Abstract
Digitization and the internet have been changing how companies and customers interact, forcing organizations to adapt more efficient services that generate value in the purchasing and communication processes. This research aims to identify key factors that influence satisfaction with the use of chatbots among young people from Colombia, based on 126 surveys administered via Google Forms. The methodological design adopted a quantitative approach, with an exploratory-descriptive scope that involved a structural equation model PLS-SEM of the survey data. The information analysis tested the hypotheses concerning the constructs of the conceptual model and chatbot user satisfaction by means of p value and path coefficients. The results suggest that there is an effect of robotic knowledge of use and resolution of pending cases on the perception of having access to up-to-date information during the chatbot experience. The main expectations regarding chatbot use were also identified, such as resolving the issue, being attended to quickly and the effectiveness of the service. This study also has practical implications for chatbot designers and developers in the governments of all countries, especially in developing countries like Colombia.
1. Introduction
The digital age and the internet have been changing the way in which companies and customers interact, in addition to introducing new market requirements, which force companies to adapt their strategies to include more efficient value-added services (Naimi-Sadigh et al., Citation2022). In response to these new demands, according to (Misischia et al., Citation2022), the application of artificial intelligence (AI) has emerged, which is the ability to make machines intelligent, for making decisions characteristic of human interactions (Singh & Thakur, Citation2020). AI uses machine learning, language processing and robotics techniques, which are commonly known as chatbots or virtual assistants (Pelau et al., Citation2021). Its application has been facilitated due to the rapid development of information and communication technologies (ICTs) (Smutny & Schreiberova, Citation2020).
Thus, conversational agents or chatbots represent an efficient form of communication by simulating a conversation between a system and a user, as they perform certain tasks faster and more accurately compared to humans and have the option of improving customer service and therefore customer satisfaction with the service provided (Chen et al., Citation2021; Pelau et al., Citation2021). Similarly, among the benefits of using these virtual assistants is quick and easy access to required information, which saves time (Okonkwo & Ade Ibijola, Citation2021), allowing the login of multiple users simultaneously (Wu et al., Citation2020), which can be adapted to users’ tasks and emotions (Graesser, Citation2016) and can offer faster and more personalized services (Dijmărescu et al., Citation2022; Okonkwo & Ade Ibijola, Citation2021), among other advantages.
At an international level, chatbots are being widely used by business clients in many sectors. Today, they can be found in various areas, such as marketing, customer service, technical support, psychology, education and training (Topal et al., Citation2021). Likewise, these technological tools are becoming an increasingly popular option for interacting with users, attracting new customers and improve the payment of products and services (Andronie et al., Citation2021; Smutny & Schreiberova, Citation2020). However, according to (Følstad et al., Citation2018), the literature currently lacks knowledge on the factors related to the acceptance of virtual assistive technology by users and to measure the level of satisfaction provided by these services.
For Colombia, the figures of the Ministry of Information Technology and Communications (MinTIC) report 43.2% coverage of the total internet demand during the year 2021 (Ministerio de Tecnologías de la Información y Comunicaciones-MinTIC, Citation2022); that is, more than half of the country still lacks access to this service, which is necessary to make use of digital tools such as chatbot technology. Additionally (Garcia, Citation2022), argues that there is an absence of the common use of virtual assistants in markets due to a lack of connectivity. Likewise (Beltrán & Murcia, Citation2022), explains that, the low acceptance of digital transformation in business by consumers in Colombia is due to ignorance of the applications and the challenges of cultural changes.
With respect to university students (Gabrielli et al., Citation2021), argue that with the technological and digital era, they must be increasingly informed, with the ability to immediately consult information that is up-to-date, a situation that had greater relevance during the pandemic caused by COVID-19. The reason for the latter is that people from all economic sectors, including students and administrators in most universities, were challenged by several pandemic-related issues along with the restrictions imposed by the government, which faced an avalanche of questions and community consultations on different topics (Sweidan et al., Citation2021). Therefore, answering different questions instantly and accurately became necessary. Moreover, according to (Smutny & Schreiberova, Citation2020), during the pandemic, there was exponential growth in the mobile device market to maintain connectivity and remain informed.
Another point that justifies the importance of studying the use of chatbots in young university students is that today, students do not access useful information through traditional methods; rather, the use of digital media and technological tools is more popular (Sweidan et al., Citation2021). Despite the growing development of chatbots in the service industry, which indicates the continuous transformation taking place in the economic sector, research on the key attributes of the intention of continuity and satisfaction concerning the service based on conversational agents continues to emerge. In addition, developing chatbot user satisfaction studies is important to constantly improve the user experience, identifying potential problems and opportunities and correcting chatbot performance and personalization based on user needs, including establishing trust and value in the service.
Thus, this study offers valuable information on the characteristics and behaviours of the university population and on chatbot technology that may be important for developing strategies for the promotion and acceptance of this service among students. This research also complements the research gap, in terms of studies on developing economies, where innovation such as chatbots technologies are recognized as having the potential to generate a competitive advantage and contribute to the sustainable growth of companies in that context. Given the above, this study aims to identify the factors that influence user satisfaction among young university students in Medellin, Colombia, in the context of an emerging economy. To this end, the following research questions are proposed:
RQ1:
Is there a positive relationship between knowledge of use chatbots and access to updated information?
RQ2:
Is there a positive effect of paying attention to natural language when interacting with chatbots on access to updated information?
RQ3:
Does the resolution of pending cases have a positive effect on access to updated information via chatbots?
RQ4:
Is there a correlation between knowledge of use chatbots and the ability to effectively resolve pending cases?
RQ5:
Can it be confirmed that the resolution of pending cases through chatbots positively influences the attention paid to natural language during these interactions?
RQ6:
Can it be said that knowledge of use of chatbots has a positive effect on the attention paid to natural language when communicating with these systems?
This paper is structured as follows: it begins with the second section that presents a conceptualization and background on the use of chatbots, followed by the proposed model and the research hypothesis that links chatbots user satisfaction through the proposed factors: Knowledge of use, Attention to natural language, Access to updated information and Resolution of pending cases. The third section shows the methodological design for sample selection and data collection, detailing the data processing through the statistical technique Partial Least Squares Structural Equation Modeling (PLS-SEM) selected to analyse complex relationships between these observed and unobserved variables in the model. Next, the results obtained with other studies are described and discussed. Finally, the most important conclusions on the fulfillment of the proposed objective are presented and the practical implications, limitations and future study of the study are presented.
2. Theoretical background
The use of information systems based on natural language processing (NLP) is a field that has been studied for many years. This system has evolved in the last decade, allowing dialogues between a computer and a human to be established and is also known as Chatbot (Nain, Citation2018). More specifically (Puschmann, Citation2017), indicates that the word Chatbot originates from the terms “Chat” and “Bot” when referring to the interactions between human and machine in real time in the form of text-to-text, text-to-speech, voice-to-text or voice-to-speech communication.
According to (Kuberkar & Singhal, Citation2020), chatbots take as input the text, the voice or both as entered by the user; it processes the input based on trained algorithms and outputs a response to the user using contextual information to classify the user’s intentions. An example of these technologies is the chatbot assistants Apple Siri, Google Assistant and Amazon Alexa. According to (Androutsopoulou et al., Citation2019), the advent of smartphones has increased interest and access to these chatbots by anyone to ask questions and consult and translate information. Consequently, chatbots are considered among the best-known applications of artificial intelligence that continue to gain more ground in electronic commerce and services, as their implementation provides opportunities to improve customer service (Misischia et al., Citation2022).
2.1. Background on the use of chatbots and university students
The use of virtual assistants has been explored before in various contexts. On the one hand, in the face of digital transformation in the financial sector (Abdulquadri et al., Citation2021), have studied the implementation of chatbots to improve customer experience and financial inclusion in emerging markets and specially to contribute massively to personal banking. For this purpose, the researchers used the Search Access Test-SAT model to understand the adoption factors of these conversational agents in banks.
On the other hand, multiple studies on the use of chatbots using the technology adoption model (TAM) of Davis have been conducted (Davis & Davis, Citation1989), which is based on two key factors concerning the intention of use: perceived usefulness and ease of use. However, these models have been modified according to technological transformations, the advancement of task automation and the development of artificial intelligence. For example, extended factors have been added to the TAM model to better understand the adoption of virtual assistants, as in (Malik et al., Citation2021), who examined the use of chatbots by university students for e-learning, exploring two additional factors of this model: improved performance and perceived convenience. Additionally, in (Shamout et al., Citation2022), the following are proposed as influencing factors in adopting this technology: the technological context (relative advantage, complexity and cost), the organizational context (administrative support, financial support and competence of the employees) and the environmental context (competitive pressure, customer pressure and supplier support).
Additionally, the study by (Ho et al., Citation2018) reveals the information needs of the university student user since it is recommended that academic institutions divide their administrative attention between human and virtual academic advisors so that students receive the most personalized advisory service. This study demonstrated that the perception of the usefulness factor of the virtual counselling service determined whether students use it. The study by (Melián-González et al., Citation2021) analysed the use of chatbots by university students and applied it to the tourism sector, given the growing interest by hotels, travel agencies and airlines in incorporating them. Hence, it was shown that the intentions behind the use of chatbots are directly influenced by the following factors: the expected performance of chatbots, the habit of using chatbots, the hedonic component when using them, the predisposition to using self-service technologies, social influences, and whether the chatbot resembles human-like language and is not robotic.
Likewise, the application of virtual assistants in the teaching and learning processes of young university students has begun to be explored, as shown in (Gonda et al., Citation2018). Similarly (Gupta & Chen, Citation2022), focused on the development of a virtual assistant to answer requests about a course and its content, learning material, answering questions, personalizing learning and fostering the well-being of young people. In this sense (Malik, Citation2021), found that the factors of ease of use, usefulness, attitude and perceived convenience determine whether the use of chatbots by students in online learning processes is effective.
Despite the above research and the implementation of conversational agents in nearly all industries, there is no evidence on the factors that explain why student consumers are willing to interact with this technology. The reason for this lack of research is because, in regard to adopting and using new technological tools, the behaviour of the young generation is difficult to predict (Rivera et al., Citation2015). Furthermore, according to (Malik, Citation2021), the use of artificial intelligence is still in its infancy, particularly for developing countries and their technological gaps.
2.2. Proposed model and hypotheses
This section presents the conceptual model of this study, which consists of the following six hypotheses.
Relationship between the factors of knowledge of use (KU) and access to updated information (AUI): According to (Santos et al., Citation2021), knowing how to use tools with precision allows one to obtain the best results expected from the use of technology. Previous research has shown that usage awareness determines chatbot user satisfaction (Bonales et al., Citation2021; Hajal et al., Citation2021). It is also expected that chatbots help users carry out their tasks of exchanging updated information more effectively if users know how to use them, as demonstrated in (Nguyen & Liaw, Citation2022; Yang et al., Citation2022). Therefore, the following hypothesis is formulated.
H1:
Knowledge of use has a positive effect on access to updated information.
Relationship between attention to natural language (ANL) and AUI: According to (Garcia et al., Citation2022) and (Ramachandran, Citation2019), chatbots must have a high level of “humanity” and not use bot language so that the student receives simulated response options with human characteristics and the ability to imitate personality. The latter allows estimating the efficiency of the virtual assistant since its compatibility with the university scene makes it possible to better understand the problem for which it is consulted (Bonales et al., Citation2021). The dependability of AUI in conversational agents determines the continuity of use (Nguyen et al., Citation2021). Additionally, a previous study (Tlili et al., Citation2023) verified the relationship between satisfaction with using virtual assistants and the perceived quality of the information (accurate and up-to-date) of the chatbot by young university students. Therefore, the following is hypothesized:
H2:
Attention to natural language has a positive effect on access to updated information.
Relationship between resolution of pending cases (RPC) and AUI: (Abd-Alrazaq et al., Citation2020) showed that the performance of chatbots is measured by their responses to help solve users’ cases or concerns because their acceptance and use depends on this feature. In the past, studies of (Joshi, Citation2021) and (Chen et al., Citation2021) verified that one way to train a bot is resolving the user’s queries because it can accumulate information that will make it more effective in future concerns. When the virtual agent recalls previous conversations and solved cases, it becomes more adept at discovering the user’s precise intention based on available knowledge (Kuberkar & Singhal, Citation2020). Thus, these chatbots are constantly trained to provide current and specific information (Li et al., Citation2020). Thus, the study proposes the following hypothesis:
H3:
Resolution of pending cases has a positive effect on access to updated information.
Relationship between KU and RPC: In the literature, it has been empirically demonstrated that for a user to decide to adopt new technologies requires time, infrastructure and knowledge of the technological tool so that it can be used properly (Luo et al., Citation2022; Mokmin & Ibrahim, Citationn.a). According to (Sheehan et al., Citation2020), users of conversational agents seek to resolve issues about a service. However, this interaction is satisfactory if users are knowledgeable about technology because it enables them to better communicate their problem and understand the information received, increasing the satisfaction of using virtual assistants. Therefore, the following hypothesis is proposed:
H4:
Knowledge of use has a positive effect on resolving pending cases.
Relationship between RPC and ANL: According to (Kee et al., Citation2021), the chatbot solves the problems and cases of the user without excessive back-and-forth questions and facilitates user satisfaction. Additionally, the natural language used in the conversation with the virtual assistant influences the decision to use this technology (Alhumaid et al., Citation2022; Joshi, Citation2021). When attended to by a bot (Sheehan et al., Citation2020), verifies that users must experience human interaction; therefore, a robotic language decreases the adoption of this tool. This hypothesis is presented as follows:
H5:
Resolution of pending cases has a positive effect on attention to natural language
Relationship between KU and ANL: (Ramachandran, Citation2019) showed a correspondence between having knowledge about how the chatbot works and the latter’s ability to communicate in a friendly and social way because it makes it easier to access technology. Thus, a good conversation between the virtual agent and users is born from receiving attention that is more human (Cordeiro & Batista, Citation2020; Singh & Thakur, Citation2020). This design encourages the consumer to decide to use and feel satisfied with this technology. This reasoning is formulated as the following hypothesis:
H6:
Knowledge of use has a positive effect on attention to natural language.
For this study, it was suggested that there is a connection between user satisfaction when using chatbots and accessing updated information provided by this artificial intelligence. This follows what was stated in (Sweidan et al., Citation2021), where in the current context, young university students tend to seek information through digital media and technological tools instead of traditional methods, in order to have real-time and updated information. Therefore, the availability of relevant and real-time information by chatbots contributes to a more satisfactory experience in its use. Considering the above, the definitions of the factors that originated the hypotheses served as the basis for proposing this original model, so the questions in the questionnaire are also original. Figure presents the model proposed in this study:
3. Materials and methods
This study was developed from a methodology using a quantitative approach and inferential scope proposing Partial Least Squares Structural Equations Model (PLS-SEM) which was empirically validated through the application of a self-administered questionnaire, focused on to identify the factors that influence user satisfaction among young university students in Medellin, Colombia, in the context of an emerging economy. Initially, the questionnaire was designed to focus on five specific sections: the first section corresponds to the sociodemographic information of the surveyed sample; the second section captures the knowledge and importance of chatbots; the third section focuses on the financial information of the respondents; the fourth section describes the instrument facilitating the analysis of the proposed connections between the constructs to be evaluated on a Likert scale; and finally, the fifth section presents the expectations regarding the virtual assistance service.
Similarly, according to (Huang, Citation2021), PLS-SEM is a technique used for the detection or development of predictive models, useful for examining causality between latent variables and has proven to be more appropriate than the Structural Linear General Relationship Model, which is very used in exploratory research.
In other words, PLS-SEM focuses on determining if there is a significant causal relationship between the variables of interest, which makes it a suitable tool for the creation of theoretical models (Tobi & Kampen, Citation2018). Thus, the choice of this PLS-SEM technique is presumed adequate for this study, where it was used as a method to explore the relationship between the variables: Knowledge of use, Attention to natural language, Access to updated information, Resolution of pending cases.
3.1. Instrument
The survey consists of an instrument that was developed from questions to analyse the proposed connections between the constructs and was evaluated on a five-point Likert scale using statements ranging from totally disagree to totally agree. Next, Table presents the factors and items to be analysed in this study, with their respective descriptions.
Table 1. Items of the questionnaire used in the study
3.2. Sample
The information used in this study was collected through a survey was distributed during the last quarter of the year 2022 to a sample of 240 people, of which 126 usable responses were received for the analysis, indicating a response rate of 52.5%, which according to (Sekaran & Bougie, Citation2016) is valid and statistically acceptable. The participants were students in their last semester of technical, technological, professional, and graduate programs in the areas of administration, economics, and technology from two universities in Medellin, Colombia and were selected by non-probabilistic sampling at the convenience of the study, i.e., participants accessible to the researcher as proposed by (Mweshi & Sakyi, Citation2020). The survey was conducted using Google Forms. In the first section of the survey, participants were asked for socio-demographic information; most respondents were between the ages of 27 and 35. The next section assessed knowledge and importance of chatbots in financial services, with almost all respondents indicating that they were aware of chatbots. In terms of financial information, most participants had a savings or checking account as a financial product. Finally, the expectations of the virtual assistance service show that the service is expected to be fast and to solve problems.
The context in which the study was conducted is related to the municipality of Medellín, and the choice of this context is based on its suitability to represent a diverse population of university students. Medellín, as a developing educational and technological center, provides a conducive environment to study technology adoption among youth. University students, as a relevant and technologically connected audience, provide an appropriate approach to analyze the adoption of chatbots in education and beyond. The choice of the community and the student audience is based on the idea that the results obtained can have broad and practical implications for the design of assistance services and the adoption of technology in other similar settings. Table below presents the socio-demographic information of the sample and additional details about the study.
Table 2. Sociodemographic questions and complementary information
From the responses of the participants, it can be seen that the most common age range among the respondents was 27 to 35 years, followed by the range of 18 to 26 years. Another characteristic of the students is that most of them were studying at the technological and professional academic level and, at the same time, more than half worked and earned between 1 and 3 legal minimum wages. The sample also reveals that most know what a chatbot is, however, many do not consider it important for the use of financial services. Among the financial products most used by students are credit cards and checking or savings accounts. They also stated that the satisfaction of using the chatbot in these services is excellent and good for its ability to help solve the problem. This may also be related to the fact that most of the students belong to the Millennial generation or digital natives, which may facilitate their affinity with these technologies. But almost all young students expect technology to have a language similar or close to their own, as this will influence their ability to understand the responses that chatbots provide.
3.3. Data processing and instrument
To analyse the results generated from the instrument, a factor analysis was used to test the causal connections proposed using the statistical software SmartPLS 4 (Ringle et al., Citation2022). In this manner, the convergent validity, the discriminant validity, and the reliability of the constructs are examined, and finally, the hypotheses are contrasted to analyse the results, which will facilitate the model construction for the use of chatbots among young university students.
4. Analysis of results
For the analysis of the results, this study uses a structural equation model (SEM), which is considered a multivariate multiple regression technique that allows to examine the extent to which the variables can be measured, that is, unobservable latent variables (constructs). Likewise, the SEM allows modeling the relationships between several predictor variables (independent or exogenous) and criterion variables (dependent or endogenous) (Lowry, Citation2014). In this sense, partial least squares (PLS-SEM) is used with a predictive rather than confirmatory approach. Next, the measurement model is examined to determine the convergent and discriminant validity of the model, and the structural model is also analyzed with PLS estimation (Norabuena Mendoza et al., Citation2021).
4.1. Measurement model
Convergent validity is the degree of certainty that the proposed indicators measure the same latent variable or factor. The purpose of determining whether a measurement model has convergent validity is to indicate whether the construct is adequately measured by the proposed indicators. The measure is determined by the magnitude of the factor loadings and their level of significance, such that the measures must result in a positive correlation to be considered valid (Gheshlagh et al., Citation2018).
Subsequently, the indicators are subjected to an acceptance criterion, where each item must obtain a value greater than 0.7 (Lloret-Segura et al., Citation2014; Martínez & Sepúlveda, Citation2012). Thus, in Table , the factor loadings for the chatbot use model by young people are presented; the table indicates that each of the constructs meets the acceptance criteria, both at the individual level and in terms of the values obtained in the averages. Finally, it was necessary to eliminate the item “ANL3” from the construct “attention to natural language” since it did not comply with the established criteria; therefore, the results are translated into four factors and 12 items.
Table 3. Cross factor loadings
Table presents the convergent validity statistics of the measurement model. The factor loads that meet the previously established criteria are included, as well as the value of the variance inflation factor (VIF) to ensure that the model does not have multicollinearity problems that affect internal validity. It has been established in the literature that VIF values should not exceed 5 (Ramírez et al., Citation2014). Another statistic analyzed is the average variance extracted (AVE), which indicates that the latent variable (construct) explains more than half of the variance of its indicators. Similarly, it postulates that the construct shares more variance with its assigned indicators compared to other constructs in the model. Therefore, values greater than 0.5 are recommended (Alamer, Citation2022).
Table 4. Convergent validity of the model
The internal consistency method based on Cronbach’s Alpha (CA) and Composite Reliability (RC) reliability coefficient allows estimating the reliability of a measurement instrument with a set of items that are expected to measure the same construct or a single theoretical dimension of a latent construct; in other words, it is the average of the correlations between the items that are part of an instrument (Streiner, Citation2003).
CA and CR value ranges from 0 to 1; the closer the alpha value is to 1, the greater the internal consistency of the items analysed. If the items are positively correlated, then the variance in the sum of the items increases. Therefore, if the scores on all items were identical, and therefore, the scores were perfectly correlated, the value would be equal to 1. On the other hand, if the items were totally independent, they would not show any relationship (Frías-Navarro & Soler, Citation2012). The measure can be interpreted with different guidelines; however, the most used are as follows: values less than 0.5 are considered unacceptable; from 0.5 to 0.59, poor; from 0.6 to 0.69, questionable; from 0.7 to 0.79, acceptable; from 0.8 to 0.89, good; and from 0.9 to 1, excellent (Gliem & Gliem, Citation2003).
Discriminant validity is one of the most widely used statistical tools to evaluate measurement scales in qualitative research; it is responsible for the measurements of the constructs or variables used in multiple disciplines of knowledge (Prieto & Delgado, Citation2010), is an indispensable condition for the development and comparison of scientific theories in these fields of knowledge (Martínez-García & Martínez-Caro, Citation2009).
As an acceptance criterion, for the measurements of the same construct to be valid, they must have high correlation values that show convergent validity; however, for there to be discriminant validity, this correlation must be lower, showing differentiation between constructs (Cheung et al., Citation2023). In this study, discriminant validity is assessed using the Fornell-Lacker criterion, which, according to (Maur, Citation2023), postulates that a latent construct shares more variance with its assigned indicators than with another latent variable in the structural model. Consequently, the values of each construct should be higher than the others. This can be seen in Table , where it is evident that this criterion is met for the measurement model, thus maintaining that the model is reliable and valid in convergent and discriminant terms.
Table 5. Fornell-Lacker criterion
4.2. Structure model
The evaluation of the relationships in the structural model is performed using the path coefficients. For this purpose, the statistics Path, T-value and p-value are used, which are calculated using the bootstrapping method in SmartPLS 4, which is considered to be the most widely used method for estimating the standard error (Kock, Citation2014). According to the literature, the appropriate values for the p-value are a significance level of 0.05 (1–95%), so if p ≤ 0.05, the hypothesis is accepted. In addition, the T value is considered as a p-test evaluation where the threshold value is set at 1.96 (Kock, Citation2016). The results are shown in Table and show that four of the six hypotheses proposed for the model are met.
Table 6. Hypothesis testing
The results allow us to report that access to updated information is positively affected only by the resolution of pending cases. In this way, it is shown that the knowledge of use and the attention with the robotic language are not relevant to the access to updated information on chatbots in university students. The most relevant relationship is given by the knowledge of use for the resolution of pending cases, and this, in turn, on the attention with robotic language. To support the results of a predictive approach, the R2, i.e. the proportion of total variance explained (Hair et al., Citation2017), is evaluated and can be seen in Figure . The researchers suggest that values of 0.25 are considered weak, values of 0.5 moderate and substantial values of 0.75 to support the prediction of endogenous variables (Binz Astrachan et al., Citation2014). The variable access to updated information received a substantial value, the variable care with robotic language moderate and the variable resolution of pending cases weak.
Figure 2. Model of chatbot use among young university students. Note: *R2; **p value. Source: the Authors.
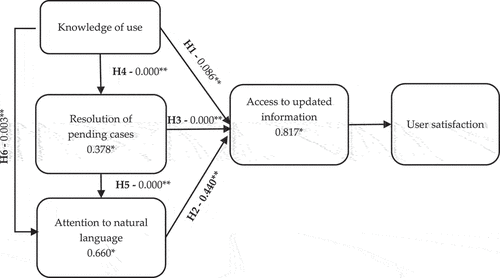
Likewise, the size of the f2 effects is evaluated in each of the relationships, also with a predictive purpose. For this case, the evaluators report that values of 0.02 to 0.15 are considered small effects, values of 0.15 to 0.35 medium effects, and values greater than 0.35 large effects (Legate et al., Citation2021). The relationships: Resolution of pending cases → Access to updated information (1.069), Resolution of pending cases → Attention to natural language (0.805) and Knowledge of use → Resolution of pending cases (0.607) obtained large effects. The other relationships obtained weak effects. The results are also presented in the SmartPLS graph, as shown in Figure .
Finally, as a last predictive approach, Q2 is examined, which is considered relevant with values greater than 0 in the redundancy report with cross-validation indicating predictive relevance. Similarly, the values of 0.02, 0.15, and 0.35 reflect that an endogenous variable has small, medium, or large predictive relevance, respectively (Kock, Citation2016). Thus, the three endogenous variables access to updated information (0.393), care with robotic language (0.371) and resolution of pending cases (0.364) stand out with great predictive relevance. With this, it is shown that the model proposed in this study meets the validity and construction criteria, demonstrating predictive relevance.
5. Discussion
This study contribute to the growing body of knowledge surrounding the utilization and acceptance of chatbots, particularly among young university students. By delving into the factors that influence user satisfaction with chatbot technology, this research enhances our understanding of the complex interplay between various dimensions.
In (Rossmann et al., Citation2020), the influence of the conceptualized dimensions of the service, the service costs, and reuse intention on customer satisfaction is also evidenced, which contrasts with the results obtained in this study. However, in previous studies, functionality, reliability, security, usability related to usage knowledge, graphic appearance, human-sounding machine language, personalization and productivity, which related to resolution of pending cases and have also been demonstrated to be key factors likely to influence user perspectives on using chatbots (Meerschman & Verkeyn, Citation2019), echoing the results obtained. The above can also be reinforced by (Bilquise et al., Citation2023) where anthropomorphic characteristics of the chatbot are relevant to the satisfaction and use of these technologies by obtaining a significant p-value in their study.
For this reason, this study provides theoretical contributions to the topic of the use and acceptance of chatbots by young university students and identifies the most relevant factors that influence satisfaction with the use of this type of technology.
On the other hand, the results of (Pillai & Sivathanu, Citation2020) indicate that the predictors of chatbot use for hotel and tourism services in India are variables related to the TAM, where the intention to use the technology is determinant to know the chatbots functionalities. Likewise (Ashfaq et al., Citation2020), revealed that satisfaction with the electronic chatbot service is a strong determinant and predictor of user satisfaction with chatbots. Therefore, chatbots must improve the quality of their information and service to increase user acceptance. Finally, the study by (Kasilingam, Citation2020) where they sought to identify the association of determinant variables and the intention to use chatbots to buy, confirmed that the satisfaction of using chatbots is considerably influenced by knowledge of use; in turn (Kwangsawad & Jattamart, Citation2022), investigated the potential variables that influence users’ intentions to use chatbot services in a community enterprise, highlighting the importance of access to up-to-date information for decision-making.
Chatbots have been identified by companies as an excellent opportunity to better exploit social networks and obtain competitive differentiation through relationship marketing in the digital environment (Gunawan & Sulaeman, Citation2020). Furthermore, it is a relatively new technology and is expected to dominate mobile commerce and shopping applications in the future (Pillai & Sivathanu, Citation2020). Because user satisfaction stimulates the proper adoption of the technology, providing a competitive advantage and a positive impact on profitability, it is important to keep up-to-date with the latest technologies [83]. Hence, it is important to know the factors involved and staying aligned with user preferences and adapting strategies accordingly will be critical for organizations looking to harness the potential of chatbots effectively.
6. Conclusions
This research identified that the proposed factors, knowledge of use, attention to natural language, access to updated information, and resolution of pending cases, influence university users’ satisfaction with chatbot technology. The results also indicate that for university students, there is a strong relationship between resolution of pending cases and perceived access to updated information, which suggests the importance of solve cases with this technology to assimilate and take advantage of the information the bot provides in conversation. Likewise, young university students think that having access to updated information during the chatbot experience is related to the resolution of pending cases, that is, resolving doubts and queries with the conversational agent.
On the other hand, this type of empirical research reveals the importance of knowing the factors that affect the use of chatbots independent of the population, in this case, young university students. In turn, this type of conversation systems technology paves the way to recommend the inclusion of other variables that may become relevant according to the application of the chatbot, such as variables that seek to make the technology appropriate for two-way benefit: customers and company.
The results also suggest that chatbots are a technology that offers great opportunities, which has even been adapted to the contexts of many countries, companies and institutions due to the various conditions to which it may be subjected. Its adaptability makes it a relatively new technology that has emerged in different areas, especially in the market, since its excellent programming and operation has made people interested in knowing about chatbots and implementing them according to their needs.
These findings make it possible to empirically evaluate the usability and experience of university students with the chatbot-based advisory system, opening many future lines for researchers to explore the design of these conversational agents and thus obtain better coverage and efficiency in services where they are implemented. In this sense, it is recommended to investigate the impact of chatbots with anthropomorphic characteristics on students and their applicability in social networks.
6.1. Limitations
The provided text discusses a study on the use of chatbots among young college students and presents a model to analyze the factors that influence the adoption and acceptance of chatbot technology. Based on the text, several limitations of the study can be identified. First, the sample size of 126 participants could be considered relatively small to draw strong conclusions, especially considering the diversity that exists among college students. In addition, most of the participants in the study were technical workers or technologists, which could introduce a bias toward those with more technological familiarity.
Another limitation relates to the generalizability of the findings. The study focused on specific young university students from the municipality of Medellín, which may limit the applicability of the findings to a broader population. Cultural, geographic, and contextual differences between different regions could affect the applicability of the results elsewhere.
In addition, it is important to consider that the data used in the study were collected through questionnaires, which means that they are based on the self-reported responses of the participants. This approach could introduce biases such as social desirability bias, where participants respond in ways they perceive to be socially acceptable rather than reflecting their true attitudes.
The design of the study is also a potential limitation. The use of a cross-sectional design means that the data was collected at a single point in time, which may not fully capture the dynamics of how adoption and acceptance of chatbot technology change over time. In addition, the study tends to focus mainly on internal factors that influence chatbot adoption among university students, while other external factors such as institutional support, availability of alternative resources, and technological infrastructure could play a significant role in adoption, technology and were not extensively explored in the study.
6.2. Practical implications
This study has important practical implications for chatbot designers and developers, especially in governments of developing countries similar to Colombia. The study highlights key expectations around the use of chat agents, such as problem solving, speed of service, and service effectiveness. These findings suggest that chatbot designers should prioritize the ability to solve problems efficiently and quickly and provide an effective service that meets user demands.
Particularly in developing countries, where resources may be limited, this study urges designers and developers to focus on optimizing chatbots to address specific problems and provide effective solutions. In addition, speed of service is identified as a key expectation, underscoring the importance of developing chatbots that can provide quick and accurate responses to improve the user experience.
The practical implications of this study extend beyond the realm of chatbot designers and developers to governments in developing countries. The findings suggest that the successful implementation of chatbots in government services could play a fundamental role in citizen satisfaction by addressing their needs in a timely and effective manner. This suggests that governments, particularly in countries with limited resources, could take advantage of chatbot technology to streamline customer service processes and provide effective responses to citizen inquiries.
As a result, governments in developing countries could explore the strategic integration of chatbots into various platforms and public services. In doing so, they could not only improve access to government information and services, but also free up valuable resources by automating repetitive and routine tasks. In addition, this integration could result in greater operational efficiency and a more positive experience for citizens seeking to resolve issues and access services in a timely manner. As a result, this study suggests that chatbots could be a valuable tool for improving the quality of interaction between citizens and government services in resource-constrained environments.
This study not only has practical implications for chatbot designers and governments in developing countries, but also opens up perspectives in education. The results highlight the importance of speed, efficiency, and problem-solving in the services provided by chatbots, characteristics that could be applied in educational environments to provide instant answers to students’ queries and support in various aspects of learning. The strategic integration of chatbots in education could improve the learning experience, facilitate communication between students and teachers, and promote faster access to educational resources.
6.3. Future research
In terms of future research, it is recommended that the application of the instrument be extended to other demographic groups, particularly those who are not students. This approach would allow for a more complete understanding of the consumer experience across different services. By including individuals who are not part of a university environment, a broader range of perspectives and expectations regarding the adoption and use of chatbots could be captured. This expansion of demographic reach could shed light on how different demographics perceive and use this emerging technology in a variety of contexts.
Additionally, the inclusion of more diverse demographic groups could provide valuable insights to improve companies that are in the early stages of integrating chatbots into their services. By understanding how people outside of the university setting experience interacting with chatbots, these companies could adjust their deployment and customization strategies to more effectively address the needs and preferences of a broader audience. This future research could also identify potential barriers or unique challenges that may arise when deploying chatbots to different demographics, allowing companies to anticipate and address potential issues early on.
Finally, in terms of future research, it would be valuable to extend this study to explore how the findings could be applied in broader contexts, such as m-commerce, citizen service-oriented public institutions, and the development of intelligent robots. For example, one could explore how consumers’ expectations of fast and effective problem-solving translate into interactions with chatbots on mobile commerce platforms, and how these perceptions affect purchase decisions and customer loyalty. In addition, understanding how citizens interact with chatbots in public institutions could lead to significant improvements in government service delivery, optimizing information delivery and citizen service. As for intelligent robots, exploring how they can be effectively integrated into different industries and environments to improve user experience and operational efficiency would be a promising area of research that would further expand the scope of this study.
Author contributions
Jerri Alejandro López Sánchez and Maria Camila Bermeo Giraldo designed this study. Alejandro Valencia Arias carried out the conceptualization of the study and data curation. Raúl Eduardo Bao García wrote the first version of the methodology and the results section. Ada Gallegos wrote the first version of the summary and discussion section, and realized project management. Jerri Alejandro López Sánchez, Alejandro Valencia Arias and Maria Camila Bermeo reviewed and critically edited the entire manuscript. All authors approved the final version of this article for publication.
Acknowledgments
We would like to thank Universidad Señor de Sipán (Perú) for translating the manuscript into English.
Disclosure statement
No potential conflict of interest was reported by the author(s).
Data availability statement
The data that support the findings of this study are available from the corresponding author upon reasonable request.
Additional information
Funding
Notes on contributors
Jerri Alejandro López-Sánchez
Jerri Alejandro López-Sánchez received the title of Biomedical Engineer in 2019 and the degree of master’s in management of Technological Innovation, Cooperation and Regional Development in 2023 from the ITM University Institution of Colombia. He has 4 years of experience as an innovation manager. His main fields of study are open innovation, entrepreneurship, technologies 4.0, technology transfer and the social appropriation of knowledge.
María Camila Bermeo-Giraldo
Maria Camila Bermeo-Giraldo received the degree of Industrial Engineer in 2015 and the degree of master’s in administrative engineering in 2020 from the Faculty of Mines of the National University of Colombia. She has 5 years of experience as a university professor. Her main fields of study are innovation, simulation, ethical simulation, ethical climate and technology transfer and management. technology transfer and management.
Alejandro Valencia-Arias
Alejandro Valencia-Arias received a degree in Administrative Engineering in 2010, a master’s degree in systems Engineering in 2013 and a PhD in Engineering in 2018 from Universidad Nacional Colombia. He has 9 years of experience as a university professor. His research interests include simulation, marketing research and statistics. He also has experience in the area of agent-based modelling and system dynamics, and his specialty is the development of social models.
Raúl Eduardo Bao García
Raúl Bao García serves as the Academic Vice Chancellor at USMP and has been a member of the University Council since June 2006. He earned his bachelor’s degree in industrial engineering from the National University Federico Villarreal. Additionally, he holds a master’s degree in education with a focus on Computer and Educational Technology from the University of Hartford in Connecticut, USA. Dr. Bao García also holds a Doctorate in Business Administration from ESAN-ESADE in Barcelona, Spain, and a Doctorate in Education from the National University Federico Villarreal in Peru. His expertise spans across the fields of education, technology, and business administration.
Ada Gallegos
Ada Gallegos is a university lecturer and researcher. She holds a Doctorate in Government and Public Policies as well as a Doctorate in Education. Her specialization in Public Affairs was completed as a Fulbright scholar through the Hubert Humphrey program, certified by then-President of the United States, Barack Obama. As a dedicated researcher, she leads research teams composed of both national and international scholars. On an international level, she serves as the Chair of the Board for the Consortium for Women Leaders in Public Service (CWLPS), headquartered in Washington DC.
References
- Abd-Alrazaq, A., Safi, Z., Alajlani, M., Warren, J., Househ, M., & Denecke, K. (2020). Technical metrics used to evaluate health care chatbots: Scoping review. Journal of Medical Internet Research, 22, e18301. https://doi.org/10.2196/18301
- Abdulquadri, A., Mogaji, E., Kieu, T. A., & Nguyen, N. P. (2021). Digital transformation in financial services provision: A Nigerian perspective to the adoption of chatbot. Journal of Enterprising Communities: People and Places in the Global Economy, 15(2), 258–20. https://doi.org/10.1108/JEC-06-2020-0126
- Alamer, A. (2022). Exploratory structural equation modeling (ESEM) and bifactor ESEM for construct validation purposes: Guidelines and applied example. Research Methods in Applied Linguistics, 1(1), 100005. https://doi.org/10.1016/j.rmal.2022.100005
- Alhumaid, K., Alnazzawi, N., Akour, I., Khasoneh, O., Alfaisal, R., & Salloum, S. (2022). An integrated model for the usage and acceptance of stickers in WhatsApp through SEM-ANN approach. International Journal of Data & Network Science, 6(4), 1261–1272. https://doi.org/10.5267/j.ijdns.2022.6.008
- Andronie, M., Lăzăroiu, G., Ștefănescu, R., Ionescu, L., & Cocoșatu, M. (2021). Neuromanagement decision-making and Cognitive Algorithmic processes in the technological adoption of mobile commerce Apps. Oeconomia Copernicana, 12(4), 863–888. https://doi.org/10.24136/oc.2021.034
- Androutsopoulou, A., Karacapilidis, N., Loukis, E., & Charalabidis, Y. (2019). Transforming the communication between citizens and government through AI-guided chatbots. Government Information Quarterly, 36, 358–367. https://doi.org/10.1016/j.giq.2018.10.001
- Ashfaq, M., Yun, J., Yu, S., & Loureiro, S. M. C. I. (2020). I, chatbot: Modeling the determinants of users’ satisfaction and continuance intention of AI-powered service agents. Telematics and Informatics, 54, 101473. https://doi.org/10.1016/j.tele.2020.101473
- Beltrán, M. A., Murcia, C. E. Integración del Digital Business a la banca en Colombia y la adaptación de sus consumidores, Trabajo de grado, Universidad Cooperativa de Colombia. Retrieved July 18, 2022, from http://repository.ucc.edu.co/bitstream/20.500.12494/15553/1/2019_Digital_banca_revolucion.pdf
- Bilquise, G., Ibrahim, S., & Salhieh, S. E. M. (2023). Investigating student acceptance of an academic advising chatbot in higher education institutions. Education and Information Technologies, 1–26. https://doi.org/10.1007/s10639-023-12076-x
- Binz Astrachan, C., Patel, V. K., & Wanzenried, G. (2014). A comparative study of CB-SEM and PLS-SEM for theory development in family firm research. Journal of Family Business Strategy, 5(1), 116–128. https://doi.org/10.1016/j.jfbs.2013.12.002
- Bonales, G., Pradilla, N., & Martínez, E. (2021). Chatbot como herramienta comunicativa durante la crisis sanitaria COVID-19 en España. ComHumanitas: revista científica de comunicación, 11(3), 1–22. https://doi.org/10.31207/rch.v11i3.270
- Chen, J. V., Thi Le, H., & Tran, S. T. T. (2021). Understanding automated conversational agent as a decision aid: Matching agent’s conversation with customer’s shopping task. Internet Research, 31(4), 1376–1404. https://doi.org/10.1108/INTR-11-2019-0447
- Cheung, G. W., Cooper-Thomas, H. D., Lau, R. S., & Wang, L. C. (2023). Reporting reliability, convergent and discriminant validity with structural equation modeling: A review and best-practice recommendations. Asia Pacific Journal of Management;, 2023, 1–39. https://doi.org/10.1007/s10490-023-09871-y
- Cordeiro, I. D., & Batista, I. M. D. S. (2020). La experiencia del usuario en el proceso de adquirir información para planear el viaje el caso del chatbot de kayak. Estud Perspect Tur, 29(3), 792–816. http://www.scielo.org.ar/scielo.php?script=sci_arttext&pid=S1851-17322020000300792&lng=es&tlng=es
- Davis, F., & Davis, F. (1989). Perceived usefulness, perceived ease of use, and user acceptance of information technology. MIS Quarterly, 13(3), 319–339. https://doi.org/10.2307/249008
- Dijmărescu, I., Iatagan, M., Hurloiu, I., Geamănu, M., Rusescu, C., & Dijmărescu, A. (2022). Neuromanagement decision making in facial recognition biometric authentication as a mobile payment technology in retail, restaurant, and hotel business models. Oeconomia Copernicana, 13(1), 225–250. https://doi.org/10.24136/oc.2022.007
- Følstad, A., Nordheim, C. B., & Bjørkli, C. A. (2018). What makes users trust a chatbot for customer service? An exploratory interview study. In S. S. Bodrunova (Ed.), Internet Science (pp. 194–208). Springer International Publishing.
- Frías-Navarro, D., & Soler, M. P. (2012). Prácticas del análisis factorial exploratorio (AFE) en la investigación sobre conducta del consumidor y marketing. Suma Psicol, 19(1), 45–58. http://www.scielo.org.co/scielo.php?script=sci_arttext&pid=S0121-43812012000100004&lng=en&tlng=es
- Gabrielli, S., Rizzi, S., Bassi, G., Carbone, S., Maimone, R., Marchesoni, M., & Forti, S. (2021). Engagement and effectiveness of a healthy-coping intervention via chatbot for university students during the COVID-19 pandemic: Mixed methods proof-of-concept study. JMIR Mhealth Uhealth, 9(5), e27965. https://doi.org/10.2196/27965
- Garcia, J. F. Desarrollo de un chatbot como apoyo a la experiencia del cliente en un hotel en Colombia, Trabajo de grado, Universidad de los Andes. Retrieved July 20, 2022, from https://repositorio.uniandes.edu.co/bitstream/handle/1992/55148/26483.pdf?sequence=1&isAllowed=y.
- Garcia, G., Fuertes-Alpiste, M., Molas-Castell, N. Briefing paper: Los chatbots en educación. Retrieved September 20, 2022, from http://openaccess.uoc.edu/webapps/o2/handle/10609/85786.
- Gheshlagh, R. G., Tabrizi, K. N., Dalvandi, A., & Ebadi, A. (2018). Development and validation of resilience scale in patients with cardiovascular and respiratory diseases. Iranian Red Crescent Medical Journal, 20, 7. https://doi.org/10.5812/ircmj.14129
- Gliem, J. A., Gliem, R. R. (2003). Calculating, interpreting, and reporting Cronbach’s alpha reliability coefficient for Likert-type scales. Proceedings of the Midwest Research-to-Practice Conference in Adult, Continuing, and Community, Columbus, Ohio (pp. 83–88). The Ohio State University.
- Gonda, D., Lei, C. U., Chai, Y., Hou, X., Tam, V. (2018). Pre-conference workshop—Chatbot tutors for blended learning: Why bother? And where to start? In 2018 IEEE International Conference on Teaching, Assessment, and Learning for Engineering (TALE), 1211–1212. IEEE.
- Graesser, A. C. (2016). Conversations with AutoTutor help students learn. International Journal of Artificial Intelligence in Education, 26(1), 124–132. https://doi.org/10.1007/s40593-015-0086-4
- Gunawan, G., & Sulaeman, M. (2020). Determining factors in the use of digital marketing and its effect on marketing performance in the creative industries in Tasikmalaya. Budapest International Research and Critics Institute (BIRCI-Journal): Humanities and Social Sciences, 3(3), 2543–2550. https://doi.org/10.33258/birci.v3i3.1239
- Gupta, S., & Chen, Y. (2022). Supporting inclusive learning using chatbots? A chatbot-led interview study. Journal of Information Systems Education, 33(1), 98–108. https://aisel.aisnet.org/jise/vol33/iss1/11
- Hair, J., Hollingsworth, C. L., Randolph, A. B., & Chong, A. Y. L. (2017). An updated and expanded assessment of PLS-SEM in information systems research. Industrial Management & Data Systems, 117(3), 442–458. https://doi.org/10.1108/IMDS-04-2016-0130
- Hajal, G. E., Daou, R. A. Z., Ducq, Y. (2021). Human firewall: Cyber awareness using WhatApp AI chatbot. Proceedings of the 2021 IEEE 3rd International Multidisciplinary Conference on Engineering Technology (IMCET), Beirut, Lebanon (pp. 66–70). IEEE.
- Ho, C. C., Lee, H. L., Lo, W. K., Lui, K. F. A. (2018). Developing a chatbot for college student programme advisement. Proceedings of the 2018 International Symposium on Educational Technology (ISET), Osaka, Japan (pp. 52–56). IEEE.
- Huang, C. H. (2021). Using PLS-SEM model to explore the influencing factors of learning satisfaction in Blended learning; Education Sciences. Education Sciences, 11(5), 249. https://doi.org/10.3390/EDUCSCI11050249
- Joshi, H. (2021). Perception and adoption of customer service chatbots among millennials: An empirical validation in the Indian context. Proceedings of the 17th International Conference on WebInformation Systems and Technologies (WEBIST 2021), Valletta, Malta (pp. 197–208). ScitePress.
- Kasilingam, D. L. (2020). Understanding the attitude and intention to use smartphone chatbots for shopping. Technology in Society, 62, 101280. https://doi.org/10.1016/j.techsoc.2020.101280
- Kee, K. F., Calyam, P., & Regunath, H. (2021). The role of vidura chatbot in the diffusion of KnowCOVID-19 gateway. Human-Machine Communication, 3, 47–64. https://doi.org/10.3316/INFORMIT.099941590265302
- Kock, N. (2014). Stable P value calculation methods in PLS-SEM. Laredo, TX: ScriptWarp Systems, 1–15.
- Kock, N. (2016). Hypothesis testing with confidence intervals and P values in PLS-SEM. Hypothesis testing with confidence intervals and P values in PLS-SEM. International Journal of E-Collaboration, 12(3), 1–6. https://doi.org/10.4018/IJeC.2016070101
- Kuberkar, S., & Singhal, T. K. (2020). Factors influencing adoption intention of AI powered chatbot for public transport services within a smart city. International Journal of Emerging Technologies in Learning, 11(3), 948–958 https://www.academia.edu/download/63770912/AI_Chatbot_for_Public_Transport20200628-57077-1v0mieb.pdf.
- Kwangsawad, A., & Jattamart, A. (2022). Overcoming customer innovation resistance to the sustainable adoption of chatbot services: A community-enterprise perspective in Thailand. Journal of Innovation & Knowledge, 7(3), 100211. https://doi.org/10.1016/j.jik.2022.100211
- Legate, A. E., Hair, J. F., Jr., Chretien, J. L., & Risher, J. J. (2021). PLS-SEM: Prediction-oriented solutions for HRD researchers. Human Resource Development Quarterly, 34(1), 91–109. https://doi.org/10.1002/hrdq.21466
- Li, Y., Grandison, T., Silveyra, P., Douraghy, A., Guan, X., Kieselbach, T., Li, C., Zhang, H. (2020). Jennifer for COVID-19: An NLP-powered chatbot built for the people and by the people to combat misinformation. Proceedings of The NLP COVID-19 Workshop (pp. 1–9). Association for Computational Linguistics.
- Lloret-Segura, S., Ferreres-Traver, A., Hernández-Baeza, A., & Tomás-Marco, I. (2014). El análisis factorial exploratorio de los ítems: Una guía práctica, revisada y actualizada. Anales de Psicología, 30(3), 1151–1169. https://doi.org/10.6018/analesps.30.3.199361
- Lowry, P. B. (2014). James G. Partial least squares (PLS) structural equation modeling (SEM) for building and testing behavioral causal theory: When to choose it and how to use it. IEEE Transactions on Professional Communication;, 5(2), 123–146. https://doi.org/10.1109/TPC.2014.2312452
- Luo, B., Lau, R. Y. K., Li, C., & Si, Y.-W. (2022). A critical review of state-of-the-art chatbot designs and applications. WIREs Data Mining and Knowledge Discovery, 12(1), e1434. https://doi.org/10.1002/widm.1434
- Malik, M. (2021). A review of empirical research on internet & mobile banking in developing countries using UTAUT model during the period 2015 to April 2020. Journal of Internet Banking and Commerce, 25(2), 1–22. https://www.researchgate.net/profile/Mehreen-Malik-2/publication/351074027_A_Review_of_Empirical_Research_on_Internet_Mobile_Banking_in_developing_countries_using_UTAUT_Model_during_the_period_2015_to_April_2020/links/60831d48907dcf667bbd935b/A-Review-of-Empirical-Research-on-Internet-Mobile-Banking-in-developing-countries-using-UTAUT-Model-during-the-period-2015-to-April-2020.pdf
- Malik, R., Shrama, A., Trivedi, S., & Mishra, R. (2021). Adoption of chatbots for learning among university students: Role of perceived convenience and enhanced performance. International Journal of Emerging Technologies in Learning (iJet), 16(18), 200–212. https://doi.org/10.3991/ijet.v16i18.24315
- Martínez-García, J. A., & Martínez-Caro, L. (2009). La validez discriminante como criterio de evaluación de escalas. ¿teoría o estadística? Universitas Psychologica, 8(1), 27–36. http://www.scielo.org.co/scielo.php?script=sci_arttext&pid=S1657-92672009000100002&lng=en&tlng=es
- Martínez, C. M., & Sepúlveda, M. A. R. (2012). Introducción al análisis factorial exploratorio. Revista colombiana de psiquiatria, 41, 197–207. https://doi.org/10.1016/S0034-7450(14)60077-9
- Maur, A. (2023). Evaluation of the Unmodified measurement models. Electronic Feedback in large university statistics Courses: The Longitudinal effects of Quizzes on Motivation, Emotion, and Cognition. Springer Fachmedien Wiesbaden.
- Meerschman, H. Verkeyn, J. (2019). Towards a Better Understanding of Service Quality Attributes of a Chatbot. [ Doctoral dissertation, master’s dissertation], Ghent University.
- Melián-González, S., Gutiérrez-Taño, D., & Bulchand-Gidumal, J. (2021). Predicting the intentions to use chatbots for travel and tourism. Current Issues in Tourism, 24, 192–210. https://doi.org/10.1080/13683500.2019.1706457
- Ministerio de Tecnologías de la Información y Comunicaciones-MinTIC. Penetración de abonados de Internet móvil por demanda a nivel nacional. Retrieved August 10, 2022, from https://colombiatic.mintic.gov.co/679/w3-propertyvalue-47272.html
- Misischia, C. V., Poecze, F., & Strauss, C. (2022). Chatbots in customer service: Their relevance and impact on service quality. Procedia computer science, 201, 421–428. https://doi.org/10.1016/j.procs.2022.03.055
- Mokmin, N. A. M., & Ibrahim. (N.A). The evaluation of chatbot as a tool for health literacy education among undergraduate students. Education and Information Technologies, 2021(26), 6033–6049. https://doi.org/10.1007/s10639-021-10542-y
- Mweshi, G. K., & Sakyi, K. (2020). Application of sampling methods for the research design. Archives of Business Review, 8(11), 180–193. https://doi.org/10.14738/abr.811.9042
- Naimi-Sadigh, A., Asgari, T., & Rabiei, M. (2022). Digital transformation in the value chain disruption of banking services. Journal of the Knowledge Economy, 13(2), 1212–1242. https://doi.org/10.1007/s13132-021-00759-0
- Nain, S. (2018). A Medical ChatBot. International Journal of Computer Trends and Technology, 60(1), 41–45. https://doi.org/10.14445/22312803/IJCTT-V60P106
- Nguyen, D. M., Chiu, Y.-T. H., & Le, H. D. (2021). Determinants of continuance intention towards banks’ chatbot services in Vietnam: A necessity for sustainable development. Sustainability, 13, 7625. https://doi.org/10.3390/su13147625
- Nguyen, G. T., & Liaw, S.-Y. (2022). Understanding the factors affecting the small and medium enterprises adoption of cloud computing: A literature review. International Journal of Business, Management and Economics, 3(2), 149–162. https://doi.org/10.47747/ijbme.v3i2.635
- Norabuena Mendoza, C., Huamán Osorio, A., & Ramirez Asis, E. (2021). Modelo de Ecuaciones Estructurales (Con estimación PLS) basado en calidad de servicio y lealtad del Cliente de las Cajas Rurales Peruanas. Ciencias Administrativas, 18(18), 3–14. https://doi.org/10.24215/23143738e081
- Okonkwo, C. W., & Ade Ibijola, A. (2021). Chatbots applications in education: A systematic review. Computers and Education: Artificial Intelligence, 2, 100033. https://doi.org/10.1016/j.caeai.2021.100033
- Pelau, C., Dabija, D.-C., & Ene, I. (2021). What makes an AI Device human-like? The role of interaction quality, Empathy and perceived Psychological anthropomorphic characteristics in the acceptance of artificial intelligence in the service industry. Computers in Human Behavior, 122, 106855. https://doi.org/10.1016/j.chb.2021.106855
- Pillai, R., & Sivathanu, B. (2020). Adoption of AI-based chatbots for hospitality and tourism. International Journal of Contemporary Hospitality Management, 32(10), 3199–3226. https://doi.org/10.1108/IJCHM-04-2020-0259
- Prieto, G., & Delgado, A. R. (2010). Fiabilidad y validez. Pap Psicól, 31(1), 67–74. http://www.cop.es/papeles
- Puschmann, T. B. (2017). Fintech. Business & Information Systems Engineering, 59(1), 69–76. https://doi.org/10.1007/s12599-017-0464-6
- Ramachandran, A. (2019). User adoption of chatbots. SSRN Electronic Journal, 1–14. https://doi.org/10.2139/SSRN.3406997
- Ramírez, P. E., Mariano, A. M., & Salazar, E. A. (2014). Propuesta metodológica para aplicar modelos de ecuaciones estructurales con PLS: El caso del uso de bases de datos científicas en estudiantes universitarios. Revista ADMPG, 7(2), 133–139. https://revistas.uepg.br/index.php/admpg/article/view/14062
- Ringle, C. M., Wende, S., & Becker, J.-M. (2022). SmartPLS 4. SmartPLS GmbH. http://www.smartpls.com
- Rivera, M., Gregory, A., & Cobos, L. (2015). Mobile application for the timeshare industry. Journal of Hospitality & Tourism Technology, 6(3), 242–257. https://doi.org/10.1108/JHTT-01-2015-0002
- Rossmann, A., Zimmermann, A., & Hertweck, D. (2020). The impact of chatbots on customer service performance. In J. Spohrer & C. Leitner (Eds.), Advances in the Human Side of Service Engineering (pp. 237–243). Springer International Publishing: Cham.
- Santos, V. M. R., Lima, T., Medeiros, F. P. A. D., Rodrigues, N., Lira, H. (2021). An exploratory analysis on the perception of teachers regarding the monitoring of interactions and activities in the collaborative tools of virtual learning environments. Proceedings of the 2021 16th Iberian Conference on Information Systems and Technologies (CISTI), Chaves, Portugal (pp. 1–6). IEEE.
- Sekaran, U., & Bougie, R. (2016). Métodos de investigación para los negocios: un enfoque de desarrollo de habilidades. John Wiley & Sons.
- Shamout, M., Ben Abdallah, R., Alshurideh, M., Alzoubi, H., Al Kurdi, B., & Hamadneh, S. (2022). A conceptual model for the adoption of autonomous robots in the supply chain and logistics industry. Uncertain Supply Chain Management, 10(2), 577–592. https://doi.org/10.5267/j.uscm.2021.11.006
- Sheehan, B., Jin, H. S., & Gottlieb, U. (2020). Customer service chatbots: Anthropomorphism and adoption. Journal of Business Research, 115, 14–24. https://doi.org/10.1016/j.jbusres.2020.04.030
- Singh, S., Thakur, H. K. (2020). Survey of various AI chatbots based on technology used. Proceedings of the 2020 8th International Conference on Reliability, Infocom Technologies and Optimization (Trends and Future Directions) (ICRITO), Noida, India (pp. 1074–1079). IEEE.
- Smutny, P., & Schreiberova, P. (2020). Chatbots for learning: A review of educational chatbots for the Facebook Messenger. Computers & Education, 151, 103862. https://doi.org/10.1016/j.compedu.2020.103862
- Streiner, D. L. (2003). Being inconsistent about consistency: When coefficient alpha does and doesn’t matter. Journal of Personality Assessment, 80(3), 217–222. https://doi.org/10.1207/S15327752JPA8003_01
- Sweidan, S. Z., Abu Laban, S. S., Alnaimat, N. A., & Darabkh, K. A. (2021). SIAAA-C: A student interactive assistant android application with chatbot during COVID-19 pandemic. Computer Applications in Engineering Education, 29(6), 1718–1742. https://doi.org/10.1002/cae.22419
- Tlili, A., Shehata, B., Adarkwah, M. A., Bozkurt, A., Hickey, D.T., Huang, R., & Agyemang, B. (2023). What if the devil is my guardian angel: ChatGPT as a case study of using chatbots in education. Smart Learn Environment, 10(15), 1–24. https://doi.org/10.1186/s40561-023-00237-x
- Tobi, H., & Kampen, J. K. (2018). Research design: The methodology for interdisciplinary research framework. Quality and Quantity, 52(3), 1209–1225. https://doi.org/10.1007/s11135-017-0513-8
- Topal, A. D., Eren, C. D., & Geçer, A. K. (2021). Chatbot application in a 5th grade science course. Education and Information Technologies, 26(5), 6241–6265. https://doi.org/10.1007/s10639-021-10627-8
- Wu, E. H. K., Lin, C. H., Ou, Y. Y., Liu, C. Z., Wang, W. K., & Chao, C. Y. (2020). Advantages and constraints of a hybrid model K-12 E-learning assistant chatbot. IEEE Access, 8, 77788–77801. https://doi.org/10.1109/ACCESS.2020.2988252
- Yang, C.-C., Li, C.-L., Yeh, T.-F., & Chang, Y.-C. (2022). Assessing Older Adults’ intentions to use a Smartphone: Using the meta–Unified theory of the acceptance and use of technology. International Journal of Environmental Research and Public Health, 19(9), 5403. https://doi.org/10.3390/ijerph19095403