ABSTRACT
This study explores benefits of studying two related subjects, mathematics and physics, in teacher education. German teacher students study two subjects as a major, which allows for investigating how the development of content knowledge in one subject, mathematics, benefits students’ content knowledge in the other subject, physics. The sample included teacher students from 12 major teacher education universities in Germany. Each of the N = 104 physics teacher students, whose second subject was mathematics, answered two tests assessing their content knowledge in (1) mathematics and (2) physics. Our results reveal an influence of taking mathematics as a second subject on students’ physics content knowledge. However, the effect size is only small. We found this influence increased over the course of study, indicating that the study of academic mathematics provided increasing opportunities to learn physics over the course of study. Even when controlling for general cognitive abilities, there remains substantial and significant effect of mathematics on physics content knowledge. Mathematical knowledge proves to be relevant in all physical subdomains except for optics and solid-state physics. Overall, the present study confirms benefits of studying physics when also studying mathematics, and thus a synergy effect in studying two subjects in scope of teacher education.
Introduction
Teacher professional competence is widely considered a precondition for effective teaching and learning (Abell Citation2007; Berry, Friedrichsen, and Loughran Citation2015; Kunter et al. Citation2013). Supporting teacher students in their development of such competence is one goal of teacher education. Teacher education commonly consists of an in-service training phase, preceded by a higher education phase (Tatto et al. Citation2012). In-service teacher training aims at enabling teachers to successfully plan, implement and reflect on instruction; higher education teacher training aims at providing teachers with the knowledge bases foundational to the development of professional competence (Tatto et al. Citation2012). These teacher professional knowledge bases include, for example, knowledge about what to teach (i.e. curricular knowledge), how to teach (i.e. pedagogical knowledge), and how to assess students’ knowledge (i.e. assessment knowledge) (e.g. Gess-Newsome Citation2015). The most critical knowledge base, however, is content knowledge. Content knowledge has, for example, been found to determine the quality of teacher explanations (Lachner and Nückles Citation2016) or teachers’ ability to identify student misconceptions (Sadler et al. Citation2013). More specifically, content knowledge has been found critical to the development of pedagogical content knowledge (e.g. Baumert et al. Citation2010; Rollnick Citation2017) that in turn determines quality teaching (e.g. Kunter et al. Citation2013; Kulgemeyer and Riese Citation2018) and thus effective learning (e.g. Keller, Neumann, and Fischer Citation2017; Tchoshanov et al. Citation2017).
Teachers’ content knowledge
Content knowledge (CK) is the knowledge of a teacher of his or her discipline (Kind Citation2014); that is, ‘the academic content of the discipline’ (Gess-Newsome Citation2015, 32). A teachers’ CK is characterized by the scope and quality of the knowledge (Shulman Citation1986). The scope of a teachers’ knowledge is defined by the amount of facts or concepts a teacher knows across different areas of the discipline. The quality of a teachers’ CK can be defined by the teachers’ pervasion of the discipline’s structure; that is, the extent to which a teacher, beyond the accepted facts or concepts, knows how the knowledge in a domain is organized and how it is established (Schwab Citation1978). How deeply a teacher must have pervaded the structure of his or her discipline – that is, how far the quality of a teacher’s CK must go beyond the knowledge of the accepted facts or concepts – is subject to an ongoing debate (e.g. Ball, Thames, and Phelps Citation2008; Dreher, Lindmeier, and Heinze Citation2018; Nixon, Toerien, and Luft Citation2019; Wu Citation2018). Grossman, Schoenfeld, and Lee (Citation2005) suggest, for example, that beyond knowing the content they are supposed to teach, teachers must also have knowledge that enables them to follow current developments in their discipline.
The development of teachers’ content knowledge
Much research has shown that teachers’ CK develops mainly during the higher education phase of teacher training (e.g. Blömeke et al. Citation2008; Kleickmann et al. Citation2013; Krauss, Baumert, and Blum Citation2008). Teacher students’ CK developed in particular in higher education programs that provided teacher students with rigorous and demanding learning opportunities (Kleickmann et al. Citation2013; Schmidt, Cogan, and Houang Citation2011; Tatto and Senk Citation2011). This applies in particular to teacher students studying their subject at the master level. Naturally, studying two subjects at an academic level requires sacrifices in terms of the quantity of learning opportunities (see Neumann et al. Citation2017, for details). The quantity of learning opportunities (i.e. time-on-task) is known to be one of the major predictors for learning (Anderson Citation1981; Wise and Okey Citation1983). As a result, teacher students studying two subjects should progress less in learning CK compared to students studying one subject – unless there is a benefit from studying two subjects. So far, however, there is little research on the potential benefits of studying two subjects on the development of teacher students’ CK. Only one recent study examined the benefits from studying two subjects, namely mathematics and economics. The findings support the conclusion that since academic economics heavily draws on mathematics, studying mathematics as a second subject has a substantial benefit for the development of teacher students’ CK in economics (Jeschke et al. Citation2019).
Academic physics also heavily draws on mathematics, maybe even more so than economics. In fact, mathematics is regarded the language of physics (e.g. Redish and Kuo Citation2015) having an existential function in the modeling of and reasoning about physical phenomena (e.g. Uhden et al. Citation2012). For example, calculus concepts such as differentiation and integration are necessary to understand how kinematics concepts such as velocity and acceleration relate to each other. Likewise, gradient, divergence, and curl (key ideas of vector analysis) are applied in the Maxwell equations (forming the foundation of electromagnetism). The study of mathematics and physics should thus come with a substantial benefit for students’ progression in the development of physics CK.
In this study, we aimed to examine this hypothesis about the relationship between teacher students’ CK of physics and mathematics more closely. In particular, we asked:
(RQ1) How does physics teacher students’ physics CK differ over the course of their studies and their choice of the second subject?
(RQ2) How is mathematics CK correlated with physics CK?
Hypothesis: The correlation of mathematics and physics CK is of at least medium effect size.
(RQ3) What role does mathematics CK play for physics CK in different areas of physics?
Materials and methods
In order to answer our research questions, we utilized data from a large-scale study, the Measuring the Professional Knowledge of pre-service Mathematics and Science Teachers study (German acronym: KiL, Kleickmann et al. Citation2014). The original purpose of this study was to develop assessments for mathematics and science teacher students’ professional knowledge and to measure teacher students’ professional knowledge at different stages of higher education teacher training. We specifically use data on teacher students’ mathematics and physics CK from the KiL study across the five years of higher education teacher training (see Heinze et al. Citation2016; Sorge et al. Citation2019).
Instruments
Development of the instruments to assess mathematics and physics teacher students’ CK in the KiL study followed a rigorous process. First, teacher education policy documents (e. g. KMK, Citation2008) and teacher education programs of major higher education institutions were extensively analyzed. Second, existing instruments were reviewed and items selected accordingly to cover the range of contents typically addressed in teacher education. Where needed, additional items were developed to ensure sufficient coverage. Third, a pilot study was carried out to obtain evidence on the reliability and validity with which the instruments measure mathematics and physics CK (for details see Heinze et al. Citation2016; Sorge et al. Citation2019). Finally, based on the results from the pilot study, items were revised and the final instruments were constructed.
Mathematics content knowledge
The mathematics CK test covered the areas of arithmetic/algebra, analysis, geometry, stochastic, and numeric with a focus on arithmetic/algebra. Based on the pilot study, 54 items were bundled in two different booklets for teacher students’ aiming to teach (a) the academic track and (b) the non-academic track. A total of 41 items exhibited favorable psychometric properties (e. g. measurement invariance across study progress, significant influence of the year of study on total score) and were thus included in the data analysis. Of these 41, 32 items were simple- or complex-multiple-choice, nine items were open-ended. shows a sample open-ended item on arithmetic and algebra.
Physics content knowledge
The physics CK test included 59 items covering mechanics, electrodynamics, optics, thermodynamics, solid state physics, atomic and nuclear physics, special relativity and quantum mechanics. Psychometric analyses confirmed that all items yielded sufficient quality (e.g. measurement invariance across study progress, significant influence of the year of study on total score). 50 of these items were multiple-choice, the remaining open-ended and true-false-items. shows a sample item on mechanics. The item is also an example of how modeling physical phenomena requires mathematics: The problem needs to be translated into a mathematical equation, which is then solved using mathematical operations.
Sample
The present study involves data from N = 200 teacher students (41% female) on the physics CK test and from N = 505 teacher students (62% female) on the mathematics CK test. N1 = 130 students studied both, physics and mathematics, N1’ = 70 studied other science subjects (59%) and other subjects such as language, arts, or music (41%). N2 = 104 students (40% female) provided data on both tests and were, therefore, the prime focus of this investigation (mean age 23.6 years, SD = 2.8 years). shows which samples were employed to approach the research questions. Most students of our sample were in the first three years of their study (). Data was collected in the second half of the academic year at 12 German universities (ranging from 2 to 31 participants per site). The N2 = 104 students came from all 12 universities, with no notable difference to the overall sample.
Table 1. Samples employed to answer the research questions (RQ).
The samples N1 and N1’ did not differ in gender, age, GPA, and distribution of years of enrollment, yet differed in the participation rate for two universities and in the rate of student teachers aspiring to teach at the academic track between both groups (65% for physics/mathematics and 81% for physics/other). Thus, we included the aspired academic track in subsequent analyses for RQ1.
Data analysis
In order to obtain estimates of teacher students’ mathematics CK, we fitted a Rasch model to the data of all 505 mathematics teacher students. Analogously, we fitted a Rasch model to the data of all 200 physics teacher students to obtain estimates on their physics CK. Following this procedure, the sample of interest (i.e. N = 104 physics/mathematics students) were included in both Rasch models. Standard fit indices (e. g. WMNSQ) indicated a good fit of the model to the data (mathematics: 0.89–1.08; physics: 0.88–1.23). Both tests showed good to very good EAP/PV- and WLE-reliabilities (EAP/PV // WLE mathematics: .81 // .79, physics: .85 // .84). Further data analysis thus included WLE as estimates of student teachers’ mathematics and physics CK allowing for using the full sample without missings. Differential item function (DIF) tests for the physics CK instrument revealed a significant difference in item difficulties for N1 and N1’ for 4 items with only a small to medium effect size indicating sufficient measurement comparability between the two groups under investigation in RQ1.
To answer RQ1, we compared the CK of physics teacher students with and without mathematics as their second subject in a regression analysis, including the year of study and aspired track as moderating variables. In order to address RQ2, we determined the correlation between teacher students’ mathematics and physics CK. To account for a possible shared variance due to general cognitive abilities, we controlled for the influence of the Grade Point Average (‘Abitur’; GPA, coded with a range from 1-insufficient to 6-very good) on mathematics and physics CK respectively. For RQ3, we used structural equation modeling (SEM) to determine to which extent different groups of items (parcels by subdomain) are affected by the latent physics ability on the one hand and the mathematics achievement as an exogenous factor on the other (e. g. Beaujean Citation2014). To evaluate the SEM model fit, we used Hu and Bentler’s (Citation1999) recommendation: comparative fit index CFI > 0.95; root-mean-square error of approximation RMSEA < 0.06; standardized root-mean-square residual SRMR < 0.08.
Results
Descriptives
provides descriptive statistics on mathematics and physics CK, GPA, and years of enrollment for the N = 104 students participating in both, the mathematics and the physics test. displays correlations between the respective variables mathematics CK, physics CK, GPA, and years of enrollment.
Table 2. Mean, standard deviation, and range of mathematics CK and physics CK as well as of Grade Point Average (GPA, German “Abitur”), and years of enrollment.
The influence of mathematics as a second subject for physics knowledge (RQ1)
To investigate the role of mathematics as a second subject for student teachers’ development of physics CK, we performed a regression analysis. Results show that mathematics as a second subject, years of enrollment, and the interaction between the years of study and mathematics as a second subject are significant predictors for physics teacher students’ CKFootnote1 (). illustrates this interaction: the influence of mathematics as a second subject on physics CK increases over the course of the study, indicating that additional learning opportunities arise over the course of study (for details see Table 1 in the online supplemental). Note that the interaction effect accounts for the negative main effect of mathematics as a second subject and implies that studying mathematics impedes the development of CK in the early years of study yet promotes physics CK in the later years of enrollment (from about year 3 on) .
Figure 4. Teacher students’ physics CK (with and without mathematics as their second subject) across the years of enrollment. N = 200.
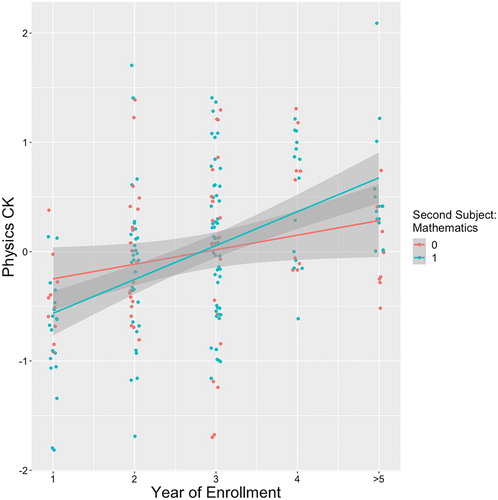
Table 3. Pearson correlation coefficients between mathematics CK, physics CK, GPA, and years of enrollment.
Table 4. Regression analysis of physics teacher students’ CK based on their second subject and their progress in their teacher education program.
Relation between mathematics and physics knowledge (RQ2)
The above results indicated that physics teacher students benefit from studying mathematics as a second subject. We now focus on N2 = 104 teacher students, who participated in the physics and mathematics tests, and investigate how their physics CK is related to their mathematics CK. illustrates the relationship between mathematics and physics CK for this sample. A linear model of participants’ physics CK as a function of their mathematics CK was significant (F(1, 102) = 49.36, p < .001) indicating that teacher students’ mathematics CK explains a significant amount of variance of their physics CK (β = .57). 33% of the variance in physics CK is attributed to mathematics CK.
Figure 5. Physics CK as a function of mathematics CK. Blue line: Linear model fitting the data best. Gray zone: standard error bounds of the regression line. N = 104.
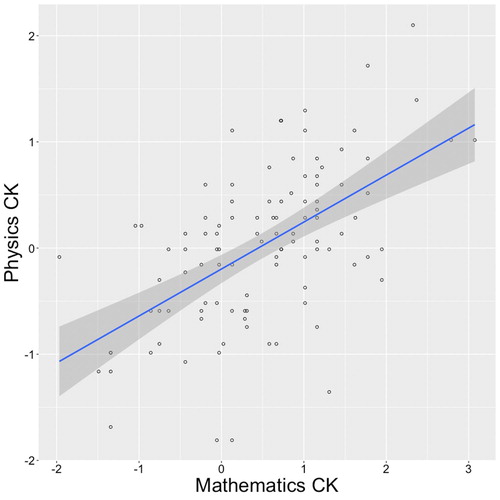
The relation between mathematics and physics CK might also mean that both share a particular amount of general cognitive abilities. To control for such influence, we performed a path analysis () including mathematics and physics CK together with participants’ high school GPA as a proximal measure for their general cognitive abilities (e. g. Sackett et al. Citation2009; Schmitt et al. Citation2009). We found significant effects of GPA on physics (β = .24, SE = .12, p < .01) and mathematics CK (β = .51, SE = .14, p < .001). GPA has an indirect effect on physics CK through mathematics CK of .23 (bias-corrected bootstrap 95% CI [.17,.46]), leading to a total effect from GPA on physics CK of .47. The relation between mathematics and physics CK marginally decreased (β = .45, SE = .07, p < .001) compared to the above linear model, yet it is statistically significant with a moderate effect size. Overall, 37% variance in physics CK was explained by both predictors. Thus, only a small amount of the relation between physics and mathematics CK may be attributed to the confounding of teacher students’ cognitive abilities.
Mathematics CK and individual physics subdomains (RQ3)
To explore which physics subdomains might be more impacted by teacher students’ mathematics CK, we estimated an SEM (based on Maximum Likelihood) including physics CK as a latent factor loading on eight sub-samples of physics items, and mathematics CK influencing the item sub-samples (). High School GPA was used as an additional controlling variable. Again, we focused on the N = 104 physics/mathematics teacher students.
Figure 7. SEM relating mathematics CK to eight physics subdomains compromising latent physics CK (ME: mechanics, EM: classical electromagnetism, OP: optics, TH: thermodynamics, SS: solid state physics, AN: physics of atoms and nuclei, SR: special relativity, QM: quantum mechanics). *** p < .001, ** p < .01, all loadings significant at p < .01, reliability for physics CK at McDonald’s Ω = .82, N = 104.
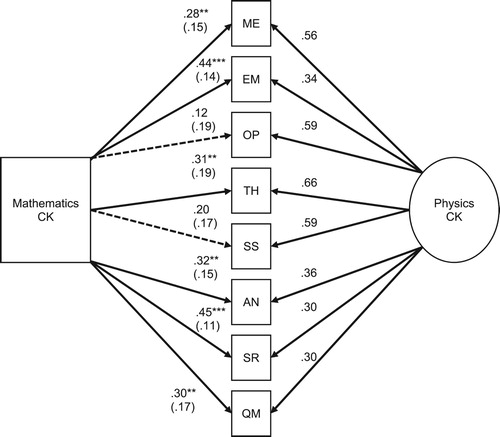
Results show a good overall model fit, χ2 (27) = 35.8, p = .12, RMSEA = .06, SRMR = .05, CFI = .97. We found mathematics CK to significantly impact all physics subdomain parcels except optics and solid-state physics. Given that physical objects in optics and solid-state physics are represented by mathematical models as well (e. g. Snell’s law, cubic crystal systems), the missing significance may also be an artifact due to the actual item design. The strongest influences were found for electromagnetism and special relativity, which typically address (objects in) fields (e. g. electron in an electronic field, relationship between two different observers traveling relative two each other) and thus require quite abstract understanding. Yet, all loadings of the physics subdomain parcels on the latent physics CK factor are significant in this model, even for subdomains that are strongly influenced by mathematics CK.
Discussion and conclusion
The present study investigated the relationship between teacher students’ physics and mathematics CK. As mathematics is regarded to be the language of physics and used to model physics phenomena, and given that certain teacher education programs (such as in Germany) require teacher students to study both subjects on a broad and deep academic level, we assumed synergy effects to occur. Our results revealed such effects of studying mathematics as a second subject on developing physics CK, although the effect size was only small. Quite interesting, the synergy effects are prominent in the mid to later years of the study; in contrast, mathematics as a second subject seems to hinder the development of teacher students’ physics CK in the first and second year. It is well known that undergraduate mathematics students are exposed to serious challenges in their early years of study (e.g. Hoyles, Newman, and Noss Citation2001; Neumann, Jeschke, and Heinze Citation2020; Rach and Heinze Citation2017). Given that mathematics teacher students (as in our sample) typically take the same mathematics courses as the mere mathematics majors, teacher students likely experience the same challenges as well. This may result in putting less effort in taking the physics courses for the benefit of taking the mathematics courses – in particular, compared to physics teacher students with another second subject. However, the later years of the physics studies typically include courses on theoretical physics which are characterized by a stark mathematization and pose mathematics-related challenges to the students. A solid and deep understanding of mathematical concepts may then be beneficial. Overall, our study may also indicate the importance of adequate timing of opportunities to learn. If students learn mathematics concepts concerted to physics concepts, in which the mathematics concepts are employed, synergy effects may even increase.
As every empirical study, the present is subject to limitations. The employed instruments were designed to cover the breadth and depth of mathematics and physics teacher students’ content knowledge and thus included only a limited number of items per subdomain. To further generalize the relationship between mathematics CK and single physics subdomains, more specific items would be helpful. Additionally, our study employed a cross-sectional design; to investigate the effects of particular lectures or seminars, teacher students should be surveyed in a longitudinal manner.
Nevertheless, our study indicates, that teacher students’ physics CK may benefit from additional learning opportunities in academic mathematics and that the synergy effects vary along with students’ learning progression. Given that other (scientific) disciplines also make use of mathematical models and representations, it might be interesting to investigate teacher students of mathematics and (science) subjects other than physics as well (see Jeschke et al., Citation2019). Likewise, it remains an open question, to what extent learning opportunities in university teacher education help to develop other facets of teacher professional knowledge such as pedagogical content knowledge across different domains. Finally, our results suggest that synergy effects may exist in other areas of higher education (see also Brückner et al. Citation2020; Jeschke et al. Citation2019; Klein et al. Citation2019), and are worth further investigation.
Supplemental Material
Download PDF (131.2 KB)Disclosure statement
No potential conflict of interest was reported by the author(s).
Additional information
Funding
Notes
1 We repeated the data analyses without the two universities with differing participation rates. We did not find differences in effect sizes compared to the full sample.
References
- Abell, S. K. 2007. “Research on Science Teacher Knowledge.” In Handbook of Research on Science Education, edited by S. K. Abell, and N. G. Lederman, 1105–49. Mahwah, NJ: Lawrence Erlbaum.
- Anderson, L. W. 1981. “Instruction and Time-on-Task: A Review.” Journal of Curriculum Studies 13: 289–303.
- Ball, D. L., M. H. Thames, and G. Phelps. 2008. “Content Knowledge for Teaching: What Makes it Special?” Journal of Teacher Education 59 (5): 389–407.
- Baumert, J., M. Kunter, W. Blum, M. Brunner, T. Voss, A. Jordan, U. Klusmann, S. Krauss, M. Neubrand, and Y.-M. Tsai. 2010. “Teachers’ Mathematical Knowledge, Cognitive Activation in the Classroom, and Student Progress.” American Educational Research Journal 47 (1): 133–80.
- Beaujean, A. 2014. Latent Variable Modeling Using R. A Step-by-Step Guide. New York: Routledge.
- Berry, A., P. Friedrichsen, and J. Loughran. 2015. Re-examining Pedagogical Content Knowledge in Science Education, 28–42. New York: Routledge.
- Blömeke, B., G. Kaiser, B. Schwarz, R. Lehmann, S. Seeber, C. Müller, and A. Felbrich. 2008. “Entwicklung des fachbezogenen Wissens in der Lehrerausbildung.” In Professionelle Kompetenz angehender Lehrerinnen und Lehrer. Wissen, Überzeugungen und Lerngelegenheiten deutscher Mathematikstudierender und -refendare; erste Ergebnisse zur Wirksamkeit der Lehrerausbildung, edited by S. Blömeke, G. Kaiser, and R. Lehmann, 135–70. Münster: Waxmann.
- Brückner, Sebastian, Olga Zlatkin-Troitschanskaia, Stefan Küchemann, Pascal Klein, and Jochen Kuhn. 2020. “Changes in Students’ Understanding of and Visual Attention on Digitally Represented Graphs Across Two Domains in Higher Education: A Postreplication Study.” Frontiers in Psychology 11: 2090.
- Dreher, A., A. Lindmeier, and A. Heinze. 2018. “What Kind of Content Knowledge do Secondary Mathematics Teachers Need? A Conceptualization Taking Into Account Academic and School Mathematics.” Journal für Mathematikdidaktik 39 (2): 319–41. doi:https://doi.org/10.1007/s13138-018-0127-2.
- Gess-Newsome, J. 2015. “A Model of Teacher Professional Knowledge and Skill Including PCK: Results of the Thinking from the PCK Summit.” In Re-examining Pedagogical Content Knowledge in Science Education, edited by A. Berry, P. Friedrichsen, and J. Loughran, 28–42. New York: Routledge.
- Grossman, P., A. Schoenfeld, and C. Lee. 2005. “Teaching Subject Matter.” In Preparing Teachers for a Changing World. What Teachers Should Learn and be Able to do, edited by L. Darling-Hammond, and J. Bransford, 301–31. San Francisco: Jossey-Bass.
- Heinze, A., A. Dreher, A. Lindmeier, and C. Niemand. 2016. “Akademisches versus schulbezogenes Fachwissen – ein differenzierteres Modell des fachspezifischen Professionswissens von angehenden Mathematiklehrkräften der Sekundarstufe.” [Academic vs school-related content knowledge: A differentiated model of discpline-specific teacher knowledge of pre-service secondary Mathematics teachers]. Zeitschrift für Erziehungswissenschaft 19 (2): 329–49.
- Hoyles, C., K. Newman, and R. Noss. 2001. “Changing Patterns of Transition from School to University Mathematics.” International Journal of Mathematical Education in Science and Technology 32 (6): 829–45.
- Hu, L., and P. M. Bentler. 1999. “Cutoff Criteria for fit Indexes in Covariance Structure Analysis: Conventional Criteria Versus new Alternatives.” Structural Equation Modeling 6 (1): 1–55.
- Jeschke, C., C. Kuhn, A. Lindmeier, O. Zlatkin-Troitschanskaia, H. Saas, and A. Heinze. 2019. “What is the Relationship Between Knowledge in Mathematics and Knowledge in Economics? Investigating the Professional Knowledge of (pre-Service) Teachers Trained in two Subjects.” Zeitschrift für Pädagogik 65 (4): 511–24.
- Keller, M. M., K. Neumann, and H. E. Fischer. 2017. “The Impact of Physics Teachers’ Pedagogical Content Knowledge and Motivation on Students’ Achievement and Interest.” Journal of Research in Science Teaching 54 (5): 586–614.
- Kind, V. 2014. “Science Teachers’ Content Knowledge.” In Exploring Mathematics and Science Teachers’ Knowledge: Windows Into Teacher Thinking, edited by H. Venkat, M. Rollnick, M. Askew, and J. Loughran, 15–28. Oxford: Routledge.
- Kleickmann, T., J. Großschedl, U. Harms, A. Heinze, S. Herzog, F. Hohenstein, O. Köller, et al. 2014. “Professionswissen von Lehramtsstudierenden der Mathematisch-Naturwissenschaftlichen Fächer – Testentwicklung im Rahmen des Projekts KiL. [Professional Knowledge of Teacher Students in Mathematics and the Science Subjects – Test Development in Scope of the Project KiL].” Unterrichtswissenschaft 42 (3): 280–88. doi:https://doi.org/1403280.
- Kleickmann, T., D. Richter, M. Kunter, J. Elsner, M. Besser, S. Krauss, and J. Baumert. 2013. “Teachers’ Content Knowledge and Pedagogical Content Knowledge: The Role of Structural Differences in Teacher Education.” Journal of Teacher Education 64 (1): 90–106.
- Klein, P., S. Küchemann, S. Brückner, O. Zlatkin-Troitschanskaia, and J. Kuhn. 2019. “Student Understanding of Graph Slope and Area Under a Curve: A Replication Study Comparing First-Year Physics and Economics Students.” Physical Review Physics Education Research 15 (2): 020116.
- Krauss, S., J. Baumert, and W. Blum. 2008. “Secondary Mathematics Teachers’ Pedagogical Content Knowledge and Content Knowledge: Validation of the COACTIV Constructs.” ZDM Mathematics Education 40: 873–92.
- Kulgemeyer, C., and J. Riese. 2018. “From Professional Knowledge to Professional Performance: The Impact of CK and PCK on Teaching Quality in Explaining Situations.” Journal of Research in Science Teaching 55 (10): 1393–418.
- Kultusministerkonferenz (KMK). 2008. Ländergemeinsame inhaltliche Anforderungen für die Fachwissenschaften und Fachdidaktiken in der Lehrerbildung [Common Substantial Requirements for Content and Pedagogical Content in Teacher Training]. Berlin: Sekretariat der Kultusministerkonferenz.
- Kunter, M., U. Klusmann, J. Baumert, D. Richter, T. Voss, and A. Hachfeld. 2013. “Professional Competence of Teachers: Effects on Instructional Quality and Student Development.” Journal of Educational Psychology 105 (3): 805–20. doi:https://doi.org/10.1037/a0032583.
- Lachner, A., and M. Nückles. 2016. “Tell me why! Content Knowledge Predicts Process-Orientation of Math Researchers’ and Math Teachers’ Explanations.” Instructional Science 44 (3): 221–42. https://link.springer.com/article/10.1007/s11251-015-9365-6.
- Neumann, K., H. Härtig, U. Harms, and I. Parchmann. 2017. “Science Teacher Preparation in Germany.” In Model Science Teacher Preparation Programs: An International Comparison of What Works Best, edited by J. Pedersen, T. Isozaki, and T. Hirano, 29–52. Charlotte, NC: Information Age Publishing.
- Neumann, I., C. Jeschke, and A. Heinze. 2020. “First Year Students’ Resilience to Cope with Mathematics Exercises in the University Mathematics Studies.” Journal für Mathematik-Didaktik. doi:https://doi.org/10.1007/s13138-020-00177-w.
- Nixon, R., R. Toerien, and J. Luft. 2019. “Knowing More Than Their Students: Characterizing Secondary Science Teachers’ Subject Matter Knowledge.” School Science and Mathematics 119: 150–60.
- Rach, S., and A. Heinze. 2017. “The Transition from School to University in Mathematics: Which Influence do School-Related Variables Have?” International Journal of Science and Mathematics Education 15 (7): 1343–63. doi:https://doi.org/10.1007/s10763-016-9744-8.
- Redish, E. F., and E. Kuo. 2015. “Language of Physics, Language of Math: Disciplinary Culture and Dynamic Epistemology.” Science & Education 24: 561–90. doi:https://doi.org/10.1007/s11191-015-9749-7.
- Rollnick, M. 2017. “Learning About Semi Conductors for Teaching—the Role Played by Content Knowledge in Pedagogical Content Knowledge (PCK) Development.” Research in Science Education 47: 833–68. doi:https://doi.org/10.1007/s11165-016-9530-1.
- Sackett, P. R., N. R. Kuncel, J. J. Arneson, S. R. Cooper, and S. D. Waters. 2009. “Does Socioeconomic Status Explain the Relationship Between Admissions Tests and Post-Secondary Academic Performance?” Psychological Bulletin 135 (1): 1–22.
- Sadler, P. M., G. Sonnert, H. P. Coyle, N. Cook-Smith, and J. L. Miller. 2013. “The Influence of Teachers’ Knowledge on Student Learning in Middle School Physical Science Classrooms.” American Educational Research Journal 50 (5): 1020–49. doi:https://doi.org/10.3102/0002831213477680.
- Schmidt, W. H., L. Cogan, and R. Houang. 2011. “The Role of Opportunity to Learn in Teacher Preparation: An International Context.” Journal of Teacher Education 62 (2): 138–53.
- Schmitt, N., J. Keeney, F. L. Oswald, T. J. Pleskac, A. Q. Billington, R. Sinha, and M. Zorzie. 2009. “Prediction of 4-Year College Student Performance Using Cognitive and Noncognitive Predictors and the Impact on Demographic Status of Admitted Students.” Journal of Applied Psychology 94: 1479–97.
- Schwab, J. J. 1978. Science, Curriculum and Liberal Education. Chicago: University of Chicago Press.
- Shulman, L. S. 1986. “Those who Understands: Knowledge Growth in Teaching.” Educational Researcher 15 (2): 4–14.
- Sorge, S., J. Kröger, S. Petersen, and K. Neumann. 2019. “Structure and Development of pre-Service Physics Teachers’ Professional Knowledge.” International Journal of Science Education 41 (7): 862–89. doi:https://doi.org/10.1080/09500693.2017.1346326.
- Tatto, M. T., J. Schwille, S. L. Senk, L. Ingvarson, G. Rowley, R. Peck, K. Bankov, M. Rodriguez, and M. Reckase. 2012. Policy, Practice, and Readiness to Teach Primary and Secondary Mathematics in 17 Countries: Findings from the IEA Teacher Education and Development Study in Mathematics (TEDS-M). Amsterdam, Netherlands: International Association for the Evaluation of Educational Achievement.
- Tatto, M. T., and S. Senk. 2011. “The Mathematics Education of Future Primary and Secondary Teachers: Methods and Findings from the Teacher Education and Development Study in Mathematics.” Journal of Teacher Education 62: 115–20. doi:https://doi.org/10.1177/0022487110386798.
- Tchoshanov, M., M. D. Cruz, K. Huereca, K. Shakirova, L. Shakirova, and E. N. Ibragimova. 2017. “Examination of Lower Secondary Mathematics Teachers’ Content Knowledge and Its Connection to Students’ Performance.” International Journal of Science and Mathematics Education 15: 683–702. doi:https://doi.org/10.1007/s10763-015-9703-9.
- Uhden, O., R. Karam, M. Pietrocolo, and G. Pospiech. 2012. “Modelling Mathematical Reasoning in Physics Education.” Science & Education 21: 485–506.
- Wise, K. C., and J. R. Okey. 1983. “A Meta-Analysis of the Effects of Various Science Teaching Strategies on Achievement.” Journal of Research in Science Teaching 20 (5): 419–35.
- Wu, H.-H. 2018. “The Content Knowledge Mathematics Teachers Need.” In Mathematics Matters in Education, edited by Y. Li, W J Lewis, and J. Madden, 43–91. Cham: Springer.