these are moderate to strong (range .54-.67). These strong within-category correlations and weak-to-moderate correlations between categories are an indication that the word characteristics could be meaningfully divided in three latent constructs of word form, word meaning, and word exposure.
Table 4. Correlations between word characteristics and item difficulty and item latency.
In order to test whether the eight observed measures reflect three latent constructs of word form, word meaning and word exposure, a confirmatory factor analysis was performed. Robust (Huber-White) standard errors were calculated to evaluate parameter estimates, and full information maximum likelihood was used to account for missing values in the word characteristics. The fit of the confirmatory factor analysis and structural equation models was assessed using the root-mean-square error of approximation (RMSEA) which should be lower than .07 (Steiger, Citation2007), the relative chi-squared (ratio of chi-squared and degrees of freedom) which should be lower than 3.0 (Carmines & McIver, Citation1981), and the Comparative Fit Index (CFI) and Tucker-Lewis Index (TLI) which should be higher than .95 (Hu & Bentler, Citation1999). The hypothesized model fit the observed data: χ2(17) = 24.01 (ratio χ2/df = 1.41), RMSEA = 0.04, CFI = 0.995, and TLI = 0.992. The confirmatory factor analysis showed that the eight observed word characteristics could be described by three latent variables representing word form, word meaning, and word exposure.
Form, meaning, and exposure predicting word recognition
After establishing the hypothesized factor structure using the word characteristics, the next step was to use the latent constructs word form, word meaning, and word exposure to predict item difficulty and item latency. Therefore, a structural equation model was performed in order to answer the research question: to what extent can item difficulty and latency be explained from word form, word meaning, and word exposure? We build the model up toward our theoretical assumption that accuracy precedes speed by first predicting item difficulty and latency from word form, meaning and exposure as an intermediate step. Second, we include the regressor of item difficulty predicting item latency as our final model. Without item difficulty predicting item latency we observed a similar result to the final model for form significantly (p < .001) predicting item latency. Meaning does not predict item latency (p = .265), while exposure is a significant (p < .001) predictor of item latency. This model explained 73% of the variance of item latency (R2 = .731).
In our final model, item difficulty and latency (mean reaction time) were both predicted from word form, meaning, and exposure. Furthermore, item latency was predicted from item difficulty. The resulting model showed a good fit to the observed data: χ2(27) = 59.32 (ratio χ2/df = 2.20), RMSEA = 0.066, CFI = 0.986, and TLI = 0.976. presents the standardized regression coefficients from this model and presents the structural equation model including the factor loadings, correlations among latent constructs (in italics), and regression weights.
Table 5. SEM regression estimates.
Figure 3. SEM structure with latent variable structure, factor loadings, correlations among latent constructs, and regression estimates.
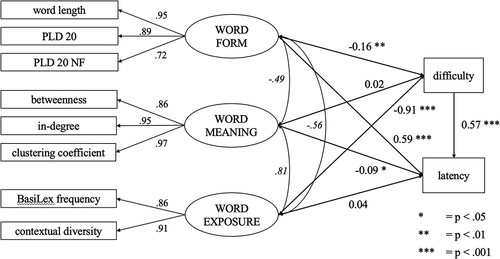
Item difficulty was significantly predicted by word form and exposure. Item latency was
significantly predicted by item difficulty, form, and meaning.
Discussion
The aim of the present study was to investigate how word form, word meaning, and word exposure predict item difficulty and latency of children’s word recognition across the upper primary grades. Children from grades 3, 4, and 5 completed a lexical decision task with a representative sample of 300 words randomly selected from the words they encounter in school. We found evidence for the hypothesis that word form and word exposure predict item difficulty. High frequency, high contextual diversity words were easier to recognize than low frequency, low contextual diversity words. Although word form characteristics and item difficulty were found to be positively associated, word form turned out to be a negative predictor of item difficulty. This might be due to a suppression effect caused by the strong relation between word exposure and item difficulty. Furthermore, contrary to our hypothesis, we did not find a significant effect of word meaning on item difficulty. Based on the moderate correlations between word meaning characteristics, and item difficulty it seems there is a relation between associative connectedness and item difficulty. However, there may not have been enough variance in the item difficulty parameters (due to high response accuracy). When combined with word form and word exposure, the effect of word meaning on item difficulty is too subtle, a finding Yap and Balota (Citation2009) have reported as well.
Regarding word recognition latency, the mean reaction time was significantly predicted by item difficulty, word meaning, and word form. Our hypothesis that item difficulty predicts item latency was supported by the fact that more difficult words were processed slower. The main effect of word meaning on latency showed that more connected words were processed faster, which has been shown in previous studies (De Deyne et al., Citation2013) and can be considered a semantic richness effect often described in the literature (see e.g., Pexman et al., Citation2008). The source of the semantic richness effect was also challenged in simulations with a connectionist network in that a stable activation pattern was evidenced faster in the case of words with richer semantic representations (Plaut & Shallice, Citation1993). Furthermore, we found that short words with a low mean Levenshtein distance to phonological neighbors with a high mean frequency were processed faster compared to long words with a high mean Levenshtein distance to phonological neighbors with a low mean frequency. This finding is in line with findings by Brysbaert et al. (Citation2016).
The findings of the present study have gained new insights in the nature of children’s word recognition accuracy and latency in a single design by combining established effects on children’s (and adult’s) word recognition in a novel manner. The present study included a large set of word characteristics to predict both item difficulty and latency of words children encounter during primary school. The effect of knowledge of form on children’s word processing has often been established (Hulme, Bowyer-Crane, Carroll, Duff, & Snowling, Citation2012; Patel, Snowling, & De Jong, Citation2004), especially for younger children who are still learning to become fluent decoders. With age, the effect of form-related variables on word recognition becomes smaller (Schröter & Schroeder, Citation2017), as a consequence of adopting a lexical search approach to reading as opposed to decoding letter-by-letter.
In our study, a large word exposure effect was observed (on item difficulty and indirectly via item difficulty on item latency). In time, as children reach adulthood, a smaller word exposure effect could be expected. Namely, Davies et al. (Citation2017) found decreasing effects of word frequency and age of acquisition with age with a lexical decision task fit for all ages. However, Schröter and Schroeder (Citation2017) reported a decreasing effect size of word frequency across ages when frequencies were retrieved from a children’s books corpus and an increasing effect size when using adult corpora. The fit between source and frequency range of the target words appears to play a crucial part in the size of the word frequency effect on word recognition.
In the present study, it was evidenced that in the upper primary grades, word meaning is gaining importance in predicting processing speed. It was shown that word centrality in an associative network predicts word recognition latency not only in adults, as previously established, but also in children. This is in line with previous studies that investigated the importance of semantic richness-related variables for children’s word recognition (e.g., Hsiao, Bird, Norris, Pagán, & Nation, Citation2020). In our case, the centrality of words was derived from adult responses on a free association task. Interestingly, Cremer, Dingshoff, De Beer, and Schoonen (Citation2010) showed differences between adult and child association responses, where children’s responses were more dispersed across categories compared to adults. In our study, the centrality metrics were used as a word characteristic and not as a proxy of the connectedness of the word in the child’s mental lexicon. Further research is needed to fully uncover the characteristics of a child word association network compared to an adult network.
It is important to note that our methodology has shown that it is possible to use more generalized concepts of word characteristics by using latent constructs. By doing so, arbitrary choices for a particular measure to avoid collinearity issues do not need to be made. It has also been shown that the scalability of the lexical decision items can be assessed using the Rasch framework. We found that children’s responses on the items across the grades can be described by the Rasch model, indicating one underlying trait of word recognition accuracy. This is in line with earlier work claiming that across grade levels one latent trait of word recognition can be expected to emerge, given that the complexity of words introduced in the school curriculum increases more or less uniformly throughout formal education (Biemiller & Slonim, Citation2001).
A limitation of this study is that scalability of word recognition has not been established across all primary grades. Future research could assess scalability of word recognition starting from the early grades. As young readers are still developing their decoding skills, it is interesting to find out to what extent a latent trait of word recognition across all primary grades would also emerge. A second limitation of the present study is the cross-sectional design which makes it difficult to make claims about how word recognition develops. The descriptive statistics per grade indicate a larger gain in accuracy between grade 3–4 (compared to the gain in accuracy between 4–5), whereas the gain in speed is larger from grade 4–5 compared to grade 3–4. These differences were not further investigated, but are in line with Juul et al. (Citation2014) who argue that accuracy precedes speed in word recognition development. Furthermore, the present study has measured word form, word meaning, and word exposure using relevant word characteristics that capture a large part of each construct whereas other measures could be added, such as number of senses and semantic diversity. In defining our model, we did not have the power to contrast our three-dimensional model with a two-dimensional model, combining word meaning and word exposure given the high correlations between these word characteristics. Given these limitations, the present study can only be seen as a first step in explaining word recognition accuracy and latency in children.
To conclude, the present study has provided empirical evidence that the word recognition accuracy and latency of words children encounter during primary school can be predicted from latent constructs of word form, meaning and exposure. More specifically, word form and exposure predict word recognition item difficulty, and item difficulty, word form, and meaning predict word recognition item latency.
Supplemental Material
Download Zip (21.5 KB)Disclosure statement
No potential conflict of interest was reported by the author(s).
Supplementary material
Supplemental data for this article can be accessed on the publisher’s website.
Notes
1. We checked if the three versions of the lexical decision task were similar using one-way ANOVAS to test if the means of the eight word characteristics, mean item difficulty, and mean item latency significantly differed. None of these were statistically significant (all p’s > .20).
References
- Adelman, J. S., Brown, G. D., & Quesada, J. F. (2006). Contextual diversity, not word frequency, determines word-naming and lexical decision times. Psychological Science, 17(9), 814–823. doi:10.1111/j.1467-9280.2006.01787.x
- Andrews, S. (1997). The effect of orthographic similarity on lexical retrieval: Resolving neighborhood conflicts. Psychonomic Bulletin & Review, 4(4), 439–461. doi:10.3758/BF03214334
- Baayen, R. H., Piepenbrock, R., & Van Rijn, H. (1993). The celex lexical database. Technical report, Linguistic Data Consortium. Philadelphia: University of Pennsylvania.
- Balota, D. A., Cortese, M. J., Sergent-Marshall, S. D., Spieler, D. H., & Yap, M. J. (2004). Visual word recognition of single-syllable words. Journal of Experimental Psychology. General, 133(2), 283–316. doi:10.1037/0096-3445.133.2.283
- Biemiller, A., & Slonim, N. (2001). Estimating root word vocabulary growth in normative and advantaged populations: Evidence for a common sequence of vocabulary acquisition. Journal of Educational Psychology, 93(3), 498–520. doi:10.1037/0022-0663.93.3.498
- Brysbaert, M., & Cortese, M. J. (2011). Do the effects of subjective frequency and age of acquisition survive better word frequency norms? Quarterly Journal of Experimental Psychology, 64(3), 545–559. doi:10.1080/17470218.2010.503374
- Brysbaert, M., Mandera, P., & Keuleers, E. (2018). The word frequency effect in word processing: An updated review. Current Directions in Psychological Science, 27(1), 45–50. doi:10.1177/0963721417727521
- Brysbaert, M., & New, B. (2009). Moving beyond Kučera and Francis: A critical evaluation of current word frequency norms and the introduction of a new and improved word frequency measure for American English. Behavior Research Methods, 41(4), 977–990. doi:10.3758/BRM.41.4.977
- Brysbaert, M., Stevens, M., Mandera, P., & Keuleers, E. (2016). The impact of word prevalence on lexical decision times: Evidence from the Dutch Lexicon project 2. Journal of Experimental Psychology. Human Perception and Performance, 42(3), 441–458. doi:10.1037/xhp0000159
- Carmines, E. G., & McIver, J. P. (1981). Analyzing models with unobserved variables: Analysis of covariance structures. In G. W. Bohrnstedt & E. F. Borgatta (Eds.), Social measurement (pp. 65–116). Beverly Hills, CA: Sage.
- Castles, A., Davis, C., Cavalot, P., & Forster, K. (2007). Tracking the acquisition of orthographic skills in developing readers: Masked priming effects. Journal of Experimental Child Psychology, 97(3), 165–182. doi:10.1016/j.jecp.2007.01.006
- Castles, A., & Nation, K. (2006). How does orthographic learning happen? In S. Andrews (Ed.), From inkmarks to ideas: Current issues in lexical processing (pp. 151–179). Hove, England: Psychology Press. doi:10.4324/9780203841211
- Castles, A., Rastle, K., & Nation, K. (2018). Ending the reading wars: Reading acquisition from novice to expert. Psychological Science in the Public Interest, 19(1), 5–51. doi:10.1177/1529100618772271
- Cremer, M., Dingshoff, D., De Beer, M., & Schoonen, R. (2010). Do word associations assess word knowledge? A comparison of L1 and L2, child and adult word associations. International Journal of Bilingualism, 15(2), 187–204. doi:10.1177/1367006910381189
- Davies, R. I., Arnell, R., Birchenough, J. H., Grimmond, D., Houlson, S., & Greene, R. L. (2017). Reading through the life span: Individual differences in psycholinguistic effects. Journal of Experimental Psychology. Learning, Memory, and Cognition, 43(8), 1298–1338. doi:10.1037/xlm0000366
- De Deyne, S., Navarro, D. J., & Storms, G. (2013). Better explanations of lexical and semantic cognition using networks derived from continued rather than single-word associations. Behavior Research Methods, 45(2), 480–498. doi:10.3758/s13428-012-0260-7
- Dufau, S., Lété, B., Touzet, C., Glotin, H., Ziegler, J. C., & Grainger, J. (2010). A developmental perspective on visual word recognition: New evidence and a self-organising model. European Journal of Cognitive Psychology, 22(5), 669–694. doi:10.1080/09541440903031230
- Fagiolo, G. (2007). Clustering in complex directed networks. Physical Review E, 76(2). doi:10.1103/PhysRevE.76.026107
- Freeman, L. C. (1978). Centrality in social networks conceptual clarification. Social Networks, 1(3), 215–239. doi:10.1016/0378-8733(78)90021-7
- Frishkoff, G. A., Perfetti, C. A., & Collins-Thompson, K. (2010). Lexical quality in the brain: ERP evidence for robust word learning from context. Developmental Neuropsychology, 35(4), 376–403. doi:10.1080/87565641.2010.480915
- Ghyselinck, M., Lewis, M. B., & Brysbaert, M. (2004). Age of acquisition and the cumulative-frequency hypothesis: A review of the literature and a new multi-task investigation. Acta Psychologica, 115(1), 43–67. doi:10.1016/j.actpsy.2003.11.002
- Hambleton, R. K., Swaminathan, H., & Rogers, H. J. (1991). Fundamentals of item response theory. Newbury Park, CA: Sage.
- Herdağdelen, A., & Marelli, M. (2017). Social media and language processing: How Facebook and Twitter provide the best frequency estimates for studying word recognition. Cognitive Science, 41(4), 976–995. doi:10.1111/cogs.12392
- Hoedemaker, R. S., & Gordon, P. C. (2017). The onset and time course of semantic priming during rapid recognition of visual words. Journal of Experimental Psychology. Human Perception and Performance, 43(5), 881–902. doi:10.1037/xhp0000377
- Hsiao, Y., Bird, M., Norris, H., Pagán, A., & Nation, K. (2020). The influence of item-level contextual history on lexical and semantic judgments by children and adults. Journal of Experimental Psychology. Learning, Memory, and Cognition, 46(12), 2367. doi:10.1037/xlm0000795
- Hsiao, Y., & Nation, K. (2018). Semantic diversity, frequency and the development of lexical quality in children’s word reading. Journal of Memory and Language, 103, 114–126. doi:10.1016/j.jml.2018.08.005
- Hu, L. T., & Bentler, P. M. (1999). Cutoff criteria for fit indexes in covariance structure analysis: Conventional criteria versus new alternatives. Structural Equation Modeling: A Multidisciplinary Journal, 6(1), 1–55. doi:10.1080/10705519909540118
- Hulme, C., Bowyer-Crane, C., Carroll, J. M., Duff, F. J., & Snowling, M. J. (2012). The causal role of phoneme awareness and letter-sound knowledge in learning to read: Combining intervention studies with mediation analyses. Psychological Science, 23(6), 572–577. doi:10.1177/0956797611435921
- Johns, B. T., Dye, M., & Jones, M. N. (2016). The influence of contextual diversity on word learning. Psychonomic Bulletin & Review, 23(4), 1214–1220. doi:10.3758/s13423-015-0980-7
- Jones, M. N., Dye, M., & Johns, B. T. (2017). Context as an organizing principle of the lexicon. In B. H. Ross Ed., Psychology of learning and motivation (Vol. 67, pp. 239–283). Cambridge, MA: Academic Press doi:10.1016/bs.plm.2017.03.008
- Juul, H., Poulsen, M., & Elbro, C. (2014). Separating speed from accuracy in beginning reading development. Journal of Educational Psychology, 106(4), 1096–1106. doi:10.1037/a0037100
- Karageorgos, P., Müller, B., & Richter, T. (2019). Modelling the relationship of accurate and fluent word recognition in primary school. Learning and Individual Differences, 76, 101779. doi:10.1016/j.lindif.2019.101779
- Keuleers, E., Brysbaert, M., & New, B. (2010). SUBTLEX-NL: A new measure for Dutch word frequency based on film subtitles. Behavior Research Methods, 42(3), 643–650. doi:10.3758/BRM.42.3.643
- Keuning, J., & Verhoeven, L. (2008). Spelling development throughout the elementary grades: The Dutch case. Learning and Individual Differences, 18(4), 459–470. doi:10.1016/j.lindif.2007.12.001
- Maki, W. S. (2008). A database of associative strengths from the strength-sampling model: A theory-based supplement to the Nelson, McEvoy, and Schreiber word association norms. Behavior Research Methods, 40(1), 232–235. doi:10.3758/BRM.40.1.232
- Marinus, E., & de Jong, P. F. (2010). Size does not matter, frequency does: Sensitivity to orthographic neighbors in normal and dyslexic readers. Journal of Experimental Child Psychology, 106(2–3), 129–144. doi:10.1016/j.jecp.2010.01.004
- Martens, V. E., & de Jong, P. F. (2006). The effect of word length on lexical decision in dyslexic and normal reading children. Brain and Language, 98(2), 140–149. doi:10.1016/j.bandl.2006.04.003
- Meara, P., & Wolter, B. (2004). V_Links: Beyond vocabulary depth. Angles on the English Speaking World, 4, 85–96.
- Monster, I., Tellings, A., Burk, W. J., Keuning, J., Segers, E., & Verhoeven, L. (2021). Assessing children’s incremental word knowledge in the upper primary grades. Language Testing, 38(4), 536–557. doi:10.1177/0265532220961541
- Nation, K., & Castles, A. (2017). Putting the learning into orthographic learning. In K. Cain, D. L. Compton, and R. K. Parilla (Eds.), Theories of reading development (pp. 147–168). Amsterdam, The Netherlands: John Benjamins Publishing Company. doi:10.1075/swll.15
- Nation, K. (2017). Nurturing a lexical legacy: Reading experience is critical for the development of word reading skill. NPJ Science of Learning, 2(1), 3. doi:10.1038/s41539-017-0004-7
- Nelson, D. L., McEvoy, C. L., & Schreiber, T. A. (2004). The university of South Florida free association, rhyme, and word fragment norms. Behavior Research Methods, Instruments and Computers, 36(3), 402–407. doi:10.3758/BF03195588
- New, B., Ferrand, L., Pallier, C., & Brysbaert, M. (2006). Reexamining the word length effect in visual word recognition: New evidence from the english lexicon project. Psychonomic Bulletin & Review, 13(1), 45–52. doi:10.3758/BF03193811
- Patel, T. K., Snowling, M. J., & de Jong, P. F. (2004). A cross-linguistic comparison of children learning to read in English and Dutch. Journal of Educational Psychology, 96(4), 785–797. doi:10.1037/0022-0663.96.4.785
- Perea, M., Soares, A. P., & Comesaña, M. (2013). Contextual diversity is a main determinant of word identification times in young readers. Journal of Experimental Child Psychology, 116(1), 37–44. doi:10.1016/j.jecp.2012.10.014
- Perfetti, C. (2017) Lexical quality revisited. In E. Segers, E., and Van den Broek, P. (Eds.), Developmental Perspectives in Written Language and Literacy: In Honor of Ludo Verhoeven, 51–68.Amsterdam, The Netherlands: John Benjamnins Publishing Company.
- Pexman, P. M., Hargreaves, I. S., Siakaluk, P. D., Bodner, G. E., & Pope, J. (2008). There are many ways to be rich: Effects of three measures of semantic richness on visual word recognition. Psychonomic Bulletin & Review, 15(1), 161–167. doi:10.3758/PBR.15.1.161
- Pexman, P. M. (2012). Meaning-based influences on visual word recognition. In J. S. Adelman (Ed.), Current issues in the psychology of language. Visual word recognition: Meaning and context, individuals and development (pp. 24–43). New York, NY: Psychology Press.
- Plaut, D. C., & Shallice, T. (1993). Deep dyslexia: A case study of connectionist neuropsychology. Cognitive Neuropsychology, 10(5), 377–500. doi:10.1080/02643299308253469
- R Core Team. (2020). R: A language and environment for statistical computing. R Foundation for Statistical Computing, Vienna, Austria. Retrieved from https://www.R-project.org
- Rasch, G. (1960). Probabilistic models for some intelligence and attainment tests. Copenhagen, Denmark: Danish Institute for Educational Research.
- Rastle, K. (2016). Visual word recognition. In Hickok, G., and Small, S. L. Neurobiology of language. (pp. 255–264). San Diego, CA: Academic Press. doi:10.1016/C2011-0-07351-9
- Reichle, E. D., & Perfetti, C. A. (2003). Morphology in word identification: A word experience model that accounts for morpheme frequency effects. Scientific Studies of Reading, 7(3), 219–238. doi:10.1207/S1532799XSSR0703_2
- Richter, T., Isberner, M. B., Naumann, J., & Neeb, Y. (2013). Lexical quality and reading comprehension in primary school children. Scientific Studies of Reading, 17(6), 415–434. doi:10.1080/10888438.2013.764879
- Rosseel, Y. (2012). Lavaan: An R package for structural equation modeling and more. Version 0.5–12 (BETA). Journal of Statistical Software, 48(2), 1–36. doi:10.18637/jss.v048.i02
- Schröter, P., & Schroeder, S. (2017). The developmental lexicon project: A behavioral database to investigate visual word recognition across the lifespan. Behavior Research Methods, 49(6), 2183–2203. doi:10.3758/s13428-016-0851-9
- Schröter, P., & Schroeder, S. (2018). Differences in visual word recognition between L1 and L2 speakers: The impact of length, frequency, and orthographic neighborhood size in german children. Studies in Second Language Acquisition, 40(2), 319–339. doi:10.1017/S0272263117000201
- Share, D. L. (1995). Phonological recoding and self-teaching: Sine qua non of reading acquisition. Cognition, 55(2), 151–218. doi:10.1016/0010-0277(94)00645-2
- Share, D. L. (2004). Orthographic learning at a glance: On the time course and developmental onset of self-teaching. Journal of Experimental Child Psychology, 87(4), 267–298. doi:10.1016/j.jecp.2004.01.001
- Steiger, J. H. (2007). Understanding the limitations of global fit assessment in structural equation modeling. Personality and Individual Differences, 42(5), 893–898. doi:10.1016/j.paid.2006.09.017
- Stoet, G. (2010). PsyToolkit - A software package for programming psychological experiments using Linux. Behavior Research Methods, 42(4), 1096–1104. doi:10.3758/BRM.42.4.1096
- Stoet, G. (2017). PsyToolkit: A novel web-based method for running online questionnaires and reaction-time experiments. Teaching of Psychology, 44(1), 24–31. doi:10.1177/0098628316677643
- Tellings, A., Hulsbosch, M., Vermeer, A., & Van den Bosch, A. (2014). BasiLex: An 11.5 million words corpus of Dutch texts written for children. Computational Linguistics in the Netherlands Journal, 4, 191–208.
- Van den Boer, M., De Jong, P. F., & Haentjens-van Meeteren, M. M. (2012). Lexical decision in children: Sublexical processing or lexical search? Quarterly Journal of Experimental Psychology, 65(6), 1214–1228. doi:10.1080/17470218.2011.652136
- Verhelst, N. D., Glas, C. A. W., & Verstralen, H. H. F. M. (1995). OPLM one parameter model computer program and manual. Arnhem, Cito.
- Verhelst, N. D., & Glas, C. A. W. (1995). The one parameter logistic model. In Fischer, G. H., and Molenaar, I. W. Rasch models. (pp. 215–237). New York, NY: Springer.
- Yap, M. J., & Balota, D. A. (2009). Visual word recognition of multisyllabic words. Journal of Memory and Language, 60(4), 502–529. doi:10.1016/j.jml.2009.02.001
- Yap, M. J., Pexman, P. M., Wellsby, M., Hargreaves, I. S., & Huff, M. (2012). An abundance of riches: Cross-task comparisons of semantic richness effects in visual word recognition. Frontiers in Human Neuroscience, 6, 6. doi:10.3389/fnhum.2012.00072
- Yarkoni, T., Balota, D., & Yap, M. (2008). Moving beyond Coltheart’s N: A new measure of orthographic similarity. Psychonomic Bulletin & Review, 15(5), 971–979. doi:10.3758/PBR.15.5.971