Abstract
The aim of this study was to determine whether diameter at breast height (DBH, at 1.3 m) and total height of radiata pine (Pinus radiata D. Don) trees showed any significant relationships with microsite estimates of solar radiation. A total of 77 plots were established in two even-aged stands of radiata in Nundle State Forest, New South Wales, Australia. Within these plots, tree DBH and height measurements were recorded and their relationships with solar radiation evaluated. Airborne light detection and ranging (LiDAR) data were processed to generate a high resolution digital elevation model (DEM), and the DEM was used for calculating the incoming solar radiation. Overall, at both study sites, taller and larger trees were found on areas with lower solar radiation, possibly due to a lower loss in soil moisture. The findings of this study suggest that LiDAR-derived DEM estimates of solar radiation are significantly correlated with DBH and height variation, and therefore suitable for use as a sub-compartment stratification variable as well as for possible inclusion in fine-scale estimates of P. radiata growth and productivity.
1. Introduction
Tree height and diameter at breast height (DBH) are key variables used in allometric equations for numerous forest inventory parameters, including stand stem volume and biomass. Significant intra-compartment variation in tree shape and size, however, can challenge the acceptable levels of precision and bias required for Planning Unit estimates of total stem volume and biomass from traditional plot sampling strategies, which are based on compartment level stratification using only compartment age and silvicultural history. Knowledge of intra-compartment variability in tree height and DBH is important as these small area inventories are used for operational yield predictions and facilitate local silvicultural and harvesting decisions. It is well established that stand variations are mainly governed by an inherited developmental tendency, that can be modified by local climatic, edaphic, topographical and stand factors (e.g. Woollons, Snowdon, and Mitchell Citation1997). Numerous models have been proposed that attempt to increase precision and predictive power by utilizing some of these site attributes as adjunct explanatory variables (e.g. Woollons, Snowdon, and Mitchell Citation1997; Mason, Methol, and Cochrane Citation2011). Variables such as elevation or rainfall, derived from meteorological data or moderate spatial resolution remotely sensed data, have generally been used for this approach (Mason, Methol, and Cochrane Citation2011). However, simple interpolation and extrapolation of climatic point data or the use of moderate resolution digital elevation models (DEMs) may not be appropriate for plantation sites with topographic heterogeneity.
Solar radiation or light availability is one of the most important environmental factors affecting tree growth (Loetsch and Haller Citation1973; Van Laar and Akça Citation2007). The spatial and temporal heterogeneity of incoming solar radiation can directly affect microsite conditions such as soil temperature and soil moisture, which cumulatively influences tree physiological functions such as photosynthesis and respiration and hence stand growth and production (Tovar-Pescador et al. Citation2006; Alvarez et al. Citation2013). Specifically, solar radiation was found to be a primary parameter affecting growth rates of radiata pine (Pinus radiata D. Don) trees in Chile, with plantations receiving high incoming solar radiation on sites not exposed to water or nutrient limitations exhibiting the highest productivities (Alvarez et al. Citation2013). It is also a key input for many process-based models of forest growth and production (e.g. Landsberg and Waring Citation1997; Coops and Waring Citation2001). These sophisticated biophysical-based models can provide reliable estimates of stand volume and biomass and are not limited to sites under which they were developed. However, they have demanding data requirements that can limit multi-scale applications and have encountered difficulties in obtaining precise areal estimates at an affordable cost (Mummery and Battaglia Citation2002; Miehle et al. Citation2006; Mason, Methol, and Cochrane Citation2011). Although, a number of studies have reported the importance of spatial variation in local climate, including solar radiation, on stand-level parameters of P. radiata growth (e.g. Byrne, Landsberg, and Benson Citation1986; Mason, Methol, and Cochrane Citation2011; Alvarez et al. Citation2013), the relationship between solar radiation and tree-scale attributes such as height and DBH using a high spatial resolution DEM (<3 m) has not been investigated.
Solar radiation varies with the geometry of the receiving objects and slope and aspect gradients (Kumar, Skidmore, and Knowles Citation1997). There are different interpolation techniques for computing the potential solar radiation or the amount of direct or indirect (diffuse and reflected) radiation under clear-sky conditions from meteorological and climatological stations (e.g. kriging, spline functions and weighted average procedures) (Tovar-Pescador et al. Citation2006). More recently models have been developed that calculate insolation maps, including both direct and diffuse solar radiation, directly from DEMs (e.g. Álvarez, Mitasova, and Allen Citation2011). In addition, airborne light detection and ranging (LiDAR) data deliver detailed 3D structural information, which has been used in capturing a range of terrain and forest measurements (e.g. Heurich Citation2008; Im, Jensen, and Hodgson Citation2008; Turner et al. Citation2011). The high spatial resolution DEM derived from LiDAR provides efficient and accurate information of the ground surface, compared with the traditional methods (e.g. orthophotography or measurements from meteorological/climatological stations) (Turner Citation2006; Petroselli Citation2012; Li Citation2013; White et al. Citation2013). The detailed information from LiDAR-derived DEMs, integrated within geographical information systems (GIS), has led to the rapid, cost-efficient and accurate estimation of solar radiation (e.g. Álvarez, Mitasova, and Allen Citation2011; Li Citation2013). Other LiDAR-derived models including digital surface model (DSM) and canopy height model (CHM) have also gained popularity over the last few years as important fundamental input data for modelling tree structural characteristics (e.g. Hyyppä et al. Citation2008; Edson and Wing Citation2011; Yu et al. Citation2011). However, while many studies have demonstrated the utility of LiDAR in forest management applications (e.g. Popescu and Wynne Citation2004; Coops et al. Citation2007; Watt et al. Citation2013; Chen and Zhu Citation2013) to the best of our knowledge no studies have used LiDAR data to explore relationships between spatial variation in tree stem attributes and solar radiation at the micro-terrain scale.
In the Hanging Rock State Forest (SF) plantation, New South Wales (NSW), Australia, despite the relatively homogeneous environmental conditions, such as similar climatic (temperature and rainfall), edaphic (geology and soil type) and genetic sources, the radiata pine trees exhibited significant height and DBH variation within two even-aged stands. Differences in microclimate factors such as topographical factors and light, which in turn influence soil moisture and nutrients, may be the reason for this variation. The effect of topography on tree height and DBH was demonstrated in earlier work by the authors and each variable showed significant relationships with the topographical factors of aspect and slope (Saremi et al. Citation2014a, Citation2014b). In the present study, solar radiation and its relationship to the observed variation in tree height and DBH was investigated in two even-aged radiata stands by applying a multivariate procedure that effectively utilizes the information of the tree characteristics as well as the solar radiation data produced by the LiDAR-derived DEM. Prior to the main investigation, relationship between tree height with DBH were conducted to see the pattern of variations in these variables. Multivariate techniques can combine several tree characteristics and test the effect of different factors on all the characteristics simultaneously. A significant relationship could indicate solar radiation derived from a LiDAR DEM as a potential microsite variable for radiata pine growth and yield models.
2. Methods
2.1. Study area
This study was conducted in the Hanging Rock SF (31° 26ʹ 24″–31° 28ʹ 15″ S, 151° 14ʹ 20″–151° 16ʹ 43″ E), P. radiata plantation, near the town of Nundle in the northern tablelands of NSW, Australia (). Two even-aged stands were selected within 7000-ha plantation. These plantations are located in an undulating plateau characterized by hilly topography. The SF covers an elevation range of 1055 to 1325 m asl. The slope range is between 0° and 45° and a wide range of aspects are found in the plantation (). The annual rainfall is approximately 1050 mm. The geological materials that make up this plateau comprise largely basalt rock and fertile Tertiary basalt soils (Ryan and Holmes Citation1986). The commercial plantation estate contains over 100 compartments, representing a range of age classes and silvicultural treatments; however, just two stands were evaluated due to funding limitations. The first stand was planted in 1977 (34-year-old age class) and the second stand was established in 2002 (9-year-old age class). The initial stocking rate of the 1977 and 2002 stands were reported to be approximately 1300 and 1000 stems per ha, respectively; however, self-thinning processes and patchy establishment resulted in irregular stem density over the years ().
Figure 1. (a) The location of the study area in Australia, (b) the Hanging Rock State Forest and the extent of the study areas, (c) The location of the plots at each study site on a 2 m resolution LiDAR-derived DEM.
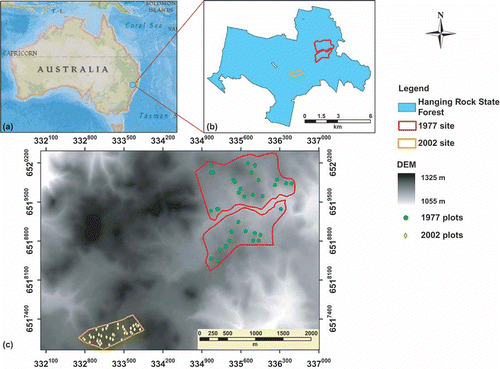
Table 1. The range of slopes and aspects in the State Forest (in percentage).
Table 2. Summary of the field data collected in this study.
2.2. Field survey data
The ground survey plots used in this study were originally established for another study by New South Wales Department of Primary Industries (NSW DPI) and the Forestry Corporation NSW (FCNSW). These plots were initially stratified using focal-based stand level metrics extracted from a LiDAR-derived CHM (1 m resolution). Mean dominant height (MDH) and stocking rate were used for the stratification process. In total, 55 plots in the 1977 stand and 53 plots in the 2002 stand were established. However, to achieve the main aim of this study only those plots and individual trees in the field that could be accurately identified within the LiDAR data were selected. As a result, a total of 34 plots in the 1977 stand and a total of 43 plots in the 2002 stand were selected. The selected plots were assumed to be a random subset of the established plots in the analysis (e.g. the range of LiDAR heights in the sampled plots was similar to that in the population). From each plot, in July 2011, tree diameters (DBH at 1.3 m) were measured for every tree, and five to six trees were then selected for height measurement. These five or six trees from each plot were chosen based on the diameter range across each plot and represent a subsample of the total variability of the trees diameter seen across the plot. The tree heights were measured three times with a digital hypsometer (Haglöf Vertex IV Hypsometer, Sweden), and DBH was measured with a diameter tape (the diameter scale is determined by the formula, circumference divided by pi or 3.1416). The tree height and DBH precision were made to 10–30 cm and 1 cm, respectively. The relationship between DBH and height is tested below with the simple regression analysis. Additional information relating to the study sites is shown in .
The centre of each survey plot was provided as GPS waypoints and manually marked in the imagery prints in the field. Two GPS units were used for this study, the ScoutPak GPS system (Juniper Systems and OnPOZ Precision Positioning, Logan, Utah, USA) and the Garmin Oregon® 550 (Garmin International). The circular plots selected for this study presented a range of different local topographical features, for example aspects ().
2.3. LiDAR data
In June 2011, discrete return airborne LiDAR data were acquired with a Trimble Harrier 68i system mounted on a Cessna U206G airplane. The LiDAR swaths were collected along an approximately 1 km width with 40% swath overlap. The flying altitude was approximately 1155 m. The footprint size was 0.5 m and the average posting density was 3.6 points per square metre. The LiDAR points were captured, processed, geo-referenced and classified by Terranean Mapping Technologies Pty Ltd into ground and non-ground categories using MARSÒ software (Merrick & Company).
2.4. Solar radiation model
FUSION software and LiDAR data viewer (LDV) visualizing system from the US Forest Service (McGaughey Citation2007) were used for creating two high resolution DEMs (3 m for the 1977 stand and 2 m for the 2002 stand) from the LAS ground point data for the two study sites. Kraus and Pfeifer (Citation1998) have described the bare-earth surface algorithm (GroundFilter algorithm) and also the suitability of using airborne LiDAR for DEM generation. The DEMs provided information about topographic characteristics including elevation, aspect, slope and skyview (a parameter that describes the portion of visible sky limited by relief) in grid cells. The DEM also provided the artificial horizon and relative position of the sun (Duguay Citation1993).
Solar radiation modelling was calculated by integrating the LiDAR-derived DEMs in ArcInfo workstation (ArcGIS 10, ESRI). The implemented solar radiation model used in this study is adapted from Kumar, Skidmore, and Knowles (Citation1997). This program calculates the short wave radiation received at the surface of the earth over a period of time, under clear-sky conditions. Based on the geometry and geographic location, each point of the surface presents a different artificial horizon and a different relative position to the sun’s orbit. As a consequence, for every location insolation can be calculated based on surface orientation and shadowing effects (Kumar, Skidmore, and Knowles Citation1997; Tovar-Pescador et al. Citation2006). Solar radiation received at a site depends on the solar flux outside the atmosphere, the optical air mass and water vapour and aerosol content of the atmosphere.
The program computes time of sunrise and sunset and determines the amount of solar radiation generated during this period for any given day. The inputs used for modelling include LiDAR-derived DEMs, latitude of the site in degrees, a day number for the start and end of calculations (1 January being day 1 and 31 December being day 365) and time interval, in minutes, between calculations of flux. Values for latitude were negative as the study sites are located in the southern hemisphere. The models were generated monthly, and the time interval between calculations was set at 15 minutes. For every grid cell in the area (grid size of 3 m for the 1977 stand and 2 m for the 2002 stand) the direct and diffuse solar irradiance can be estimated based on the relative position of the sun at each point in time.
The solar flux (Io) outside the atmosphere is given by Kreith and Kreider (Citation1978) and Duffie and Beckman (Citation1991) (Equation (1)).
where So is the solar constant and gives the irradiance of an area perpendicular to the sun’s rays just outside the atmosphere and at the mean sun–earth distance. N is the day number for which the calculation is being performed. A value of 1367 Wm−2 was used for the solar constant in the modelling, based on values suggested by Duffie and Beckman (Citation1991) and the World Radiation Centre. Equation (1) accounts for variation in the solar irradiance at the top of the atmosphere throughout the year as a result of the elliptical nature of the earth’s path around the sun.
Solar radiation is attenuated as it passes through the atmosphere and can be estimated using Bouger’s Law (Kreith and Kreider Citation1978). Absorption by atmospheric molecules is a selective process that converts incoming energy to heat, and is mainly due to water, oxygen, ozone and carbon dioxide. Equations describing the absorption effects of the above are given by Turner and Spencer (Citation1972). A number of other gases absorb radiation but their effects are relatively minor and for most practical purposes can be neglected (Forster Citation1984). Bouger’s Law uses the air mass ratio, which is the relative mass of air through which solar radiation must pass to reach the surface of the earth, and is the ratio of actual path length mass to the mass when the sun is directly overhead. The adjustment in air mass for local altitude is made in terms of the local atmospheric pressure (Kreith and Kreider Citation1978; Frouin et al. Citation1989). These then take care of local aerosol and water vapour content for local conditions. Detailed implementation of the equations is provided in Kumar, Skidmore, and Knowles (Citation1997).
The solar altitude angle changes continuously throughout the day. The noon solar angle altitude varies seasonally, resulting in variation in solar radiation distribution throughout the seasons (Kumar, Skidmore, and Knowles Citation1997). Due to the longer day-lengths, November, December and January are the months which receive the most radiation in the southern hemisphere. In order to determine the different amounts of energy received by each tree seasonally, estimates of radiation values were calculated during summer and winter across the 1977 and 2002 stands.
2.5. Tree detection from LiDAR
A CHM was generated in FUSION from LiDAR point cloud and was computed as the difference between the upper canopy surface or DSM and DEM values. The measured field trees were identified manually within the LiDAR data. Two GPS devices (horizontal accuracy of around ±5 m), crown interpretation and canopy gap arrangements made it possible to locate and match the individual field trees to the LiDAR data. To reduce spatial error, only trees that could be accurately located in the LiDAR data were selected. A total of 193 trees in the 1977 stand and 254 trees in the 2002 stand were identified. The heights of these trees were individually estimated in LDV FUSION using the LiDAR point cloud, and their coordinates and elevation were also recorded. In the previous study by the authors (Saremi et al. Citation2014a), the LiDAR extracted heights were compared to the field measured heights, and a high correlation was demonstrated between the two variables (for the 1977 stand R2 = 0.87 and relative RMSE = 1.49 and for the 2002 stand R2 = 0.9 and relative RMSE = 0.66). Therefore in this study, LiDAR heights were used for data analysis.
The tree tops were identified from the CHM by local maxima. Maxima were identified as being the highest pixels in a 3 × 3 m search window. The tree tops were used as markers to improve the accuracy of matching the solar radiation to the tree locations. The local maxima algorithm used in FUSION is similar to that reported in Popescu and Wynne (Citation2004). The tree tops (2 × 2 m cells in the 2002 stand and 3 × 3 m cells in the 1977 stand) were then matched to the solar radiation values.
2.6. Data analysis
A multivariate mixed linear model procedure was used to explain the variation in the dependent variables DBH and height (expressed as a vector) by employing a set of independent variables (summer and winter solar radiation) with the available observational data.
The multivariate mixed model was written as
where Y is the vector of dependent variables, X is the design matrix for fixed effects, b is the vector of fixed independent variables, Z is the design matrix for random effects, u is the vector of random effects and e is the matrix of random errors. The multivariate mixed model was fitted using the residual maximum likelihood (REML) method. The basic advantage in the above approach is that the dependent variables DBH and height of trees are modelled simultaneously.
It should be noted that the ground survey design in this study was based on a height by stocking stratification with equal numbers of plots per strata. Since the strata themselves had differing numbers of plots the selection probabilities were not equal for all plots in the survey. However, the data were analysed from a model-based perspective which essentially treats the sampled plots as ‘fixed’ irrespective of how they were selected. In extrapolating these results to a larger population the key consideration is whether the distribution of solar radiation values in the sample enables the model to be correctly determined, and this is the assumption underlying the analysis.
Plot number and stratum were included as a random factor while winter and summer solar radiation data were fitted as fixed effects in the model. Plots in this study site were selected according to a stratified random sampling scheme; however, they were treated as random in the analysis. The error term is composed of a variance matrix which has separate components for height and DBH and a term which represents the covariance between these two. The R package Asreml-R, version 3.0.1 (Butler et al. Citation2009) which is implemented in the R statistical software package (R Development Core Team Citation2011) was used for the analysis. The error distribution was assumed to be Gaussian. Wald statistics were computed for the fixed effects in the model and used to assess the significance of these effects. The residuals from the model were inspected to confirm that the assumptions of normality were approximately satisfied.
3. Results
3.1. Height–DBH relationship
For each study site, the relationship between tree height and DBH was analysed using a non-linear regression of height versus the log of DBH. Although, the height and DBH relationships () were significant for both 2002 and 1977 stands (P < 0.01 and P < 0.001, respectively) there was considerable variation in tree size within both stands.
3.2. The 1977 stand
Multivariate models were constructed separately for the 1977 stand. The height and DBH of the 193 trees were fitted to winter and summer solar radiation factor using a multivariate mixed linear model (Equation (3)).
presents the sum of squares, Wald statistics and probabilities of exceeding the Wald statistics for the multivariate model described in Equation (2). Based on these results, the mean, random plots, strata and error terms were highly significant (P < 0.001). The relationship between the winter radiation and the DBH and height variables was very significant at P < 0.01; however, no significant relationship existed between summer radiation and height and DBH.
Table 3. Estimated sum of squares, Wald statistics, probability and the random effect results of the multivariate model in the 1977 stand.
The linear models between both height and DBH with winter radiation are represented in . The results suggest that taller trees and trees with larger DBH were seen in areas with lower values of winter solar radiation. As solar radiation reached to 1200 MJ m–2 over the season (approximately, 13.3 MJ m–2 day–1), more shorter trees with height less than 35 m and more smaller trees with DBHs of less than 55 cm were exhibited. The same trends were seen in summer solar radiation; however, they were not significant.
Figure 4. The relationships between (a) height with winter radiation, (b) DBH with winter radiation, (c) height with summer radiation and (d) DBH with summer radiation in the 1977 stand. The line is based on the multivariate model and shows the relationship between predicted height and DBH vs. summer and winter radiation. The R2 values are calculated from the height and DBH residuals and are the same for both summer and winter radiation.
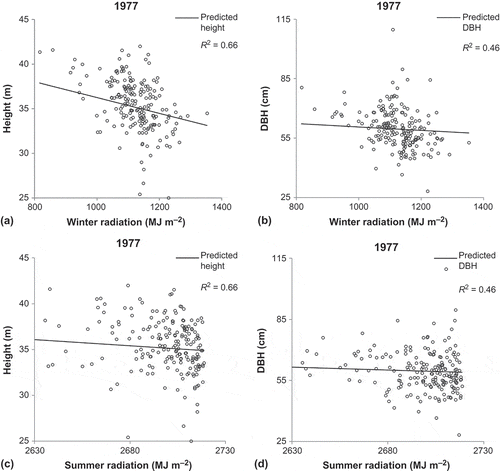
3.3. The 2002 stand
Similar to the 1977 stand, the younger trees were also fitted to winter and summer solar radiation factors through the multivariate mixed linear model (Equation (3)). shows the analysis of variance between the height and DBH of the 9-year-old trees with winter and summer radiation. Based on the results, the mean, random plots, strata and error term were all highly significant (P < 0.001). Unlike the older trees, summer radiation showed significant relationship with the height and DBH of the younger trees (P < 0.001). A significant relationship was also seen among the DBH and height of these trees with different levels of winter radiation (P < 0.01).
Table 4. Estimated sum of squares, Wald statistics, probability and the random effect results of the multivariate model in the 2002 stand.
illustrates the relationship between the DBH and height of trees for the 2002 site with winter and summer radiation. In both winter and summer radiation, taller trees are associated with low levels of radiation, and as the radiation increases shorter trees are found ( and ). A similar trend is also seen in the DBH, where thicker trees are associated with lower levels of radiation.
Figure 5. The relationships between (a) height with winter radiation, (b) DBH with winter radiation, (c) height with summer radiation and (d) DBH with summer radiation in the 2002 stand. The line is based on the multivariate model and shows the relationship between predicted height and DBH vs. summer and winter radiation. The R2 values are calculated from the height and DBH residuals and are the same for both summer and winter radiation.
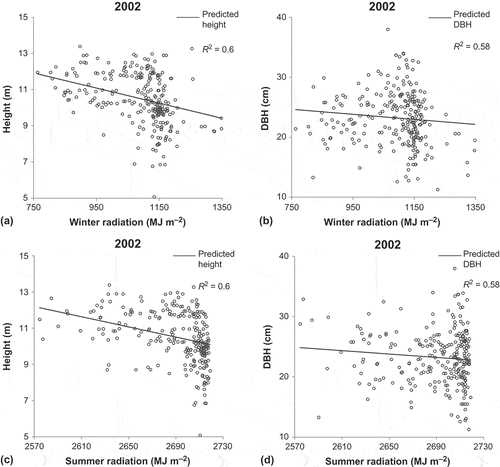
The amount of solar radiation received on northerly and southerly aspects in the 1977 stand, is illustrated in . The figure shows very small differences between the northerly and southerly aspects during the summer months; however, during the winter season the differences between the aspects are more evident.
4. Discussion
Despite similar climatic and edaphatic conditions at both 2002 and 1977 sites, our study revealed micro-scale variation in radiata tree height and DBH, and this response was shown to be associated with variation in the microsite estimates of solar radiation. Numerous other studies have shown that the height–diameter relationship can vary in response to a range of environmental and stand conditions, including solar radiation, but not at a spatial resolution exceeding that derived from a LiDAR DEM (e.g. Curtis Citation1967; Watt and Kirschbaum Citation2011). While not part of our study, the potential benefits of including high spatial resolution estimates of solar radiation into tree taper functions for sub-compartment prediction purposes may present an affordable option to improve model precision.
At finer scales, apart from cloudiness and other atmospheric heterogeneities, the distribution of incoming solar radiation is affected by topography (Kumar, Skidmore, and Knowles Citation1997; Kumar and Skidmore Citation2000; Aguilar, Herrero, and Polo Citation2010). Variability in elevation, slope angle, slope orientation (aspect) and shadowing can create strong local gradients in solar radiation which directly and indirectly affect air and soil heating, water balances and vegetation canopy (Dubayah and Van Katwijk Citation1992; Dubayah Citation1994). Generally, in the southern hemisphere, northerly aspects receive far more radiation than southerly aspects, and the winter months receive about half the solar radiation as the summer months (Kumar, Skidmore, and Knowles Citation1997). However, during summer this contrast diminished and both south and north facing slopes received the same amount of solar radiation. Kumar, Skidmore, and Knowles (Citation1997) also reported that during December and January (summer) the radiation in southerly aspect is very close to that in northerly aspect. The very small differences between the northerly and southerly aspects in can be a possible reason for the results obtained for the 1977 stand, where the analysis did not reveal any statistically significant association between the summer radiation and DBH and height of the older trees. Also in winter the sun is low in the sky and the range within the solar radiation is higher (700 to 1500 MJ m−2) than for the summer radiation (2550 to 2750 MJ m−2), and this may explain the significant relationships which existed between the height and DBH of trees and the winter radiation.
Winter radiation explained DBH and height variation at both study sites, wherein both older and younger stands, larger and taller trees were associated with lower values of winter radiation. Sites with high incoming solar radiation conserve less soil water (Belsky Citation1994). The availability of soil water is a critical issue in countries like Chile and Australia, where it affects the growth performance of radiata pine trees (Snowdon et al. Citation2000; Alvarez et al. Citation2013). The 1977 and 2002 study sites have a mean annual rainfall of approximately 1000 mm and without a seasonal dry period during the spring or summer months. However, the results of this study suggest that these plantations appear to have been subjected to periods of limiting soil water content and higher rates of evapotranspiration during their growing period. Medlyn (Citation1998) reported that environmental stress, such as low water availability, affected radiata pine sites with high incoming solar radiation and reduced the ability of trees to use the light for photosynthesis (light use efficiency). Alvarez et al. (Citation2013) also indicated that water restricted radiata pine sites had low values of light use efficiency. The results of this study are supported by Gray et al. (Citation2005), who found most of the conifer species in Sierra National Forest, California on the microsites with relatively high soil moisture and relatively low solar radiation.
The reliability of DEMs for estimating solar radiation has been evaluated in several studies (e.g. Tovar-Pescador et al. Citation2006; Kumar Citation2009; Ruiz‐Arias et al. Citation2009). Although the incoming solar radiation at every point of the DEM is determined by topographic features such as elevation and surface orientation; it should be noted that the same DEM with different resolutions can produce slightly different estimations of topographical factors (Raaflaub and Collins Citation2006) and consequently different estimations of solar radiation (Ruiz‐Arias et al. Citation2009). The usefulness of LiDAR-derived DEMs, however, in providing high spatial resolution estimates of solar radiation has been stated in several other studies (Turner Citation2006; Arnold and Rees Citation2009). Our observed correlations between fine resolution estimates of solar radiation with DBH and height variation illustrate the suitability of this environmental parameter for use as a sub-compartment stratification variable as well as a spatial auxiliary variable in small area estimates. The operational derivation of LiDAR-derived surfaces of solar radiation has significantly improved with the recent release of commercial software (e.g. the solar radiation analysis tools in the Spatial Analyst extension of ArcGIS 10.1 & 10.2) and the r.sun module (Suri and Hofierka Citation2004) implemented under an open-source GRASS GIS environment (Neteler and Mitasova Citation2008). These packages can provide rapid, cost-efficient estimates of solar radiation at a sub-compartment scale.
5. Conclusion
DBH and tree height are variables commonly used for estimating growth and yield of radiata stands as well as for estimates of biomass. The spatial variation of these tree characteristics within single-aged compartments result from complex interactions between the stand management, micro-climatic factors, site factors, natural disturbances and intra-specific competition (Moeur Citation1993). This research has confirmed that the DBH and height of radiata pine trees are influenced by the incoming solar radiation, derived from a LiDAR DEM. Tall and large DBH trees were found in areas with low levels of radiation. The results presented here suggest that lack of soil moisture may be an important limiting factor for these plantations on the northern tablelands of NSW. It is recommended that similar studies need to be carried out in other regions in order to confirm the potential effect of solar radiation on tree height and DBH and the importance of including this factor into P. radiata growth and yield models.
Acknowledgements
The authors would like to thank FCNSW for providing the LiDAR data, and the NSW DPI for help in conducting the field surveys used in this study. We would like to express our deep gratitude to the Editors and the two anonymous reviewers for their constructive comments and suggestions to improve the quality of the paper.
References
- Aguilar, C., J. Herrero, and M. J. Polo. 2010. “Topographic Effects on Solar Radiation Distribution in Mountainous Watersheds and Their Influence on Reference Evapotranspiration Estimates at Watershed Scale.” Hydrology and Earth System Sciences 14 (12): 2479–2494. doi:10.5194/hess-14-2479-2010.
- Alvarez, J., H. L. Allen, T. J. Albaugh, J. L. Stape, B. P. Bullock, and C. Song. 2013. “Factors Influencing the Growth of Radiata Pine Plantations in Chile.” Forestry 86 (1): 13–26. doi:10.1093/forestry/cps072.
- Álvarez, J., H. Mitasova, and H. L. Allen. 2011. “Estimating Monthly Solar Radiation in South-Central Chile.” Chilean Journal of Agricultural Research 71 (4): 601–609. doi:10.4067/S0718-58392011000400016.
- Arnold, N., and G. Rees. 2009. “Effects of Digital Elevation Model Spatial Resolution on Distributed Calculations of Solar Radiation Loading on a High Arctic Glacier.” Journal of Glaciology 55 (194): 973–984. doi:10.3189/002214309790794959.
- Belsky, A. J. 1994. “Influences of Trees on Savanna Productivity: Tests of Shade, Nutrients, and Tree-Grass Competition.” Ecology 75 (4): 922–932. doi:10.2307/1939416.
- Butler, D., B. R. Cullis, A. R. Gilmour, and B. J. Gogel. 2009. Asreml-R Reference Manual, Release 3. Queensland: Department of Primary Industries and Fisheries.
- Byrne, G. F., J. J. Landsberg, and M. L. Benson. 1986. “The Relationship of Above-Ground Dry Matter Accumulation by Pinus radiata to Intercepted Solar Radiation and Soil Water Status.” Agricultural and Forest Meteorology 37 (1): 63–73. doi:10.1016/0168-1923(86)90028-6.
- Chen, Y., and X. Zhu. 2013. “An Integrated GIS Tool for Automatic Forest Inventory Estimates of Pinus radiata from LiDAR Data.” Giscience & Remote Sensing 50 (6): 667–689.
- Coops, N. C., T. Hilker, M. Wulder, B. St-Onge, G. Newnham, A. Siggins, and J. Trofymow. 2007. “Estimating Canopy Structure of Douglas-Fir Forest Stands from Discrete-Return Lidar.” Trees – Structure and Function 21 (3): 295–310. doi:10.1007/s00468-006-0119-6.
- Coops, N. C., and R. H. Waring. 2001. “Estimating Forest Productivity in the Eastern Siskiyou Mountains of Southwestern Oregon Using a Satellite Driven Process Model, 3-PGS.” Canadian Journal of Forest Research 31 (1): 143–154. doi:10.1139/x00-146.
- Curtis, R. O. 1967. “Height-Diameter and Height-Diameter-Age Equations for Second Growth Douglas-Fir.” Forest Science 13: 365–375.
- Dubayah, R., and V. Van Katwijk. 1992. “The Topographic Distribution of Annual Incoming Solar Radiation in the Rio Grande River Basin.” Geophysical Research Letters 19: 2231–2234. doi:10.1029/92GL02284.
- Dubayah, R. C. 1994. “Modeling a Solar Radiation Topoclimatology for the Rio Grande River Basin.” Journal of Vegetation Science 5: 627–640. doi:10.2307/3235879.
- Duffie, J. A., and W. A. Beckman. 1991. Solar Engineering of Thermal Processes. New York: John Wiley & Sons.
- Duguay, C. 1993. “Radiation Modeling in Mountainous Terrain Review and Status.” Mountain Research and Development 13 (4): 339–357. doi:10.2307/3673761.
- Edson, C., and M. G. Wing. 2011. “Airborne Light Detection and Ranging (LiDAR) for Individual Tree Stem Location, Height, and Biomass Measurements.” Remote Sensing 3 (11): 2494–2528. doi:10.3390/rs3112494.
- Forster, B. C. 1984. “Derivation of Atmospheric Correction Procedures for LANDSAT MSS with Particular Reference to Urban Data.” International Journal of Remote Sensing 5: 799–817. doi:10.1080/01431168408948861.
- Frouin, R., D. W. Lingner, C. Gautier, K. S. Baker, and R. C. Smith. 1989. “A Simple Analytical Formula to Compute Clear Sky Total and Photosynthetically Available Solar Irradiance at the Ocean Surface.” Journal of Geophysical Research 94: 9731–9742. doi:10.1029/JC094iC07p09731.
- Gray, A. N., H. S. J. Zald, R. A. Kern, and M. North. 2005. “Stand Conditions Associated with Tree Regeneration in Sierran Mixed-Conifer Forests.” Forest Science 51 (3): 198–210.
- Heurich, M. 2008. “Automatic Recognition and Measurement of Single Trees Based on Data from Airborne Laser Scanning over the Richly Structured Natural Forests of the Bavarian Forest National Park.” Forest Ecology and Management 255 (7): 2416–2433. doi:10.1016/j.foreco.2008.01.022.
- Hyyppä, J., H. Hyyppä, D. Leckie, F. Gougeon, X. Yu, and M. Maltamo. 2008. “Review of Methods of Small-Footprint Airborne Laser Scanning for Extracting Forest Inventory Data in Boreal Forests.” International Journal of Remote Sensing 29 (5): 1339–1366. doi:10.1080/01431160701736489.
- Im, J., J. R. Jensen, and M. E. Hodgson. 2008. “Object-Based Land Cover Classification Using High-Posting-Density LiDAR Data.” GIScience & Remote Sensing 45 (2): 209–228. doi:10.2747/1548-1603.45.2.209.
- Kraus, K., and N. Pfeifer. 1998. “Determination of Terrain Models in Wooded Areas with Airborne Laser Scanner Data.” ISPRS Journal of Photogrammetry and Remote Sensing 53 (4): 193–203. doi:10.1016/S0924-2716(98)00009-4.
- Kreith, F., and J. F. Kreider. 1978. Principles of Solar Engineering. New York: McGraw-Hill.
- Kumar, L. 2009. “A Comparison of ArcGIS Modelled and Ground Recorded Solar Radiation Data an Opportunities for Utilisation in Environmental Models.” In Proceedings of the 10th South East Asian Survey Congress, Bali.
- Kumar, L., and A. K. Skidmore. 2000. “Radiation – Vegetation Relationships in an Eucalyptus Forest.” Photogrammetric Engineering and Remote Sensing 66 (2): 193–204.
- Kumar, L., A. K. Skidmore, and E. Knowles. 1997. “Modelling Topographic Variation in Solar Radiation in a Gis Environment.” International Journal of Geographical Information Science 11 (5): 475–497. doi:10.1080/136588197242266.
- Landsberg, J. J., and R. H. Waring. 1997. “A Generalised Model of Forest Productivity Using Simplified Concepts of Radiation-Use Efficiency, Carbon Balance and Partitioning.” Forest Ecology and Management 95 (3): 209–228. doi:10.1016/S0378-1127(97)00026-1.
- Li, D. 2013. “Using GIS and Remote Sensing Techniques for Solar Panel Installation Site Selection”. Master of Science, University of Waterloo, Waterloo.
- Loetsch, F., and K. E. Haller. 1973. Forest Inventory. Vol. II. München: BLV Verlagsgesellschaft mbH.
- Mason, E. G., R. Methol, and H. Cochrane. 2011. “Hybrid Mensurational and Physiological Modelling of Growth and Yield of Pinus radiata D. Don Using Potentially Useable Radiation Sums.” Forestry 84: 99–108. doi:10.1093/forestry/cpq048.
- McGaughey, R. J. 2007. Fusion Manual, version 2.90. http://www.fs.fed.us/
- Medlyn, B. E. 1998. “Physiological Basis of the Light Use Efficiency Model.” Tree Physiology 18: 167–176. doi:10.1093/treephys/18.3.167.
- Miehle, P., S. J. Livesley, C. Li, P. M. Feikema, M. A. Adams, and S. K. Arndt. 2006. “Quantifying Uncertainty from Large-Scale Model Predictions of Forest Carbon Dynamics.” Global Change Biology 12 (8): 1421–1434. doi:10.1111/j.1365-2486.2006.01176.x.
- Moeur, M. 1993. “Characterizing Spatial Patterns of Trees Using Stem-Mapped Data.” Forest Science 39: 756–775.
- Mummery, D., and M. Battaglia. 2002. “Data Input Quality and Resolution Effects on Regional and Local Scale Eucalyptus Globulus Productivity Predictions in North-East Tasmania.” Ecological Modelling 156 (1): 13–25. doi:10.1016/S0304-3800(02)00042-X.
- Neteler, M., and H. Mitasova. 2008. Open Source GIS: A GRASS GIS Approach. The International Series in Engineering and Computer Science. 3rd ed. New York: Springer.
- Petroselli, A. 2012. “LIDAR Data and Hydrological Applications at the Basin Scale.” GIScience & Remote Sensing 49 (1): 139–162. doi:10.2747/1548-1603.49.1.139.
- Popescu, S. C., and R. H. Wynne. 2004. “Seeing the Trees in the Forest: Using Lidar and Multispectral Data Fusion with Local Filtering and Variable Window Size for Estimating Tree Height.” Photogrammetric Engineering and Remote Sensing 70 (5): 589–604. doi:10.14358/PERS.70.5.589.
- R Development Core Team. 2011. R: A Language and Environment for Statistical Computing. Vienna: R foundation for statistical computing.
- Raaflaub, L. D., and M. J. Collins. 2006. “The Effect of Error in Gridded Digital Elevation Models on the Estimation of Topographic Parameters.” Environmental Modelling and Software 21: 710–732. doi:10.1016/j.envsoft.2005.02.003.
- Ruiz‐Arias, J. A., J. Tovar‐Pescador, D. Pozo‐Vázquez, and H. Alsamamra. 2009. “A Comparative Analysis of Dem‐Based Models to Estimate the Solar Radiation in Mountainous Terrain.” International Journal of Geographical Information Science 23 (8): 1049–1076. doi:10.1080/13658810802022806.
- Ryan, P. J., and G. I. Holmes. 1986. “Geology of Hanging Rock and Nundle State Forests”. Technical Paper No. 37, Sydney.
- Saremi, H., L. Kumar, R. Turner, and C. Stone. 2014a. “Airborne Lidar Derived Canopy Height Model Reveals a Significant Difference in Radiata Pine (Pinus radiata D. Don) Heights Based on Slope and Aspect of Sites.” Trees – Structure and Function 28 (3): 733–744. doi:10.1007/s00468-014-0985-2.
- Saremi, H., L. Kumar, R. Turner, C. Stone, and G. Melville. 2014b. “Impact of Local Slope and Aspect Assessed from Lidar Records on Tree Diameter in Radiata Pine (Pinus radiata D. Don) Plantations.” Annals of Forest Science. doi:10.1007/s13595-014-0374-4.
- Snowdon, P., D. Eamus, P. Gibbons, P. K. Khanna, H. Keith, R. J. Raison, and M. U. F. Kirschbaum. 2000. “Synthesis of Allometrics, Review of Root Biomass and Design of Future Woody Biomass Sampling Strategies.” National Carbon Accounting System Technical Report 17. Canberra: Australian Greenhouse Office.
- Suri, M., and J. Hofierka. 2004. “A New GIS-Based Solar Radiation Model and its Application to Photovoltaic Assessments.” Transactions in GIS 8 (2): 175–190. doi:10.1111/j.1467-9671.2004.00174.x.
- Tovar-Pescador, J., D. Pozo-Vázquez, J. A. Ruiz-Arias, J. Batlles, G. López, and J. L. Bosch. 2006. “On the Use of the Digital Elevation Model to Estimate the Solar Radiation in Areas of Complex Topography.” Meteorological Applications 13 (3): 279–287. doi:10.1017/S1350482706002258.
- Turner, R., C. Stone, A. Kathuria, and T. Penman. 2011. “Towards an Operational Lidar Resource Inventory Process in Australian Softwood Plantations.” In Proceedings of the 34th International Symposium for Remote Sensing of the Environment (ISRSE), Sydney.
- Turner, R. E., and M. M. Spencer. 1972. “Atmospheric Model for Correction of Spacecraft Data.” In Proceedings of 8th international symposium on remote sensing of the environment, 895–934. Ann Arbor: Environmental Research Institute of Michigan.
- Turner, R. S. 2006. “An Airborne Lidar Canopy Segmentation Approach for Estimating Above-Ground Biomass in Coastal Eucalypt Forests”. PhD, University of New South Wales, Sydney.
- Van Laar, A., and A. Akça. 2007. Forest Mensuration (Managing Forest Ecosystems #13), Vol. 13, 383 p. 2nd ed. Dordrecht: Springer.
- Watt, M., A. Meredith, P. Watt, and A. Gunn. 2013. “Use of Lidar to Estimate Stand Characteristics for Thinning Operations in Young Douglas-Fir Plantations.” New Zealand Journal of Forestry Science 43 (1): 18. doi:10.1186/1179-5395-43-18.
- Watt, M. S., and M. U. F. Kirschbaum. 2011. “Moving beyond Simple Linear Allometric Relationships between Tree Height and Diameter.” Ecological Modelling 222 (23–24): 3910–3916. doi:10.1016/j.ecolmodel.2011.10.011.
- White, J. C., M. A. Wulder, M. Vastaranta, N. C. Coops, D. Pitt, and M. Woods. 2013. “The Utility of Image-Based Point Clouds for Forest Inventory: A Comparison with Airborne Laser Scanning.” Forests 4 (3): 518–536. doi:10.3390/f4030518.
- Woollons, R. C., P. Snowdon, and N. D. Mitchell. 1997. “Augmenting Empirical Stand Projection Equations with Edaphic and Climatic Variables.” Forest Ecology and Management 98 (3): 267–275. doi:10.1016/S0378-1127(97)00090-X.
- Yu, X., J. Hyyppä, M. Vastaranta, M. Holopainen, and R. Viitala. 2011. “Predicting Individual Tree Attributes from Airborne Laser Point Clouds Based on the Random Forests Technique.” ISPRS Journal of Photogrammetry and Remote Sensing 66 (1): 28–37. doi:10.1016/j.isprsjprs.2010.08.003.