Abstract
Fungal damage has a devastating impact on soybean quality and end-use. The current visual method for identifying damaged soybean seeds is based on discoloration and is subjective. The objective of this research was to classify healthy and fungal-damaged soybean seeds and discriminate among various types of fungal damage using near-infrared (NIR) spectroscopy. A diode-array NIR spectrometer, which measured reflectance [log(1/R)] from 400 to 1700 nm, was used to obtain spectra from single soybean seeds. Partial least square (PLS) and neural network models were developed to differentiate healthy and fungal-damaged seeds. The highest classification accuracy was more than 99% when the wavelength region of 490–1690 nm was used under a two-class PLS model. Neural network models yielded higher classification accuracy than the PLS models for five-class classification. The average of correct classifications was 93.5% for the calibration sample set and 94.6% for the validation sample set. Classification accuracies of the validation sample set were 100, 99, 84, 94, and 96% corresponding to healthy seeds, Phomopsis, Cercospora kikuchii, soybean mosaic virus (SMV), and downy mildew damaged seeds, respectively.
#Contribution No. 03-163-J from the Kansas Agricultural Experiment Station. Mention of a trademark or proprietary product does not constitute a guarantee or warranty of the product by the U.S. Department of Agriculture and does not imply its approval to the exclusion of other products that also may be suitable.
Introduction
Loss of soybean production to pathogens is a serious problem in the United States. According to a report from North Central Regional Committee on soybean disease, the 3-year average loss due to pathogens (1989–1991) was more than 13% of total production accounting about 6.4×106 metric tons in the north central United States.Citation1 Fungal damage occurs most frequently when warm and wet weather delays the soybean harvest.Citation2 Citation3 More than 40 bacteria, fungi, and viruses can infest soybean seeds. However, Phomopsis, Personospora manshurca Syd (downy mildew), soybean mosaic virus (SMV), Cercospora kikuchii, and Fusarium graminearum are the most economically important; and these fungal pathogens have a devastating effect on both chemical and physical properties of soybean; which limits end-use.Citation4 Mbuvi et al. studied the effect of fungal damage on physical properties of soybean and found that fungal damage reduces seed density by 4%, volume and weight by 13%, and increases breakage susceptibility by 20 times compared with healthy seeds.Citation5 Pathogens such as Phomopsis and C. kikuchii significantly affect oil quality. Research showed that oil produced from such infested soybean seeds has elevated free fatty acid concentration and low refining yield. The oil has darker color, lower oxidative stability, and poor flavors.Citation6 Fungal-damaged soybean seeds may also have higher protein and lower carbohydrate contents compared with healthy soybean seeds.Citation7 Citation8 Fungal-damaged soybeans could contain some mycotoxins produced by some fungi. Aflatoxin, T-2 toxin, diacetoxyscirpenol (DAS), HT2 toxin, low levels of vomitoxin (DON), zearalenone (F2), and fumonisin (B1) were also found in fungal-damaged soybean seeds.Citation3 Fungal damage, therefore, is a critical factor for soybean grading and marketing.
Biological factors such as fungal damage, that affect soybean quality cannot be quantified easily and the criteria for these visual judgments have been defined only recently.Citation9 Symptoms such as discoloration of a seed coat caused by fungal damage are usually used as an indicator of soybean quality and classification of the type of fungi. The maximum limits for damaged soybeans are ≥1, 2, 5, and 10% for US Nos. 1, 2, 3, and 4 designations, respectively.Citation10 The maximum limit on fungal-damaged soybeans is very restricted, especially for US Nos. 1 and 2 soybeans. Fungal damage can be determined by physical properties of soybean seed coats using machine vision. Casady developed a color-image processing system to classify soybean seeds into healthy and symptomatic seeds infested by Phomopsis, Alternaria, and C. kikuchii.Citation11 With this system, the fungal-damaged seeds were correctly determined in 77–91% of the soybean seeds tested. Ahmad et al. used a color classifier for classifying fungal-damaged soybean seeds.Citation12 Their color analysis showed differences between the healthy and fungal-damaged seeds. The average classification accuracy was 88% for the healthy and fungal-damaged seeds. However, the classification accuracies for Phomopsis, Alternaria, F. graminearum, and C. kikuchii were only 45, 30, 62, and 83%, respectively.
Machine vision is the application of electronic imaging to enable the visual inspection of an object or a scene. Image analysis can provide a means of extracting useful information from an image for quality measurement of grains and oil seeds. However, machine vision cannot provide specific information related to chemical composition because machine vision is limited to the visible region of the spectrum. Wang et al. developed classification models to measure damaged soybean seeds using near-infrared (NIR) spectroscopy.Citation13 The classification accuracies were 100, 98, 97, 64, 97 and 83% for sound seeds and those damaged by weather, frost, sprout, heat, and mold, respectively. The results indicated that NIR technology should be considered for the classification of fungal-damaged soybean seeds. The objective of this research was to classify healthy and fungal-damaged soybean seeds and discriminate among various types of fungal damage using NIR spectroscopy.
Materials and Methods
Soybean samples were obtained from GIPSA Federal Grain Inspection Service (FGIS) (Kansas City, MO) and the Department of Agronomy, Kansas State University. Healthy and fungal-damaged seeds were manually classified by trained grain inspectors. Seeds of five categories were used for this study. The categories were healthy seeds (natural yellowish color), Phomopsis damaged seeds (white to gray mycelium and shriveled seed coat), C. kikuchii damaged seeds (violet to dark purple color and discolored areas ranged from scattered specks to entire seed surface), SMV damaged seeds (mottled with black or various shades of brown bleeding from the hilum), and downy mildew damaged seeds (superficial milky white encrustation of mycelium and oospores).
Single soybean seed spectra from 400 to 1700 nm were collected with a diode-array NIR spectrometer (DA7000, Perten Instruments, Springfield, IL). Spectrometer measures reflectance from 400 to 1700 nm using an array of silicon sensors for spectrum region from 400 to 950 nm at 7-nm bandwidth and indium–gallium arsenide sensors for spectrum region from 950 to 1700 nm at 11-nm bandwidth. All data is then interpolated to 5-nm intervals. Diode-array NIR spectrometer collected spectra at a rate of 30 s−1. Single soybean seeds were placed in a black V-shaped trough (12-mm long, 10-mm wide, and 5-mm deep) and illuminated with halogen light via a fiber bundle (8-mm diameter) positioned 13-mm from the top of the trough and oriented 45° from vertical. A 2-mm reflectance probe, oriented vertically 9.5-mm from the top of the trough, carried the reflected energy to a spectrometer. The procedures included collecting a baseline, collecting eight spectra from each seed, and averaging the eight spectra for each seed. A total of 500 healthy seeds and 800 fungal-damaged seeds infested by Phomopsis, C. kikuchii, SMV, or downy mildew (200 each) were measured, for a total of 1300 soybean seeds.
Partial least squares (PLS) (Galactic Industries, Salem, NH) and neural networks (NeuralWare, Inc., Pittsburgh, PA) were used for data analysis and model development. Soybean seeds first were separated equally into calibration and validation samples sets, based on even and odd numbers. Healthy and fungal-damaged seeds were assigned constant values of 1.0 and 2.0 for two-class models, respectively. A seed was considered to be correctly categorized if the predicted value lay on the same side of the midpoint of assigned values. For five-class models, healthy and fungal-damaged seeds infested by Phomopsis, C. kikuchii, SMV, or downy mildew were assigned constant values of 1.0, 2.0, 3.0, 4.0, and 5.0, respectively. For PLS models, the model performance is reported as the cross-validation of each calibration sample set and prediction of validation sample sets. The number of PLS factors used was the minimum required to give the best classification results. For neural network models, the determination of major parameters such as learning cycles, learning rate (η), and momentum (α), were described by Wang et al.Citation13 The network with the highest validation accuracy was recorded as the best model. Visible (490–750 nm), NIR (750–1690 nm), and full wavelength (490–1690 nm) regions were used to develop classification models. Spectral data below 490 nm and above 1690 nm were discarded because of signal noise.
Grams/32 software (Galactic Industries, Salem, NH) was used for determination of soybean seed color. Reflectance spectra from 490 to 750 nm were transferred into L*a*b* color space using Grams/32 (Galactic Industries, Salem, NH) software and were used for soybean seed color determination. In the L*a*b* color space, L* varies from 0 (black) to 100 (perfect white); a* ranges from −100 to 100 and measures green when negative and red when positive; b* varies from −100 to 100 and is a measure of blue when negative and yellow when positive.
Results and Discussion
The full wavelength region of 490–1690 nm gave the highest percentage of correct classification for both cross-validation and prediction (>99%) (Table ). The use of the visible or NIR wavelength region alone resulted in low classification accuracy. When compared to healthy soybean seeds, the energy absorption of fungal-damaged soybean seeds was higher in the visible region and lower in the NIR region (data not shown). The differences in energy absorption indicate differences in color and chemical composition between healthy and fungal-damaged soybean seeds.
Table 1 Classification accuracies (%) of healthy and fungal-damaged soybean seeds using two-class PLS models
Fungal-damaged soybean seeds are usually associated with discoloration and fissures on the seed coats. In addition, fungal damage also results in wrinkled, cracked, and crusty seed coats with less roundness. The degree of discoloration varies with type of fungi. The average spectra of healthy and fungal-damaged seeds showed that the energy absorption values of Phomopsis and downy mildew damaged seeds were lower than the healthy seeds in the visible wavelength region, where as C. kikuchii and SMV damaged seeds had higher energy absorption values than the healthy seeds in the NIR region (Fig. ). Phomopsis and downy mildew damage result in white to gray and milky white color on the seed coat. Cercospora kikuchii and SMV damage results in violet to darker purple and black color on the seed coat. The color differences between the healthy and fungal-damaged seeds were further confirmed by L, a, and b values (Table ). Healthy seeds had higher L values than C. kikuchii and SMV damaged seeds and lower L values than Phomopsis and downy mildew damaged seeds. This response indicates that C. kikuchii and SMV damaged seeds are darker than healthy seeds, and healthy seeds are darker than Phomopsis and downy mildew damaged seeds. Healthy seeds had higher b values than fungal-damaged seeds, indicating healthy seeds are more yellow than fungal-damaged seeds. However, there were no significant differences between healthy seeds and SMV damaged seeds in a value.
Table 2 The color variation between healthy and fungal-damaged soybean seeds measured as L, a, and b values in L*a*b* color space
Figure 1. Average visible and near-infrared absorption curves for healthy soybean seeds, and Phompsis, C. kikuchii, SMV, and downy mildew damaged soybean seeds.
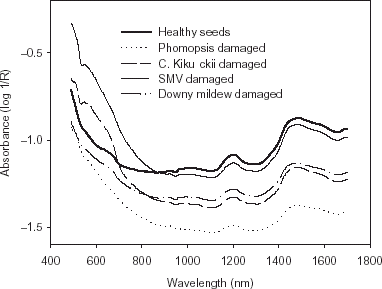
The peaks and valleys of the beta coefficients curve from the PLS model illustrate the significant differences in energy absorption between the healthy and fungal-damaged soybean seeds (Fig. ). The differences in energy absorption in the NIR region indicate differences in chemical composition between healthy and fungal-damaged soybean seeds. The beta coefficient peaks around 565, 650, and 705 nm are related to color differences between healthy and fungal-damaged seeds. The beta coefficient peaks around 970 and 1465 nm are related to NH bonds representing protein content.Citation14 The peaks around 1330 and 1600 nm are related to CH bonds representing fiber and starch contents.Citation15 The fungal-damaged soybean seeds may have higher protein and less carbohydrate contents than healthy soybean seeds.Citation5 Citation7 Citation8 Citation16 Phomopsis seed decay is the most destructive disease of soybean seeds.Citation2 Citation17 Citation18 Severely affected seeds are elongated, heavily cracked or fissured and shriveled with less mass, volume, density, and more surface area compared to healthy seeds. Cercospora kikuchii damage reduces density or mass and causes degradation of seed-coat protein.Citation19
Figure 2. Beta coefficients curve of PLS model (10 PLS factors) for classification of healthy and fungal-damaged soybean seeds.
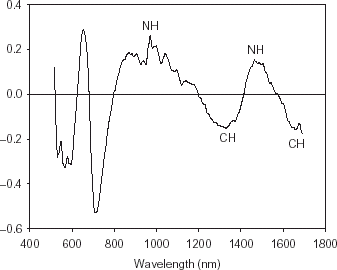
Preliminary results showed that the neural network without a hidden layer yielded higher classification accuracies for both calibration and validation sample sets.Citation20 Citation21 Thus, neural network models without a hidden layer were developed. The optimum neural network parameters, learning cycle of 150,000, learning rate of 0.7, and momentum of 0.5, were used for neural network training. The performance of neural network models is summarized in Table . In general, calibration and validation sample sets had similar classification accuracies. The neural network using the wavelength region of 490–1690 nm yielded the highest classification accuracy. More than 90% of healthy, Phomopsis, SMV, and downy mildew damaged soybean seeds were correctly classified for both calibration and validation sample sets, respectively. Cercospora kikuchii damaged seeds were the most difficult-to-classify. However, 83% and 84% of C. kikuchii damaged soybeans were correctly classified for calibration and validation sample sets, respectively, when the wavelength region of 490–1690 nm was used. About 16% of C. kikuchii damaged soybean seeds were misclassified into healthy and Phomopsis damaged soybean categories, and about 6% of SMV damaged seeds were misclassified into healthy seeds (data not shown).
Table 3 Effect of wavelength region on classification accuracies (%) of healthy and fungal-damaged soybean seeds using five-class neural network models.Footnotea
Conclusion
Healthy and fungal-damaged soybean seeds can be easily classified using PLS models with NIR. Classification accuracy was more than 99% when the wavelength region of 490–1690 nm was used under a two-class model. Five-class neural network models were developed to discriminate among various types of fungal damage. The average of correct classifications was 93.5% for the calibration sample set and 94.6% for the validation sample set. The classification accuracies of the validation sample set were 100, 99, 84, 94, and 96% corresponding to healthy seeds, Phomopsis, C. kikuchii, SMV, and downy mildew damaged seeds, respectively.
Notes
#Contribution No. 03-163-J from the Kansas Agricultural Experiment Station. Mention of a trademark or proprietary product does not constitute a guarantee or warranty of the product by the U.S. Department of Agriculture and does not imply its approval to the exclusion of other products that also may be suitable.
References
- Doupnik , B.J.R. 1993 . Soybean production and disease loss estimates for north central United States from 1989 to 1991 . Plant Dis. , 77 : 1170 – 1171 . [CSA]
- Sinclair , J.B. and Backman , P.A. 1989 . Compendium of Soybean Diseases, , 3rd Ed. pp. 38. St. Paul, , USA : American Phytopathological Society .
- Wilson , R.F. 1995 . Dealing with the problems of fungal damage in soybean and other oilseeds . JAOCS , 72 : 1413 – 1414 . [CSA]
- Sinclair , J.B. 1992 . Discoloration of soybean seeds—an indicator of quality . Plant Dis. , 76 : 1087 – 1091 .
- Mbuvi , S.W. , Litchfield , J.B. and Sinclair , J.B. 1989 . Physical properties of soybean seeds damaged by fungi . T. ASAE , 32 : 2093 – 2096 .
- List , G.R. 1980 . Handbook of Soybean Oil Processing and Utilization Champaign, , USA : American Oil Chemists Society .
- Pathan , M.P. , Sinclair , J.B. and McClary , R.D. 1989 . Effect of Cerocospora kikuchii on soybean seed germination and quality . Plant Dis. , 73 : 720 – 723 . [CSA]
- Wilson , R.F. , Novitzky , W.P and Fenner , G.P. 1995 . Effect of fungal damage on seed composition and quality of soybeans . JAOCS , 72 : 1425 – 1429 . [CSA]
- Sinclair , J.B. 1995 . Reevaluation of grading standards and discounts for fungus-damaged soybean seeds . JAOCS , 72 : 1415 – 1419 . [CSA]
- USDA. 1994 . Grain Inspection Handbook; subpart J—U.S. standards for soybean Washington, D.C., , USA : U.S. Department of Agriculture .
- Casady , W.W. , Paulsen , M.R. , Reid , J.F. and Sinclair , J.B. 1992 . A trainable algorithm for inspection of soybean seed quality . T. ASAE , 35 : 2027 – 2033 . [CSA]
- Ahmad , I.S. , Reid , J.F. and Paulsen , M.R. 1999 . Color classifier for symptomatic soybean seeds using image processing . Plant Dis. , 83 : 320 – 327 . [CSA]
- Wang , D. , Ram , M.S. and Dowell , F.E. 2002 . Classification of damaged soybean seeds using near-infrared spectroscopy . T. ASAE , 45 : 1943 – 1948 .
- Shenk , J.S. , Workman , J.J. and Westerhaus , M.O. 2001 . “ Application of NIR spectroscopy to agricultural products ” . In InHandbook of Near-Infrared Analysis Edited by: Burnes , D.A. and Ciurczak , E.W. 419 – 474 . New York : Marcel Dekker Inc. .
- Barton , F.E. II; and Burdick , D. 1979 . Preliminary study on the analysis of forages with a filter-type near-infrared reflectance spectrometer . J. Agric. Food Chem. , 27 : 1248 – 1252 .
- Paulsen , M.R. 1990 . Using machine vision to inspect oilseeds . INFORM , 1 : 50 – 55 .
- Sinclair , J.B. 1993 . Phomopsis seed decay of soybeans—a prototype for studying seed disease . Plant Dis. , 77 : 329 – 334 .
- Velicheti , P.K. , Kollipara , K.P. , Sinclair , J.B. and Hymowitz , T. 1992 . Selective degradation of protein by Cerocospora kikuchii and Phomopsis longicolla. . Plant Dis. , 76 : 779
- Bochereau , L. , Bourgine , P. and Palagos , B. 1992 . A method for prediction by combining data analysis and neural networks: application to prediction of apple quality using near-infrared spectra . J. Agric. Engng. Res. , 51 : 207 – 216 .
- Dowell , F.E. 1994 . Neural network parameter effects on object classification and wavelength selection . Optical Engng. , 33 : 2728 – 2732 .
- Wang , D. , Dowell , F.E. and Lacey , R.E. 1999 . Single wheat kernel color classification using neural networks . T. ASAE , 42 : 233 – 240 .