ABSTRACT
Recent developments in machine translation (MT) might have led some people to believe that soon professional translation will not be needed, but most translator trainers are aware of the high demand for the quality that MT systems cannot deliver without human intervention. It is thus important that professional translators, trainers and their students appreciate when and how MT can best be deployed, even if they do not use it much themselves. This can be accomplished by enhancing their MT literacy, which encompasses an understanding of the basics, risks and benefits of the technology. Trainers can prepare their students to provide advice to clients who might be interested in using MT for their multilingual content but do not have the expertise to judge when it would be enough to meet their needs. Drawing on the example of knowledge dissemination in higher education, this article presents survey results that suggest MT is being used far more widely than previously assumed. We highlight some of the risks associated with uninformed use of this technology, discuss how they can be mitigated by translation professionals with consulting competence, and outline some training scenarios which could contribute to developing societal AI literacy in general.
1. Introduction
According to key monitors, the language industry has been booming recently and is estimated to continue that way, with healthy rates of growth for services into the foreseeable future (e.g. Nimdzi Citation2022a; Slator Citation2022). This sounds like good news for translators and translation trainers, who might be inclined to focus their efforts on the high-end, prestige markets that value human creativity and ethics as well as the client-, audience- and risk-awareness that language professionals bring to the table. However, we argue that professional translators and trainers with a high degree of MT literacy are also well-placed to provide consulting services to clients who do not have the background or linguistic expertise to judge what combination of (post-edited) MT output and professional translation might be appropriate for their multilingual content.
The need to include training in post-editing and MT in various components of translation programmes was identified early on (e.g. Gaspari, Almaghout, and Doherty Citation2015; Mellinger Citation2017; O’Brien Citation2002) and calls for it have become more persistent in Europe with the prominence afforded to the Technology component of the EMT (Citation2022) competence framework. As the quality of NMT has improved so much since Google Translate first offered it in 2016, many computer-aided translation (CAT) solutions are now including the option of MT when there are no translation memory (TM) suggestions of the minimum match level. This development is blurring the distinction between TM and MT for many less informed users and, at least when technology is involved, changing the task of translation to primarily (post-)editing. This type of information is included in many translator training programmes and would be an excellent way to introduce the notion of MT literacy to future language professionals, who may otherwise think that knowing how to use MT output (or not) for their own purposes is all they are supposed to be taking away from their translation technology courses.Footnote1
Rather than treating MT as a threat, translators with a high degree of MT literacy realise that existing and future tools can be combined with human intelligence to increase productivity and job satisfaction. Constant adaptation and acquisition of new skills are indispensable for a language professional’s competence profile, or as Van der Meer (Citation2020, 308) put it: ‘The role of professional translators will not vanish, but it will evolve – again – through technology’. Way (Citation2020, 327) and othersFootnote2 refer to the importance of the ‘human-in-the-loop’ in any type of translation process, and DePalma (Citation2020) is even more explicit about who should be in charge. He discusses the notion of augmented translation intelligence, pointing out that tools should be inserted where appropriate but that language professionals should be in the centre and control the flow of the translation process.
MT literacy and post-editing skills can prepare translators to cope with future developments in AI and language technology as well as to provide advice to clients when time and resources are too tight for prestige translation services. With their cultural, genre and service provision expertise, translators with MT literacy would be in an excellent position to act as consultants, but they need to understand what MT literacy looks like for different target audiences. Assessing how much, what kind and how MT-related information should be conveyed requires oral communication and didactic skills, which have often been neglected in traditional translator training programmes. Knowing how to use MT for their daily work does not automatically mean knowing how to educate other users about it.
In the following, we sketch out how ubiquitous the use of MT might already be in many sectors by presenting relevant results of a large-scale survey carried out at numerous universities in a multilingual country in which language teaching has been a high priority at all levels of education for decades. We explain what MT literacy is (cf. Bowker and Buitrago Ciro Citation2019), how it can help manage expectations for high-quality translation on demand, and how trainers can leverage their own MT literacy to empower their students not only to use this technology in an informed way but also to advise others on appropriate use as well.
2. Importance of translation in knowledge dissemination
Most scholars agree that translation is everywhere we look and indeed that translation has been involved in much if not most theorising and knowledge dissemination throughout history (see Blumczynski Citation2017). Whereas literate multilinguals were responsible for producing those translations until the beginning of the 21st century, neural machine translation (NMT) engines started generating very convincing output in many language combinations as of 2015 (see Slator Citation2019 or Way Citation2020 for helpful overviews). Most people are unaware that much of what they read is the product of human or machine translation processes, although some have started to deliberately use the translation options offered by computer browsers and social media platforms.
One of the most important players in the management of the translation, language and speech data that feed into MT solutions is TAUS,Footnote3 the Translation Automation User Society. As its CEO Van der Meer (Citation2021) has pointed out: ‘[t]ranslation as a social good, not owned by anyone and free to the user, exists alongside translations owned and paid for by governments and corporations’. His claim that the industry is heading to ‘singularity’ (i.e. human translators no longer involved in the process) by 2030 has been challenged (cf. Melby and Kurz Citation2021) but does suggest that MT is being relied on for multilingual content in more contexts than many in our discipline have realised (or would like to acknowledge). In the following sections, we present a study that explores how widespread the use of MT is in higher education, a context not usually considered a major consumer of language services.
2.1. Potential for MT in knowledge dissemination
Researchers in various disciplines are under increasing pressure to publish (or perish) in high-prestige journals that are usually in English, the current lingua franca of science. The improvements in MT quality that have been widely broadcast in the media (e.g. Hassan et al. Citation2018) might provide a tempting alternative to the effort required to improve their own language competence for both scientists and the media professionals who specialise in reporting science. For certain language combinations, the raw output from some of the freely available systems is often convincing (and misleadingly fluent; cf. Martindale and Carpuat Citation2018), so might be seen as a quick, economical, accessible alternative to involving a third party who has the competence to provide professional translation.
Typical problems in raw MT output that we have identified for the language combinations we work with can be categorised as related to accuracy, adaptation, coherence and conventions (see ). Some of these problems would be very difficult to detect if the reader has access only to the MT output (e.g. omissions, wrong word choice, inappropriate translations of proper nouns, wrong pronoun use, wrong abbreviations) and could result in a misleading text. Others might be obvious but not particularly problematic for someone with subject area expertise (e.g. repetitions, lack of cultural adaptation, wrong word form, wrong punctuation symbols). However, some might cause so much confusion (e.g. word inventions, non-translations, lack of or incorrect links, inconsistent use of terminology) that even an informed reader might be hard pressed to derive the intended meaning.
Table 1. Typical problems in raw MT output.
For example, the raw NMT output for a university press releaseFootnote4 about a new process for brewing beer could easily be revised by someone who knows about the subject area to achieve a more coherent, comprehensible text (see , with problems highlighted). This is often the case for combinations involving well-resourced languages, especially if the MT engine has been well trained.
Press releases about scientific results tend to be written in more accessible language than the actual journal articles, which MT engines sometimes handle less well. For example, part of a sentence of an abstract written in English was simply omitted in the German NMT output (see ), perhaps because of the complexity and density of the source text. In this particular case, the omitted clause was very important because it summarised the main finding of the study. These types of problems are a challenge to identify and remedy even for trained bilingual post-editors, not to mention those who rely on MT because they are only familiar with one of the languages in the combination.
To assess whether MT is being used for such high-register academic texts, a consortiumFootnote5 of researchers came together with the common objective of determining: a) how widespread the use was in universities in their country; and b) whether the university staff and students were using it in an informed way. Their interest was triggered by several initiatives focusing on digitalisation and digital skills in higher education (e.g. van Laar et al. Citation2019) as well as the increasing importance of MT in society in general (e.g. Kenny Citation2022; Vieira et al. Citation2022; Vieira, O’Hagan, and O’Sullivan Citation2021) and, in light of the recent pandemic, crisis communication in particularFootnote6 (e.g. O’Brien and Federico Citation2020). The method chosen for this survey of the use of MT in university settings was an online questionnaire, as described in the next section.
2.2. A survey of the use of MT by university researchers
A review of the literature, web resources and contact with colleagues in the disciplineFootnote7 provided us with an excellent basis for devising questions to assess the use and awareness of MT as well as attitudes towards it (e.g. Bowker Citation2019, Citation2020a, Citation2020b; Nurminen Citation2019; O’Brien and Ehrensberger-Dow Citation2020; O’Brien, Michel, and Marie-Josée Citation2018). It comprised up to 250 choices, depending on the respondent’s profile and branching path taken. The majority of those items were closed questions with yes/no/NA, multiple answer options, or slider scales. In addition to text fields if the option ‘other’ was chosen, there were some open questions that allowed the respondents to provide more information. There were no compulsory items other than the consent form and a filtering question to ensure that the respondent was affiliated with one of the country’s institutes of higher education.Footnote8
The questionnaire was prepared by a multilingual team in English with a view to translation and then translated by professionals into three other languages (i.e. German, French, Italian) in order to maximise the probability of respondents in our country being able to access it in their L1. The professional translators served as the first pilot testers, and their feedback was incorporated into all four versions. Interestingly, they said that they had learned a lot, and we realised that the survey had inherent educational potential for respondents. The first data collection phase was in the spring of 2021, when the online questionnaire was distributed through multipliers at the four universities of the consortium, and the second was in the fall of 2021, when it was disseminated more widely within the country. The interest was higher among the consortium universities (about 3,500 respondents) than among the other universities (about 2,500 respondents), resulting in a total sample of just over 6,000 respondents. Of those who specified, 51% comprised various categories of students (BA, MA, PhD), 43% had staff profiles (researchers, teachers, academic support), and 6% said they were both. For the purposes of considering MT in knowledge dissemination, we focus on the responses of the 1,196 members of staff who self-identified as researchers (i.e. those that chose ‘mainly research’, ‘teaching and research’, or ‘mainly research and academic support’ in response to the question ‘What do you spend most of your time doing at the university’). All of the questions concerning use of MT were optional and many allowed multiple answers, so all of the percentages reported below are the proportion of the total number of researchers.
An overwhelming majority of the researchers responded in the affirmative to the question of whether they had ever used a machine translation system (i.e. 81%), but they also tend to think that most university staff are not aware of the risks of using MT (i.e. 54%; compared to 47% of other staff and 37% of the students). Not surprisingly, about half of the researchers said that they use MT because it is fast, free and easy, but almost 40% also said that they did so because of its good quality.
A majority (i.e. about two-thirds) said that they use MT from their dominant language into a non-dominant language or vice versa, but about 35% also said they use it to translate between two non-dominant languages. When asked to provide more specific information (see ), over half of the researchers said that they used MT to translate individual words and over 40% to find alternate translations. Over 40% of the researchers said they use MT either to process (i.e. understand or read) or to produce (i.e. write) texts, and almost 25% said they use it to improve their language skills.
Figure 3. Reported use of MT by university researchers in a nationwide survey (% of respondents, multiple responses possible).
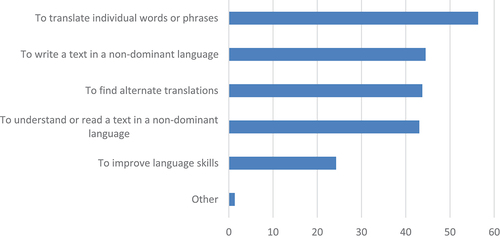
In response to the question of what types of documents they translate using MT, the researchers’ responses were similar to the rest of the sample in that email was mentioned most frequently (see ). Combined with the relatively high percentages of responses to the other types of documents related to research (i.e. articles, reports, presentations), these results suggest that researchers in universities in this particular country (i.e. Switzerland) are relying quite heavily on MT to do their core work of creating and disseminating knowledge.
Figure 4. Types of documents translated by university researchers using MT, according to a nationwide survey (% of respondents, multiple responses possible).
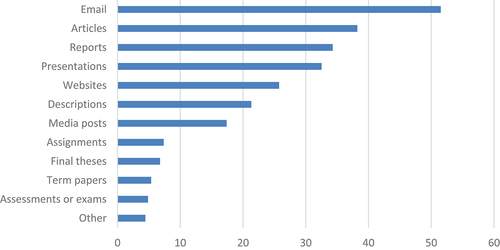
Only about 25% or fewer of the researchers indicated that they had concerns about using MT systems (e.g. security or altered or distorted content or style; see top half of ). Although a small percentage said MT had blocked their ideas, more seemed quite positive about the effects they had experienced using MT (i.e. inspiring ideas, new ways of expression, enlightenment about content in other languages; see bottom half of ).
Figure 5. Concerns and effects of using MT, according to university researchers in a nationwide survey (% of respondents, multiple responses possible).
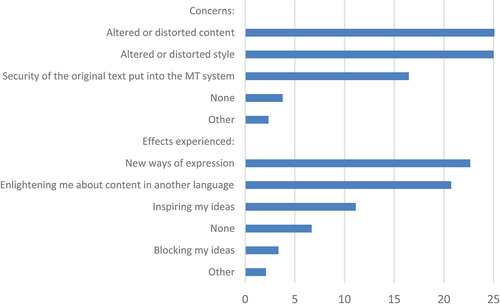
When asked specifically about the probability of various possible repercussions of using MT, however, the researchers were more cautious. The average probabilities predicted by the respondents for issues involving misunderstanding, miscommunication, academic integrity and reputation were about or over 50% (see ). They considered intellectual property, legal and ethical issues to be less likely, perhaps because so many of them reported using MT to understand texts and not necessarily to produce them (see ). This stands in contrast to several findings on teachers’ concerns regarding plagiarism issues (e.g. Vinall and Hellmich Citation2021) or the fear that students using MT might graduate ‘without having demonstrated the ability to communicate effectively in the language of instruction’ (Groves and Mundt Citation2021, 8).
Figure 6. Repercussions of using MT, according to university researchers in a nationwide survey (average probability).
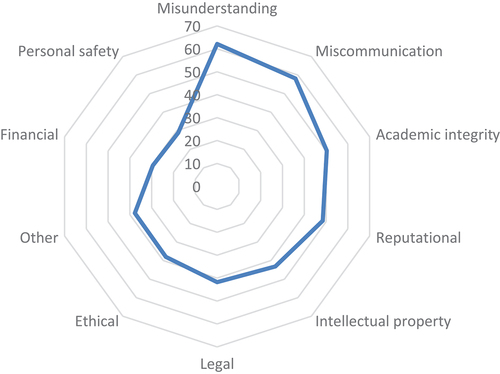
The researchers seemed quite confident about their ability to deal with MT output. In response to the question ‘How do you check that the machine translation is accurate?’, many of them trusted their own judgement by comparing it to the original text (46%) or translating it back into the original language (34%). Some said that they would ask a native speaker (25%) or use a second MT system (17%). The relatively low proportion that do the latter contrasts with studies of patent professionals, who often use more than one MT system in their work (see Nurminen Citation2021). Nevertheless, 70% of the respondents said that they (sometimes) make modifications to the translated texts after using an MT system, and 25% even deployed the strategy of pre-editing the text (i.e. making modifications to the original text before running it through an MT system).
2.3. Possible consequences of relying on MT for knowledge dissemination
Depending on their language competence in the source and target languages, researchers may be able to detect and compensate for problems associated with raw MT. For example, doing desk research with internet browsers or online bibliography services could lead to publications written in languages that the researcher is unfamiliar with. The assumption that a free online MT engine would be adequate to obtain a basic understanding of the content (i.e. gist translation) is reasonable if the researcher knows the domain well. Otherwise, the risk is great that the researcher dismisses something that might otherwise be quite useful, especially if the MT output does not make sense.
Furthermore, researchers could be tempted to translate search keywords using MT. This bears a high potential for errors since NMT systems are most effective at sentence level (i.e. each word is best translated within the context of the utterance it appears in). This is especially true for polysemic words (e.g. ‘translation’ itself has a very different meaning in biology or medicine). The MT output for isolated terms provides no indication of the associated domain, making it difficult to assess whether the resulting keywords actually fit the researcher’s needs. Our survey suggests that this might be a real issue, since 56% of the researchers indicated that they use MT to translate individual words or phrases.
Perhaps even more worrying, the MT output might be fluent but so misleading that the researcher unintentionally misrepresents other scholars’ work. Macken, Van Brussel, and Daems (Citation2019) have shown that NMT systems sometimes produce ‘invented’ words, which are particularly problematic and much harder to detect in genres that deal with new concepts and knowledge.
Using MT when working with multilingual verbal data or preparing questionnaires in several languages can also introduce potential sources of error into research. Good practice would dictate that competent bilingual post-editors and/or professional translators be involved in the process to ensure accuracy and quality (cf. Harkness et al. Citation2010), but researchers might be tempted to forego their services for reasons of speed, availability, or cost. However, translating questionnaires requires a good command of the target culture in order to preserve ecological validity. Cultural differences might also impact the level of formality and the way questions are asked in general. Current MT systems cannot take cultural specificities into consideration – hence using only MT to produce multilingual questionnaires could, in the worst case, affect the respondents’ behaviour. More generally, resorting to MT to process verbal data entails the risk of cultural elements relevant for the research question being left out or mistranslated.
Doing, presenting and publishing research are challenges, but they are compounded when any or all of them must be done in a non-dominant language. People may think that researchers should simply improve their language competence, but that would ignore the reality of countless ex-pat researchers who are expected to be able to talk and write about their work as well as teach in the local language. In addition to the temporal and financial constraints mentioned in the previous paragraph, researchers who pride themselves on being experts in their field may have trouble seeking help with such core aspects of their job. From our own practice, we know of cases in which established researchers tried to pass off MT output as their own (non-native) productions and requested ‘proofreading’ from native-speaker colleagues.
Of course, all of the above also holds for those who are involved in disseminating science to the general public (see Davier and Conway Citation2019 for an excellent overview of the role of translation in journalism). And depending on their own language repertoire, they might also be relying on MT to help them understand the research. This type of complex scenario can be applied to many other domains, which is where MT literacy becomes important not only for the members of those discourse communities but also for language professionals who might be consulted about meeting translation needs.
3. MT literacy training for different groups of users
One of the objectives of the online questionnaire described in the previous section was to heighten the awareness of university staff and students in our country about the risks and opportunities inherent in the use of the MT, and many of the responses towards the end of the questionnaire suggested this had been successful at least in some cases. In particular, the items about whether there were institutional guidelines concerning MT or training (fewer than 2% of the researchers responded in the affirmative) seemed to have heightened their awareness of that need.
Many of the researchers expressed an interest in learning more about how MT systems work (43% on a dedicated website; 29% in documentation; 20% in a training session), and only 19% said they were not really interested. A similar pattern emerged in response to the questions about learning how to use MT systems effectively (i.e. 42% on a dedicated website; 30% in documentation; 28% in a training session; 15% not really interested). The online questionnaire also prompted many people to contact our research team to inquire whether resources were already available online (one of the goals of the project) or whether specific training workshops could be organised. This made us realise that there was a need for consulting services related to MT literacy that both translators and their trainers could be qualified to provide.
In the following sections, we present some basics of MT literacy training that could be adapted to various target audiences before outlining possible training scenarios for consulting purposes. The latter include possible input for a continuing education short course for established translation professionals who are interested in learning more about MT and post-editing as well as a short module for MA translation students about to enter the language industry. Finally, we provide two concrete examples of MT literacy training for target audiences without a translation background that directly relate to our survey results.
3.1. Some basics of MT literacy training
Bowker and Buitrago Ciro (Citation2019) were the first to make explicit suggestions on what constituted MT literacy. Although their target audience was primarily the scholarly community, their definition of the components of MT literacy (2019, 88; listed below) is a very useful basis for translators offering consulting services to other users:
understand the basics of how MT systems process texts
understand how MT systems are or can be used to find, read and/or produce texts
appreciate the wider implications associated with the use of MT
evaluate how (machine) translation-friendly a text is
create or modify a text so that it could be translated more easily by a MT system
modify the output of a MT system to improve its accuracy and readability.
Since NMT has established its dominance, promoting MT literacy can be considered to be in the interest of developing societal AI literacy more generally (for a conceptualisation of the latter, see Ng et al. Citation2021). Forcada’s (Citation2017) article is a useful resource for translators to understand what NMT is and how much it differs from translation memories, which are based on previously translated and verified segments (usually, but not exclusively by trained professionals). Pérez-Ortiz, Forcada, and Sánchez-Martínez (Citation2022, 141) also explain this difference very clearly:
The first thing you should know about neural machine translation (NMT) is that it considers translation as a task involving operations on numbers performed by mathematical systems called artificial neural networks: these systems take a sentence and transform it into a series of numbers.
Most free browser-based systemsFootnote9 are fast, easy to use and based on translating isolated sentences (which is why coherence issues are common problems). The output varies depending on the availability and quality of the training data (which is why it is poorer for under-resourced languages and specialised domains). One of the current challenges for NMT developers of online engines is that the web resources they rely on for training might themselves be raw MT output. This is less of an issue for those that rely on curated training data from aligned bilingual corpora and/or existing TMs (e.g. DeepL and customised systems such as eTranslation, used by EU institutions and others).
Customised NMT systems share some of the limitations of the free systems and are relatively costly and time-consuming to develop, but they offer some important advantages. Because they are based on the client’s own bilingual corpora (e.g. TMs) and terminology, they can be domain- and even genre-specific. Most importantly for most clients, they offer the security of being stored on local servers and only accessible to authorised users. As tempting as it might be to rely on the free systems for economical reasons, they are not secure and their use seriously compromises confidentiality. Some of the providers of other systems offer professional, licenced versions at a fee to ensure data is not shared with third parties.
MT literacy includes not only the awareness of the risk associated with data security but also those associated with personal and safety liability. In a study of the use of raw MT output in emergency room instructions, Taira et al. (Citation2021, 3364) found examples that ranged from the obviously incorrect (e.g. ‘You may take anti-tank missile as much as you need for pain’ instead of ‘You can take over the counter ibuprofen as needed for pain’) to the dangerously confusing (e.g. ‘Do not take anymore soybean until your doctor reviews the result’ instead of ‘Do not take any more Coumadin until your doctor reviews the result’). This does not seem to be an isolated finding: from their meta-analysis of risks and potential associated with MT in medical and legal settings, Vieira, O’Hagan, and O’Sullivan (Citation2021, 1526) conclude levels of awareness vary and highlight the need for MT literacy and guidelines on the use of MT, pointing out that ‘duty of care could be breached’ otherwise. There have been similar calls within the legal community for heightened awareness and legally binding standards (e.g. Yanisky-Ravid and Martens Citation2019).
In addition to the risks associated with undetected errors in MT output and the security risks mentioned above, Ottmann and Carmen (Citation2020) point out that it is not clear who is liable for damages incurred through the use of MT. These can include loss of reputation, discrimination, legal consequences, injury to persons and property damage. In extreme cases, users would feel compelled to extend their liability insurance to cover such risks. The human values of reflection, logic, empathy, cultural sensitivity and ethical judgement are all missing from raw machine output, but the expectations of those still exist on the part of the receivers of translated texts.
One of the problems with the use of MT that has received quite a bit of attention in the research literature is the propagation of various types of bias (e.g. Bender et al. Citation2021). The most commonly mentioned MT bias occurs in translations between languages that do and do not mark gender on nouns (Savoldi et al. Citation2021). For example, the English sentence ‘The nurse could not lift the heavy patient, although he was actually quite strong’. illogically becomes a female nurse lifting a strong male patient in the German version.Footnote10 Such biases are not limited to gender or nouns, since stereotypes associated with ethnicity, demographic group and other socially distinguishing features can be reproduced in training sets and magnified in machine learning systems that are tuned to produce the ‘best’ solution or translation. As Birhane and Uday Prabhu (Citation2021, 1541) so aptly put it: ‘Feeding AI systems on the world’s beauty, ugliness and cruelty, but expecting it to reflect only the beauty is a fantasy’.
Awareness of risks and the danger of various types of bias is an important aspect of MT literacy, namely being able to determine how ‘MT-friendly’ a source text is. Nitzke, Hansen-Schirra, and Canfora (Citation2019, 239) developed a decision model ‘which includes factors like text type, the MT system, the required quality of the final text, turnaround time and life span of the translation’. They suggest that such decisions might be made before translators or post-editors are involved in the process (e.g. by clients or project managers). However, in light of the convergence of TMs and MT mentioned above, their proposal for a ‘post-editing competence model’ (2019, 250) that includes risk assessment competence, consulting competence, MT competence and post-editing competence in addition to core translation competences would seem equally relevant for translators and their trainers. It would also serve as an excellent model for a translation curriculum infused with a healthy dose of MT literacy awareness.Footnote11 In the following, we explain how such awareness can be leveraged into consulting competence in this area through targeted training.
3.2. MT literacy training for consulting purposes
Over the past few years, we have started incorporating input on MT literacy into professional development sessions in post-editing that we regularly offer to professional translators. Before addressing the specificities of the post-editing task and practical exercises, the translators are introduced to the concept of MT literacy as defined by Bowker and Buitrago Ciro (Citation2019) and reflect on the increasing role of MT in society and implications for their profession. With them, we explore an extended concept of MT literacy adapted to their context and the role it can occupy in translators’ competence profiles:
basic knowledge about MT systems and how they differ from human intelligence
knowing how to use MT (being aware of cognitive potentials and risks, developing strategies to foster one’s own creativity, using the tool in a way that makes translators enjoy their work and maintains motivation)
being an expert for translation among other experts (being able to talk to MT developers, having a clear self-concept)
being an expert for the use of MT for clients, colleagues and society as a whole (being able to explain the potential and risks of MT to non-translators, acting as an MT consultant)
being a change manager for digitalisation (taking the initiative to shape developments by providing input for workflows, being adaptive, promoting exchange among peers and building networks).
We focus on encouraging the practitioners to recognise that they already have the expertise to evaluate MT output in their language combinations as well as its appropriateness for the genres and domains they are familiar with. In addition, we try to empower them to continue learning about the limits and possibilities of this technology in preparation for non-translators turning to them for advice. Feedback from various post-editing training sessions suggests that introducing professionals to this extended concept of MT literacy helps them to incorporate their new role as MT experts into their self-concept and increases their agency.
The second training scenario for consulting purposes that we have designed is a 4-hour workshop with 2nd year MA translation students, designed to inform them about and sensitise them to lay uses and perceptions of MT. The workshop begins with input on the following topics:
brief overview on how MT works (as a reminder from their other courses)
post-editing from a process-oriented, cognitive and emotional point of view
machine translation today: perceptions and uses of MT among the broad public.
The major part of the workshop is then dedicated to a role play and its preparation. A fictitious situation is introduced in which the students are the head of translation services within a company that recently decided to acquire an internal MT engine or an MT licence (e.g. DeepL Pro) to save costs and time. The students are asked to prepare and hold four meetings with other characters, played by the teachers:
the company’s CEO, who has asked them to find the best MT provider for the company’s needs
the translators in their team, to announce and explain what changes will occur
an MT provider of their choice, to discuss prices and conditions
the head(s) of other departments within the company, who use free online services for various purposes and should now follow a stricter protocol.
In these role plays, it is important that students find appropriate arguments to convince the target audience they have to deal with. After our workshops, the participants fill out a brief feedback survey. The results so far indicate heightened empowerment, as many of the MA students felt more secure about their own value, and a higher degree of agency, since many wrote that they now realise that explaining the risks and opportunities of MT to others will be part of their job.
3.3. Other MT literacy training scenarios
Another training scenario we have developed and tested is a module not only for prospective translators but also for students interested in other language-related professions (journalism, communication, language teaching). It is part of a general applied linguistics course that is mandatory for all 1st-year BA students in our faculty and comprises a pre-recorded introductory video lesson available online followed a week later by streamed small-group sessions with exercises and use cases. The lecture covers the following topics:
overview of the current (and local) need for translation and multilingualism
background knowledge about language-related AI
overview of existing tools
potential and risks associated with MT
strengths of language technology vs. those of people and how to successfully combine them.
The exercises include a recent use (or misuse) case that provides a frame for discussion within the real-life context of most of the participants, who are invited to debate the impact and relevance of MT for their future professions. The exercises are intended to allow the students from different professional profiles to apply knowledge about MT to their specific contexts.
The survey discussed above was carried out one month after the first iteration of this training scenario. When extracted from the rest of the sample, the responses of the BA students in applied linguistics showed a higher awareness of MT-related risks than the other groups, including the researchers. A focus group interview carried out six months after the training session revealed that, although awareness of and knowledge about MT had been raised by this training, the students seemed to be less confident about dealing with MT output, suggesting a reduction in agency and empowerment. It would probably require more targeted practice with MT for them to gain confidence and develop MT literate behaviours. We therefore consider this type of input a basis for MT literacy that should be further developed and applied in other courses throughout a study programme.
We have also developed various training scenarios for non-students and outline one here as inspiration for what translators or trainers might want to offer themselves. In this example, a half-day professional development workshop was designed for university administration staff that could easily be adapted to other contexts. It is structured like the other scenarios, with slightly different content:
overview of current MT systems
overview of quality issues associated with MT output
clarification of legal constraints (data privacy)
potential, implications and good practices associated with MT for email
MT on social media and websites: implications for reading and writing
limitations of MT (i.e. when professional translation is needed).
After the workshop, the participants stated that they felt more inclined to use MT to produce other-language emails and knew how to avoid the major pitfalls. Some declared that they were more aware of the privacy issues linked to using free online MT tools and would change their behaviour accordingly.
There are many other forms in which MT literacy consulting services can be provided. For example, Bowker (Citation2021) outlines the strengths and weaknesses of five formats that she has tested for different target groups, ranging from optional 1-hour library workshops open to everyone at the university to an 18-hour summer institute course on MT and MT literacy. Independent of the format, Bowker stresses that if the training is not done by language professionals, then the trainers themselves would need to be trained. She and her colleagues have made resources that could be useful to MT literacy trainers and consultants freely available in various languages on a dedicated website.Footnote12
The explicit objective of the recently completed project MultiTrainNMT is to provide accessible explanations of deep learning and neural machine translation and to empower MT users throughout society.Footnote13 Several units of the resulting open access book (Kenny Citation2022) and accompanying training materials would be directly relevant to those offering MT literacy training and could be supplemented with information about the potential benefits, risks and other implications of using MT in the respective users’ specific contexts. With their sensitivity to target audiences’ needs, meta-linguistic awareness and deep cultural knowledge, MT literate translators would be ideally placed to provide such training to commercial clients as well as governmental agencies and non-profit organisations.
4. Conclusions
As indicated by our survey results, MT seems be used with relatively little reflection by otherwise highly educated professionals who might benefit from those with translation expertise. For translators, MT literacy comprises a set of skills that they master in order to perform their translation tasks and a competence that they could share with others. This includes actively shaping the implementation of technology by deciding when to use MT, developing strategies to preserve and foster creativity, and being aware of the complementarity of human and artificial intelligence. MT literacy also presupposes the capacity to engage self-confidently in constructive collaboration with MT developers and to promote appropriate use of MT by providing advice.
This extended concept of MT literacy has consequences for the professional self-concept of translators in general. It suggests that, to meet present and future requirements, translators have to feel comfortable playing a (pro)active part in shaping the way language technologies are viewed and deployed by themselves, clients, colleagues, and society as a whole. MT literacy as we understand it is a key competence in empowering translators to bring their expertise to the table when language-related technologies are developed and implemented, and to increasingly incorporate the role of language consultants into their professional self-concept.
Acknowledgments
This work was supported by Swiss universities under the P-8 programme and the DigLit team, with special thanks to Sara Cotelli Kureth, Elizabeth Steele and Romina Schaub-Torsello. We would also like to express our appreciation for the constructive feedback from two anonymous reviewers.
Disclosure statement
No potential conflict of interest was reported by the author(s).
Additional information
Funding
Notes
1. See He and Tao (Citation2022) for a discussion of the similar concept ‘translation technological thinking competence’.
2. See Slator (Citation2022).
4. The MT engine used was the free version of DeepL in March 2022; the source text is available at https://www.zhaw.ch/de/ueber-uns/aktuell/news/detailansicht-news/event-news/mit-hanf-statt-hopfen-nachhaltiges-bier-brauen/.
5. The consortium comprises researchers from the Bern University of Applied Sciences, the University of Neuchâtel, the Zurich University of Teacher Education, and the Zurich University of Applied Sciences.
7. Many thanks to Lynne Bowker, Mary Nurminen and Sharon O’Brien for their generosity in sharing their questionnaires and expertise with us.
8. No ethics approval is required for anonymous surveys in the country in which this research took place. The English version of the survey can be accessed under https://maureen.ehrensberger.org/files/MTLiteracySurveyDisseminationPurposes.pdf
9. See Nimdzi (Citation2022b) for a list of currently available MT systems.
10. The German version produced by Google Translate in March 2022 was: Die Krankenschwester konnte den schweren Patienten nicht anheben, obwohl er eigentlich ziemlich stark war. The French, Spanish and Italian versions also suggest that the (male) patient, but not the nurse, is strong.
11. In which case, it would be advisable to include pre-editing as an aspect of consulting competence, with a focus on educating clients on how to ‘to ensure that the [MT] input text is written in a very clear way with little ambiguity’ (Bowker Citation2020b, 12).
References
- Bender, E. M., T. Gebru, A. McMillan-Major, and S. Shmitchell. 2021. “On the Dangers of Stochastic Parrots: Can Language Models Be Too Big?” In Proceedings of the 2021 ACM Conference on Fairness, Accountability, and Transparency, 610–623. https://doi.org/10.1145/3442188.3445922.
- Birhane, A., and V. Uday Prabhu. 2021. “Large Image Datasets: A Pyrrhic Win for Computer Vision?” In 2021 IEEE Winter Conference on Applications of Computer Vision (WACV), 1536–1546. https://doi.org/10.1109/WACV48630.2021.00158.
- Blumczynski, P. 2017. Ubiquitous Translation. London: Routledge. https://doi.org/10.4324/9781315646480.
- Bowker, L. 2019. “Machine Translation Literacy: Academic Libraries’ Role.” Association for Information Science and Technology (AsiS&T) 56 (1): 618–619. https://doi.org/10.1002/pra2.108.
- Bowker, L. 2020a. “Chinese Speakers’ Use of Machine Translation as an Aid for Scholarly Writing in English: A Review of the Literature and a Report on a Pilot Workshop on Machine Translation Literacy.” Asia Pacific Translation and Intercultural Studies 3 (7): 1–11. https://doi.org/10.1080/23306343.2020.1805843.
- Bowker, L. 2020b. “Machine Translation Literacy Instruction for International Business Students and Business English Instructors.” Journal of Business & Finance Librarianship 25 (1–2): 1–19. https://doi.org/10.1080/08963568.2020.1794739.
- Bowker, L. 2021. “Machine Translation Use Outside the Language Industries: A Comparison of Five Delivery Formats for Machine Translation Literacy Instruction.” TRITON 2021 Translation and Interpreting Technology Online 25–36. https://doi.org/10.26615/978-954-452-071-7_004.
- Bowker, L., and J. Buitrago Ciro. 2019. Machine Translation and Global Research: Towards Improved Machine Translation Literacy in the Scholarly Community. Bingley, UK: Emerald Publishing Limited. https://doi.org/10.1108/9781787567214.
- Davier, L., and K. Conway, eds. 2019. Journalism and Translation in the Era of Convergence. Amsterdam: John Benjamins Publishing Company. https://doi.org/10.1075/btl.146.
- DePalma, D. A. 2020. “Augmented Translation Intelligence.” In Maschinelle Übersetzung für Übersetzungsprofils, edited by J. Porsiel, 28–40. Berlin: BDÜ Weiterbildungs- und Fachverlagsgesellschaft.
- EMT. 2022. European Master’s in Translation Competence Framework 2022. Brussels: European Commission.
- Forcada, M. 2017. “Making Sense of Neural Machine Translation.” Translation Spaces 6 (2): 291–309. https://doi.org/10.1075/ts.6.2.06for.
- Gaspari, F., H. Almaghout, and S. Doherty. 2015. “A Survey of Machine Translation Competences: Insights for Translation Technology Educators and Practitioners.” Perspectives: Studies in Translatology 23 (3): 333–358. https://doi.org/10.1080/0907676X.2014.979842.
- Groves, M., and K. Mundt. 2021. “A Ghostwriter in the Machine? Attitudes of Academic Staff Towards Machine Translation Use in Internationalised Higher Education.” Journal of English for Academic Purposes 50 (100957): 1–11. https://doi.org/10.1016/j.jeap.2021.100957.
- Harkness, J. A., M. Braun, B. Edwards, and P. Timothy. L. L. Johnson, P. M. Peter, B.-E. Pennell, and T. W. Smith, eds. 2010. Survey Methods in Multinational, Multiregional, and Multicultural Contexts. Hoboken, NJ: John Wiley & Sons. https://doi.org/10.1002/9780470609927.
- Hassan, H., A. Aue, C. Chen, V. Chowdhary, J. Clark, C. Federmann, X. Huang, et al. 2018. “Achieving Human Parity on Automatic Chinese to English News Translation.” ArXiv: 180305567 [Cs]. http://arxiv.org/abs/1803.05567.
- He, Y., and Y. Tao. 2022. “Unity of Knowing and Acting: An Empirical Study on a Curriculum Approach to Developing students’ Translation Technological Thinking Competence.” The Interpreter and Translator Trainer 16 (3): 348–366. https://doi.org/10.1080/1750399X.2022.2101849.
- Kenny, D., edited by 2022. Machine Translation for Everyone: Empowering Users in the Age of Artificial Intelligence. Berlin: Language Science Press.
- Macken, L., L. Van Brussel, and J. Daems. 2019. “Nmt’s Wonderland Where People Turn into Rabbits. A Study on the Comprehensibility of Newly Invented Words in NMT Output.” Computational Linguistics in the Netherlands Journal 9:67–80.
- Martindale, M. J., and M. Carpuat. 2018. “Fluency Over Accuracy: A Pilot Study in Measuring User Trust in Imperfect MT.” In Proceedings of AMTA 2018 1:13–25. http://aclweb.org/anthology/W18-1803.
- Melby, A. K., and C. Kurz. 2021. “Data: Of Course! MT: Useful or Risky. Translators: Here to Stay!” Multilingual 198. https://multilingual.com/issues/november-december-2021/data-of-course-mt-useful-or-risky-translators-here-to-stay/.
- Mellinger, C. D. 2017. “Translators and Machine Translation: Knowledge and Skills Gaps in Translator Pedagogy.” The Interpreter and Translator Trainer 11 (4): 280–293. https://doi.org/10.1080/1750399X.2017.1359760.
- Ng, D., T. K. Jac, K. L. Leung, K. Samuel, W. Chu, and M. S. Qiao. 2021. “Conceptualizing AI Literacy: An Exploratory Review.” Computers and Education: Artificial Intelligence 2 (100041): 1–11. https://doi.org/10.1016/j.caeai.2021.100041.
- Nimdzi. 2022a. The 2022 Nimdzi 100: The Ranking of Top 100 Largest Language Service Providers. https://www.nimdzi.com/nimdzi-100-top-lsp/#market-size-and-growth.
- Nimdzi. 2022b. Language Technology Atlas. https://www.nimdzi.com/language-technology-atlas/.
- Nitzke, J., S. Hansen-Schirra, and C. Canfora. 2019. “Risk Management and Post-Editing Competence.” Journal of Specialised Translation 31:239–259.
- Nurminen, M. 2019. “Decision-Making, Risk, and Gist Machine Translation in the Work of Patent Professionals.” In Proceedings of the 8thEuropean Association for Machine Translation. 32–42. https://aclanthology.org/W19-7204.pdf.
- Nurminen, M. 2021. “Investigating the Influence of Context in the Use and Reception of Raw Machine Translation.” PhD diss., Tampere University.
- O’Brien, S. 2002. “Teaching Post-Editing: A Proposal for Course Content.” In 6th EAMT Workshop Teaching Machine Translation. 99–106. https://aclanthology.org/2002.eamt-1.11.pdf.
- O’Brien, S., and M. Ehrensberger-Dow. 2020. “MT Literacy – a Cognitive View.” Translation, Cognition & Behavior 3 (2): 145–164. https://doi.org/10.1075/tcb.00038.obr.
- O’Brien, S., and M. F. Federico. 2020. “Crisis Translation: Considering Language Needs in Multilingual Disaster Settings.” Disaster Prevention & Management 29 (2): 129–143. https://doi.org/10.1108/DPM-11-2018-0373.
- O’Brien, S., S. Michel, and G. Marie-Josée. 2018. “Machine Translation and Self-Post-Editing for Academic Writing Support: Quality Explorations.” In Translation Quality Assessment, edited by J. Moorkens, S. Castilho, F. Gaspari, and S. Doherty, 237–262. Cham, CH: Springer. https://doi.org/10.1007/978-3-319-91241-7_11.
- Ottmann, A., and C. Carmen. 2020. “Risiken und Haftungsfragen bei neuronaler maschineller Übersetzung.” In Maschinelle Übersetzung für Übersetzungsprofils, edited by J. Porsiel, 171–184. Berlin: BDÜ Weiterbildungs- und Fachverlagsgesellschaft.
- Pérez-Ortiz, J. A., M. L. Forcada, and F. Sánchez-Martínez. 2022. “How Neural Machine Translation Works.” In Machine Translation for Everyone: Empowering Users in the Age of Artificial Intelligence, edited by D. Kenny, 141–164. Berlin: Language Science Press. https://doi.org/10.5281/zenodo.6760020.
- Savoldi, B., M. Gaido, L. Bentivogli, M. Negri, and M. Turchi. 2021. “Gender Bias in Machine Translation.” arXiv: 210406001 [Cs] 9:845–874. https://doi.org/10.1162/tacl_a_00401.
- Slator. 2019. Slator 2019 Language Industry Market Report. https://slator.com/slator-2019-language-industry-market-report/.
- Slator. 2022. Slator 2022 Language Industry Market Report. https://slator.com/slator-2022-language-industry-market-report/.
- Taira, B. R., V. Kreger, A. Orue, and L. C. Diamond. 2021. “A Pragmatic Assessment of Google Translate for Emergency Department Instructions.” Journal of General Internal Medicine 36 (11): 3361–3365. https://doi.org/10.1007/s11606-021-06666-z.
- Van der Meer, J. 2020. “Translation Technology – Past, Present and Future.” In The Bloomsbury Companion to Language Industry Studies, edited by E. Angelone, M. Ehrensberger-Dow, and G. Massey, 285–310. London: Bloomsbury Academic. https://doi.org/10.5040/9781350024960.0017.
- Van der Meer, J. 2021. “Translation Economics of the 2020s.” Multilingual 196. https://multilingual.com/issues/july-august-2021/translation-economics-of-the-2020s/.
- van Laar, E., J. A. M. Alexander, J. van Deursen, A. G. M. van Dijk, and J. de Haan. 2019. “The Sequential and Conditional Nature of 21st-Century Digital Skills.” International Journal of Communication 13:3462–3487.
- Vieira, L. N., M. O’Hagan, and C. O’Sullivan. 2021. “Understanding the Societal Impacts of Machine Translation: A Critical Review of the Literature on Medical and Legal Use Cases.” Information, Communication & Society 24 (11): 1515–1532. https://doi.org/10.1080/1369118X.2020.1776370.
- Vieira, L. N., C. O’Sullivan, X. Zhang, and M. O’Hagan. 2022. “Machine Translation in Society: Insights from UK Users.” Language Resources and Evaluation 57 (2): 893–914. https://doi.org/10.1007/s10579-022-09589-1.
- Vinall, K., and E. A. Hellmich. 2021. “Down the Rabbit Hole: Machine Translation, Metaphor, and Instructor Identity and Agency.” Second Language Research & Practice 2 (1): 99–118. http://hdl.handle.net/10125/69860.
- Way, A. 2020. “Machine Translation: Where are We at Today?” In The Bloomsbury Companion to Language Industry Studies, edited by E. Angelone, M. Ehrensberger-Dow, and G. Massey, 311–332. London: Bloomsbury Academic.
- Yanisky-Ravid, S., and C. Martens. 2019. “From the Myth of Babel to Google Translate: Confronting Malicious Use of Artificial Intelligence— Copyright and Algorithmic Biases in Online Translation Systems.” Seattle University Law Review 43 (99): 99–168. https://doi.org/10.2139/ssrn.3345716.