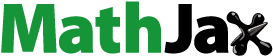
Abstract
Molecular modeling has been employed in the search for lead compounds of chemotherapy to fight cancer. In this study, pharmacophore models have been generated and validated for use in virtual screening protocols for eight known anticancer drug targets, including tyrosine kinase, protein kinase B β, cyclin-dependent kinase, protein farnesyltransferase, human protein kinase, glycogen synthase kinase, and indoleamine 2,3-dioxygenase 1. Pharmacophore models were validated through receiver operating characteristic and Güner–Henry scoring methods, indicating that several of the models generated could be useful for the identification of potential anticancer agents from natural product databases. The validated pharmacophore models were used as three-dimensional search queries for virtual screening of the newly developed AfroCancer database (~400 compounds from African medicinal plants), along with the Naturally Occurring Plant-based Anticancer Compound-Activity-Target dataset (comprising ~1,500 published naturally occurring plant-based compounds from around the world). Additionally, an in silico assessment of toxicity of the two datasets was carried out by the use of 88 toxicity end points predicted by the Lhasa’s expert knowledge-based system (Derek), showing that only an insignificant proportion of the promising anticancer agents would be likely showing high toxicity profiles. A diversity study of the two datasets, carried out using the analysis of principal components from the most important physicochemical properties often used to access drug-likeness of compound datasets, showed that the two datasets do not occupy the same chemical space.
Introduction
In spite of the enormous efforts and progress in the field of cancer research, cancer is the second most common disease-related cause of human mortality, only next to myocardial infarction.Citation1 According to the recent World Health Organization reports, cancer alone is responsible for ~7.6 million deaths (~13% of all deaths) annually,Citation2 and it is estimated that the threat of cancer-related diseases will worsen if no measures are taken.Citation3 Additionally, plant-derived NPs have played a significant role in drug discovery, by being an important source of several clinically useful drugs,Citation4–Citation8 including anticancer agents.Citation9
A cancerous growth is often defined as any malignant tumor. A neoplasm or tumor is an abnormal mass of tissue whose growth exceeds that of normal tissues and whose growth is uncoordinated with that of the latter. Such cancerous growth continues in the same manner after cessation of the stimuli that had initiated it.Citation9,Citation10 The methods of cancer treatment include radiation therapy, surgery, and chemotherapy. However, the first two treatment methods are only successful at the early localized stages of the disease, while chemotherapy is the main treatment method for malignancies because it has the ability to cure widespread cancer even at the later stages. In the search for lead compounds of cancer chemotherapy and prevention, research groups have often resorted to medicinal plants, based on their ethnobotanical uses in the treatment of several cancer-related symptoms and ailments.Citation9,Citation11–Citation25 This is because local and traditional knowledge has been the starting point for many successful drug development projects over the last few decades, as recorded in several literature references.Citation9,Citation10,Citation16–Citation18,Citation26–Citation28 The African flora, for example, is known to contain promising anticancer agents, both based on traditional knowledge and isolated bioactive metabolites that have tested positive against a number of cancer cell lines.Citation29–Citation31 In silico methods of drug discovery programs have proven to be useful in quickly providing lead compounds from enormous databases and also help in lead optimization. This justifies the development of compound libraries, particularly “focused” library scaffolds of compounds that have shown some potency in experimental assays against a particular disease or a drug target. A recent study showed that ~400 compounds from the African medicinal plants, with demonstrated in vitro and/or in vivo anticancer, cytotoxic, and antiproliferative activities, have been identified.Citation32 This dataset (AfroCancer) has been compared with a larger dataset of ~1,500 compounds contained in the Naturally Occurring Plant-based Anticancer Compound-Activity-Target (NPACT) database and with the much larger dataset contained within the Dictionary of Natural Products.Citation32–Citation34 The drug-likeness properties of these plant-derived anticancer datasets have been explored in the previous studies, proving to be interesting starting points for anticancer lead discovery.Citation32,Citation33 It is noteworthy that approximately half of known anticancer drugs are either NPs or NP-related synthetic compounds.Citation35–Citation37 Despite the enormous potential of NPs from the African flora,Citation38–Citation40 little has been done to exploit them into real marketable drugs.Citation41 This calls for efforts from within the continent for laying the groundwork for anticancer drug discovery projects from the floral matter.
The application of in silico (computer-based) modeling in the search for lead compounds is a promising endeavor in drug discovery, since it often accelerates the process and cuts down costs.Citation42 Virtual screening methods are useful because, in principle, they narrow down the number of compounds to be actually tested in biological assays. This is practicable when the in silico scoring methods are sufficiently able to discriminate between active and inactive ones.Citation43,Citation44 The approaches of docking,Citation45–Citation47 quantitative structure-activity relationship,Citation48,Citation49 and pharmacophore searchingCitation47,Citation49–Citation53 have been previously employed with relative success in anticancer lead generation programs. The previous in silico modeling efforts targeting NPs from the African flora have been focused on the building of datasets for virtual screeningCitation30,Citation54–Citation56 and pharmacokinetic profiling of the derived datasets.Citation30,Citation57–Citation59 The aim of the current study was to employ molecular modeling methods to access the toxicity profiles of the aforementioned datasetsCitation32,Citation33 of promising plant-derived anticancer agents and to evaluate the performance of derived pharmacophore models for virtual screening, in a quest to identify new and/or promising anticancer lead compounds from the African flora.
Materials and methods
Data collection and analysis
The dataset for naturally occurring compounds with promising anticancer properties (AfroCancer) has been recently described.Citation32 The compounds of the NPACT database were downloaded from the official webpage (http://crdd.osdd.net/raghava/npact/)Citation34 and prepared for virtual screening as previously described.Citation33
Pharmacophore modeling approach
All pharmacophore modeling was carried out using the LigandScout software (Inte:Ligand, Vienna, Austria).Citation60 All protein–ligand complexes in this study were retrieved from the protein databankCitation61 and prepared as previously described.Citation32 The datasets of active compounds against each drug target were identified from the literature sources cited in the Results section (). Small molecule modeling of the compounds active against each target was carried out using the Molecular Operating Environment (MOE) (Montreal, QC, Canada) softwareCitation62 running on a Linux workstation with a 3.5 GHz Intel Core 2 Duo processor (San Jose, CA, USA). The three-dimensional (3D) structures were generated using the builder module of MOE, and energy minimization was subsequently carried out using the MMFF94 force fieldCitation63 until a gradient of 0.01 kcal/mol was reached. In generating the 3D structures, both R and S forms of stereoisomers were generated in the case of racemic mixtures. Additionally, the ligand databases were given a preliminary treatment using the LigPrep software (Schrödinger LLC, NY, USA).Citation64 Protonation states at biologically relevant pH were correctly assigned (group I metals in simple salts were disconnected, strong acids were deprotonated, strong bases were protonated, and explicit hydrogens were added), and conformers were generated. The generated conformer datasets were further inspected visually, eg, piperazines were mono- or diprotonated taking into account the neighboring groups; piperidines, pyrrolidines, and tertiary aliphatic amines were charged positively always; pyrimidones were also represented as hydroxy pyrimidines; and for ethylenediamines, both neutral and monoprotonated forms were generated. The 3D structures of the compounds and conformers were then saved as individual .mol2 files, subsequently included into a MOE database (.mdb) file and exported to the .ldb file, which is suitable for use in virtual screening workflows using the LigandScout software.Citation60 The performance of a pharmacophore model in virtual screening experiments is often tested by its ability to discriminate between known active compounds and decoys (supposed to be inactive). Such enrichment tests were performed on the set of active compounds (training set) and later used to screen for hits from the AfroCancer and NPACT libraries, using the generated pharmacophore models. In order to avoid artificial enrichment in assessing the performances of the generated pharmacophore models in virtual screening, decoy libraries were generated using the commercially available database Directory of Useful Decoys, Enhanced (DUD-E; http://dude.docking.org),Citation65 ie, 50 decoys per active ligand. Simplified Molecular Input Line Entry System strings of the active compounds were provided for the DUD-E decoy generation tool, and 50 decoys per active ligand were generated based on similar physical properties of active compounds, such as molecular weight (MW), Log P, H-bond donors (HBDs) and H-bond acceptors (HBAs), number of rotatable bonds, and net molecular charge. The motivation behind using decoys with similar one-dimensional physicochemical properties but dissimilar two-dimensional topology is to avoid artificial enrichment in assessing the performances of the generated pharmacophore models in virtual screening.
Table 1 Description of selected human anticancer drug targets used to generate the structure-based pharmacophores in this study
Both libraries of active compounds and decoys were run through or “fitted into” the generated pharmacophore models. The poses were scored (ranked) using the pharmacophore fit score function implemented in LigandScout.Citation60 In general, true positive (TP)/false positive (FP) hit rates, true negative (TN)/false negative (FN) hit rates, enrichment factor (EF), goodness of hits, and the receiver operating characteristic (ROC) curve–area under the ROC curve (AUC) are among the most common quality parameters used in the pharmacophore model evaluation experiments. To assess the performance of each pharmacophore scoring scheme used in this study, some important measures were considered, eg, the percentage yield of active compounds (Ya), percentage actives, and Goodness of a Hit list (Güner–Henry [GH] scoring). The percentage yield of actives was defined as the ratio of actives found in the hit list to the total number of compounds in the hit list, given by the following equation:
In silico toxicity assessment methods
Toxicity prediction was carried out using Lhasa’s expert knowledge-based predictive tool, Derek software Version 3.0.1 (Lhasa Ltd, Leeds, UK),Citation69 running on Nexus 1.5.0 platform. The two datasets (AfroCancer and NPACT) were input in .sdf format. The chosen species was human beings, and 88 toxicity end points were predicted. A full list of the predicted end points has been provided in the Supplementary materials. The toxicity prediction run was carried out for both AfroCancer and NPACT datasets, and the results were analyzed.
Diversity analysis of the AfroCancer and NPACT datasets
Diversity analysis was done by the principal component analysis (PCA) method [68] implemented in the MOE package.Citation62 A number of simple descriptor parameters for the two datasets (AfroCancer and NPACT) were generated using the molecular descriptor calculator included in the QuSAR module of the MOE package.Citation62 The computed descriptors include the MW, number of rotatable single bonds, lipophilicity (log P), solubility (log S), number of HBAs, number of HBDs, total hydrophobic surface area, total polar surface area, number of oxygen, number of chiral centers, number of rings, and number of Lipinski violations. In order to select the optimum number of molecular descriptors for the molecules of both datasets, QuSAR-Contingency (a statistical application in MOE) was employed. The dimensionality of the best selected descriptors, obtained from QuSAR-Contingency, was further reduced by linear transformation of the data by PCA.Citation70 This resulted in a new (smaller) table of descriptors that are uncorrelated and normalized (mean =0 and variance =1). All computed descriptors were employed in the PCA, while the minimum variance was set at 98% for the final set of principal components retained. The 3D plots of the best three principal components (PCA1, PCA2, and PCA3) for both datasets were plotted on the same set of coordinate axes, with PCA1 on the x-axis, PCA2 on the y-axis, and PCA3 on the z-axis, each point on a dataset in the coordinate axes representing a molecule. In order to analyze the diversity of the scaffolds in both datasets, the compounds were fragmented using the retrosynthetic combinatorial analysis procedure algorithm.Citation71 The LibMCS program of JKlustor was used for maximum common substructure clustering of the two datasets.Citation72 The frequency of occurrence of each scaffold in the two datasets was computed and ranked. The most common substructures (most common substructure selection [MCSS]) of the two datasets were then tabulated and compared.
Overall rationale of the modeling methods
Data related to the protein–ligand complexesCitation73–Citation89 used to generate pharmacophore models for virtual screening are listed in . The biological significance of the drug targets for which the pharmacophore models were generated has been discussed in our previous study.Citation32 Pharmacophore-based virtual screening is often an efficient method for the identification of potentially new lead compounds for further development from a virtual database.Citation66–Citation68 Molecules that satisfy the features of the pharmacophore models used as the 3D query are retained as hits. In this study, 3D pharmacophore models were generated and validated, while the best pharmacophore models were used as a 3D search query for retrieving potent molecules from the AfroCancer and NPACT chemical databases, which could be proposed for bioassays. The performance of each pharmacophore model was evaluated using ROC analysis,Citation90 based on their ability to selectively capture diverse active compounds against the chosen targets from a large list of decoys or inactives. The numbers of conformers of actives and decoys for each target and characteristics for GH or “goodness of hit” scoring (eg, Sp, Se, EF, Ya, and % yield of actives) are listed in . In this study, the GH method was used to assess the pharmacophore models generated. This is because this method has been previously used to quantify model selectivity (best model), accuracy of hits, and the recall of actives from a molecule dataset consisting of known actives and inactives with relative success.Citation91–Citation93 The GH score has been applied for quantifying model selectivity and covering the activity space from database mining successfullyCitation47,Citation93–Citation98 and for evaluating the effectiveness of similarity search in databases containing both structural and biological activity data.Citation92 GH scoring contains a coefficient used to penalize excessive hit list size when evaluating hit lists. This coefficient is calibrated by weighting the score with respect to the yield and coverage. The GH scores were computed following EquationEquation 7(7) , and the values are listed in , ranging from 0 to 1, with 0 indicating a null model and 1 a perfect model (containing only actives and no decoys).Citation68 For a good model, the GH value is expected to be >0.7.Citation47 The most promising models have been marked with an asterisk (*) on the respective GH score value. Finally, the ROC curve is a function of Se versus the Sp, and the AUC value is an important method of measuring the performance of the test.
Table 2 Summary of performances of pharmacophore models in virtual screening study
Results and discussion
Assessment of pharmacophore models generated
For the 1IEP target, for example, the best derived pharmacophore model was composed of a combination of an HBA (Acc) feature located on the carbonyl of the native ligand separated from an HBD (Don1) and two hydrophobic centers (Hyd1 and Hyd2), respectively, by 4.97 Å, 4.14 Å, and 3.64 Å (). The second HBD feature (Don2) was located between the two hydrophobic centers, with respective distances of 2.90 Å and 3.80 Å from Hyd1 and Hyd2 (). Scoring of this pharmacophore model using the pharmacophore fit score implemented in LigandScoutCitation58 resulted in the identification of nine out of the 45 generated active conformers in the hit list composed of only 12 hits (hit rate =0.52; ). Even though the hit rate is weak, the Ya, % yield, Se, Sp, EF, and GH scores are, respectively, 75%, 20%, 0.20, 0.99, 3,825, and 0.75 ().
Figure 1 A pharmacophore model used to screen for potential active compounds against the 1IEP target.
Abbreviations: HBDs, H-bond donors; HBAs, H-bond acceptors.
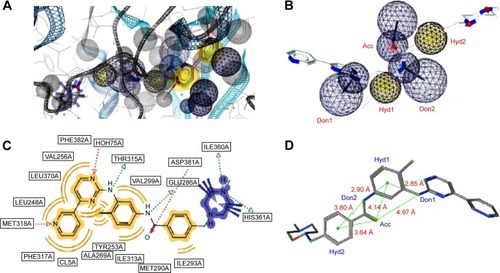
Two separate high-performance pharmacophore models were generated for the 3PE1 target (I and II; ) with the common features comprising the HBD (Don), the acceptor (Acc2), and the hydrophobic center (Hyd2; ). Even though the pharmacophore model 3PE1-I was composed of many more features (as shown in ) than the 3PE1-II (), the first model gave a much higher yield, selectivity, and GH score. Meanwhile, the second model performed better in terms of Ya values because it scored up to 41 out of the 47 compounds in the hit list, even though a total of up to 274 compounds were present in the original list of actives. The performance of this human protein kinase pharmacophore model was also tested for the Protein Data Bank code 3PE2 (with only three features, one corresponding to the common acceptor in models 3PE1-I and 3PE1-II; ). The specificity of this pharmacophore model was comparable with that of the earlier models (3PE1-I and 3PE1-II), while the GH score was midway between that of the 3PE1 models. The performances of these pharmacophores reveal that the 3PE1-I model could be suitable for initially screening a huge dataset, while the more specific model (3PE1-II) could be more suitable in cases of small datasets, which might be the results from a hit list derived from a primary screen of a large dataset using the 3PE1-I model query. The third model (3PE2) is rather less selective, “picking up” too many FPs, thus resulting in a very low Se value (0.04) and rendering this model the least desirable of the three pharmacophores that could be used in screening for human protein kinase inhibitors in this study.
Figure 2 Two sets of combinations of high-performance pharmacophore models for the 3PE1 target.
Abbreviations: HBDs, H-bond donors; HBAs, H-bond acceptors.
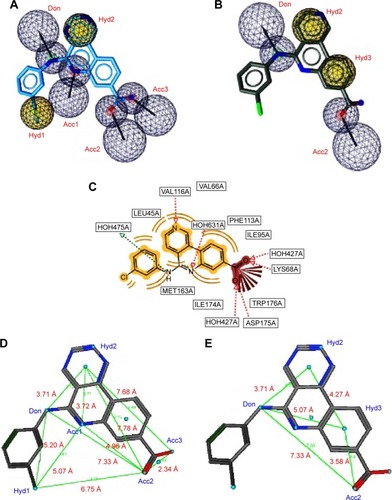
Figure 3 A pharmacophore model used to screen for potential active compounds against the 3PE2 target.
Abbreviations: HBDs, H-bond donors; HBAs, H-bond acceptors; FPs, false positives.
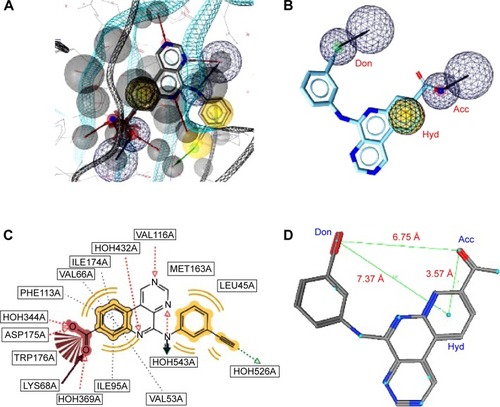
The validated model for the 4ACM target has been shown in , while the remaining models are given in Figures S1–S5. The 4ACM model is composed of two HBAs centered, respectively, on the sulfonyl and pyrazine ring; one HBD on the amino substituent of the pyrazine ring; and a hydrophobic center on the benzene ring. The interactions between the native ligand used to generate this model and the target binding site residues are shown in , while the distances between feature centers are shown in . This model was able to capture up to 23 out of the 42 compounds (% yield of actives =55%) in the original database of actives, with a hit rate of 3.51% from the total database of actives and decoys. Meanwhile, the Se, Sp, and GH values for this model were all ≥0.55. It was generally observed that the best models were 1IEP, 2XMY, 3E37-I, 3PE1-I, 4ACM, and 4BBG-III, while the other models still needed refinement. The 2XMY model (Figure S2), for example, was able to capture seven out of the 14 active compounds in the original database of actives (50% yield of actives), with the strength of the model being the fact that the Se, Sp, and GH scores were all ≥0.50. Meanwhile, the 3E37-I model (Figure S3-I) was able to capture six out of the ten active compounds in the original dataset of actives as the top scoring hits. Our study also involved pharmacophore models for the protein kinase B (PKB) β, Protein Data Bank code 2JDO, which has been recently explored by Vyas et alCitation47 in a virtual screening study, giving a much weaker model than reported in the previous study.
Figure 4 A pharmacophore model used to screen for potential active compounds against the 4ACM target.
Abbreviations: HBDs, H-bond donors; HBAs, H-bond acceptors; GH, Güner–Henry.
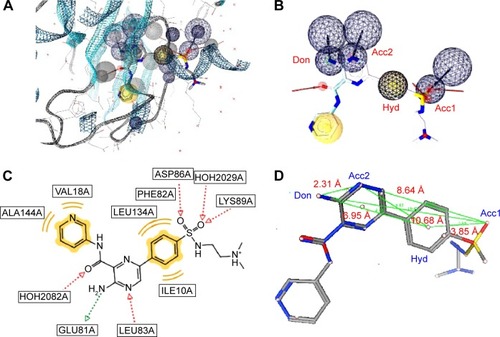
The ROC curves used to evaluate the performances of the generated pharmacophore models are shown in and for three of the “best” models. shows the enrichment curve for the 3PE1-I model. The TP rate has also been plotted against the FP rate and used to compare the two of the 4BBG models (I and III) for the purpose of comparison (). Both 4BBG models show better enrichment than the 3PE1-I model, with the 4BBG-III model () having a higher AUC value than the 4BBG-I model ().
Pharmacophore-based virtual screening of AfroCancer and NPACT datasets
The best derived pharmacophore hypotheses were employed as 3D search queries against the AfroCancer and NPACT databases. Compounds that had their chemical groups spatially overlapping (mapping) with the corresponding features, ie, molecules that satisfied all the features of the pharmacophore model used as the 3D query of the particular pharmacophoric model, were captured as hits and ranked following their pharmacophore fitness score, as implemented in LigandScout.Citation60 A summary of the virtual screening results (number of hits and hit rates) for the AfroCancer and NPACT datasets, using the established and validated pharmacophore models, is given in . It was generally observed that, apart from the null case (model 4BBG-II), the hit rates for the virtual screening of the much smaller AfroCancer dataset were higher than those for the NPACT. Moreover, a plot of the ranks of the retrieved hits by pharmacophore fit scores against the number of compounds screened in the respective databases showed a better performance for the AfroCancer, eg, for the 4ACM pharmacophore model; this curve is shown in .
Table 3 Comparison of virtual screening results (number of compounds in hit list and hit rates) for the AfroCancer and NPACT datasets of the pharmacophore models generated
Figure 7 Enrichment curves obtained by screening the database compounds consisting of the AfroCancer database (blue) and NPACT database (green), using the 4ACM pharmacophore query features.
Abbreviation: NPACT, Naturally Occurring Plant-based Anticancer Compound-Activity-Target.
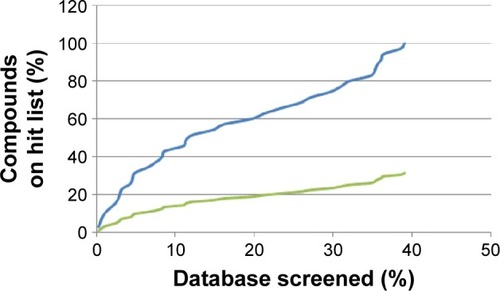
Assessment of the toxicological profiles of the AfroCancer and NPACT datasets
Assessment of the toxicological profiles of the AfroCancer and NPACT datasets was run on the Derek Nexus platform.Citation69 Derek Nexus is an expert, knowledge-based software that gives informative toxicity predictions quickly. The ability to predict potential toxicity of either the parent compound or its metabolites is important in the novel drug design programs.Citation99,Citation100 Computer-based assessment of potential toxicity has become increasingly popular in the past decade.Citation99–Citation102 Thus, early and accurate in silico toxicity prediction using Derek Nexus is an acceptable way of identifying potentially toxic chemicals, by helping experts to reject unsuitable drug candidates.Citation99 The Derek system is able to perceive chemical substructures within molecules and relate these to a rule base, linking the substructures with likely types of toxicity.Citation99–Citation102 It is intended to aid the selection of compounds based on toxicological considerations or separately to indicate specific toxicological properties to be assayed early in the evaluation of a compound, thus saving time, cutting down costs, and saving the lives of some laboratory animals.Citation103,Citation104 This works through the identification of toxicity alerts within the chemical structures of a possible drug candidate. Alerts are collections of structural features observed to result in toxicological activity. Lhasa’s Nexus platform helps automate alert identification by mining descriptions of activating structural features or substructures directly from toxicity datasets, which have been included within the program’s knowledge base.Citation105 Each rule contained in the rule base describes the relationship between a structural feature or toxicophore and its associated toxic effect. Derek possesses the particular ability to report the reasoning behind its predictions.Citation102 The rules are derived by an evaluation of toxicological, mechanistic, and physicochemical data.Citation103 This is achieved by an argumentation-based approach using general toxicological and physicochemical concepts, eg, log P.Citation102,Citation103 In this study, an attempt has been made to identify structural patterns within the AfroCancer and NPACT datasets, which may be related to toxicity of some of the compounds.
Of the 390 compounds within the AfroCancer dataset, 1,330 tautomers were generated. A corresponding 5,357 tautomers were also generated for the NPACT dataset. Predictions were carried out for all the tautomers. In some cases, the output “NOTHING TO REPORT” was recorded. This means that these compounds do not contain any toxicophores that are described in Derek’s current knowledge base. The proportion of tautomers with the NOTHING TO REPORT output was 5.93% for AfroCancer and 4.39% for NPACT. This could also imply that the models in DEREK may not be in the applicability domain of the compounds with the NOTHING TO REPORT output. The remaining compounds showed a number of toxicophores identified with a wide range of toxicity end points. The Derek Nexus prediction includes an overall conclusion about the likelihood of toxicity in a structure and detailed reasoning information for the likelihood (or confidence level). The confidence levels are classified as CERTAIN, PROBABLE, PLAUSIBLE, EQUIVOCAL, and DOUBTED, based on experimental evidence and computed physicochemical parameters. It is important to mention that an outcome “CERTAIN” indicates that the query compounds themselves (or very closely related analogs) have been tested and found to be active. A definition of these confidence levels is provided in Table S1. As an example, chromosome damage in vitro was predicted to be CERTAIN for two compounds from the AfroCancer dataset (). The log P-value of both compounds, imperatorin and bergapten (isolated from the stem of Balsamocitrus paniculata and the related Cameroonian rutaceae species),Citation106,Citation107 was computed to be 4.01, while the computed log Kp was −1.52. The coumarin imperatorin is an antimutagene,Citation108 also known to exert antihypertrophic effect both in vitro and in vivo,Citation109 as well as antimicrobial activities.Citation107 Both imperatorin and bergapten are also known to be components of the apiaceous Bishop’s weed (Ammi majus), growing wild in Egypt and the Mediterranean (a plant used for the treatment of leucoderma and skin diseases).Citation110 The two coumarins activated the Derek alert for psoralen, which causes human chromosome damage in the in vitro chromosome aberration test.Citation111 Experimental evidence suggests that activity in the in vitro chromosome aberration test may be a result of their DNA intercalating properties. The noncovalent binding of psoralen and several derivatives between two base pairs of DNA has been demonstrated.Citation112 This mechanism is also supported by the weak mutagenic activity observed for several psoralen derivatives in the Ames test in Salmonella typhimurium strain TA1537,Citation113 a strain that appears sensitive to other DNA intercalators.Citation114,Citation115
Figure 8 Psoralen substructure responsible for chromosome damage predicted as CERTAIN for two compounds from the AfroCancer dataset.
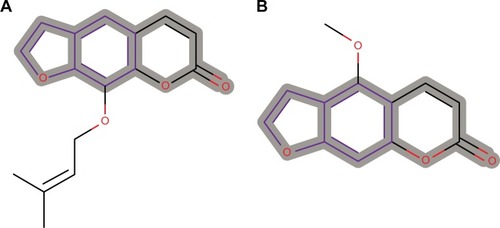
For the NPACT dataset, hepatotoxicity was predicted to be CERTAIN for one tautomer, ocular toxicity for two tautomers, and skin sensitization for six tautomers. The toxicophores responsible for these outcomes will be discussed separately. A total of 22 and 32 end points gave predictions, ranging from CERTAIN to DOUBTED for the AfroCancer and NPACT datasets, respectively (). “DOUBTED” means Derek predicts this compound to be INACTIVE but is not very confident about the conclusion, maybe because of absence of further experimental evidence. A bar chart showing the distribution of the percentage of compounds per likelihood for each end point has been represented in for both AfroCancer and NPACT datasets for chromosome damage in vitro, along with the totals. It was observed that the proportions of tautomers predicted with extreme likelihoods for the various toxicity end points (CERTAIN, PROBABLE, and DOUBTED) were relatively small when compared with the likelihoods PLAUSIBLE and EQUIVOCAL; eg, the bar charts in show that 8.5% and 9.4% of the generated tautomers were predicted as PLAUSIBLE for chromosome damage in vitro (an end point whose data are well distributed among the various aforementioned likelihoods) for the AfroCancer and NPACT datasets, respectively, when compared with equivalent percentages of 0.45% and 0.29% for the likelihoods CERTAIN, PROBABLE, and DOUBTED put together. Regarding the totals (), 93.6% of the generated tautomers were predicted as either PLAUSIBLE or EQUIVOCAL for all likelihoods of the AfroCancer dataset, with an equivalent 80.5% for the NPACT dataset. The Derek program uses the terms “CERTAIN”, “PROBABLE”, and “PLAUSIBLE” to express confidence in predictions based on criteria such that a record in the database reports the query itself to have been tested on the query species and found to be active, a record in the database reports the query itself to have been tested on a species related to the query species (but not on the species itself) and found to be active, and there are no relevant test results in the database but the query triggers at least one alert for activity and it is within the applicability domain for the model. The terms DOUBTED and IMPROBABLE are not weak predictions of activity – they mean that there are clear reasons to predict inactivity. Predictions of PLAUSIBLE or EQUIVOCAL may also imply that either experimental evidence is not strong enough to demonstrate that such toxicity is CERTAIN or that there are both arguments for and against such likelihood. Among the end points computed, the proportion of tautomers with predictions for skin sensitization was most pronounced, corresponding to 47.2% of all predictions for the AfroCancer dataset and 36.9% for the NPACT dataset. Our previous study had shown that the predicted maximum transdermal transport rates, Jm (in μcm−2⋅h−1), computed from the aqueous solubility (Swat) and skin permeability (Kp) and MW using the relation 8:
Table 4 Summary of toxicity predictions for AfroCancer and NPACT datasets
Figure 9 Bar charts showing the distribution of toxicity predictions per likelihood.
Abbreviation: NPACT, Naturally Occurring Plant-based Anticancer Compound-Activity-Target.
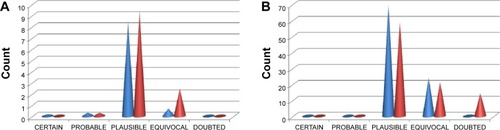
Diversity of the AfroCancer and NPACT datasets
In order to reduce redundancy and enhance the coverage of biological activity and chemical space, a dataset for virtual screening must have the requirement of diversity. PCA was used as a means of comparing the extent of diversity of the two datasets. This consists of reducing the dimensionality of the calculated descriptors by linearly transforming the data, by calculating a new and smaller set of descriptors, which are uncorrelated and normalized. The PCA scatter plot of the previously calculated physicochemical properties of the AfroCancer (red) and NPACT dataset (blue), shown in , is a visual representation of the molecules in the respective datasets, as described by the three best selected principal components (PCA1, PCA2, and PCA3). Each point shown corresponds to a molecule, with the spread of the points representing the diversity of the respective datasets. In this study, a comparison was carried out for the diversity of the two datasets, with the intention of examining the chemical spaces of the two datasets. From the 3D scatter plot of the three best principal components, obtained from the uncorrelated normalized descriptors (), the regions close to the center of the cube contain some regions of intersection of chemical spaces of the two datasets. The larger number of outliers in the case of the NPACT dataset (away for the center and toward the left side of the cube) indicates a wider sampling of the chemical space, when compared with the AfroCancer collection. However, it stands out clear that the right side of the cube is dominated by components from the AfroCancer dataset, while the left side is dominated by components from the NPACT dataset. Overall, the NPACT occupies a wider region of chemical space than the AfroCancer. The first three PCAs could explain 89% (AfroCancer) and 87% (NPACT) of variance of the individual datasets. The MCSS panel for compound selection in the two datasets () is based on the substructures that can be synthetically combined and are common in “drug-like” molecules, allowing a direct selection and identification of compounds containing such substructures. The panel highlights the large diversity of the rings present in the NPs of both datasets, but a broad diversity is clearly seen in the MCSSs, apart from ethers, olefins, and carbonyl-bonded aromatic esters, featuring among the most common fragments obtained in the two datasets.
Figure 10 A PCA plot showing the comparison of the chemical space defined by the NPs in the AfroCancer (red) datasets and the chemical space represented by NPs in the NPACT (cyan) datasets, with the first three principal components projected, respectively, in the x, y, and z directions of space.
Abbreviations: PCA, principal component analysis; NPs, natural products; Var, variance.
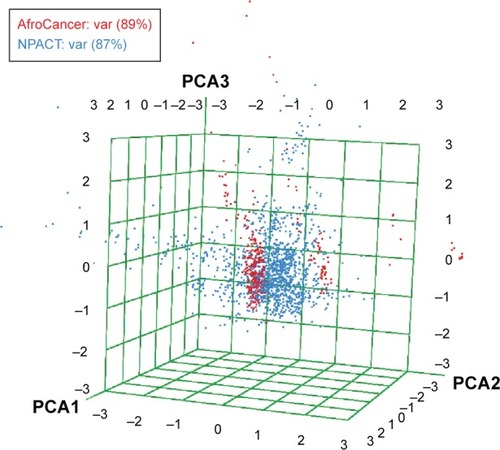
Conclusion
Based on this study, three main objectives have been attained: pharmacophore models for virtual screening aimed at the identification of naturally occurring anticancer agents have been generated and validated, the toxicity of the components of two datasets containing naturally occurring potential anticancer agents has been assessed in computer-based methods, and a diversity analysis of the two datasets has been carried out. This sets the stage for further identification and development of high affinity compounds with potential activities against eight known anticancer drug targets, including tyrosine kinase, PKB β, cyclin-dependent kinase, protein farnesyltransferase, human protein kinase, glycogen synthase kinase, and indoleamine 2,3-dioxygenase 1. The advantage of this work is that both datasets evaluated are relatively small and easy to screen virtually, even using a laptop personal computer. After virtual screening runs using the established pharmacophore-based models, a significant numbers of virtual “hits” have been identified from the datasets of both compounds. These could be further assayed using classical “wet lab” experiments. Moreover, predictions from Derek show that only a few of the compounds in both datasets could be potentially toxic to an extent that might make them unsuitable for investigation as potential cancer therapy. Diversity analysis using the three most important principal components of the computed physicochemical properties of the compounds in the two datasets shows that the databases do not occupy the same chemical space. The two datasets therefore represent reasonable starting virtual libraries for the identification of naturally occurring plant-based anticancer lead compounds for drug discovery.
Acknowledgments
Financial assistance is acknowledged from Lhasa Limited, Leeds, UK. Computational resources were made available through the Molecular Simulations Laboratory, Department of Chemistry, University of Buea, Cameroon. InteLigand Inc. is acknowledged for the academic license to use LigandScout. FN-K currently holds a Georg Forster fellowship from the Alexander von Humboldt Foundation, Germany, while CVS is currently a PhD student funded by the German Academic Exchange Services (DAAD).
Disclosure
The authors report no conflicts of interest in this work.
References
- GibbsJBMechanism-based target identification and drug discovery in cancer researchScience200028754601969197310720316
- WHO Media Centre [webpage on the Internet]Fast sheet Nº 2972013 Available from: http://www.who.int/mediacentre/factsheets/fs297/en/index.htmlAccessed February 4, 2014
- [webpage on the Internet]Globocan Fast Stats2008 Available from: http://www.iarc.fr/en/media-centre/iarcnews/2010/globocan2008.phpAccessed August 4, 2013
- NewmanDJCraggGMSnaderKMThe influence of natural products upon drug discoveryNat Prod Rep200017321523410888010
- HostettmannKTerreauxCSearch for new lead compounds from higher plantsChimia20005411652657
- SaklaniAKuttySKPlant-derived compounds in clinical trialsDrug Discov Today200813316117118275914
- SalimAAChinYWKinghornADDrug discovery from plantsRamawatKGMerillonJMBioactive Molecules and Medicinal PlantsHeidelbergSpringer2008124
- KinghornADPanLFletcherJNChaiHThe relevance of higher plants in lead compound discovery programsJ Nat Prod20117461539155521650152
- GrothausPGCraggGMNewmanDJPlant natural products in anticancer drug discoveryCurr Org Chem2010141617811791
- AshutoshKMedicinal ChemistryNew Delhi, IndiaNew Age International Ltd2007794
- DesaiAGQaziGNGanjuRKMedicinal plants and cancer chemopreventionCurr Drug Metab20089758159118781909
- HeinrichMBremnerPEthnobotany and ethnopharmacy – their role for anti-cancer drug developmentCurr Drug Targets20067323924516515525
- CraggGMNewmanDJNature: a vital source of leads for anticancer drug developmentPhytochem Rev200982313331
- HollósyFKériGPlant-derived protein tyrosine kinase inhibitors as anticancer agentsCurr Med Chem Anticancer Agents20044217319715032721
- ClardyJWalshCLessons from natural moleculesNature2004432701982983715602548
- ReddyLOdhavBBhoolaKDNatural products for cancer prevention: a global perspectivePharmacol Ther200399111312804695
- LucasDMStillPCPérezLBGreverMRKinghornADPotential of plant-derived natural products in the treatment of leukemia and lymphomaCurr Drug Targets201011781282220370646
- WangHKLeeKHPlant-derived anticancer agents and their analogs currently in clinical use or in clinical trialsBot Bull Acad Sinica199738225235
- PanLChaiHKinghornADThe continuing search for antitumor agents from higher plantsPhytochem Lett2010311820228943
- CraggGMNewmanDJPlants as a source of anti-cancer agentsJ Ethnopharmacol20051001727916009521
- BalunasMJKinghornADDrug discovery from medicinal plantsLife Sci200578543144116198377
- SrivastavaVNegiASKumarJKGuptaMMKhanujaSPPlant-based anticancer molecules: a chemical and biological profile of some important leadsBioorg Med Chem200513215892590816129603
- CraggGMGrothausPGNewmanDJImpact of natural products on developing new anti-cancer agentsChem Rev200910973012304319422222
- Gurib-FakimAMedicinal plants: traditions of yesterday and drugs of tomorrowMol Aspects Med200627119316105678
- NobiliSLippiDWitortENatural compounds for cancer treatment and preventionPharmacol Res200959636537819429468
- LeeKHAnticancer drug design based on plant-derived natural productsJ Biomed Sci19996423625010420081
- GordalizaMNatural products as leads to anticancer drugsClin Transl Oncol200791276777618158980
- ConnorsTAnticancer drug development: the way forwardOncologist19961318018110387985
- FadeyiSAFadeyiOOAdejumoAAOkoroCMylesELIn vitro anticancer screening of 24 locally used Nigerian medicinal plantsBMC Complement Altern Med20131317923565862
- Ntie-KangFZofouDBabiakaSBAfroDb: a select highly potent and diverse natural product library from African medicinal plantsPLoS One2013810e7808524205103
- SawadogoWRSchumacherMTeitenMHDicatoMDiederichMTraditional West African pharmacopeia, plants and derived compounds for cancer therapyBiochem Pharmacol201284101225124022846603
- Ntie-KangFNwodoJNIbezimAMolecular modeling of potential anticancer agents from African medicinal plantsJ Chem Inf Model20145492433245025116740
- Ntie-KangFLifongoLLJudsonPNSipplWEfangeSMNHow “drug-like” are naturally occurring anti-cancer compounds?J Mol Model2014201113
- MangalMSagarPSinghHRaghavaGPAgarwalSMNPACT: naturally occurring plant-based anti-cancer compound-activity-target databaseNucleic Acids Res201341D1D1124D112923203877
- CraggGMNewmanDJPlants as a source of anti-cancer and anti-HIV agentsAnn Appl Biol20031432127133
- NewmanDJCraggGMNatural products as sources of new drugs over the 30 years from 1981 to 2010J Nat Prod201275331133522316239
- NewmanDJCraggGMNatural products as sources of new drugs over the last 25 yearsJ Nat Prod200770346147717309302
- HostettmannKMarstonANdjokoKWolfenderJLThe potential of African plants as a source of drugsCurr Org Chem20004109731010
- SandbergFPerera-IvarssonPEl-SeediHRA Swedish collection of medicinal plants from CameroonJ Ethnopharmacol2005102333634316098698
- El-SeediHRBurmanRMansourAThe traditional medical uses and cytotoxic activities of sixty-one Egyptian plants: discovery of an active cardiac glycoside from Urginea maritimaJ Ethnopharmacol2013145374675723228916
- EfangeSMNNatural products: a continuing source of inspiration for the medicinal chemistIwuMMWoottonJCEthnomedicine and Drug Discovery; Advances in Phytomedicine1Amsterdam, NetherlandsElsevier Science20026169
- DiMasiJAHansenRWGrabowskiHGThe price of innovation: new estimates of drug development costsJ Health Econ200322215118512606142
- KlebeGVirtual ligand screening strategies; perspective and limitationsDrug Discov Today2006111358059416793526
- KubinyiHStructure-based design of enzyme inhibitors and receptor ligandsCurr Opin Drug Discov Devel199811415
- ChenHYaoKNadasJPrediction of molecular targets of cancer preventing flavonoid compounds using computational methodsPLoS One201275e3826122693608
- PaulJGnanamRJayadeepaRMArulLAnti cancer activity on Graviola, an exciting medicinal plant extract vs various cancer cell lines and a detailed computational study on its potent anti-cancerous leadsCurr Top Med Chem201313141666167323889049
- VyasVKGhateMGoelAPharmacophore modeling, virtual screening, docking and in silico ADMET analysis of protein Kinase B (PKB β) inhibitorsJ Mol Graph Model201342172523507201
- FanYShiLMKohnKWPommierYWeinsteinJNQuantitative structure-antitumor activity relationships of camptothecin analogues: cluster analysis and genetic algorithm-based studiesJ Med Chem200144203254326311563924
- ZhouHWuSZhaiSDesign, synthesis, cytoselective toxicity, structure-activity relationships, and pharmacophore of thiazolidinone derivatives targeting drug-resistant lung cancer cellsJ Med Chem20085151242125118257542
- ChiangYKKuoCCWuYSGeneration of ligand-based pharmacophore model and virtual screening for identification of novel tubulin inhibitors with potent anticancer activityJ Med Chem200952144221423319507860
- KimDYKimKHKimNDDesign and biological evaluation of novel tubulin inhibitors as antimitotic agents using a pharmacophore binding model with tubulinJ Med Chem200649195664567016970393
- OjimaIChakravartySInoueTA common pharmacophore for cytotoxic natural products that stabilize microtubulesProc Natl Acad Sci U S A19999684256426110200249
- Pérez-SacauEDíaz-PenãteRGEstévez-BraunASynthesis and pharmacophore modeling of naphthoquinone derivatives with cytotoxic activity in human promyelocytic leukemia HL-60 cell lineJ Med Chem200750469670617249647
- Ntie-KangFMbahJAMbazeLMCamMedNP: building the Cameroonian 3D structural natural products database for virtual screeningBMC Complement Altern Med2013138823590173
- Ntie-KangFOnguénéPAScharfeMConMedNP: a natural product library from medicinal plants in Central AfricaRSC Adv20144409419
- Ntie-KangFAmoa OnguénéPFotsoGWVirtualizing the p-ANAPL library: a step towards drug discovery from African medicinal plantsPLoS One201493e9065524599120
- Ntie-KangFLifongoLLMbahJAIn silico drug metabolism and pharmacokinetic profiles of natural products from medicinal plants in the Congo BasinIn Silico Pharmacol201311225505657
- Ntie-KangFMbahJALifongoLLAssessing the pharmacokinetic profile of the CamMedNP natural products database: an in silico approachOrg Med Chem Lett2013311024229455
- OnguénéPANtie-KangFMbahJAThe potential of anti-malarial compounds derived from African medicinal plants, part III: an in silico evaluation of drug metabolism and pharmacokinetics profilingOrg Med Chem Lett201441626548985
- WolberGLangerTLigandScout: 3D-pharmacophores derived from protein-bound ligands and their use as virtual screening filtersJ Chem Inf Model200545116016915667141
- BermanHMWestbrookJFengZThe protein data bankNucleic Acids Res200028123524210592235
- Chemical Computing Group IncMolecular Operating Environment, Version 2010MontrealChemical Computing Group Inc2010
- HalgrenTAMerck molecular forcefieldJ Comput Chem199617490441
- SchrödingerLLCLigPrep Software, Version 2.5New YorkSchrödinger LLC2011
- MysingerMMCarchiaMIrwinJJShoichetBKDirectory of useful decoys, enhanced (DUD-E): better ligands and decoys for better benchmarkingJ Med Chem201255146582659422716043
- GünerOFHenryDRPharmacophore perception, development, and use in drug designGünerOFMetric for Analyzing Hit Lists and Pharmacophores; IUL Biotechnology SeriesLa Jolla, CAInternational University Line2000191212
- GünerOFWaldmanMHoffmannRDKimJHPharmacophore perception, development, and use in drug designGünerOFStrategies for Database Mining and Pharmacophore DevelopmentIUL Biotechnology Series1st EditionLa Jolla, CAInternational University Line2000213236
- LangerTHoffmannRDPharmacophores and Pharmacophore SearchesWeinheim, GermanyWILEY-VCH2006338340
- Lhasa Limited [webpage on the Internet]Derek Nexus Available from: http://www.lhasalimited.org/products/derek-nexus.htmAccessed May 19, 2015
- WoldSEsbensenKGeladiPPrincipal component analysisChemometr Intell Lab Syst198723752
- LewellXQJuddDBWatsonSPHannMMRECAP – retrosynthetic combinatorial analysis procedure: a powerful new technique for identifying privileged molecular fragments with useful applications in combinatorial chemistryJ Chem Inf Comput Sci19983835115229611787
- ChemAxon [webpage on the Internet]JChem Software, Version 5.11.32012 Available from: https://www.chemaxon.com/jchem/doc/user/LibMCS.htmlAccessed May 19, 2015
- NagarBBornmannWGPellicenaPCrystal structures of the kinase domain of c-Abl in complex with the small molecule inhibitors PD173955 and imatinib (STI-571)Cancer Res200262154236424312154025
- WisniewskiDLambekCLLiuCCharacterization of potent inhibitors of the Bcr-Abl and the c-Kit receptor tyrosine kinasesCancer Res200262154244425512154026
- ZimmermannJBuchdungerEMettHMeyerTLydonNBPotent and selective inhibitors of the Abl-kinase: phenylaminopyrimidine (Pap) derivativesBioorg Med Chem Lett19973297187192
- KrakerAJHartlBGAmarAMBarvianMRShowalterHDMooreCWBiochemical and cellular effects of c-Src kinase-selective pyrido[2, 3-d]pyrimidine tyrosine kinase inhibitorsBiochem Pharmacol200060788589810974196
- McHardyTCaldwellJJCheungKMDiscovery of 4-amino-1-(7H-pyrrolo[2,3-d]pyrimidin-4-yl)piperidine-4-carboxamides as selective, orally active inhibitors of protein kinase B (Akt)J Med Chem20105352239224920151677
- CaldwellJJDaviesTGDonaldAIdentification of 4-(4-aminopiperidin-1-yl)-7H-pyrrolo[2,3-d]pyrimidines as selective inhibitors of protein kinase B through fragment elaborationJ Med Chem20085172147215718345609
- DonaldAMcHardyTRowlandsMGRapid evolution of 6-phenylpurine inhibitors of protein kinase B through structure-based designJ Med Chem200750102289229217451235
- WangSGriffithsGMidgleyCADiscovery and characterization of 2-anilino-4-(thiazol-5-yl)pyrimidine transcriptional CDK inhibitors as anticancer agentsChem Biol201017101111112121035734
- HastMAFletcherSCummingsCGStructural basis for binding and selectivity of antimalarial and anticancer ethylenediamine inhibitors to protein farnesyltransferaseChem Biol200916218119219246009
- BattistuttaRCozzaGPierreFUnprecedented selectivity and structural determinants of a new class of protein kinase ck2 inhibitors in clinical trials for the treatment of cancerBiochemistry201150398478848821870818
- PierreFStefanENédellecAS7-(4H-1,2,4-triazol-3-yl) benzo[c][2,6]naphthyridines: a novel class of Pim kinase inhibitors with potent cell antiproliferative activityBioorg Med Chem Lett201121226687669221982499
- HouZNakanishiIKinoshitaTStructure-based design of novel potent protein kinase CK2 (CK2) inhibitors with phenyl-azole scaffoldsJ Med Chem20125562899290322339433
- PierreFReganCFChevrelMCNovel potent dual inhibitors of CK2 and Pim kinases with antiproliferative activity against cancer cellsBioorg Med Chem Lett20122293327333122460033
- BergSBerghMHellbergSDiscovery of novel potent and highly selective glycogen synthase kinase-3β (GSK3β) inhibitors for Alzheimer’s disease: design, synthesis, and characterization of pyrazinesJ Med Chem201255219107911922489897
- CoghlanMPCulbertAACrossDASelective small molecule inhibitors of glycogen synthase kinase-3 modulate glycogen metabolism and gene transcriptionChem Biol200071079380311033082
- GoodJAWangFRathOOptimized S-trityl-L-cysteine-based inhibitors of kinesin spindle protein with potent in vivo antitumor activity in lung cancer xenograft modelsJ Med Chem20135651878189323394180
- TojoSKohnoTTanakaTCrystal structures and structure-activity relationships of imidazothiazole derivatives as IDO1 inhibitorsACS Med Chem Lett20145101119112325313323
- FawcettTAn introduction to ROC analysisPattern Recognit Lett2006278861874
- YangJMShenTWA Pharmacophore-based evolutionary approach for screening selective estrogen receptor modulatorsProteins200559220522015726586
- MueggeISelection criteria for drug-like compoundsMed Res Rev200323330232112647312
- Ntie-KangFKannanSWichapongKOwono OwonoLCSipplWMegnassanEBinding of pyrazole-based inhibitors to Mycobacterium tuberculosis pantothenate synthetase: docking and MM-GB(PB)SA analysisMol Biosyst201410222323924240974
- LiaoHSLiuHLChenWHHoYStructure-based pharmacophore modeling and virtual screening to identify novel inhibitors for anthrax lethal factorMed Chem Res201423837253732
- KoideYUemotoKHasegawaTPharmacophore-based design of sphingosine 1-phosphate-3 receptor antagonists that include a 3,4-dialkoxybenzophenone scaffoldJ Med Chem200750344245417266196
- LuSHWuJWLiuHLThe discovery of potential acetylcholinesterase inhibitors: a combination of pharmacophore modeling, virtual screening, and molecular docking studiesJ Biomed Sci201118821251245
- SealAYogeeswariPSriramDOSDD ConsortiumWildDJEnhanced ranking of PknB inhibitors using data fusion methodsJ Cheminform201351223317154
- KalvaSAzhagiya SingamERRajapandianVSaleenaLMSubramanianVDiscovery of potent inhibitor for matrix metalloproteinase-9 by pharmacophore based modeling and dynamics simulation studiesJ Mol Graph Model201449253724473069
- SandersonDMEarnshawCGComputer prediction of possible toxic action from chemical structure; the DEREK systemHum Exp Toxicol19911042612731679649
- GreeneNJudsonPNLangowskiJJMarchantCAKnowledge-based expert systems for toxicity and metabolism prediction: DEREK, StAR and METEORSAR QSAR Environ Res1999102–329931410491855
- MarchantCABriggsKALongAIn silico tools for sharing data and knowledge on toxicity and metabolism: Derek for Windows, Meteor, and ViticToxicol Mech Methods2008182–317718720020913
- RidingsJEBarrattMDCaryRComputer prediction of possible toxic action from chemical structure: an update on the DEREK systemToxicology19961061–32672798571398
- GreeneNComputer software for risk assessmentJ Chem Inf Comput Sci1997371148150
- MarchantCAComputational toxicology: a tool for all industriesWiley Interdiscip Rev Comput Mol Sci201223424434
- SherhodRGilletVJJudsonPNVesseyJDAutomating knowledge discovery for toxicity prediction using jumping emerging pattern miningJ Chem Inf Model201252113074308723092382
- HappiENWaffoAFWansiJDNgadjuiBTSewaldNO-prenylated acridone alkaloids from the stems of Balsamocitrus paniculata (Rutaceae)Planta Med201177993493821243586
- TsassiVBHussainHMeffoBYAntimicrobial coumarins from the stem bark of Afraegle paniculataNat Prod Commun20105455956120433072
- WallMEWaniMCHughesTJTaylorHPlant antimutagenic agents, 1. General bioassay and isolation procedureJ Nat Prod19885158668733060564
- ZhangYCaoYZhanYDuanHHeLFuranocoumarins-imperatorin inhibits myocardial hypertrophy both in vitro and in vivoFitoterapia20108181188119520691250
- KhalidHAbdallaWEAbdelgadirHOpatzTEfferthTGems from traditional North-African medicine: medicinal and aromatic plants from SudanNat Prod Bioprospect20122392103
- National Toxicology Program (NTP)Toxicology and carcinogenesis studies of 8-methoxypsoralen (CAS No 298-81-7) in F344/N rats (gavage studies)National Toxicology Program Report, Additional Content: TR-359Chapel Hill, USA1989
- Dall’AcquaFTerbojevichMMarcianiSVedaldiDRecherMInvestigation of the dark interaction between furocoumarins and DNAChem Biol Interact1978211103115566637
- QuintoIAverbeckDMoustacchiEHrisohoZMoronJFrameshift mutagenicity in Salmonella typhimurium of furocoumarins in the darkMutat Res1984136149546371513
- FergusonLRDennyWAThe genetic toxicology of acridinesMutat Res199125821231601881402
- AlbertiniSBösMGockeEKirchnerSMusterWWichmannJSuppression of mutagenic activity of a series of 5HT2c receptor agonists by the incorporation of a gem-dimethyl group: SAR using the Ames test and a DNA unwinding assayMutagenesis19981343974039717178