Abstract
Commercial meat paté samples, comprised of 100% pork (n = 7), 100% beef (n = 5) meat, and binary mixtures (beef and pork, w/w) (n = 18) were used. Fresh samples were analysed in a scanning spectrophotometer NIRSystems 6500 in reflectance mode (1100–2500 nm). Principal component analysis (PCA) and stepwise linear discriminant analysis (SLDA) were used to classify samples according to the animal species based on their near infrared reflectance (NIR) spectra. Full cross validation was used as validation method when classification models were developed. Both beef and pork paté samples were classified correctly (100%) while binary mixture samples only achieved 72% of correct classification using SLDA technique. The results demonstrated the usefulness of NIR spectra combined with chemometrics as an objective and rapid method to classify paté samples according to meat type. Nevertheless, NIR spectroscopic methods might provide initial screening in the food chain and enable more costly methods to be used more efficiently.
Se utilizaron muestras de patés de carne comerciales, compuestos de 100% carne de cerdo (n = 7), 100% carne de buey (n = 5) y mezclas binarias (buey y cerdo) (n = 18). Las muestras frescas se analizaron en un espectrofotómetro de escaneo NIRSystems 6500 en modo reflectante (1100–2500 nm). El análisis de componente principal (PCA) y el análisis discriminante lineal progresivo (SLDA) se usaron para clasificar muestras de según las especie animal basándose en sus espectros NIR. La validación cruzada completa se utilizó como método de validación cuando se desarrollaron métodos de clasificación. Las muestras de ambos patés, de buey y cerdo, se clasificaron correctamente (100%), mientras las muestras de mezcla binaria sólo alcanzaron un 72% de clasificación correcta usando la técnica SLDA. Los resultados mostraron la utilidad del espectro NIR combinado con quimometria como un objetivo y método rápido para clasificar muestras de paté según el tipo de carne. Sin embargo, los métodos espectroscópicos NIR podrían proveer una revisión inicial de la cadena de comida y permitir que métodos costosos fuesen usados más eficientemente.
Introduction
Near infrared reflectance (NIR) spectroscopy has been traditionally developed in animal science to evaluate the chemical composition of forages and feedstuffs (Baeten & Dardenne, Citation2002; Batten, Citation1998; Bertrand, Citation2002; McClure, Citation2004). The information available in the literature relates extensively with applications of NIR spectroscopy to determine gross chemical composition such as dry matter (DM), crude protein (CP), fat (oil) and amino acids content among other chemical components in a wide range of samples (Baeten & Dardenne, Citation2002; Batten, Citation1998; Bertrand, Citation2002; McClure, Citation2004). Compared with applications for quantitative analysis, little information is available on the suitability of NIR spectroscopy on the qualitative analysis of foods and agricultural products (Blanco & Villaroya, Citation2002).
An authentic food is one which is what it purports to be, in other words one which conforms to the description provided by the producer or processor (Cordella, Moussa, Martel, Sbirrazzuoli, & Lizzani-Cuvelier, Citation2002). Important aspects of this description may relate to the process history of a product (e.g. fresh meat opposed to frozen meat) or its geographic origin (Cozzolino, Vaz Martins, & Murray, Citation2002). Food adulteration has been practiced since Biblical times but has become more sophisticated in the recent past (Cozzolino et al., Citation2002; Downey, Citation1998; Murray, Aucott, & Pike, Citation2001). Foods or ingredients most likely to be targets for adulteration include those, which are of high-value or are subject to the vagaries of weather during their growth or harvesting (Cozzolino et al., Citation2002; Downey, Citation1998; Murray et al., Citation2001). The practice of adulteration arises for two main reasons: firstly it can be profitable, and secondly adulterants can be easily mixed and are subsequently difficult to detect. Most uses of NIR spectroscopy have involved the development of NIR calibrations for the quantitative prediction of composition; this was a rational strategy to pursue during the initial stages of its application, given the type of equipment available, the state of development of the emerging discipline of chemometrics and the overwhelming commercial interest in solving such problems (Cozzolino et al., Citation2002; Downey, Citation1994, Citation1996, Citation1998; Murray et al., Citation2001). A modern manufacturing industry is in a continuing demand for new, rapid and cheaper methods to directly measure the composition of food and food ingredients in order to assure the safety of the food produced for the consumers.
Increasing consumer concern also relates to meat speciation for religious reasons, and also BSE (Bovine Spongiform Encephalopathy) and E. coli 0157 infective risks (Cozzolino et al., Citation2002; Downey, Citation1994, Citation1996, Citation1998; Murray et al., Citation2001). This imposed great pressure on the food manufacturing industry to guarantee the safety of animal by-products. Tissue speciation of animal by-products, as well as speciation of animal derived by-products fed to all classes of domestic animals, is now the most important uncertainty which the food industry must resolve to allay consumer concern.
One of the advantages of NIR spectral technology is not only to assess chemical structures through the analysis of the molecular bonds in the near infrared spectrum but also to build an optical model characteristic of the samples which behaves like the “finger print” of the sample (Baeten & Dardenne, Citation2002; Batten, Citation1998; Bertrand, Citation2002; McClure, Citation2004). This opens up the possibility of using spectra to determine complex attributes of organic materials that are in some way related to molecular chromophores. These may be attributes such as bioavailability, provenance, organoleptic scores or classification of materials for authentication. However, it is well known that visual examination of the NIR spectra cannot discriminate between authentic and adulterated product (Cozzolino et al., Citation2002; Downey, Citation1994, Citation1996, Citation1998; Murray et al., Citation2001). In this context, the application of statistical packages and multivariate data analysis techniques like principal component analysis (PCA) or discriminant analysis (e.g. discriminant partial least squares) opens the possibility to understand the optical properties of the sample and allows a classification without the chemical information (Adams, Citation1995; Naes, Isaksson, Fearn, & Davies, Citation2002). NIR spectroscopic methods may provide initial screening in the food chain and enable more costly methods to be used more productively on suspects' specimens and will be easily implemented by the industry.
The objective of this study was to explore the usefulness of NIR spectroscopy combined with chemometrics to discriminate between paté samples according to the animal origin.
Materials and methods
Samples
Commercial meat paté samples, comprised of 100% pork (n = 7), 100% beef (n = 5) meat and binary mixtures (beef and pork, w/w) (n = 18) were used. The samples were purchased from local supermarkets and considered as authentic as defined by the supplier (both label and composition). Mix samples were generated in the laboratory as binary mixtures of beef and pig paté based on weight (w/w). All samples were homogenised during one minute with a food multiprocessor blender (Philips RI – 3142, Brazil) in order to present to the instrument a homogenised sample. The blender cup was washed first with hot water, followed by cold water and towel dried between samples.
Spectra collection
Samples were analysed in a scanning spectrophotometer NIRSystems 6500 (NIRSystems, Silver Spring, MD, USA) in reflectance mode (1100–2500 nm) using the sample transport module in a rectangular (1/4) quartz cup (NIRSystems part number 0IH – 0379, NIRSystems, MD, USA). Reflectance data were stored as log (1/R) (R = reflectance) at 2 nm intervals (700 data points). Samples were scanned once (no repeated spectral measurements were made) and were not rotated when spectra collection was completed. Computer operation and spectral data collection were manipulated using ISI version 3.1 software (InfraSoft International, Port Matilda, PA, USA).
Data analysis
Spectra were exported from the ISI software in ASCII format into The Unscrambler software (version 7.5, CAMO ASA, Norway) for chemometric analysis. PCA was performed using the NIR raw spectra or after pre-processing using the second derivative or the standard normal variates transformation (SNV) in order to reduce baseline variation and enhance the spectral features (Adams, Citation1995; Naes et al., Citation2002).
Stepwise linear discriminant analysis (SLDA) is a supervised classification technique where the number of categories and the sample that belong to each category are previously defined (Adams, Citation1995; Naes et al., Citation2002). The method produces a number of orthogonal linear discriminant functions, equal to the number of categories minus one, that allow the samples to be classified in one or another category. It is based on the normal distribution and the assumption that the covariance matrices of the two groups are identical. SLDA defines the distance of an object from the centre of a class, following two rules, the first obtains a measurement analogous to a score in the PCA method, often called the linear discriminant function; and the second determines the Mahalanobis distance to the centroid of any given group, a form of class distance. The basic theory of SLDA is to classify the dependent variable, dividing an n-dimensional descriptor space into two regions that are separated by a hyper plane defined by a linear discriminant function. In this study, the independent variables were the principal component scores generated from a PCA analysis of the NIR spectra (700 data points) and the discriminatory property defined by type of meat paté (e.g. beef or pork) or the binary mixture. Because SLDA used the pooled covariance matrix, the method is considered quite stable, even for training data sets. The use of the principal component scores instead of the whole wavelengths reduces the dimensionality of the data set and improves the stability of the model. The SLDA models were validated using full cross validation (Adams, Citation1995; Martens & Dardenne, Citation1998; Naes et al., Citation2002). The use of full cross validation was reported by other authors to be a good validation method when small data sets are used (Martens & Dardenne, Citation1998). SLDA was carried out using JMP software (version 5.01, SAS Institute Inc., Cary, NC, USA) on the PCA sample scores on components 1–3, which gave the highest level of separation in the PCA models developed.
Results and discussion
shows the mean NIR spectrum of beef, pork and binary mixtures (w/w) of meat paté samples after SNV transformation. The NIR mean spectra of the paté samples were very similar and no visual differences between them were observed. Absorption bands in the NIR region were observed around 1200 nm, at 1480 nm (O‒H stretch second overtone) associated with water, at 1700 nm (C‒H first overtones) associated with lipids, and between 1900 nm (O‒H stretch second overtone) and 2300 nm (C‒H combination tones) associated with water, amino acids and fatty acids (Miller, Citation2001; Murray, Citation1986). These wavelength bands act as reference points whose wavelength does not change across the spectra of a set of similar foods (Miller, Citation2001; Murray, Citation1986). Wavelength bands at 2058 nm and 2174 nm are related to the peptide absorption of the amide group of specific amino acids related to the different animal species. The standard deviation of the NIR spectra (not shown) showed that wavelengths associated with water (variable moisture content) and fatty acids/lipids are the most important in order to explain the variation in the set of samples according to the different animal species.
PCA was performed on the raw spectra to examine qualitative differences between the meat paté samples analysed. shows the score plot of the first two principal components (PCs) derived from the raw NIR spectra. A separation between samples related to the different species was observed. A clear separation between beef and pork meat paté samples was observed along PC1 which explains 86% of the total variance of the spectra in the set of samples analysed; the highest eigenvectors were observed around 1600 nm (C‒H first overtone), at 1900 nm (O‒H stretch first overtone) associated with water content, and between 2100 and 2300 nm (C‒H combination tones) associated with either unsaturated fatty acids or with aromatic amino acids (see ) (Miller, Citation2001; Murray, Citation1986). PC2 explains 12% of the variation and it was found to be related with the variation present in the binary mixtures. The highest eigenvectors in PC2 were found around 1450 nm and 1920 nm both related to O‒H overtones associated with water content (see ) (Miller, Citation2001; Murray, Citation1986).
shows the classification of paté samples and binary mixtures using SLDA technique. Both beef and pork meat paté samples were classified correctly (100%), while binary mixture samples only achieved 72% of correct classification using SLDA technique. It was observed that some of the samples from the binary mixture set were incorrectly classified and this might be explained by the fact that a non-uniform mixture was obtained every time, although, the two types of paté samples were combined in order to obtain a mix paté as homogeneous as possible. Additionally, the mixtures were made using the already made paté and not from the raw ingredients. This unhomogeneity produced by the artificial mix yields an NIR spectrum that holds the differences related with the process.
The ability of the NIR model to classify both the paté and binary mixtures is based on the vibrational responses of chemical bonds in the NIR region (O‒H, N‒H and C‒H). Beef and pork samples differ greatly in their chemical composition (e.g. amino acids and fatty acids profile), which respond in this region of the NIR spectrum influencing the accuracy of the classification models. This explains the good classification rates obtained between beef and pork pure samples vs. binary mixtures. The results also suggested that other characteristics (e.g. amino acids, fatty acids or water content) would explain the discrimination among samples. As suggested by other authors, NIR spectroscopy might be used as a first line of defence against accidental contamination (e.g. cross contamination), fraudulent practices or screening of the raw material (Murray et al., Citation2001). Nevertheless, NIR spectroscopic methods might provide initial screening in the food chain and enable more costly methods to be used more productively on suspect specimens and will be easily implemented.
Near infrared technology provides an alternative to other more sophisticated and time consuming analyses that would prove prohibitively costly in order to assess contamination or fraudulent adulteration in real industrial conditions.
Nowadays, the importance of food quality in human health and well being cannot be overemphasised. Consumers are concerned about the quality of the feed fed to farmed livestock whose tissues become our meat. Until now, however, too little effort has gone into surveillance of what enters the food chain. Although, consumers are less concerned with gross composition (e.g. crude protein, amino acids or moisture content), more sophisticated questions are arising about the safety of wholesomeness of food produced or derived from animal tissues or by-products: is it freedom from zoonostic infection risk and hormone and antibiotic residues? Animal welfare issues, animal provenance and honesty in production are also of consumers concern. The fact remains, however, that with only half of the live weight of an animal becoming meat, utilisation of slaughter by-products is necessary for both economic and environmental reasons. NIR spectroscopy has a role in the production plant and for surveillance at critical points in the food chain.
Conclusion
More than 88% of the meat paté samples and the binary mixtures were classified correctly into the groups assigned (e.g. beef, pork and binary mixtures). These results also show that NIR spectroscopy might be used for the identification or authentication of the product elaborated in order to guarantee the safety of the products to the consumer.
tcyt_a_512396_sup_20764266.pdf
Download PDF (158.2 KB)Acknowledgements
The work was supported by the National Institute for Agricultural Research (INIA) – Uruguay.
References
- Adams , M. J. 1995 . Chemometrics in analytical spectroscopy. Analytical spectroscopy monographs , Cambridge, , UK : The Royal Society of Chemistry .
- Baeten , V. and Dardenne , P. 2002 . Spectroscopy: Developments in instrumentation and analysis . Grasas y Aceites , 53 : 45 – 63 .
- Batten , G. 1998 . Plant analysis using near infrared reflectance spectroscopy: the potential and limitations . Australian Journal of Experimental Agriculture , 38 : 697 – 706 .
- Bertrand , D. 2002 . La spectroscopie proche infrarouge et ses applicatins dans les industries de l'alimentation animale . INRA Production Animal , 15 : 202 – 219 .
- Blanco , M. and Villaroya , I. 2002 . NIR spectroscopy: A rapid-response analytical tool . Trends in Analytical Chemistry , 21 : 240 – 250 .
- Cordella , Ch. , Moussa , I. , Martel , A-C. , Sbirrazzuoli , N. and Lizzani-Cuvelier , L. 2002 . Recent developments in food characterisation and adulteration detection: technique-oriented perspectives . Journal of Agriculture Food and Chemistry , 50 : 1751 – 1764 .
- Cozzolino , D. , Vaz Martins , D. and Murray , I. 2002 . Visible and near infrared spectroscopy of beef longissimus dorsi muscle as a means of discriminating between pasture and corn feeding regimes . Journal of Near Infrared Spectroscopy , 10 : 187 – 193 .
- Downey , G. 1994 . Qualitative analysis in the near infrared region . The Analyst , 119 : 2367 – 2375 .
- Downey , G. 1996 . Authentication of food and food ingredients by near infrared spectroscopy . Journal of Near Infrared Spectroscopy , 4 : 47 – 61 .
- Downey , G. 1998 . Food and food ingredient authentication by mid-infrared spectroscopy and chemometrics . Trends in Analytical Chemistry , 17 : 418 – 424 .
- Martens , H. A. and Dardenne , P. 1998 . Validation and verification of regression in small data sets . Chemometrics and Intelligent Laboratory Systems , 44 : 99 – 121 .
- McClure , F. W. 2004 . 204 years of near infrared technology: 1800 – 2003 . Journal of Near Infrared Spectroscopy , 11 : 487 – 498 .
- Miller , Ch.E. 2001 . “ Chemical principles of near infrared technology ” . In Near infrared technology in the agricultural and food industries , Edited by: Williams , P. C. and Norris , K. H. 19 – 39 . St Paul : American Association of Cereal Chemist .
- Murray , I. The NIR spectra of homologous series of organic compounds . NIR/NIT Conference . Edited by: Hollo , J. , Kaffka , K. J. and Gonczy , J. L. pp. 13 – 28 . Budapest : Akademiai Kiado .
- Murray , I. , Aucott , L. S. and Pike , I. H. 2001 . Use of discriminant analysis on visible and near infrared reflectance spectra to detect adulteration of fish meal with meat and bone meal . Journal of Near Infrared Spectroscopy , 9 : 297 – 311 .
- Naes , T. , Isaksson , T. , Fearn , T. and Davies , T. 2002 . A user-friendly guide to multivariate calibration and classification , Chichester : NIR Publications .
Supplementary material
Supplementary Figure 1. Near infrared mean spectrum of meat paté samples and binary mixtures.
Figura adicional 1. Espectro en el infrarrojo cercano de muestras puras y mezclas de paté de carne.
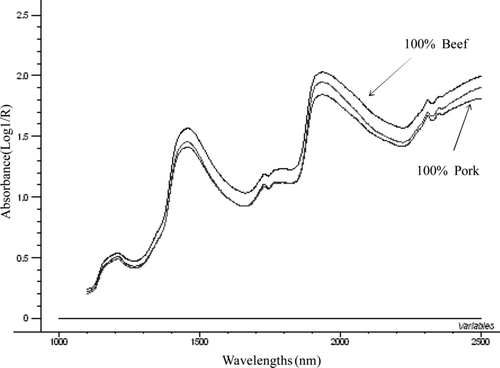
Supplementary Figure 2. Score plot of the first two principal components of paté samples and mixtures labelled according to animal species and binary mixtures.
Figura adicional 2. Scores de los dos primeros componentes principales de muestras puras y mezclas binarias de paté de carne.
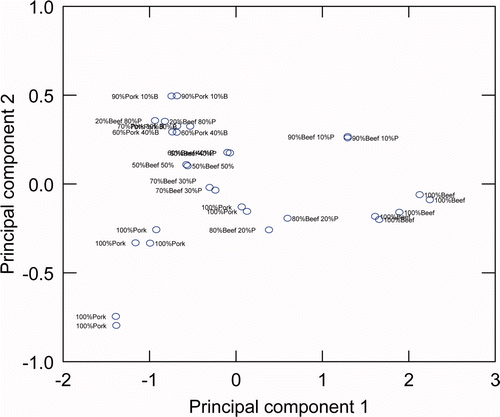
Supplementary Figure 3. Eigenvectors for the first three principal components for the classification of paté samples and binary mixtures.
Figura adicional 3. Vectores de los tres componentes principales utilizados en la clasificacion de muestras de paté de carne.
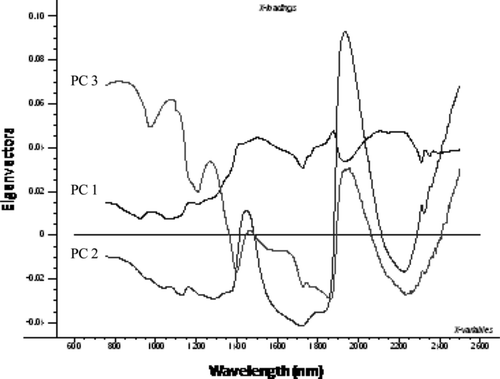