Abstract
With the increasing concern about global warming, the estimation of the CO2 sink capacity of the biosphere has become one of the main issues of remote sensing studies. This paper discusses the estimation of CO2 storage capacity in forests using airborne light detection and ranging (lidar) data. To achieve the correct biomass estimation, a processing chain consisting of the bare earth digital terrain model (DTM) extraction and individual tree crown segmentation has been constructed and applied to the dense airborne lidar data. As a result of the sample processing, the reliable bare earth DTM and tree boundaries were extracted in the study area with an overall accuracy of 71%. The total estimated CO2 storage capacity in the study area using the extracted tree crown structures is accurate, with only a 7% error level compared to field measurements.
Introduction
Global warming is a significant environmental threat that is primarily being caused by greenhouse gas (GHG) emissions from anthropogenic activities such as the increased use of fossil fuels over the past century (IPCC Citation2007). Thus, the United Nations Environment Programme (UNEP) and the World Meteorological Organization (WMO) established the Intergovernmental Panel on Climate Change (IPCC) in 1988 to provide comprehensive scientific assessments of climate change and its potential impacts. Under the international environmental treaty called the United Nations Framework Convention on Climate Change (UNFCCC), which took effect in 1994, the Kyoto Protocol was issued in 1997 as the most predominant action plan for reducing GHG emissions worldwide.
Forests play an important role in regulating the balance of GHGs in the atmosphere not only as a sink for GHG emissions due to their use of CO2 in photosynthesis, but also as a source for CO2 emissions via wildfires. As such, estimations of the extent of forest resources, particularly in terms of biomass, have been attempted in a large number of scientific surveys. In spite of the growing need to measure forest biomass, existing approaches usually depend on field surveys that are labor-intensive, costly, and time-consuming to implement. Moreover, the difficulty in assessing the biomass objectively is not easily addressed even with a highly detailed survey, as the method is likely to contain error originating from subjective judgments, overlap, and omissions during the field measurements in many sampling areas.
It has been documented that airborne light detection and ranging (lidar) is superior in terms of accuracy for investigating forestry as compared with the other technologies, such as aerial photos and interferometric synthetic aperture radar (InSAR) (Baltsavias Citation1999; Hyyppä et al. Citation2000). The use of lidar surveys has proven to be extremely valuable in the generation of high spatial resolution digital elevation models (DEMs) and for some applications such as building extraction in urban environments. Naturally, lidar is also a very valuable tool for use in forestry applications, especially considering that it has a high spatial resolving power that enables it to produce dense 3-D point clouds that can identify individual tree crowns. Indeed, it is currently the most effective tool to assess forest biomass after the suitable algorithms have been applied.
In this study, we developed an efficient method, based on the IPCC's Good Practice Guideline (GPG), for estimating forest biomass and CO2 stocks using lidar data. First, we applied a filtering step for classifying terrain and vegetation lidar points, then a digital terrain model (DTM) and a digital surface model (DSM) were established. Next, a canopy height model (CHM), which enabled the counting of individual trees in a certain area was used, and tree parameters such as the tree height, crown diameter, and 3-D crown shape, was established by consecutively mining the data with machine vision techniques. Finally, empirical regression equations were used to estimate other forest parameters such as tree volume, diameter at breast height (DBH), and biomass, which is reflective of the CO2 stocks associated with the forest. Our research is different from previous studies in terms of the algorithms that were employed. We used algorithms that employ an iterative split-and-merge technique to maximize the overall detection efficiencies for tree crown numbers and shapes. Thus, the error range of the estimated biomass was reduced by the use of such an iterative machine vision method. In addition to making algorithmic improvements aimed at achieving better accuracy, the regression model that was used to extract the biomass data from the detected tree crown information was optimized by comparing with ground reference data. Hence, this study implemented an all-in-one processing chain consisting of DTM filtering, tree crown delineation, and regression modeling techniques to extract biomass data from lidar point clouds.
The first section of this paper is devoted to a review of biomass estimation based on lidar data. The algorithms used to develop the CHM and estimate biomass are then introduced. Finally, the lidar assessment results are described along with the ground reference data that were acquired through comprehensive field measurements. Overall, this work describes a quantitative method for extracting forest biomass and CO2 stock information from small-footprint topographic lidar data.
Biosmass estimation by remote sensor data
The biomass in a vegetation canopy consists of two parts: the above ground biomass (AGB) and the below ground biomass (BGB). Because of the technical difficulty associated with establishing precise measurements of BGB, AGB has become the primary focus of biosphere research. At first, field measurements were proposed as being the most reliable approach for estimating AGB. However, field-based measurements of total biomass over nationwide scales are cost-prohibitive. Alternatively, GIS-based approaches that employ a variety of auxiliary spatial data, such as on the elevation, slope, soil, and precipitation, may be applicable for obtaining indirect estimates of biomass as was shown in Brown and Gaston (Citation1995). Even though GIS-based approaches show potential for estimating biomass over extensive global target areas, there are some unavoidable uncertainties associated with the use of these types of biomass estimates. For example, the correlation between the auxiliary spatial data and the growth of the vegetation may be weak. In consideration of the problems associated with GIS-based approaches, the exploitation of remotely sensed data sets has been proposed as the most practical means for estimating biomass. Since remote sensing data can be acquired quickly and at low cost, the application of this data to determine biomass over large target areas has many merits (such as quicker and less expensive biomass estimation) compared to the other approaches described above. Moreover, recent improvements in the temporal, spectral, and spatial resolving power of contemporary remotely sensed data have allowed for the development of reliable biomass products that can fulfill socio-economic requirements for obtaining accurate and timely data regarding climate change.
The remote sensing approaches that have been used to estimate biomass can be roughly classified into two categories: the direct measurement of geometric tree parameters and the indirect estimation of biomass through physical properties such as spectral responses (Fraser and Li Citation2002; Dong et al. Citation2003), image textures (Lu and Batistella Citation2005), and electromagnetic backscattering coefficients (Luckman et al. Citation1998; Austin, Mackey, and Van Niel Citation2003). Both medium (<100 m) and low-resolution (1 km) optical images and synthetic aperture radar (SAR) products are frequently employed in the indirect approaches because biomass extraction over an extensive target area is only feasible with either SAR and/or spaceborne optical imagery. In the indirect approach, regressions between biomass and the remotely sensed physical properties, such as spectral responses, reflectance, and backscattering, have been explored with various mathematical techniques such as multiple regression analysis (Zheng et al. Citation2004), the k-nearest neighbor approach (Fazakas, Nilsson, and Olsson Citation1999), and neural network analysis (Fraser and Li Citation2002). Despite these advances, the indirect approaches with medium and low spatial resolution remote sensing data have frequently failed to attain sufficiently high data accuracy for various reasons including data saturation, low signal-to-noise ratios, and calibration issues associated with the employed data sets.
In contrast, the direct extraction of individual tree information from high-resolution remote sensing data sets, such as those from aerial photos (Tiwari and Singh Citation1984), IKONOS satellite images (Thenkabail et al. Citation2004; Kim and Muller Citation2011), and airborne lidar (Popescu, Wynne, and Nelson Citation2003; Popescu Citation2007), has become more popular in biomass studies due to the intrinsically high accuracy of the data sets and recent improvements in the temporal and spatial resolution of commercial remote sensor data.
The accuracy of biomass estimates derived from direct tree delineation methods, especially those that employ lidar, is highly dependent on the algorithmic bases that are used. For example, a comparison study of lidar tree crown detection methods conducted by Kaartinen et al. (Citation2012) showed accuracies ranging from 20% to 102% in terms of the detected tree numbers. Along with the tree numbers, the accuracy of the data for tree crown diameters can significantly affect estimates of biomass as shown in Popescu, Wynne, and Nelson (Citation2003). In addition, the height accuracy of lidar points, which depends on the type of landcover (Hodgson et al. Citation2003; Hodgson and Bresnahan Citation2004), could also be a factor that significantly influences biomass estimates when studies employ a direct approach to data collection. Due to these potential sources of inaccuracy in the resulting biomass data, an alternative method was developed that uses the canopy height distribution and the quintile function of lidar points to implement a scale invariant model for biomass estimation (Zhao, Popescu, and Nelson Citation2009).
It should be noted that the acquisition of high spatial resolution data sets that cover meaningful target areas is not always feasible for biomass applications due to higher costs and time constraints. Furthermore, the extraction of biomass data from high spatial resolution remotely sensed data may require complicated machine vision processing and consequently, high computer processing capabilities. Biomass estimation based on tree crown detection with airborne lidar should be developed using robust machine vision algorithms to extract bare earth DTMs as shown in Raber et al. (Citation2002) and tree crown splits, which have not yet been fully implemented in commercial software solutions.
An effective strategy that could help alleviate the problems associated with estimating biomass using remotely sensed data would be to employ the direct and indirect methods together. For example, biomass extracted from high-resolution remote sensing data over some test sites can be employed in the less computationally demanding indirect regression procedures. From past studies, it is clear that lidar processing has the highest level of accuracy among all of the high spatial resolution direct approaches for biomass estimation. This is because the vertical and horizontal tree crown structures are correctly retrieved with lidar. Therefore, it is worthwhile to develop full procedures to extract highly accurate biomass estimates from lidar point clouds. Using such a procedure, highly practical CO2 sink measurements will be achievable.
As reviewed here, the merits of direct biomass estimation methods, especially using lidar, can be fully exploited under these conditions: (1) the algorithms used to delineate tree crowns must be accurate enough so that tree numbers as well as other parameters such as diameter can be retrieved correctly, (2) the employed height or the image data sets should be accurate enough for the application of algorithms, and (3) the regression model used to convert the extracted tree crown parameters into biomass has to be correctly constructed. It is anticipated that a comprehensive processing chain combining the DTM construction, tree crown detection, and the regression model of this study together with its assessment may provide a framework for future biomass estimation applications.
Methods
As presented here, a key goal of biomass estimation employing lidar is to obtain high accuracy in tree extraction. With that in mind, this study focused on the establishment of reliable DTM filtering and tree crown split algorithms. Prior to the description of the lidar data processing algorithm, the study area, data sets, and field survey to collect the ground reference data are described. Then the interpretation methods of the lidar data for the biomass estimation, consisting of DTM extraction, CHM generation, and individual tree crown segmentation, are presented.
Study area and data sets description
The study area is a part of the National Arboretum, which is located in Pocheon-si, Gyunggi-do in South Korea (upper-left: 37° 44′ 52.87″ N, 127° 9′ 50.74″ E; lower-right: 37° 44′ 56.77″ N; 127° 9′ 39.41″ E based on WGS84). This area is composed of nearly all Needle Fir (Abies holophylla MAX) and even-aged forests. The elevation of the area varies from 108 m to 139 m above the ellipsoidal height. The lidar data used in this study were acquired in April 2007 using the ALTM 3070 system. The return pulses of the lidar data were classified as first returns, last returns, and unique returns. shows more detailed information regarding the lidar data set employed in this study.
Table 1. Statement of used lidar data
Field measurements for the establishment of ground reference
The field work was carried out in July 2011 to generate the ground reference. In this work, we demarcated the boundary line using a static GPS (Trimble 5700) and a Total Station (Topcon GTS 750), and measured the position, height, and DBH of each of the trees in five sampling areas (≈400 m2). shows the demarcated boundary and locations of the sampling areas.
The locations of each tree were calculated using Euclidean distance and azimuth from the observation point. The tree heights were measured using clinometers (Tandem Suunto) and the DBH of each tree were measured using diameter tapes. The descriptive information of the tree observation is shown in .
Table 2. Collected tree information by field measurements
DTM and CHM generation
The filtering procedure of the lidar data is essential to generate the DTM, and consequently, the CHM. In this study, the Hierarchical Robust Interpolation algorithm, which works in a coarse to fine approach using data pyramids and a linear prediction (Kraus and Pfeifer Citation1998), was employed to establish the DTM. The algorithm was implemented using MATLAB to ensure compatibility with other procedures. The filtering processes were conducted thorough 3 pyramidal stages, and then the final DTM with a regular grid with 0.5 m grid spacing was generated (). The obvious improvement of the DTM quality in each pyramidal processing stage proved the effectiveness of Kraus and Pfeifer's approach, even in complicated topography.
Figure 2. Generated DTM in the process of lidar filtering: (a) the coarse DTM with 10 m resolution from step 1, (b) the DTM with 2 m resolution from step 2, (c) the fine DTM with 0.5 m resolution from step 3, (d) the final DTM.
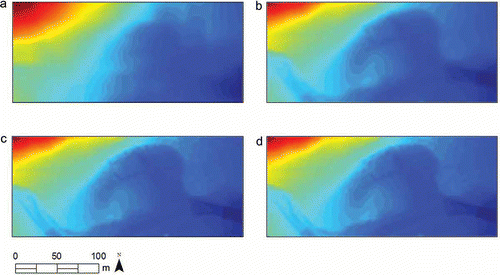
The DSM used to create the CHM for single tree detections was established using the first return pulse from the lidar data. Since the high point density of lidar in this study (4.4 points/m2) remains the conical peaks not only in a center of tree crown but also in branches, it consequently produced the overestimated tree numbers in the application of detection algorithms. In our experience, resampled density up to 1 point/m2 with kriging is ideal to apply the algorithms and quicken the operations. Thus, in order to describe the smoothing crown shape, the lidar data was resampled to 1 point/m2 density before the interpolation was done by using the ordinary kriging method with the linear variogram model of slope 1.0.
The CHM was derived simply by subtracting the DTM from the DSM (Figure 3). For that reason, the accuracy of the CHM is influenced by the accuracies of the DTM and the DSM.
Extraction of individual tree crown
In order to improve the overall accuracy of biomass estimation procedures, highly accurate tree crown segmentation is a prerequisite. Many algorithms to delineate the tree crown from 3-D point clouds and/or an auxiliary optical image have been proposed using various machine vision approaches, such as the local maximum filter (Chen et al. Citation2006; Koch, Heyder, and Weinacker Citation2006), valley following (Gougeon Citation1995; Leckie et al. Citation2003), and template matching (Korpela et al. Citation2007). In spite of such a large number of algorithms available, the existing tree delineation methodologies generally do not provide adequate solutions for over-/under-segmentation, which may result in incorrect biomass estimations in the approaches employing direct tree crown extraction. The watershed segmentation algorithm that is an approach to segment the gray level intensity image employing the flow over the intensity relief have been frequently employed with an auxiliary approach, such as a user-specified marker to prevent the over-segmentation (Najman and Schmitt Citation1996; Wang, Gong, and Biging Citation2004 Citation). However, that is not applicable for lidar data sets of forests in which the individual trees are densely attached. Therefore, we have tackled the over-/under-segmentation problems by introducing iterative split-merge routines following the initial watershed segmentation. The overall algorithms for tree crown segmentation are shown in .
At first, watershed segmentation was employed in order to split the valleys between tree crowns corresponding to step (a) of . As an auxiliary mechanism to suppress over-segmentation, we introduced an evaluation function considering the tree crown morphology. The fitness values of the tree crowns in this study are shown in EquationEquation (1)(1):
If the fitness value is below the threshold and the size of the tree crown is larger than the pre-defined limit (0.3 in this case), it is over-segmented case as shown in step (b) of . Thus, further segmentation is applied by multiplying vertical exaggeration parameters (1.5 in this case) by the height value of the target prototype tree crown which is corresponding to step (c) of . After further segmentation has been done, the overlapping relationship of the initial tree crown model, which is produced from the ellipse fitting (Fitzgibbon, Pilu, and Fisher Citation1999) from individual segments, is evaluated (step (d) of ). Then two target tree crowns are merged, only if the following two conditions are satisfied: (1) the overlapping ratio is larger than the predefined critical value (0.4 in this case); (2) the fitness cost of the candidate segments is higher than the average fitness cost of two target segments (step (e) of ). Hereby, the employed parameters were chosen empirically for minimizing over-/under-segmented components of this research but it will need more case studies to find robust values applicable for various tree allometry.
Iterative procedures based on such splits and merges are performed until the further split-and-merge procedure is stopped shown in step (f) of . The evolution of the tree crown throughout the iterations is shown in .
Figure 5. The tree crown evaluation in each iteration stage: (a) the first iteration stage, (b) the second iteration stage, (c) the final iteration stage. Note the change of segment within uniform point clouds.
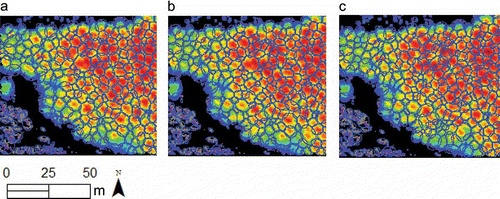
In the next stage, the gavages remaining from the split-and-merge operations were cleaned up by simple deletion or by assignment to the nearest tree crowns according to their size corresponding to step (g) of . Finally, in each segment, the circle and ellipse models are fitted again shown in step (h) of .
The final fitted tree crowns are shown in and clearly prove that the algorithms effectively delineate the valleys between tree crowns, minimizing the over- and under-segmentation. The method is also effective for the delineation of the irregular shapes frequently observed in the mixed forests, as shown in .
Figure 7. Tree crown fitting results in mixed forests; note the algorithms work well in mixed forest (a) as well as deciduous forest (b).
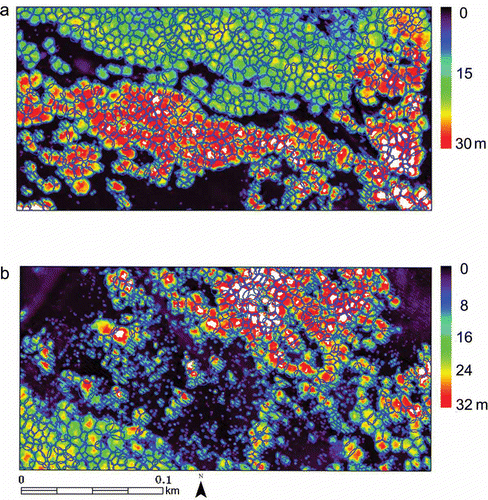
Unlike the tree heights, the DBH information of the tree cannot be measured directly using the lidar data. Therefore, in this study, we calculated the DBH of each tree by means of the six DBH-height equations, which were derived from the field measurements of the sampling area. Among those, the parabolic equation was employed to determine the DBH, because that equation has the highest coefficient of determination (R 2) of 0.7574 (see where R 2 = 0.7574).
Calculation of the biomass and CO2 storage capacity
The process of estimating the CO2 stocks within the forest is shown in .
First, the stem volume should be calculated from the tree height and DBH. Since the breast height form factors of the tree species were determined through experiments, the stem volume (SV) has been formulated simply by EquationEquation (2)(2) (Brouard Citation2009).
The first parameter required for converting the biomass into tons of CO2 is the basic wood density (BWD), which means the weight per volume, including the air gap and moisture. A study for the BWD estimation with a variety of tree species was conducted by the Korea Forest Research Institute, and indicated that the average wood densities of the conifer and deciduous trees in Korea are 0.47 and 0.8, respectively (Sohn et al. Citation2007). Thus, these values were employed to estimate the biomass of each tree in our study.
Next, the biomass expansion factors (BEFs) are required in order to expand the stem volume to the total volume of the tree. The BEFs are classified into the above ground part and the subterranean part, because a tree is composed of its branches, leaves, and roots, as well as its stem. The BEF for the above ground part is the ratio of the branches and leaves to the stem, and the BEF for the subterranean part is the ratio of the roots to the entire above ground part. Unique BEF values do not exist, due to the intrinsic uncertainties and the dependence on the vegetation zones. In the case study of a South Korean forest, Sohn et al. (Citation2007) showed that the BEFs for the above ground parts are 29% for conifers and 22% for broad-leaved trees. The BEFs for the subterranean parts were estimated to be 28% for conifers and 48% for broad-leaved trees. Thus, the entire biomass stocks (BS) of a tree can be estimated by using EquationEquation (3)(3).
Finally, the biomass of an individual tree can be converted to its carbon content by multiplying it by a carbon fraction (CF), which was given as 0.5 in previous research and the IPCC guidelines (Westlake Citation1966). The CO2 stocks (CS) of an individual tree can then be calculated by using EquationEquation (4)(4) to convert the carbon content into CO2 stocks.
Accuracy assessment and discussion
There are several factors that influence the accuracy of the estimated CO2 stocks. Specifically, the accuracy of the filtering algorithms is directly related to the DTM quality, and consequently, the estimated tree height accuracy. To assess the filtering results, the developed filtering module was applied to the reference data provided by the International Society for Photogrammetry and Remote Sensing (ISPRS) commission III (ISPRS Citation2003). Then the accuracy assessment was performed using a cross-matrix. As a result of the assessment, the filtered data showed that the type-2 errors, which are the counts of object points that have been incorrectly identified as bare earth, were 3.32% in the given test data set (). In contrast, the type-1 errors, which are the counts of the bare earth points that have been incorrectly identified as objects, were calculated to be up to 13.19% in this study. The tree species in the study area are usually tall so that the ratio of type-2 errors would be smaller than the ISPRS test site case. Therefore, the underestimation of biomass by the dominance of type-2 error may not happen as the visual validation confirmed it. The effect of tree species in the filtering accuracy and the consequent biomass estimation needs to be studied further.
Table 3. The result of quantitative assessment of the filtering performance.
Together with the error source analysis, the DTM quality was estimated by using the empirical formula (Kraus et al. Citation2006) given in EquationEquation (5)(5).
As the result of an experiment, the standard deviation (one sigma) of the derived DTM varies from –0.0416 m to +0.2794 m. The performance of each tree height and the DBH estimation method were validated with field measurements using the root mean square error (RMSE). The RMSE of the tree heights and the RMSE of the DBH were 1.4 m and 6.2 cm, respectively ().
Table 4. Comparison of tree information acquired between lidar analysis and field measurements
Overall, all of the assessment results showed that the filtering algorithms worked well in the test area data so that the error of the CO2 stocks obtained by DTM was minimized.
In the assessment of the tree crown segmentation, 25 trees of the 28 trees that were measured during the field measurements are detected by the tree delineation procedure in this study (). The missing trees in the tree delineation were mainly caused by suppressed trees that were not detected with the CHM.
Figure 10. Estimated tree positions by lidar analysis (+) and field measurements (○) in sampling area 1.
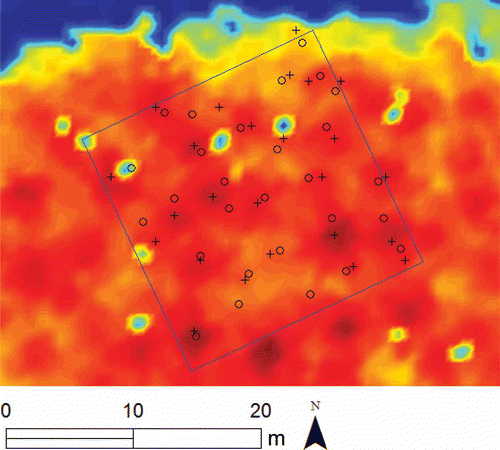
For the entire study area, to calculate the overall accuracy, the omission error and commission error are calculated based on the conventional approach of error matrix assessment (Rahman and Gorte Citation2009) using the reference data, which was produced manually using an aerial photograph. As a result, 445 trees were matched to trees found by the lidar data with an overall accuracy of 70%, commission error of 17%, and omission error of 18% ( ).
Figure 11. Estimated tree positions by lidar analysis (dark) and reference tree positions (light) produced manually.
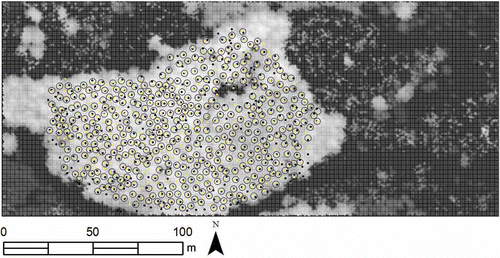
While the CO2 stock estimation based on the field measurement was 1113.21 tCO2, the CO2 stocks from the lidar data was calculated to be 1033.58 tCO2 ( ). These results show that the CO2 storage capacity estimated using the lidar analysis was only 7% less than the calculated CO2 storage capacity determined by field measurements. Considering the long temporal gap of up to 4 years between the lidar data acquisition and the field measurement, the error originating from the failures of the employed algorithms is certainly negligible. Therefore, these are highly encouraging results for the practical application of direct biomass and CO2 stock estimation based on lidar measurement and the machine vision algorithm.
Table 5. Comparison of CO2 stocks between lidar analysis and field measurements
Conclusion
In this study, a complete procedure to estimate the biomass and CO2 stock from the lidar processing by automated machine vision algorithms was developed. The deliberated assessments compared with field measurements showed the high reliability of the proposed method. It was proven that the lidar data acquisition and subsequent application of reliable algorithms have strong advantages of modeling the individual tree shapes, not only for the CO2 stock estimation but also for the extraction of a great deal of useful forestry information for other applications.
In spite of the successful biomass and CO2 stock estimation, it should be noted that the possibility of minor error sources affecting the overall accuracy of tree parameters still exists in each processing stage, such as lidar filtering, DTM generation, individual tree detection, and CO2 stocks calculation. The probability of the ill-measurement may be caused by the noise included in CHM and by the suppressed trees from the lidar data, which is too coarse to delineate strongly attached tree crowns. It may be addressed by the data fusion between the optical imagery and the range data of the lidar measurement.
In general, the reliability of direct exploitation of the lidar measurement for CO2 stocks has been well demonstrated, but the cost issue in acquiring lidar data for the whole target area still remains. Therefore, a future study will be focused on the exploitation of spaceborne Earth Observation assets referenced by the lidar measurement for the biomass and CO2 stocks application. On the other hand, the procedure in this study will be updated for a more complicated environment such as a mixed forest. In addition, the tree detection algorithm to reduce the over-/under-estimation of the number of trees has to be further developed for suppressing even small errors.
References
- Austin , J. M. , Mackey , B. G. and Van Niel , K. P. 2003 . Estimating Forest Biomass Using Satellite Radar: An Exploratory Study in a Temperate Austrian Eucalyptus Forest.” . Forest Ecology and Management , 176 ( 1–3 ) : 575 – 583 .
- Baltsavias , E. P. 1999 . A Comparison Between Photogrammetry and Laser Scanning.” . ISPRS Journal of Photogrammetry and Remote Sensing , 54 ( 2–3 ) : 83 – 94 .
- Brouard, J. S. 2009. “Genetic Gain.” http://www.abtreegene.com/toolkit/# (http://www.abtreegene.com/toolkit/#) (Accessed: 6 June 2012 ).
- Brown , S. and Gaston , G. 1995 . Use of Forest Inventories and Geographic Information Systems to Estimate Biomass Density of Tropical Forest: Application to Tropical Africa.” . Environmental Monitoring and Assessment , 38 ( 2–3 ) : 157 – 168 .
- Chen , Q. , Baldocci , D. , Gong , P. and Kelly , M. 2006 . Isolating Individual Trees in a Savanna Woodland Using Small Footprint LIDAR Data.” . Photogrammetric Engineering and Remote Sensing , 72 ( 8 ) : 923 – 932 .
- Dong , J. , Kaufmann , R. K. , Myneni , R. B. , Tucker , C. J. , Kauppi , P. E. , Liski , J. , Buermann , W. , Alexeyev , V. and Hughes , M. K. 2003 . Remote Sensing Estimates of Boreal and Temperate Forest Woody Biomass: Carbon Pools, Source, and Sinks.” . Remote Sensing of Environmental , 84 : 393 – 410 .
- Fazakas , Z. , Nilsson , M. and Olsson , H. 1999 . Regional Forest Biomass and Wood Volume Estimation Using Satellite Data and Ancillary Data.” . Agricultural and Forest Meteorology , 98–99 ( 31 ) : 417 – 425 .
- Fitzgibbon , A. , Pilu , M. and Fisher , R. B. 1999 . Direct Least Square Fitting of Ellipses.” . IEEE Transactions on Pattern Analysis and Machine Intelligence , 21 ( 5 ) : 476 – 480 .
- Fraser , R. H. and Li , Z. 2002 . Estimating Fire-Related Parameters in Boreal Forest Using SPOT VEGETATION.” . Remote Sensing and Environmental , 82 ( 1 ) : 95 – 110 .
- Gougeon , F. A. 1995 . A Crown-Following to the Automatic Delineation of Individual Tree Crown in High Spatial Resolution Aerial Images.” . Canadian Journal of Remote Sensing , 21 ( 3 ) : 274 – 284 .
- Hodgson , M. E. and Bresnahan , P. 2004 . Accuracy of Airborne LIDAR Derived Elevation: Empirical Assessment and Error Budget.” . Photogrammetric Engineering and Remote Sensing , 70 ( 3 ) : 331 – 339 .
- Hodgson , M. E. , Jensen , J. R. , Schmidt , L. , Schill , S. and Davis , B. 2003 . An Evaluation of LIDAR- and IFSAR-Derived Digital Elevation Models in Leaf-on Conditions with USGS Level 1 and Level 2 DEMs.” . Remote Sensing of Environment , 84 ( 2 ) : 295 – 308 .
- Hyyppä , J. , Hyyppä , H. , Inkinen , N. , Engdahl , M. , Linko , S. and Zhu , Y. H. 2000 . Accuracy Comparison of Various Remote Sensing Data Sources in the Retrieval of Forest Stand Attributes.” . Forest Ecology and Management , 128 ( 1–2 ) : 109 – 120 .
- IPCC . 2007 . Climate Change 2007: Synthesis Report , 30 – 39 . Geneva : IPCC . (Intergovernmental Panel on Climate Change)
- ISPRS (International Society for Photogrammetry and Remote Sensing). 2003. “ISPRS Test on Extracting DEMS from Point Clouds: A Comparison of Existing Automatic Filters.” http://www.itc.nl/isprswgIII-3/filtertest/ (http://www.itc.nl/isprswgIII-3/filtertest/) (Accessed: 6 June 2012 ).
- Kaartinen , H. , Hyyppä , J. , Yu , X. , Vastaranta , M. , Hyyppä , H. , Kukko , A. and Holopainen , M. 2012 . An International Comparison of Individual Tree Detection and Extraction Using Airborne Laser Scanning . Remote Sensing , 4 ( 4 ) : 950 – 974 .
- Kim , J. R. and Muller , J. 2011 . Tree and Building Detection in Dense Urban Environments Using Automated Processing of IKONOS Image and LIDAR Data.” . International Journal of Remote Sensing , 32 ( 8 ) : 2245 – 2273 .
- Koch , B. , Heyder , U. and Weinacker , H. 2006 . Detection of Individual Tree Crowns in Airborne LIDAR Data.” . Photogrammetric Engineering and Remote Sensing , 72 ( 4 ) : 357 – 363 .
- Korpela , I. , Dahlin , B. , Schäfer , E. , Haapaniemi , F. , Honkasalo , J. and Ilvesniemi , S. 2007 . Single-Tree Forest Inventory Using LIDAR and Aerial Images for 3D Treetop Positioning, Species Recognition, Height and Crown Width Estimation . Proceedings of the ISPRS Workshop on Laser Scanning 2007 and SilviLaser 2007 Espoo, Finland , 36 September 12–14 : 227 – 233 .
- Kraus , K. , Karel , W. , Briese , C. and Mandlburger , G. 2006 . Local Accuracy Measures for Digital Terrain Models.” . The Photogrammetric Record , 21 ( 116 ) : 342 – 354 .
- Kraus , K. and Pfeifer , N. 1998 . Determination of Terrain Models in Wooded Areas with Airborne Laser Scanner Data.” . ISPRS Journal of Photogrammetry and Remote Sensing , 54 ( 4 ) : 193 – 203 .
- Leckie , D. G. , Gougeon , F. A. , Hill , D. A. , Quinn , R. , Armstrong , L. and Shreenan , R. 2003 . Combined High-Density LIDAR and Multispectral Imagery for Individual Tree Crown Analysis.” . Canadian Journal of Remote Sensing , 29 ( 5 ) : 633 – 649 .
- Lu , D. and Batistella , M. 2005 . Exploring TM Image Texture and Its Relationships with Biomass Estimation in Rondô Nia, Brazilan Amazon.” . Acta Amazonica , 35 : 261 – 268 .
- Luckman , A. , Baker , J. , Honzák , M. and Lucas , R. 1998 . Tropical Forest Biomass Density Estimation Using JERS-1 SAR: Seasonal Variation, Confidence Limits, and Application to Image Mosaics.” . Remote Sensing of Environmental , 63 ( 2 ) : 126 – 139 .
- Najman , L. and Schmitt , M. 1996 . Geodesic Saliency of Watershed Contours and Hierarchical Segmentation.” . IEEE Transactions on Pattern Analysis and Machine Intelligence , 18 ( 12 ) : 1163 – 1173 .
- Popescu , S. C. 2007 . Estimating Biomass of Individual Pine Trees Using Airborne LIDAR.” . Biomass & Bioenergy , 31 ( 9 ) : 646 – 655 .
- Popescu , S. C. , Wynne , R. H. and Nelson , R. E. 2003 . Measuring Individual Tree Crown Diameter with LIDAR and Assessing Its Influence on Estimating Forest Volume and Biomass.” . Canadian Journal of Remote Sensing , 29 ( 5 ) : 564 – 577 .
- Raber , G. T. , Jensen , J. R. , Schill , S. R. and Schuckman , K. 2002 . Creation of Digital Terrain Models Using an Adaptive LIDAR Vegetation Point Removal Process.” . Photogrammetric Engineering and Remote Sensing , 68 ( 12 ) : 1307 – 1315 .
- Rahman , M. Z. A. and Gorte , B. G. H. September 1–2 2009 . “ Tree Crown Delineation from High Resolution Airborne LIDAR Based on Densities of High Points ” . In Proceedings of the ISPRS Workshop on Laser scanning 2009 , Vol. 38 , September 1–2 , 123 – 128 . France : Paris .
- Sohn , Y. M. , Kim , J. C. , Lee , K. H. and Kim , R. H. 2007 . Forest Biomass Assessment in Korea , 105 Seoul : Korea Forest Research Institute .
- Thenkabail , P. S. , Stucky , N. , Griscom , B. W. , Ashton , M. S. , Diels , J. , Van der Meer , B. and Enclona , E. 2004 . Biomass Estimations and Carbon Stock Calculations in the Oil Palm Plantations of African Derived Savannas Using IKONOS Data.” . International Journal of Remote Sensing , 25 ( 23 ) : 5447 – 5472 .
- Tiwari , A. K. and Singh , J. S. 1984 . Mapping Forest Biomass in India Through Aerial Photographs and Nondestructive Field Sampling.” . Applied Geography , 4 ( 2 ) : 151 – 165 .
- Wang , L. , Gong , P. and Biging , G. S. 2004 . Individual Tree-Crown Delineation and Treetop Detection in High-Spatial-Resolution Aerial Imagery.” . Photogrammetric Engineering & Remote Sensing , 70 ( 3 ) : 351 – 358 .
- Westlake , D. F. 1966 . The Biomass and Productivity of Glyceria Maxima: I. Seasonal Changes in Biomass.” . Journal of Ecology , 54 ( 3 ) : 745 – 753 .
- Zhao , K. , Popescu , S. C. and Nelson , R. F. 2009 . LIDAR Remote Sensing of Forest Biomass: A Scale-Invariant Estimation Approach Using Airborne Lasers.” . Remote Sensing of Environment , 113 ( 1 ) : 182 – 196 .
- Zheng , D. , Rademacher , J. , Chen , J. , Crow , T. , Bresee , M. , Moine , J. L. and Ryu , S. R. 2004 . Estimating Aboveground Biomass Using Landsat 7 ETM+ Aata Across a Managed Landscape in Northern Wisconsin, USA.” . Remote Sensing of Environment , 93 ( 3 ) : 402 – 411 .