Explainable Artificial Intelligence and Machine Learning: Novel Approaches to Face Infectious Diseases Challenges
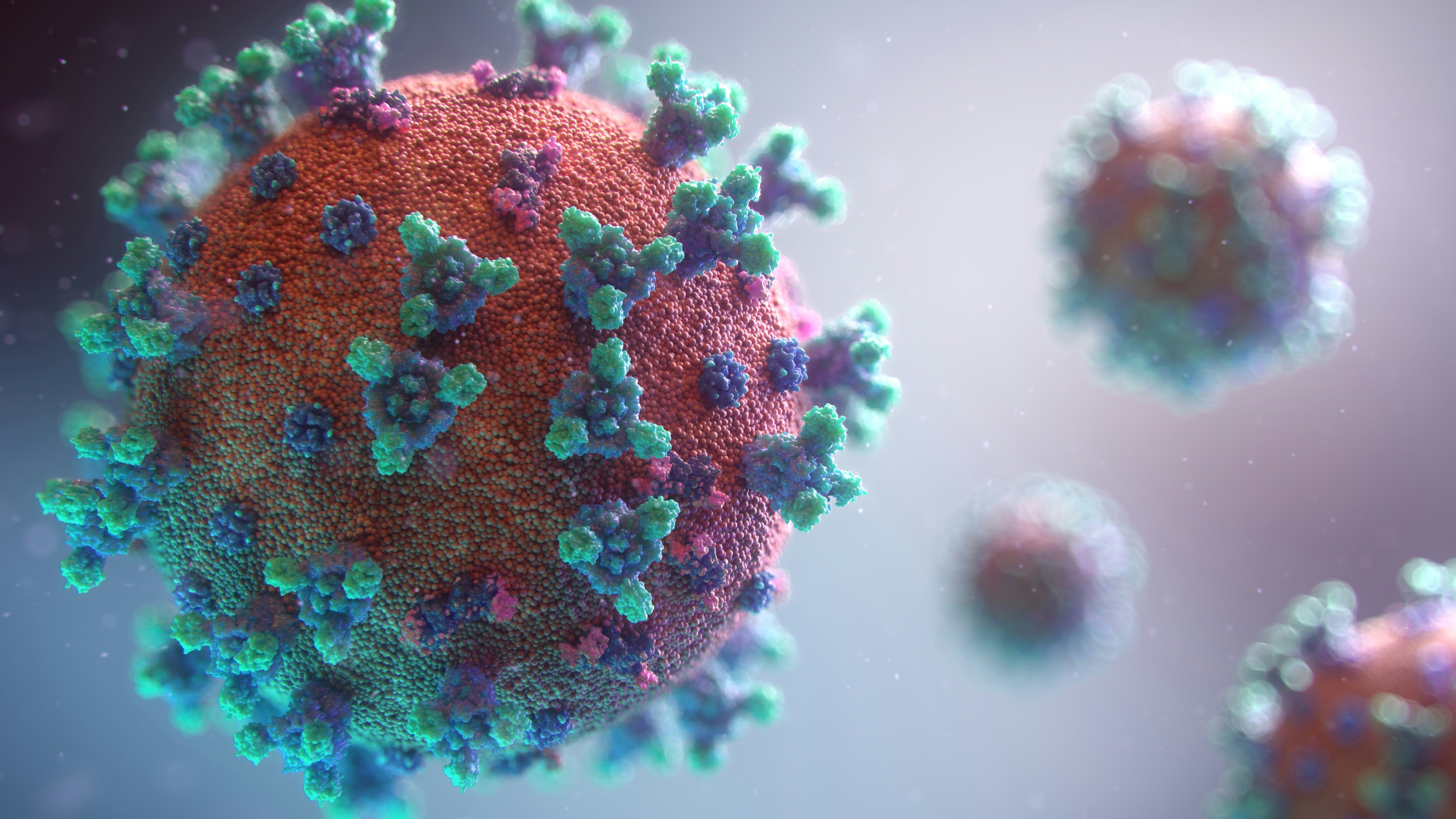
Machine learning is a branch of artificial intelligence (AI) in which computers are conferred the ability to learn from data. Classical statistics and machine learning models are a continuum in which, generally, the fewer the assumptions imposed by humans the more likely it is for machine learning models to capture complex characteristics and to evaluate their association with a given outcome/factor. Nonetheless, human involvement remains crucial for different tasks, such as but not only identifying/reducing biases and preserving interpretability of both models and results.
Machine learning is closely related to the field of “big data”. Therefore, the availability of large datasets is frequently crucial to exploit the potential of machine learning models and their promises to improve patients’ care and interventions. All of this will increasingly require a multidisciplinary approach to guarantee security, reproducibility, standardization, interpretability, and explanation of data and results. In turn, this will add notable complexity, that should comply with continuously updated and evolving ethical requirements. Furthermore, as AI models become increasingly complex and opaque, there is a growing need for explainable AI (XAI) techniques to ensure transparency and interpretability.
The future of infectious diseases is not exempt from the advent of AI and machine learning, which are increasingly employed in clinical research investigating risk, diagnosis, treatment, prevention, and prognosis of viral, bacterial, fungal, and parasitic diseases in humans. This comes with novel challenges and complexity, but also with the potential to improve patients‘ care, provided the employed models are explainable. Indeed, in the context of infectious diseases, where timely and accurate decisions are crucial, it is essential to understand how AI algorithms arrive at their predictions or recommendations. Explainable AI provides insights into the decision-making process, making the outcomes more transparent and interpretable. This transparency helps build trust among healthcare professionals, policymakers, and the general public, fostering the adoption and acceptance of AI-based systems.
Guest advisors
Dr. José de la Fuente(Instituto de Investigacion en Recursos Cinegeticos (IREC, CSIC-UCLM-JCCM), Ciudad Real, Spain)
José de la Fuente is Professor of the Higher Council of Scientific Research (CSIC) and head of the Genomics, Proteomics & Biotechnology group at SaBio, IREC, Spain, and Adjunct Professor at Oklahoma State University. His research focuses on the study of the host-vector-pathogen molecular interactions, and translation of this basic information into development of effective vaccines and other interventions for the control of infectious diseases affecting human and animal health worldwide.
Dr. Daniele Roberto Giacobbe(University of Genoa, Genoa, Italy)
Daniele Roberto Giacobbe, MD, PhD, is assistant professor of infectious diseases at the University of Genoa, Italy. He is also working as an infectious diseases specialist at San Martino Polyclinic Hospital in Genoa and is a member of the directive committee of the Italian Society of Anti-Infective Therapy (SITA). His main fields of research are severe infections due to difficult-to-treat gram-negative bacteria and invasive fungal diseases in the intensive care unit.