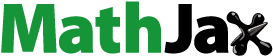
ABSTRACT
This study evaluates the performance of residential refrigerators, the worst cold chain point. Cooked ham temperature was used to quantify their performance as affected by compressor technology (single speed, SS/variable speed, VS), ambient temperature (21.1°C, LT/32.2°C, HT), load (22.5 kg, RL/39.0 kg, HL), sample type (F = fixed, M = moved to emulate consumption during meals), and door openings emulating consumer practices. Cumulative growth for each 48 h test period (LAB APIT(t)) was estimated deterministically and probabilistically, i.e. considering only means and including variability, respectively. APIT(t) at the 5°C recommended for ham yielded 0.6157 log CFU/g. The ratio APIT(t)/API5°C defined a refrigerator performance indicator (RPI) with values above and below 1 indicating poor and superior performance, respectively. While deterministic RPI values ranged from 0.99 to 1.72 (SS/LT/RL/F and VS/HT/HL/M conditions, respectively), probabilistic 95% RPI percentiles exceeded 1 suggesting poor preservation performance of the residential refrigerator tested under realistic conditions.
1. Introduction
In 2020, global pork meat production and consumption reached 97.8 and 97.1 million tonnes, respectively (COMECARNE, Citation2021; USDA, Citation2022). US regulations define cooked ham composition as a product with fat-free protein ≥ 17%, fat ≤ 3.6%, moisture ≤ 75%, dextrose ≤ 3%, salt 2.5%, carrageen ≤ 1.5%, sodium phosphate ≤ 0.5%, and sodium nitrate ≤ 0.02% (Code of Federal Regulations, Citation2011; USDA, Citation2021). Pork ham is slightly acidic (pH approx. 6), a favorable condition for microbial growth even under vacuum or modified atmosphere packaging. Moreover, lactic acid bacteria (LAB) are present during meat processing and although it does not represent a safety risk, their growth in cooked ham, favored by a microaerophilic environment and the presence of sodium chloride and sodium nitrite, is associated with the formation product surface slime (exopolysaccharides), production of off-odors, acidification (lactic and acetic acid), and myoglobin discoloration, all of which lead to product rejection by the consumer (Chávez-Martínez et al., Citation2016; Nychas et al., Citation2008; Pellissery et al., Citation2020). If ham is stored under the cooked ham recommended storage temperature (5°C), a sealed package can be stored for approximately 2 weeks, whereas opening it reduces its shelf life to 3–5 days (FDA, Citation2017; FSIS, Citation2016).
As illustrated in , more than 77% of residential refrigerators (weighted average) operate with air temperatures above the 4°C recommended by FDA for household refrigerators, reaching temperatures as high as 20°C. This situation increases food spoilage and foodborne illness risks and also the generation of food waste (Tsaloumi et al., Citation2022). These undesirably high temperatures reflect a priority given to energetic efficiency by government policies and then implemented by manufacturers and operators of cold chain units, including consumers, while ignoring the need for an effective food preservation performance to prevent food waste and preserve a high level of food quality and safety. data confirms once again that residential refrigerators are the weakest link in the food cold chain (Rodriguez-Martinez et al., Citation2019). Consequently, there is a need for a major improvement of the entire cold chain to lower the 9.3% and 23% of perishable food losses in developed and developing countries, respectively (HLPE, Citation2014; IIF-IIR, Citation2020). These losses do not include those in the consumer home for which no reliable data is available.
Table 1. Mean and maximum air temperature (T) in residential refrigerators and the percentage above the 4°C generally recommended by FDA (based on Tables 6 & 7 reported by James et al., Citation2017, and additional work cited in this table). Countries are listed based on their 2021 per capita Gross Domestic Product (GPD).
Temperature variations are unavoidable during the handling of raw materials and the manufacturing, storage, distribution (Mai et al., Citation2012), marketing (Morelli et al., Citation2012) and preservation of foods in residential refrigerators (Jofre et al., Citation2019). Thus, there is a critical need for microbial preservation performance assessment of all cold chain components based on actual temperature histories. Traditional microbial analysis methods are limited by the time it takes to receive results, whereas modern techniques are not always available for each particular application (Rodriguez-Martinez et al., Citation2020). Thus, predictive microbiology is a more promising alternative to perform product quality and safety assessments (González et al., Citation2019; Guillier, Citation2016; Reguillo-Granados et al., Citation2018; Skandamis & Panagou, Citation2016; Tenenhaus-Aziza & Ellouze, Citation2015). These mathematical models quantitatively describe the response of a given microorganism for a specific food matrix and environmental condition (Teleken et al., Citation2011). Despite advances in predictive microbiology over the past decades, the availability of mathematical model parameters for specific products in the temperature range of interest is a limiting factor. Moreover, the inherent and measurement variability of the model parameters, environment conditions, and food properties is even more limiting (Guillier, Citation2016; Rodriguez-Martinez et al., Citation2020). The lack of information on the variability of essential calculation parameters has led to the practice of using only mean or extreme values (i.e. deterministic approach). This approach does not account for the accuracy, variability, and certainty of the process, which are crucial safety and quality considerations for the decision-making of manufacturers, distributors, and retailers (Guillier, Citation2016; Poschet et al., Citation2003). Alternatively, probabilistic approaches account for the uncertainty and variability of the data and model parameters and it is the approach used in this work (Lambe, Citation2002).
The indicators assessing the performance of the refrigerated food chain used here are based on the exponential cumulative growth estimated as a function of sample temperature over an appropriate testing time (de la Cruz Quiroz et al., Citation2021). The temperature effect on the exponential growth rate is estimated using the secondary square root model (Eq. 1), whereas the exponential microbial counts increase (
) is estimated using the mean temperature Ti during each time interval (
, h) using Eq. 2. Summing the growth during each
generates a cumulative value over the 48 h refrigerator test period (
, Eq. 3) which is defined as the absolute preservation indicator, APIT(t) (de la Cruz-Quiroz et al., Citation2020). Additionally, the ratio of the APIT(t) value at the observed sample temperatures over an API value estimated at the constant temperature (Trec) recommended for the refrigerated storage of a particular refrigerated product (
) is defined as a Refrigeration Performance Indicator (RPI) (Eq. 4). By definition, RPI values smaller or larger than 1 indicate respectively, a better or worst preservation than at the constant 5°C recommended for the refrigerated storage of cooked ham. RPI values much larger than 1 would indicate a situation requiring urgent attention. In a previous study, Martínez-Martínez et al. (Citation2021) analyzed the performance of a residential refrigerator using RPI values based on listeriosis risk potential in cooked ham. Under the experimental conditions tested, deterministic RPI values reached 2.32 for the most severe condition (39 kg refrigerator load with 9 h door opening cycles, 32.2°C ambient temperature, variable speed compressor) and 1.03 for the least severe situation (22 kg refrigerator load with 9 h door opening cycles, 21.1°C ambient temperature, single speed compressor). The probabilistic analysis, i.e. when considering the parameter and experimental temperature variability, yielded 95% RPI percentiles >1 indicating poor preservation performance, i.e. for all conditions tested. In addition, this study showed strong effects of the compressor control mode, ambient temperature, and their interaction (Martínez-Martínez et al., Citation2021). The aim of the present study is to use the same time-temperature data () and analyze the performance of the same refrigerator but expressed by the risk of cooked ham spoilage by LAB. This will illustrate that the same time temperature data can be used to analyze multiple spoilage and pathogen risks. Another significant difference between this work and the one previously published is the implementation of two advances that account for the interdependence of the square model parameters, and of an outlier detection method recommended when executing the probabilistic procedures to estimate RPI values.
Figure 1. Time-temperature history for cooked ham stored in a household refrigerator (Adapted from Martínez-Martínez et al., Citation2021). a) VS/F, b) VS/M, c) SS/F, and d) SS/M. VS and SS describe variable and single speed compressor while F and M respectively describe ham samples kept in the refrigerator, and those that were removed during a meal to simulate consumer product use.
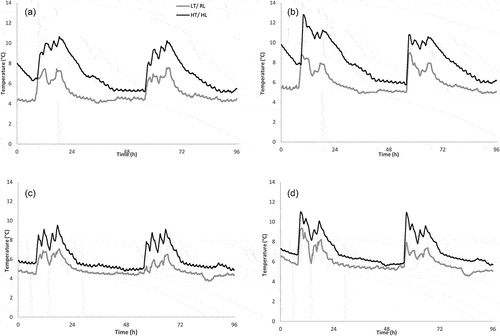
2. Materials and methods
2.1. Materials, experimental setup, and data acquisition protocol
Experimental factors affecting cooked ham temperature included ambient temperature, refrigerator compressor mode, relative humidity, fresh food compartment load, and product staying in the refrigerator or being removed during simulated mealtimes. The materials, equipment, and experimental data acquisition protocol for this work were the same as described in sections 2.1, 2.2 and 2.3, respectively, of previously published work (Martínez-Martínez et al., Citation2021). Six sliced cooked ham samples (300 g, San Rafael, Sigma Alimentos S.A. de C.V., Atitalaquia, Hidalgo, México) in flexible plastic containers (15 × 18 × 2 cm) with resealable lidding film were purchased locally along with other food products (apples, liquid white eggs, orange juice, pasteurized milk, strawberries, lettuce, ground beef, chicken breast, fish fillets, and fresh cheese) and stored inside a 760 L (26.8 ft3) customized residential refrigerator placed inside a climatic chamber set to operate at LT/HT = 21.1/32.2 ± 0.5°C and 70 ± 1% relative humidity. The unit had a 539 L (19 ft3) fresh food compartment with French-style doors and was set to operate at 5°C by means of a reciprocating R134a compressor equipped with an electronic inverter and permanent magnet motor (variable speed, 1400 to 4000 rpm, VS) or a two-poles, 60 Hz asynchronous motor (single speed, 3600 RPM, SS). The experimental data acquisition included the following procedures: food loading and temperature stabilization on day 1, door-opening cycles beginning at 8 AM and lasting 9.5 h consisted of four non-consecutive 1 h periods on day 2 and 4, and on day 3 and 5, doors remained closed in order to achieve a temperature stabilization inside the unit. Consequently, days 2 and 3 and days 4 and 5 were considered 48 h time-temperature data replicates. During the first door opening, three samples were kept in the refrigerator (F), while the remaining three were removed and returned to the refrigerator at the beginning and end of the door opening, respectively, to simulate the consumption of cooked ham by consumers during breakfast (M).
2.2. Estimation of the parameter values to calculate RPI values
Two calculation improvements were implemented in this study. First, the exponential growth phase was identified from microbial growth data published by Kreyenschmidt et al. (Citation2010) using a plot digitizer (WebPlotDigitizer Ankit Rohatgi, Pacifica, CA). This was followed by the generation for each temperature level of 1050 growth curves using the reported variability of experimental microbial counts for each measurement time. These growth curves were used to estimate 1050 values for each temperature level reported. Next, 1050 pseudo-random pairs of b and
values were generated by using the square root model (EquationEquation 3
(3)
(3) ). The second advancement was an outlier detection procedure applied to these 1050 pseudo-random pair values to minimize the under- and overestimation risk associated with their generation and following Hoaglin et al. (Citation1986) methodology. Lower (LL) and upper (LU) limits of acceptable data were identified using a constant value (k = 1.5) corresponding to a resistant rule, and two quantiles with values approximating the first and third quartiles and defined as lower and upper fourths (FL and FU), respectively. The removal of pseudo-random values that exceeded the limits changed the values of the fourths and thus of the limits. Since new outliers emerged, an iterative process was required which ended when all data pairs were within the limits. Finally, the probabilistic analysis used 1000 pairs chosen randomly from the ones left after outlier elimination.
2.3. Deterministic and probabilistic approaches for RPI values
In the deterministic approach, the experimental temperature for each F and M cooked ham sample (n = 3) and the mean values for the model parameters and
were used to estimate average RPI values. For probabilistic estimations, each of the 1000 pseudo-random
and
pair values generated as previously described were used for all time intervals in a 48 h replicate. In the case of cooked ham temperature, random temperature values for each measurement time were generated using its mean and standard deviation at each sampling time. The RPI values obtained (n = 1000) were reported as 5–95% percentile ranges.
2.3.1. Statistical analysis
Tukey tests (p = .05) were applied to compare deterministic mean RPI values using a two-level full factorial design, including compressor mode (VS, SS), ambient temperature (LT, HT), refrigerator load (RL, HL), and sample type (F, M) as the studied factors, by means of MINITAB statistical software where non-significant model terms were removed using the software integrated stepwise algorithm (αto enter/remove = 0.05).
3. Results and discussion
RPI values based on deterministic and probabilistic estimations were used to evaluate the performance of the refrigerator for the preservation of cooked ham stored in its bottom shelf. For all tested conditions, the RPI values for each 48 h period, shown no significant differences (data not shown), confirming that the work here reported is experimentally reproducible.
3.1 Estimated parameters for RPI calculations
The generation of the b and Tmin parameter pairs including the outlier detection method previously described was carried out using the experimental data for growth of LAB in cooked ham reported by Kreyenschmidt et al. (Citation2010) which included microbial growth curves at 2°C, 7°C, 10°C, and 15°C. The final LL = 0.0085 and LU = 0.0125 lower and upper limit values, respectively, generated b = 0.0105 ± 0.0007, while LL = −8.0954 and LU = −3.4168 generated Tmin = 5.7803 ± 0.8068°C. The mean values of these parameter values were transformed by rearranging Eq. 1 yielding Eq. 5 to allow a direct comparison with those reported by Kreyenschmidt et al. (Citation2010). The small deviation with respect to reported values (under 10%) reflects the procedures used to determine the statistical variability of b and Tmin values which were not reported by these authors (generation of pseudorandom values, microbial counts restricted to the exponential growth phase, and elimination of outliers).
this work
reported values
The benefits of the modified procedure to estimate the predictive model parameter values were assessed using the experimental data for the growth of Listeria monocytogenes on cooked ham reported by Szczawinski et al. (Citation2017) and used in previous work (Martínez-Martínez et al., Citation2021) without the modified procedure. The large increase in the steepness of the probabilistic curves for RPI obtained with the new procedure () indicates that the RPI will be a more powerful tool when assessing cold chains differing in cooling technology and operating protocols. The steepness difference is directly and exclusively related to a decrease in the variability since the 50% percentile RPI values were not affected. This observation confirms the effectiveness of retaining the interdependence of the generated b and Tmin pairs and of the statistical procedure used to eliminate outliers when conducting a probabilistic assessment of cold chain performance.
Figure 2. Probabilistic RPI curves at the VS/LT/RL condition previously published by Martínez-Martínez et al. (Citation2021) and values obtained using the enhanced procedure.
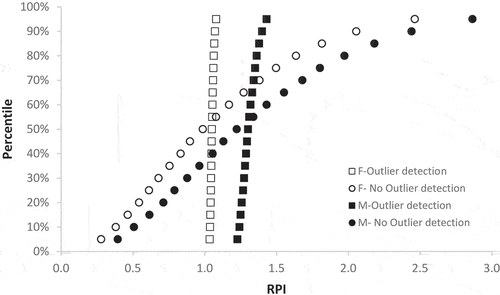
3.2. Refrigerator performance estimations using RPI values
The recommended temperature to maximize the shelf life of cooked ham is 5°C (FDA, Citation2017). Inserting this temperature and the parameter values for LAB in EquationEquation (3)(3)
(3) yielded APILAB, 5°C, 48 h = 0.6157 log CFU/g. The ratio of the API value for the highly variable observed temperatures, APILAB, T(t), over this normalization value defined the RPI which assesses the performance of refrigerated foods handling (EquationEquation 4
(4)
(4) ). RPI values smaller or larger than 1 indicate a better or worst preservation performance than at the constant 5°C recommended by FDA for cooked ham.
3.2.1. Deterministic estimations
Under VS/HT/M, the highest RPI values were 1.55 and 1.72 for RL and HL, respectively, while for SS/HT/M, the corresponding RPI values were 1.34 and 1.46 (). The statistical analysis showed the influence of the compressor mode through its interaction with ambient temperature. Under this criterion, it is shown that at LT conditions both compressors have a similar performance, while at HT the refrigerator equipped with SS compressor outperformed the refrigerator equipped with VS compressor by 14%. At first glance, this result may sound incongruent, but one should consider that the refrigerator control logic has a larger effect on refrigerator performance than the compressor itself. Besides illustrating the need for a different control logic in the compressor to speed up the temperature recovery of the refrigerator and of its content of products, the statistical analysis also introduces the importance of the ambient temperature, heat sources nearby, and the unit position with respect to walls surrounding it.
3.2.2. Probabilistic estimations
Independently of the experimental condition tested, all 95% percentile RPI values were close or above 1.0 and confirmed the strong influence of the compressor mode (). The 1.83 and 1.53 RPI 95% percentiles for VS and SS, respectively, values corresponding to HL/HT/M samples were the highest, while the lowest values, 1.04 to 1.0, observed under RL/LT/F regardless of the operation mode of the tested compressors. While these latter values are close to the desirable 1.0 value, they represent the less realistic case of cooked ham never removed from the unit. Under more realistic situations, the values exceeded 1.0 confirming the need reported in previous work using other food products and other microbial models to modify the speed logic control of VS and SS compressors to improve the food preservation capacity of the refrigeration unit (de la Cruz Quiroz et al., Citation2021; de la Cruz-Quiroz et al., Citation2020; Garcia Cortes et al., Citation2022; Martínez-Martínez et al., Citation2021; Rodriguez-Martinez et al., Citation2020). The cost and environmental impact of food spoilage, in addition to food insecurity and safety concerns, should be considered when evaluating the energy consumption of the compressor operating conditions.
4. Conclusions
Cooked ham should be stored under refrigeration to retain its freshness, reduce safety risks, and extend its shelf-life. Although the refrigerator setting should be 4°C or lower, and thus below the 5°C recommended for the storage of cooked ham, these constant recommended temperatures are rarely observed because compressor technology, ambient temperature, and consumer habits affect the product temperature history. The RPI proposed by our research group was a useful tool to identify the necessary changes in refrigerator operating conditions to protect cooked ham quality and safety, the most important being the interaction of ambient temperature and compressor technology. The traditional SS mode compressor under the more demanding conditions (HL and HT) showed better cooked ham temperature control than the VS mode compressor. However, the latter has two advantages that should be considered. First, its energy efficiency is higher and thus its heat removal capacity should be higher than SS compressors. In addition, VS compressors offer multiple operation adjustment possibilities and thus operational control adjustments could be found to allow them to deliver better food preservation than SS compressors and with better energy use performance. Finally, to improve the consumer refrigerator use habits, complementary technologies will be needed, such as interactive electronic interfaces advising consumers to adjust temperature settings, reduce product exposure to ambient temperature, and minimize door opening time and frequency. Although the development of the RPI indicators may require further research, this study and the work published so far have yielded important results. The best example is the surprising finding that advanced variable speed compressors are outperformed by single speed models just because manufacturers lack indicators such as RPI to balance energy use and preservation performance when designing them.
Nomenclature
HL | = | High load (39.0 kg) |
HT | = | High temperature (32.2°C) |
RL | = | Regular load (22.5 kg) |
LT | = | Low temperature (21.1°C) |
Tmin (°C) | = | Square root model parameter, theoretical minimum growth temperature |
b | = | Square root model parameter (its value depends on whether natural or decimal logarithms are used to express microbial counts) |
Ti (°C) | = | Mean cooked ham temperature during each short time interval ( |
Trec | = | Recommended product storage temperature (°C) |
Tred | = | Cooked ham maximum time-temperature reduction (°C) with minimum probability of product freezing risk |
CFU/g | = | Colony-forming unit per gram of cooked ham |
Δlog N48 h (log CFU/g) | = | Cumulative exponential increase in counts for each 48 h replicate defined as API |
μmax(Ti, log CFU/g h) | = | Exponential growth rate at the average temperature Ti during each short time interval ( |
API (log CFU/g) | = | Absolute preservation indicator defined as the exponential cumulative counts increase during the preservation performance test period ( |
RPI (dimensionless) | = | Refrigerator performance indicator (RPI) with values <1, ~1 and >1 predicting superior, similar, and worse performance, respectively, to the one possible at the refrigeration temperature recommended for cooked ham (5°C), whereas values >>1 would indicate a high spoilage risk |
Authors’ contributions
Author JAT participated in project conceptualization, author RdlCQ participated in data acquisition, authors EMM, DGdlG, AG, GFV, and JAT developed and executed the data analysis, authors EMM, RdlCQ and JAT prepared the original draft while all authors participated in manuscript and data analysis revisions.
Availability of data and material
Experimental data available from the authors upon request.
Disclosure statement
No potential conflict of interest was reported by the authors.
Additional information
Funding
References
- Bahk, G. -J. (2010). Statistical probability analysis of storage temperatures of domestic refrigerator as a risk factor of foodborne illness outbreak. Korean Journal of Food Science and Technology, 42(3), 373–376.
- Breen, A., Brock, S., Crawford, K., Docherty, M., Drummond, G., Gill, L., Lawton, S., Mankarious, V., Oustayiannis, A., Rushworth, G., & Kerr, K. G. (2006). The refrigerator safari. British Food Journal, 108(6), 487–494. https://doi.org/10.1108/00070700610668450
- Brennan, M., Kuznesof, S., Kendal, H., Ladha, C., & Oliver, P. (2013). Food standards agency activity recognition and temperature monitoring (ART) feasibility study - Final report. Food Standards Agency
- Byrd-Bredbenner, C., Maurer, J., Wheatley, V., Cottone, E., & Clancy, M. (2007). Food safety hazards lurk in the kitchens of young adults. Journal of Food Protection, 70(4), 991–996. https://doi.org/10.4315/0362-028X-70.4.991
- Carpentier, B., Lagendijk, E., Chassaing, D., Rosset, P., Morelli, E., & Noël, V. (2012). Factors impacting microbial load of food refrigeration equipment. Food Control, 25(1), 254–259. https://doi.org/10.1016/j.foodcont.2011.10.051
- Carrasco, E., Pérez-Rodríguez, F., Valero, A., García-Gimeno, R. M., & Zurera, G. (2007). Survey of temperature and consumption patterns of fresh-cut leafy green salads: Risk factors for listeriosis. Journal of Food Protection, 70(10), 2407–2412. https://doi.org/10.4315/0362-028X-70.10.2407
- Chávez-Martínez, A., Estrada-Gandarilla, M., Rentería-Monterrubio, A. L., & Gallegos-Acevedo, M. A. (2016). Prevalence of lactic acid bacteria in sliced cooked ham as an indicator of its shelf life. Revista Vitae, 23(1), 167–172. https://doi.org/10.17533/udea.vitae.v23n3a02
- Code of Federal Regulations. (2011). 9 CFR 424.21 (c). U.S. Office of the Federal Register.
- COMECARNE. (2021). Compendio estadístico 2021. https://comecarne.org/compendio-estadistico-2021
- de la Cruz Quiroz, R., Fagotti, F., Welti Chanes, J., & Torres, J. A. (2021). Food preservation performance of residential refrigerators: Pasteurized milk and ground beef as animal food models. Food Engineering Reviews, 13(1), 104–114. https://doi.org/10.1007/s12393-020-09230-3
- de la Cruz-Quiroz, R., Rodriguez-Martinez, V., Velazquez, G., Perez, G. M., Fagotti, F., Welti-Chanes, J., & Torres, J. A. (2020). Residential refrigerator performance based on microbial indicators of ground beef preservation assessed using predictive microbiology tools. Food and Bioprocess Technology, 13(12), 2172–2185. https://doi.org/10.1007/s11947-020-02551-5
- Derens, E., Laguerre, O., & Palagos, B. (2001). Analyse de facteurs influençant la température dans les réfrigérateurs des ménages. Bulletin de l’Académie Nationale de Médecine, 185(2), 311–322. https://doi.org/10.1016/S0001-4079(19)34558-3
- Đurić, J., Ivanović, J., Lončina, J., Šarčević, D., Đorđević, V., Bošković, M., & Baltić, M. Ž. (2013). Examination about consumers knowledge of food storage conditions in household–context of food safety. Proceedings/International 57th Meat Industry Conference: Meat and meat products-perspectives of sustainable production, Belgrade, Serbia. Institute of Meat Hygiene and Technology.
- Evans, E. W., & Redmond, E. C. (2015). Analysis of older adults’ domestic kitchen storage practices in the United Kingdom: Identification of risk factors associated with Listeriosis. Journal of Food Protection, 78(4), 738–745. https://doi.org/10.4315/0362-028x.Jfp-14-527
- FDA. (2017). Food code. Food and Drug Administration. https://www.fda.gov/food/fda-food-code/food-code-2017
- FSIS. (2016). Ham and food safety. Retrieved July 25, from https://www.fsis.usda.gov/wps/portal/fsis/topics/food-safety-education/get-answers/food-safety-fact-sheets/meat-preparation/ham-and-food-safety/ct_index
- Galvão, D., Gaspar, P. D., da Silva, P. D., & Carvalho Pires, L. C. (2016). Experimental study of the operative conditions of domestic refrigerators in the student community of the University of Beira interior. In VIII Iberian Congress | VI Ibero-American Congress of Refrigeration Sciences and Technologies. Coimbra, Portugal. International Institute of Refrigeration.
- Garcia Cortes, A., Martinez-Martinez, E., Fernandez Villanueva, G., Torres-Valdez, A. Y., Fagotti, F., Garcia Garcia, R. R., de la Cruz Quiroz, R., & Torres, J. A. (2022). Absolute preservation indicator for the assessment of refrigeration performance based on dynamic temperature measurements and predictive microbiology. Food Control, 136(108891), 1–9. https://doi.org/10.1016/j.foodcont.2022.108891
- Garrido, V., García-Jalón, I., & Vitas, A. I. (2010). Temperature distribution in Spanish domestic refrigerators and its effect on Listeria monocytogenes growth in sliced ready-to-eat ham. Food Control, 21(6), 896–901. https://doi.org/10.1016/j.foodcont.2009.12.007
- George, R. M., Burgess, P. J., & Thorn, R. D. (2010). Reducing food waste through the chill chain.
- Geppert, J., & Stamminger, R. (2010). Cold storage in private households: Recommendations and consumer real life behaviour. In 4th International Workshop Cold Chain Management. Bonn, Germany: Institut für Landtechnik.
- Gilbert, S. E., Whyte, R., Bayne, G., Lake, R. J., & van der Logt, P. (2007). Survey of internal temperatures of New Zealand domestic refrigerators. British Food Journal, 109(4), 323–329. https://doi.org/10.1108/00070700710736570
- González, S. C., Possas, A., Carrasco, E., Valero, A., Bolívar, A., Posada-Izquierdo, G. D., García-Gimeno, R. M., Zurera, G., & Pérez-Rodríguez, F. (2019). ‘MicroHibro’: A software tool for predictive microbiology and microbial risk assessment in foods. International Journal of Food Microbiology, 290, 226–236. https://doi.org/10.1016/j.ijfoodmicro.2018.10.007
- Guillier, L. (2016). Predictive microbiology models and operational readiness. Procedia Food Science, 7, 133–136. https://doi.org/10.1016/j.profoo.2016.05.003
- Hassan, H. F., Dimassi, H., & El Amin, R. (2015). Survey and analysis of internal temperatures of Lebanese domestic refrigerators. International Journal of Refrigeration, 50, 165–171. https://doi.org/10.1016/j.ijrefrig.2014.10.026
- HLPE. (2014). Food losses and waste in the context of sustainable food systems. https://www.fao.org/publications/card/en/c/f45cf2c1-aff7-4304-a11d-4fbf0e594ddb/
- Hoaglin, D. C., Iglewicz, B., & Tukey, J. W. (1986). Performance of some resistant rules for outlier labeling. Journal of the American Statistical Association, 81(396), 991–999. https://doi.org/10.1080/01621459.1986.10478363
- IIF-IIR. (2020). The Role of Refrigeration in Worldwide nutrition (2020), 6th Informatory Note on Refrigeration and Food. https://iifiir.org/en/fridoc/the-role-of-refrigeration-in-worldwide-nutrition-2020-142029
- James, C., Onarinde, B. A., & James, S. J. (2017). The use and performance of household refrigerators: A review. Comprehensive Reviews in Food Science and Food Safety, 16(1), 160–179. https://doi.org/10.1111/1541-4337.12242
- Janjić, J., Katić, V., Ivanović, J., Bošković, M., Starčević, M., Glamočlija, N., & Baltić, M. Ž. (2016). Temperatures, cleanliness and food storage practises in domestic refrigerators in Serbia, Belgrade. International Journal of Consumer Studies, 40(3), 276–282. https://doi.org/10.1111/ijcs.12252
- Jofre, A., Latorre-Moratalla, M. L., Garriga, M., & Bover-Cid, S. (2019). Domestic refrigerator temperatures in Spain: Assessment of its impact on the safety and shelf-life of cooked meat products. Food Research International, 126(108578), 1–8. https://doi.org/10.1016/j.foodres.2019.108578
- Kennedy, J., Jackson, V., Blair, I. S., McDowell, D. A., Cowan, C., & Bolton, D. J. (2005). Food safety knowledge of consumers and the microbiological and temperature status of their refrigerators. Journal of Food Protection, 68(7), 1421–1430. https://doi.org/10.4315/0362-028x-68.7.1421
- Kreyenschmidt, J., Hubner, A., Beierle, E., Chonsch, L., Scherer, A., & Petersen, B. (2010). Determination of the shelf life of sliced cooked ham based on the growth of lactic acid bacteria in different steps of the chain. Journal of Applied Microbiology, 108(2), 510–520. https://doi.org/10.1111/j.1365-2672.2009.04451.x
- Laguerre, O., Derens, E., & Palagos, B. (2002). Study of domestic refrigerator temperature and analysis of factors affecting temperature: A French survey. International Journal of Refrigeration, 25(5), 653–659. https://doi.org/10.1016/S0140-7007(01)00047-0
- Lambe, J. (2002). The use of food consumption data in assessments of exposure to food chemicals including the application of probabilistic modelling. The Proceedings of the Nutrition Society, 61(1), 11–18. https://doi.org/10.1079/PNS2001125
- Mai, N. T. T., Margeirsson, B., Margeirsson, S., Bogason, S. G., Sigurgisladottir, S., & Arason, S. (2012). Temperature mapping of fresh fish supply chains – air and sea transport. Journal of Food Process Engineering, 35(4), 622–656. https://doi.org/10.1111/j.1745-4530.2010.00611.x
- Martínez-Martínez, E., de la Cruz Quiroz, R., Fagotti, F., & Torres, J. A. (2021). Methodology for the food preservation assessment of residential refrigerators: Compressor and consumer practices effects on absolute and relative preservation indicators. International Journal of Refrigeration, 127, 260–271. https://doi.org/10.1016/j.ijrefrig.2021.03.006
- Morelli, E., Noel, V., Rosset, P., & Poumeyrol, G. (2012). Performance and conditions of use of refrigerated display cabinets among producer/vendors of foodstuffs. Food Control, 26(2), 363–368. https://doi.org/10.1016/j.foodcont.2012.02.002
- NSW Food Authority. (2009). Domestic fridge survey. NSW Food Authority.
- Nychas, G. J., Skandamis, P. N., Tassou, C. C., & Koutsoumanis, K. P. (2008). Meat spoilage during distribution. Meat Science, 78(1–2), 77–89. https://doi.org/10.1016/j.meatsci.2007.06.020
- Pellissery, A. J., Vinayamohan, P. G., Amalaradjou, M. A. R., & Venkitanarayanan, K. (2020). Spoilage bacteria and meat quality. In A. K. Biswas & P. K. Mandal (Eds.), Meat quality analysis: Advanced evaluation methods, techniques, and technologies (pp. 307–334). Academic Press. https://doi.org/10.1016/b978-0-12-819233-7.00017-3
- Poschet, F., Geeraerd, A. H., Scheerlinck, N., Nicolaï, B. M., & van Impe, J. F. (2003). Monte Carlo analysis as a tool to incorporate variation on experimental data in predictive microbiology. Food microbiology, 20(3), 285–295. https://doi.org/10.1016/S0740-0020(02)00156-9
- Rahman, M. S. (2006). State diagram of foods: Its potential use in food processing and product stability. Trends in Food Science & Technology, 17(3), 129–141. https://doi.org/10.1016/j.tifs.2005.09.009
- Reguillo-Granados, L., Pérez, F., & Valero, A. (2018). Food quality management systems in the dairy industry: A case study on the application of predictive microbiology in the microbial quality of milk. In N. Koca (Ed.), Technological approaches for novel applications in dairy processing. Intech Open. https://doi.org/10.5772/intechopen.73309
- Roccato, A. (2013). Survey conducted in Italy on the consumer refrigeration temperatures and their impact on food safety illustrated with Salmonella. In IAFP European Symposium on Food Safety. Marseille, France: International Association for Food Protection.
- Rodriguez-Martinez, V., Velazquez, G., Massa-Barrera, S., Welti Chanes, J., Fagotti, F., & Torres, J. A. (2019). Estimation of safety and quality losses of foods stored in residential refrigerators. Food Engineering Reviews, 11(3), 184–199. https://doi.org/10.1007/s12393-019-09192-1
- Rodriguez-Martinez, V., Velazquez, G., Rodriguez Altaif, R. D. J., Fagotti, F., Welti-Chanes, J., & Torres, J. A. (2020). Deterministic and probabilistic predictive microbiology-based indicator of the listeriosis and microbial spoilage risk of pasteurized milk stored in residential refrigerators. LWT - Food Science and Technology, 117(108650), 1–12. https://doi.org/10.1016/j.lwt.2019.108650
- Skandamis, P. N., & Panagou, E. Z. (2016). Predictive microbiology: Mathematics towards understanding the fate of food-borne microorganisms in food processing. In A. de Souza Sant’Ana (Ed.), Quantitative microbiology in food processing (pp. 16–67). Wiley Publishing Inc. https://doi.org/10.1002/9781118823071.ch2
- Staskel, D. M., Sweitzer, S. J., Briley, M. E., Roberts-Gray, C., & Almansour, F. D. (2009). Food safety temperatures and storage methods of preschool children’s packed meals. Journal of the American Dietetic Association, 109(9), A72. https://doi.org/10.1016/j.jada.2009.06.239
- Szczawinski, J., Ewa Szczawinska, M., Lobacz, A., Tracz, M., & Jackowska-Tracz, A. (2017). Modelling the growth rate of Listeria monocytogenes in cooked ham stored at different temperatures. Journal of Veterinary Research, 61(1), 45–51. https://doi.org/10.1515/jvetres-2017-0006
- Taoukis, P. S., Giannakourou, M. C., Koutsoumanis, K., & Bakalis, S. (2005). Modelling the effect of house hold chilled storage conditions on the risk distribution of meat products. In III International Symposium on Applications of Modelling as an Innovative Technology in the Agri-Food Chain. Leuven, Belgium: International Society for Horticultural Science.
- Teleken, J. T., Robazza, W. D. S., & Gomes, G. D. A. (2011). Mathematical modeling of microbial growth in milk. Food Science and Technology, 31(4), 891–896. https://doi.org/10.1590/S0101-20612011000400010
- Tenenhaus-Aziza, F., & Ellouze, M. (2015). Software for predictive microbiology and risk assessment: A description and comparison of tools presented at the ICPMF8 Software Fair. Food Microbiology, 45(Pt B), 290–299. https://doi.org/10.1016/j.fm.2014.06.026
- Terpstra, M. J., Steenbekkers, L. P. A., de Maertelaere, N. C. M., & Nijhuis, S. (2005). Food storage and disposal: Consumer practices and knowledge. British Food Journal, 107(7), 526–533. https://doi.org/10.1108/00070700510606918
- Tsaloumi, S., Aspridou, Z., Spyrelli, E., Nychas, G. -J.E., & Koutsoumanis, K. (2022). Development and validation of a mathematical model for pseudomonads growth as a basis for predicting spoilage of fresh poultry breast and thigh fillets. Poultry Science, 101(8), 101985. https://doi.org/10.1016/j.psj.2022.101985
- USDA. (2021). Supplement 303 to master solicitation purchase of ham, boneless, fully cooked water-added for distribution to federal food nutrition assistance program. USDA Agricultural Marketing Service. https://www.ams.usda.gov/sites/default/files/media/Supplement303HamProducts70821.pdf
- USDA. (2022). Livestock and Poultry: World Markets and Trade. https://www.fas.usda.gov/data/livestock-and-poultry-world-markets-and-trade
- Vegara, A., Rita Festino, A., DiCiccio, P., Costanzo, C., Pennisi, L., & Ianieri, A. (2014). The management of the domestic refrigeration: Microbiological status and temperature. British Food Journal, 116(6), 1047–1057. https://doi.org/10.1108/BFJ-05-2012-0103