ABSTRACT
Background: ‘Epigenetic clocks’ have been developed to accurately predict chronologic gestational age and have been associated with child health outcomes in prior work.
Methods: We meta-analysed results from four prospective U.S cohorts investigating the association between epigenetic age acceleration estimated using blood DNA methylation collected at birth and preschool age Childhood Behavior Checklist (CBCL) scores.
Results: Epigenetic ageing was not significantly associated with CBCL total problem scores (β = 0.33, 95% CI: −0.95, 0.28) and DSM-oriented pervasive development problem scores (β = −0.23, 95% CI: −0.61, 0.15). No associations were observed for other DSM-oriented subscales.
Conclusions: The meta-analysis results suggest that epigenetic gestational age acceleration is not associated with child emotional and behavioural functioning for preschool age group. These findings may relate to our study population, which includes two cohorts enriched for ASD and one preterm birth cohort.; future work should address the role of epigenetic age in child health in other study populations.
Abbreviations: DNAm: DNA methylation; CBCL: Child Behavioral Checklist; ECHO: Environmental Influences on Child Health Outcomes; EARLI: Early Autism Risk Longitudinal Investigation; MARBLES: Markers of Autism Risk in Babies – Learning Early Signs; ELGAN: Extremely Low Gestational Age Newborns; ASD: autism spectrum disorder; BMI: body mass index; DSM: Diagnostic and Statistical Manual of Mental Disorders.
Introduction
Over 8 million children ages 3–17 y in the US were diagnosed with a mental or behavioural health condition in 2018–2019 [Citation1]. Studies have shown that early identification and intervention is associated with better long-term health outcomes among affected individuals [Citation2]. However, most neurodevelopmental disorders are not diagnosed until children reach 3–5 y of age at the earliest and typically diagnoses occur in mid-childhood. There is a critical need for biomarkers at the earliest stages of life to improve the identification of children at risk.
In recent years, biologic ageing as measured using ‘epigenetic clocks’ or DNA methylation (DNAm)-based age estimators has been robustly associated with prospective overall mortality and disease-specific risk in adults [Citation3,Citation4], showing promise as early biomarkers of prospective health outcomes. The difference between chronologic age and predicted epigenetic age captures the age discordance of the individual. Over the past few years, epigenetic clocks robustly predicting gestational age [Citation5–7] have been developed and studied in association with newborn and child health outcomes. Despite lower gestational age being associated with developmental delays in children [Citation8–10], only two European studies have examined the relationship between epigenetic ageing and cognitive and behavioural traits during childhood [Citation11,Citation12]. One study reported that epigenetic gestational age deceleration estimated using cord blood DNAm was associated with increased internalizing problems and overall problems measured by the Child Behavior Checklist (CBCL) at age 4 in boys in a large Finnish cohort (n = 407) [Citation12], while the second study observed an increased odds of psychiatric problems with blood epigenetic age acceleration collected between 11 and 13 y old in a different cohort of 239 Finnish adolescents [Citation11]. However, a recent U.S study did not observe an association between epigenetic gestational age acceleration at birth and developmental delay at age 3 in a cohort of 1010 children [Citation13]. To our knowledge, no studies have evaluated the relationship between epigenetic age at birth and specific child emotional and behavioural traits in early childhood in a U.S population nor have studies considered CBCL subscale scores that may be more relevant to Diagnostic and Statistical Manual of Mental Disorders (DSM) mental disorder diagnoses.
In this study, we conducted a meta-analysis investigating the association between epigenetic age acceleration at birth and later DSM-oriented CBCL subscales and total score from four prospective U.S cohorts participating in the National Institutes of Health Environmental Influences on Child Health Outcomes (ECHO) program.
Methods
Study population
Existing prospective cohorts in the United States participating in the ECHO program were invited to participate in the current study if they had the following information before April 2021 when this study was conducted 1) DNA methylation data measured in cord or peripheral blood collected at birth from singleton infants 2) preschool age CBCL data measured. Four cohorts contributed to this meta-analysis of epigenetic age at birth and CBCL outcomes in early childhood: Early Autism Risk Longitudinal Investigation (EARLI)[Citation14], Markers of Autism Risk in Babies – Learning Early Signs (MARBLES)[Citation15], Extremely Low Gestational Age Newborns (ELGAN)[Citation16], and the Healthy Start Study[Citation17]. The EARLI and MARBLES studies are prospective studies of autism spectrum disorder (ASD) that utilizes a familial history design, i.e., it enrolled pregnant women who had a previous child with ASD, while the ELGAN study recruited women who gave birth before 28 weeks gestational age. Detailed methods for each cohort are provided in Supplementary Material.
DNA methylation measures
Umbilical cord blood or peripheral blood biosamples were collected shortly after delivery using standardized protocol at each study sites. DNA was bisulphite converted using an EZ-96 DNA methylation kit (Zymo Research, Irvine, CA). Each cohort measured DNA methylation using the Infinium HumanMethylation450 BeadChip array or Infinium HumanMethylationEPIC BeadChip array (Illumina, San Diego, CA) in cohort-specific laboratories. Quality control (QC) of samples was performed by each cohort and failed samples were excluded based on high detection p value, low sample DNA concentration, sample call rate, CpG-specific percentage of missing values, bisulphite conversion efficiency, sex verification, and other quality control metrics specific to cohorts. The data were then further processed for background correction and normalization in each cohort. Cell type proportions were estimated using a combined cord blood reference data set in each cohort [Citation18–23]. More details on quality control, normalization, and cell-type proportions are provided in the Supplemental Materials.
Epigenetic age calculation
Each cohort derived epigenetic age at birth according to a pre-specified analysis plan independently using algorithms published by Knight [Citation6]. The Knight method used an elastic net approach with 10-fold cross-validation in the training set and identified a model consisting of 148 CpG sites that can estimate epigenetic gestational age. The estimation of epigenetic age using the Knight clock was performed using R statistical software with codes supplied by Knight et al. Due to the cohort-specific QC filters applied to DNA methylation data, some CpG sites required to compute the Knight clock may have been missing. If a required CpG for the clock computation was filtered out, methylation values were either imputed using simple random sampling imputation or substituted from the closest CpG sites (less than 2000 base pairs). Epigenetic age acceleration was then derived as the residual from a linear model of epigenetic age regressed on gestational age adjusting for cell-type proportions.
CBCL assessment
In each cohort, the primary caregiver of the child or an interviewer completed the CBCL 1.5–5 questionnaire at the age of 2–3 y. Respondents choose one of three responses to the 99 characteristics listed in the CBCL: ‘not true,’ ‘somewhat or sometimes true,’ and “very true or often true. The normalized T-scores, including total problem score and DSM-oriented subscale scores (attention-deficit hyperactivity disorder problems, anxiety problems, oppositional defiant problems, pervasive development problems), were calculated, and analysed as the primary outcomes, as these are more clinically interpretable than raw scores.
Statistical analysis
Each cohort completed independent analysis to evaluate the relationship between epigenetic age acceleration at birth and CBCL outcomes in early childhood according to a pre-specified analysis plan. Descriptive analyses were conducted to examine the maternal and child characteristics and the distribution of epigenetic age/epigenetic age acceleration. Spearman correlation coefficients between chronologic gestational age, estimated epigenetic age, and epigenetic age acceleration/deceleration across Knight clock were calculated. Adjusted multivariable linear regression models were completed to evaluate the association between continuous epigenetic age acceleration at birth and continuous CBCL outcomes, adjusted for ancestry principal components (if available), maternal education, prenatal smoking exposure, maternal pre-pregnancy body mass index (BMI), and child sex. If ancestry principal components were not available, self-reported race/ethnicity was used. Missingness across covariates in each cohort was low (<2%). Subjects with missing covariates were excluded from the cohort-specific analysis. Binary CBCL outcomes based on clinical relevant cut-offs were also examined individually for each of the CBCL outcomes (≥65 vs <65 for total problem T-score and ≥60 vs <60 for DSM-oriented subscales) using adjusted logistic regression models. Random-effects meta-analyses were conducted using the results from cohort-specific analyses, which accounts for between- and within-study variance, allowing for the possibility that individual studies estimated different effect sizes. Forest plots were generated to display each study’s contribution to each summary estimate. Potential heterogeneity between studies was assessed using the Cochran Q statistic and I2. Additionally, leave-one-out and cumulative meta-analyses were conducted to evaluate the contribution of each study to the results. All meta-analyses were implemented in R 3.6 (R Project for Statistical Computing).
Results
Characteristics of participating studies are shown in Table S1. Due to the inclusion of a preterm birth cohort and cohorts enriched for a family history of autism, the mean (SD) gestational age ranged from 26.2 (1.2) weeks to 39.5 (1.2). DNAm estimates of epigenetic age, derived using the Knight clock algorithm for blood samples, showed significant positive overall correlations with chronologic gestational age (Figure S1, ranged from 0.38 to 0.62, P < 0.001 for all cohorts).
The summary estimates of the association between epigenetic age acceleration at birth and CBCL total problem scores and DSM-oriented pervasive development problem scores were −0.33 (95% CI: −0.95, 0.28) and −0.23 (95% CI: −0.61, 0.15), respectively (), based on all four studies. Between-study heterogeneity among all studies was not statistically significant (P = 0.81). Effect estimates were similar in directions and magnitudes across the leave-one-out and cumulative meta-analyses. We did not observe any significant associations between epigenetic age acceleration at birth and other DSM-oriented subscale of CBCL scores (Figure S2) nor when stratified by child sex. Sensitivity analyses examining binary CBCL outcomes did not yield materially changed results from the primary meta-analysis results.
Figure 1. Results of random effects meta-analyses of the associations between epigenetic age acceleration at birth estimated by Knight clock and childhood behavior checklist a) total-T score b) pervasive development problems assessed in early childhood. Results of individual studies were from multivariable linear regression models, which adjusted for child sex, maternal education, maternal smoking during pregnancy, and maternal pre-pregnancy BMI status. Point estimates of individual study beta estimates are shown as black squares; area of squares indicates relative weights of individual studies; point and interval overall ratio estimates are horizontal apices of black diamonds.
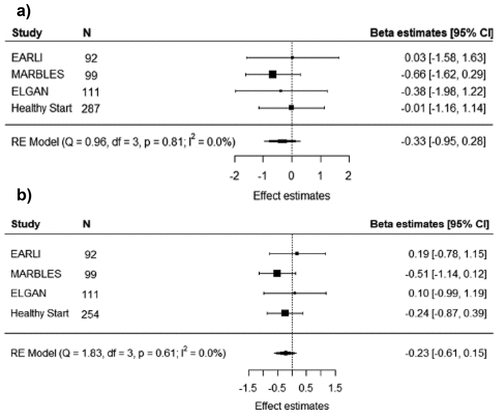
Discussion
In this meta-analysis, we observed that epigenetic gestational age acceleration was not associated with measures of child emotional and behavioural development overall or stratified by child sex in participants from four U.S cohorts.
Previous work has suggested greater total or internalizing problems with decelerated epigenetic age at birth in boys but not in girls or in combined samples [Citation12]. We did not observe significant associations overall or by child sex in the current meta-analyses. It is possible that we are not powered to detect smaller magnitude changes due to our relatively small sample size stratified by child sex and sample characteristics. Our point estimates for total score, oppositional defiant problems, and pervasive development problems association in the full study population are in the same direction as the European study and consistently indicate an inverse relationship between epigenetic age acceleration and child emotional and behaviour problems. Results from one of the familial enriched studies showed suggestive associations for DSM-oriented subscales, suggesting those with familial ASD risk may be impacted in multiple domains and biological ageing as measured by epigenetic age may be relevant to child neurodevelopment. Due to our sample size, we were not permitted to run meta-analysis among familial enriched studies alone. Although we speculate that the lack of association was due to differences in cohort and sample characteristics, the statistics of heterogeneity in the meta-analysis were not significant for most of the outcome measurements. To our knowledge, this is the first study examining the association between epigenetic age acceleration and emotional and behavioural development in early childhood in a non-European population. Our study included participants from four well-characterized prospective cohorts with diverse racial and ethnic backgrounds drawn from both high-risk and general populations. Whether mechanisms underlying these relationships differ by genetic background or by familial risk remains unclear. Understanding the impact of genetic factors on biologic age and its potential impact on child cognitive and behavioural development in general population stratified by low or high familial risk should be an area of future research.
Differences in socio-economic status (SES) between our study population and previous studies may contribute to the mixed findings. For example, the association between epigenetic ageing and child emotional and behavioural development may be modified by SES-related factors such as neighbourhood deprivation. It is also possible that nutrition or maternal history of depression or other parental mental health conditions might influence child neurodevelopment and be associated with epigenetic ageing at birth, for which we were unable to control in this study. Future studies with larger sample size would benefit from incorporating social factors related to environmental exposures and maternal/paternal mental health. In addition, we cannot rule out limited generalizability of our findings, which may be limited to those with increased familial likelihood of ASD or high risk of developmental delays.
Here, we examined cell-intrinsic ageing by adjusting for proportions of estimated blood cell types, as age-related health changes may shift blood composition resulting in differences in measures of epigenetic ageing. We did not explore second generation epigenetic clocks that incorporate lifestyle or clinical factors. Epigenetic clocks in tissues relevant to early life exposures and epigenetic clocks sensitive to health outcomes associated with clinical markers have been developed to accurately capture the biological process of ageing and age-related epigenetic shift [Citation4,Citation7]. Extrinsic or environmental factors could have influenced age-related shifts in cord blood cells and be related to child development. Future research utilizing these second generation epigenetic clocks will be informative.
Lastly, this meta-analysis included methylation data from both 450K platform and EPIC platform. Each cohort performed sample collection, DNA methylation extraction and measurement, quality control and normalization procedures according to their existing protocols. We were not able to standardize the sampling process and DNA methylation harmonization from different platforms and cohorts. It is possible that pre-processing and QC steps performed by each cohort could introduce between-sample variation and further influence meta-analysis results. However, previous studies have reported that epigenetic age acceleration estimated using different pre-processing and normalization methods was relatively consistent across platforms [Citation24], so we do not expect this to strongly impact the final meta-analysis results.
In summary, in this meta-analysis including four cohorts participating in the ECHO program, epigenetic age acceleration at birth was not associated with child emotional and behavioural problems measured by CBCL scores in early childhood. However, the null results should be interpreted in the context of our study population, which includes two cohorts enriched for ASD and one preterm birth cohort. Our findings suggest that there is a need to further investigate the associations with other standardized child cognitive and behavioural developmental measures in both high risk and general populations and in larger samples that permit examination of sex differences and the additional role of environmental and genetic factors in these relationships.
Competing interests
The authors have no conflicts of interest relevant to this manuscript to disclose. Dr. Ladd-Acosta would like to report receiving consulting fees from the University of Iowa for providing expertise on autism spectrum disorder, outside of this work.
Ethics approval and consent to participate
The institutional review boards (IRB) at organizations in each study site approved each study. All participants provided written informed consent.
Consent for publications
All authors have reviewed this manuscript and approved its publication.
Availability of data and materials
Data used for these analyses may be available through approval from individual study investigators and the Environmental Influences on Child Health Outcomes (ECHO) program.
Authors’ contribution
AS, HV, and CL-A conceptualized and designed the project. AS, CB, and SN performed the cohort-specific analysis. AS performed meta-analysis AS, HV, and CL-A drafted the manuscript. CB, KK, KB, CM, MO, RF, KL, and DF provided the manuscript revisions and editing. All authors reviewed the results and approved the submitted version of the manuscript.
Supplemental Material
Download MS Word (1.6 MB)Disclosure statement
No potential conflict of interest was reported by the author(s).
Supplementary material
Supplemental data for this article can be accessed online at https://doi.org/10.1080/15592294.2023.2254971.
Correction Statement
This article has been republished with minor changes. These changes do not impact the academic content of the article.
Additional information
Funding
References
- Bureau TUSC. Child and Adolescent Health Measurement Initiative. 2018-2019 National Survey of Children’s Health (NSCH) data query. Data Resource Center for Child and Adolescent Health supported by the U.S. Department of Health and Human Services, Health Resources and Services Administration (HRSA), Maternal and Child Health Bureau (MCHB)., 2020. [cited 2023 May 9]. www.childhealthdata.org
- Gleason MM, Goldson E, Yogman MW, et al. Addressing early childhood emotional and behavioral problems. Pediatrics. 2016;138(6). doi: 10.1542/peds.2016-3025
- Levine ME, Lu AT, Quach A, et al. An epigenetic biomarker of aging for lifespan and healthspan. (Albany NY). Aging. 2018;10(4):573–7. doi: 10.18632/aging.101414
- Lu AT, Quach A, Wilson JG, et al. DNA methylation GrimAge strongly predicts lifespan and healthspan. aging (Albany NY). Aging. 2019;11(2):303–327. doi: 10.18632/aging.101684
- Bohlin J, Håberg SE, Magnus P, et al. Prediction of gestational age based on genome-wide differentially methylated regions. Genome Biol. 2016;17(1):207. doi: 10.1186/s13059-016-1063-4
- Knight AK, Craig JM, Theda C, et al. An epigenetic clock for gestational age at birth based on blood methylation data. Genome Biol. 2016;17(1):206. doi: 10.1186/s13059-016-1068-z
- Lee Y, Choufani S, Weksberg R, et al. Placental epigenetic clocks: estimating gestational age using placental DNA methylation levels. (Albany NY). Aging. 2019;11(12):4238–4253. doi: 10.18632/aging.102049
- Aarnoudse-Moens CS, Weisglas-Kuperus N, van Goudoever JB, et al. Meta-analysis of neurobehavioral outcomes in very preterm and/or very low birth weight children. Pediatrics. 2009;124(2):717–728. doi: 10.1542/peds.2008-2816
- Potijk MR, de Winter AF, Bos AF, et al. Higher rates of behavioural and emotional problems at preschool age in children born moderately preterm. Arch Dis child. Arch Dischildhood. 2012;97(2):112–117. doi: 10.1136/adc.2011.300131
- Hochstedler KA, Bell G, Park H, et al. Gestational age at birth and risk of developmental delay: the upstate KIDS study. Am J Perinatol. 2021;38(10):1088–1095. doi: 10.1055/s-0040-1702937
- Suarez A, Lahti J, Czamara D, et al. The epigenetic clock and pubertal, neuroendocrine, psychiatric, and cognitive outcomes in adolescents. Clin Epigenetics. 2018;10(1):96. doi: 10.1186/s13148-018-0528-6
- Suarez A, Lahti J, Czamara D, et al. The epigenetic clock at birth: associations with maternal antenatal depression and child psychiatric problems. J Am Acad Child Adolesc Psychiatry. 2018;57(5):321–328.e2. doi: 10.1016/j.jaac.2018.02.011
- Polinski KJ, Robinson SL, Putnick DL, et al. Epigenetic gestational age and the relationship with developmental milestones in early childhood. Hum Mol Genet. 2023;32(9):1565–1574. doi: 10.1093/hmg/ddac302
- Newschaffer CJ . Infant siblings and the investigation of autism risk factors. J Neurodevelop Disord. 2012;4(1). doi: 10.1186/1866-1955-4-7
- Hertz-Picciotto I. A Prospective Study of Environmental Exposures and Early Biomarkers in Autism Spectrum Disorder: Design, Protocols, and Preliminary Data from the MARBLES Study. Environ Health Perspect. 2018;126(11). doi: 10.1289/EHP535
- O'Shea T, Allred E, Dammann O, et al. The ELGAN study of the brain and related disorders in extremely low gestational age newborns. Early Human Development. 2009;85(11): 719–725. doi: 10.1016/j.earlhumdev.2009.08.060
- Harrod C S, Fingerlin T E, Chasan-Taber L, et al. Exposure to prenatal smoking and early-life body composition: The healthy start study. Obesity. 2015;23(1): 234–241. doi: 10.1002/oby.20924
- Salas LA. FlowSorted.Blood.epic: Illumina EPIC data on immunomagnetic sorted peripheral adult blood cells. R package version 2.0.0. 2022.
- Bakulski KM, Feinberg JI, Andrews SV, et al. DNA methylation of cord blood cell types: applications for mixed cell birth studies. Epigenetics. 2016;11(5):354–362. doi: 10.1080/15592294.2016.1161875
- Gervin K, Salas LA, Bakulski KM, et al. Systematic evaluation and validation of reference and library selection methods for deconvolution of cord blood DNA methylation data. Clin Epigenetics. 2019;11(1):125. doi: 10.1186/s13148-019-0717-y
- de Goede OM, Razzaghian HR, Price EM, et al. Nucleated red blood cells impact DNA methylation and expression analyses of cord blood hematopoietic cells. Clin Epigenetics. 2015;7(1):95. doi: 10.1186/s13148-015-0129-6
- Gervin K, Page CM, Aass HCD, et al. Cell type specific DNA methylation in cord blood: a 450K-reference data set and cell count-based validation of estimated cell type composition. Epigenetics. 2016;11(9):690–698. doi: 10.1080/15592294.2016.1214782
- Lin X, Tan JYL, Teh AL, et al. Cell type-specific DNA methylation in neonatal cord tissue and cord blood: a 850K-reference panel and comparison of cell types. Epigenetics. 2018;13(9):941–958. doi: 10.1080/15592294.2018.1522929
- McEwen LM, Jones MJ, Lin DTS, et al. Systematic evaluation of DNA methylation age estimation with common preprocessing methods and the Infinium MethylationEPIC BeadChip array. Clin Epigenetics. 2018;10(1):123. doi: 10.1186/s13148-018-0556-2