ABSTRACT
Gadoids are a group of fish with historical importance in the fishing industry. The high demand for cod is one of the reasons why cod products are often mislabelled, and numerous observations have been made on the replacement of Atlantic cod (Gadus morhua) by cheaper species or its illegal capture in contravention of fish quotas. Fish species identification is traditionally based on morphological features, but this may be difficult in case of heat-treated or processed products, or where the species look similar, as in the Gadoid group. DNA-based approaches (using either nuclear or mitochondrial DNA) are most commonly used in this case, due to their high specificity and to the high resilience of the target molecules to food processing techniques. In this article, we identified, using an automated screening approach, novel barcode regions and their associated primers in the nuclear genome, to be used for the efficient identification of Gadoids. The barcode regions were tested on official and commercial samples, raw or mildly treated products, like frozen, or salted, as well as pre-cooked complex mixtures and processed samples, using next-generation sequencing (NGS) technique. The method proposed could complement existing fish identification strategies in establishing an efficient framework to detect and prevent frauds along the food chain.
Introduction
Seafood consumption has significantly increased over the last decades and so has the demand for fish, which is considered the most common protein source consumed worldwide. Owing to this increasing popularity, seafood has become one of the top categories associated with fraud issues. Seafood fraud involves several aspects of the industry, including economically motivated fraud (Everstine et al. Citation2013); consumer safety (Miller and Mariani Citation2010); and sustainability of fisheries (Jacquet and Pauly Citation2008; Triantafyllidis et al. Citation2010). In order to control and reduce economic fraud, the European Union (EU) has established legislation that regulates seafood labelling, requiring traceability information, such as commercial and scientific names, fishing and production methods, catch area and the fishing gear; for other processed foods, such as canned, composite products and breaded products, this information is voluntary (European Union Citation2011; European Union Citation2013). Despite these efforts, widespread seafood mislabelling has been reported in the United States (Khaksar et al. Citation2015), in Europe (Filonzi et al. Citation2010; Miller and Mariani Citation2010; Garcia-Vazquez et al. Citation2011; Nedunoori et al. Citation2017), in Asia (Xiong et al. Citation2016) and in South Africa (Cawthorn et al. Citation2011), indicating the presence of flaws in legislation on food traceability and the need for stringent control measures to guarantee efficient species identification.
Gadoids are a group of fish with historical importance in the fishing industry, representing approximately 18% of the world’s total catch (FAO Citation2014). Various species of the Gadidae family belong to this group, including Atlantic cod (Gadus morhua), Pacific cod (Gadus macrocephalus), Alaska pollock (Gadus chalcogrammus), Pollock (Pollachius pollachius) and Saithe (Pollachius virens). Most of these species look similar, which makes their morphological identification very difficult or almost impossible. The high demand for cod is one of the reasons why cod products are often mislabelled, and numerous observations have been made on the replacement of Atlantic cod (G. morhua) by cheaper species (Helyar et al. Citation2014) or its illegal capture being hidden by the use of other species’ names (Miller and Mariani Citation2010).
In the case of G. morhua, several research works and institutional publications have identified adulteration and misclassification prevalently with G. macrocephalus and G. chalcogrammus (Miller et al. Citation2012; Cutarelli et al. Citation2014; US FDA Citation2014; Mendes and Silva Citation2015).
Fish species identification is traditionally based on morphological features (Strauss and Bond Citation1990), but this may be difficult or even impossible in case of processed products. Food authenticity can be assessed using a broad variety of methods, such as those based on protein (Mazzeo and Siciliano Citation2016) or DNA analysis (Griffiths et al. Citation2014). DNA-based approaches are by far the most popular methods, mainly due to their high specificity but also to the relatively high resistance of target molecules to food processing techniques. Several methodologies have been carried out using either nuclear DNA (nDNA) or mitochondrial DNA (mtDNA). The most prominent ones are the Forensically Informative Nucleotide Sequencing (FINS), Restriction Fragment Length Polymorphism (RFLP); Single-Stranded Conformational Polymorphism (SSCP); Random Amplified Polymorphic DNA (RAPD); Amplified Fragment Length Polymorphism (AFLP); Loop-mediated isothermal amplification (LAMP) assay; High Resolution Melting (HRM) analysis; some of them already available as commercial applications (Asensio Gil Citation2007; Rasmussen and Morrissey Citation2008; Clark Citation2015; Saull et al. Citation2016; Fernandes et al. Citation2017, Citation2018; Tomás et al. Citation2017).
Within the sphere of DNA-based approaches, much attention has been devoted to DNA barcoding, which relies on sequence variations within a short and standardised region of the genome, designated as a ‘‘barcode’. DNA barcoding was shown to provide accurate species identification (Hebert et al. Citation2003). Currently, the mitochondrial genes coding for cytochrome c oxidase subunit I (COI) and cytochrome b (cytb) are considered reliable DNA barcodes for the discrimination of animal species (Hebert et al. Citation2003; Hellberg et al. Citation2014; Mueller et al. Citation2015). For the identification of fish species, mitochondrial loci have been preferred to nuclear genes because of their features: mitochondrial genes belong to a haploid genome, they are present in high copy numbers (particularly in fish tissues) and their mutation rate is greater than that of nuclear genes (Cline Citation2012; Rehbein Citation2013). The number of DNA barcodes deposited in databases is growing continuously (http://www.fishbol.org/). Despite the fact that the majority of the studies have used COI or cytb mtDNA barcoding to identify seafood products and investigate broad patterns in fish mislabelling (Rasmussen and Morrissey Citation2008; Miller and Mariani Citation2010; Cline Citation2012; Di Pinto et al. Citation2013), novel nuclear barcode regions have also been proposed for the identification for example, of flatfish species (Paracchini et al. Citation2017). The length of these nDNA barcodes is generally shorter than that of the mtDNA barcodes: this facilitates the amplification of the DNA even in the case of highly processed food products and the compatibility with the current next-generation sequencing next-generation sequencing (NGS) technologies, allowing the identification of species also in case of mixture (Paracchini et al. Citation2017).
Despite the efficacy of the sequencing methodology currently used for the identification of Gadoid species, the efficiency of barcoding can be improved by targeting additional genomic positions. In this report, we propose a set of novel nuclear barcoding targets for Gadoid species identification. The barcodes were identified using an automated screening approach, then tested on official and commercial samples, raw or mildly treated products, like frozen, or salted, as well as pre-cooked complex mixtures and processed samples.
Material and methods
Sample collection
Thirty three samples were analysed in total () and consisted of official and market samples. Official samples: samples for G. morhua (n = 4) available in the biological reference collection of the FishTrace project (https://fishtrace.jrc.ec.europa.eu/) were obtained from the Swedish Museum of Natural History. Biological samples for G. macrocephalus (n = 6) (Eastern Pacific cod from Alaska and Western Pacific cod from South Korea) were provided by the School of Aquatic and Fishery Sciences of the University of Washington (U.S.A.). The exact nomenclature of each sample was confirmed by COI barcoding. Other fish specimens (n = 23) were purchased from local markets and supermarkets. The tested specimens were sold as frozen Nordic cod (G. morhua), dried and salted cod (Gadus spp.), frozen fish sticks (Hake – Merluccius spp.), Whiting (Merlangius merlangus), Pollock (Pollachius pollachius), Saithe (Pollachius virens), frozen Alaska pollock burger and Alaska pollock fillet (G. chalcogrammus) and surimi (processed product prepared from mixed fish species).
Table 1. List of samples tested, and summary of the evidence, based on traditional barcoding techniques, regarding the correct species for all the specimens used in the study.
DNA extraction
Total DNA was extracted from 2 to 200 mg tissue sample depending on the availability and abundance of the sample following manufacturer’s recommendations (DNeasy® Blood & Tissue Kit, Qiagen). DNA concentration was quantified using Qubit dsDNA BR Assay Kit using the Qubit® 3.0 Fluorometer (Invitrogen). The Nanodrop spectrophotometer (Thermofisher) was used to evaluate the purity of the samples and ratio 260/280 nm was recorded and taken into consideration. DNA samples were diluted to a final concentration of 10 ng/μl unless lower amounts were obtained.
Primers design and selection of nuclear barcodes
Primer design of 10 candidate nuclear barcode regions was achieved through the strategy described by Paracchini et al. (Citation2017). Compared to that study, the main difference is that an additional filter was applied in order to select only primer pairs predicted to amplify a single genomic locus. This modification would allow performing the analysis using Sanger sequencing, while relying on the use of NGS in case of mixtures or complex samples.
From this shorter list, the capability of the different barcodes to differentiate the Gadoid species of interest was predicted by calculating in silico the number of inter-species differences, comparing it, when relevant, to intra-species differences. This was determined as described in the following paragraphs. All calculations were done using R version 3.3.1. (http://www.r-project.org/).
A) Intra-species differences: Per primer pair, the amplicon sequences were inspected for their origin (species). If multiple sequences were present for a single species, the sequences were aligned (using the multiple sequence alignment available through the package ‘msa’). The Levenshtein distance (i.e. the difference between two sequences) was calculated across all pairwise alignments, using the ‘StringDist’ function from the package ‘Biostrings’. A consensus sequence was also generated (using the ‘consensus’ function of the package ‘seqinr’ with ‘method = majority’) to be used as the sequence to be compared for this species in the evaluation of inter-species differences.
B) Inter-species differences: A multiple alignment across all species was generated and the Levenshtein distance across all pairwise alignments was calculated.
The results can be graphically represented, as shown in , as a distance matrix. The diagonal of this distance matrix was populated by inserting the maximal intra-species Levenshtein distance for this species (as found during step A). The complete set of Levenshtein matrices for all the genomic regions is presented in Annex 1. Based on the results, the genomic regions were ranked in order of suitability for distinguishing cod from other fish species. For each primer pair, ‘suitability’ was high when the inter-species differences were high compared to the intra-species differences. In a next step, the top performing regions were used to design primers that allow their amplification from all species within the analysis. The detailed primers sequences are reported in .
Table 2. Candidate barcode targets and primers selected for the study. The table shows the size of the predicted amplicon. The gene targeted by each barcode was determined by the annotation of the region in the G. morhua genome.
Figure 1. Rapid evaluation of the usefulness of a genomic region targeted by primer 4049-aab to distinguish one species from another.
The species used for this analysis were those for which a genome was publicly available. Each column/row shows the number of differences found between the species numbered 1–33 (between species variability, the larger the more useful the sequence is in distinguishing species). The diagonal shows the differences found between the different sequence versions of that species (within species variability, the larger the more difficult it is to uniquely identify the species). The results are colour coded red to green in the main matrix body (green = high between species variability) and purple to green on the diagonal (green = low within species variability). List of species: 1) Cynoglossus semilaevis; 2) Cyprinodon variegatus; 3) Cyprinus carpio; 4) Danio rerio; 5) Dicentrarchus labrax; 6) Gadus macrocephalus; 7) Gadus morhua; 8) Gasterosteus aculeatus; 9) Haplochromis burtoni; 10) Kryptolebias marmoratus; 11) Labrus bergylta; 12) Larimichthys crocea; 13) Lates calcarifer; 14) Lepisosteus oculatus; 15) Maylandia zebra; 16) Miichthys miiuy; 17) Neolamprologus brichardi; 18) Notothenia coriiceps; 19) Oreochromis niloticus; 20) Oryzias latipes; 21) Poecilia Formosa; 22) Poecilia latipinna; 23) Poecilia mexicana; 24) Pundamilia nyererei; 25) Pygocentrus nattereri; 26) Sinocyclocheilus anshuiensis; 27) Sinocyclocheilus graham; 28) Sinocyclocheilus rhinocerous; 29) Stegastes partitus; 30) Takifugu rubripes; 31) Tetraodon nigroviridis; 32) Thunnus orientalis; 33) Xiphophorus maculatus.
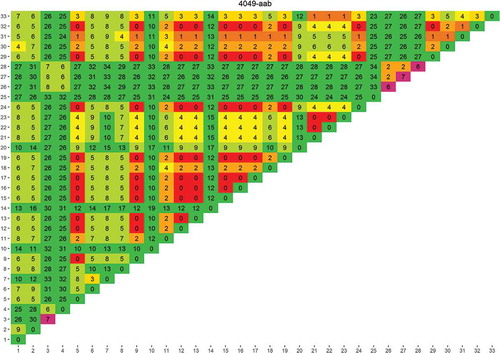
COI and nuclear barcodes sequencing
The identification of the species obtained from official samples and purchased specimens was confirmed in house using the traditional COI barcoding method, using published primers and protocols (Ward et al. Citation2005; Ivanova et al. Citation2007).
Individual PCR amplifications for nuclear target barcodes were performed in 50 μl using 2.5 U/reaction of AmpliTaq Gold DNA Polymerase, 1 x Buffer II, 2.5 mM of MgCl2 (Applied Biosystems), 200 μM dNTPs and 200 nM of each primer. DNA samples were amplified in a GeneAmp PCR System 9700 (ABI, U.S.A.), with the following cycling parameters, according to the protocol of the AmpliTaq Gold PCR system: initial denaturation at 95°C for 10 min, followed by 45 cycles of denaturation at 95°C for 30 s, annealing at 55°C or 60°C for 30 s, extension at 72°C for 30 s and final extension at 72°C for 7 min. PCR products were analysed on an agarose gel electrophoresis to verify and confirm the expected size.
PCR products were purified by Qiaquick PCR purification Kit (Qiagen GmbH, Hiden, Germany) and bi-directionally PCR sequenced with BigDye Terminator v3.1 Cycle Sequencing Kit (Applied Biosystems, Foster City, California) following manufacturer’s recommendations. Sequences were dye-terminator removed by CentriSep Spin columns (Princeton Separations) and therefore run on 3730 Genetic Analyser (Applied Biosystems, Foster City, California). Electropherograms were analysed using Sequencing analysis v5.2 software (Applied Biosystems, Foster City, California).
For the analysis of mitochondrial COI barcodes, the identification of species was performed via the Boldsystem portal (http://www.boldsystems.org/).
Meta-barcoding sequencing and analysis
NGS was performed on a GS Junior System (GS Junior System, 454 Life Sciences, Roche Applied Sciences, Basel, Switzerland). Amplicon libraries were prepared using fusion primers for bidirectional sequencing as described in the Amplicon library preparation manual (Roche Applied Sciences, Basel, Switzerland). Multiplex Identifiers (MIDs) were added in order to allow inclusion of more than one sample in the same experiment.
After amplification, amplicons were purified using AMPure XP beads (Roche Applied Sciences, Basel, Switzerland), quantified fluorometrically (Quant-iT PicoGreen dsDNA Assay kit, Life Technologies, Molecular Probes, Eugene, Oregon, U.S.A.), diluted and pooled to a final concentration of 0.5 × 106 molecules μL−1. Libraries were checked for their quality by performing a Quality Control PCR; they were subsequently visualised using Agilent DNA 1000 Chips (Agilent Bioanalyzer, Agilent Technologies, San Diego, U.S.A.). Emulsion PCR containing between 0.6 and 0.75 copies per bead (cpd) was recovered using vacuum and the successive enrichment led to an enrichment rate between 10% and 20%; only 5% of the enriched beads were subsequently loaded on the chip and sequenced. All steps were in accordance with the manufacturers’ instructions.
The output (FASTA format) was split using fastx_barcode_splitter from the Fastx-toolkit (v. 0.0.14) (Gordon and Hannon Citation2010) to isolate the reads from the different samples tested in the same run using the MID sequences. The primer sequences were then trimmed using Cutadapt (v. 1.7.1) (Martin Citation2011). Scripts were used to analyse each read against the reference files using Glsearch from the FASTA package (v. 36.3.7a) to identify matches between the entire length of each read and local regions of the reference barcode sequences. The number of mismatches allowed was set at 1%, i.e. 0 for barcodes less than 100 bp, 1 for those between 100 and 200 bp and 2 for those between 200 and 300 bp. In case of more than one hit, the most recent common ancestor for the identified species was determined using the API of the Open Tree of Life (Watanabe Citation2013), following the instructions at https://github.com/OpenTreeOfLife/germinator/wiki/Open-Tree-of-Life-Web-APIs. If no hit matched the minimum criteria the read was assigned to an ‘Unassigned’ conclusion. The main reason for this would be errors in the sequences from the 454 pyrosequencing process. The occurrences of these remained limited (on average less than 5% of total reads).
Results and discussion
In the current study, we used a strategy similar to the one we recently published (Paracchini et al. Citation2017) in order to identify a set of novel barcode regions in nuclear genomes of gadoids. The aim of the work was also to analyse the feasibility of nuclear barcode identification of mildly-treated, processed and mixed cod samples.
Barcode selection
Among the candidate barcode primers, a set of 10 pairs were selected to be evaluated experimentally (), based on their capability to distinguish species (number of inter-species and intra-species differences). This evaluation has been performed by PCR in silico simulation on all available fish genomic sequences and selecting only those ones with a single predicted amplicon. Due to this specification, it was possible to amplify these fragments and analyse them via Sanger sequencing. Details, including their annotation in the genome, are presented in . The selection of the barcodes and the length range of the amplicon (spanning from 122 to 305 bp) was also based on the capacity of the NGS technology we used.
Sample analyses
A set of different samples was analysed, including official and market samples, raw, frozen, salted and processed (two samples were baked in the oven at 200°C for 30 min). A complete list of samples included in the study is presented in . DNA was extracted from all specimens, with different absorbance ratios at 260/280 nm (from 1.2 to 2.1) and different concentrations (1.85–118 ng/uL). Moreover, depending on the type of storage of the samples, and in particular in salted specimens, different degrees of degradation were observed in accordance with the findings described by (Dalmasso et al. Citation2013).
Extracted DNAs have been used as template for PCR and sequencing by Sanger. A summary is reported in . For G. chalcogrammus three samples were tested, five samples for G. macrocephalus, four samples for G. morhua, one sample for M. merluccius, eight samples for M. paradoxus, two samples for M. merlangus, one sample for P. pollachius and one sample for P. virens. In the case of official samples, only one of the available specimens was analysed, due to the fact that the specimens belonged to the same individual. In the case of M. paradoxus, only few barcode regions were tested, because they were considered to be the most informative for species discrimination. Moreover, due to the fact that the 7226-aab, -aad, -aae, -aaf primers bind on the same genetic region (7226), the sequencing analysis was performed on only one primer pair for all the samples, i.e. 7226-aad.
Table 3. Tested primers on different samples from different species. Each primer has been tested on DNA from different samples. In case of more than one sample per species, all of them have been tested and sequenced. In cases where no PCR product was obtained (T-X), the main reason was a low PCR yield. Legend: T = PCR tested; A = amplicon present; S = amplicon sequenced; X = amplicon not present/amplicon not sequenced; – = PCR not tested.
The surimi sample, being a mixture of different species, was not tested by Sanger sequencing but with a different approach (NGS), as detailed in section 'Mixture analyses'.
For each primer pair for which a sequence of the amplicon was available for all eight fish species (i.e. 2034-aac, 4049-aab and 7226-aad) corresponding to four different genera, the produced sequences from the various samples have been compared to each other with two different objectives:
Ability to easily distinguish between Gadus and other genera.
Ability to detect a genomic region that is specific to Gadus morhua.
We found that these three tested primer pairs could be used to distinguish, by sequencing the amplicon products, the Merluccius genus, as specific differences exist for each of the conserved genomic loci that have been analysed (see ). In addition to that, primer pair 2034-aac produces an amplicon in Gadus morhua that contains an insertion in its middle that is characteristic of such a species (). This region (in blue in ) will be tested for designing a real-time PCR detection method for Gadus morhua.
Figure 2. Alignment of sequences amplified by using primer pair 4049-aab.
The alignment of sequences amplified by using primer pair 4049-aab is shown. Each sequence represents one of the following species: Gadus chalcogrammus (Gcha), G. macrocephalus (Gmac), G. morhua (Gmor), Merluccius merluccius (Mmer), M. paradoxus (Mpar), M. Merlangus (Mmel), P. virens (Pvir) and P. pollachius (Ppol). As highlighted by colours, there are six different base positions on this locus that, taken all together into account, could be used to distinguish Merluccius genus (in red) from the others (in green).
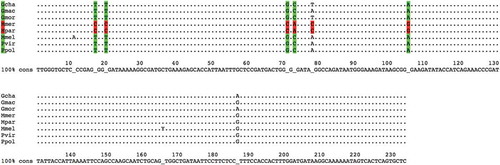
Figure 3. Alignment of sequences amplified by using primer pair 2034-aac.
The alignment of sequences amplified by using primer pair 2034-aac is shown. Each sequence represents one of the following species: Gadus chalcogrammus (Gcha), G. macrocephalus (Gmac), G. morhua (Gmor), Merluccius merluccius (Mmer), M. paradoxus (Mpar), M. Merlangus (Mmel), P. virens (Pvir) and P. pollachius (Ppol). As highlighted by colours, there are seven different base positions on this locus that, taken all together into account, can be used to distinguish the Merluccius genus (in red) from the others (in green). In addition to that, Gadus morhua has a peculiar insertion of 12 bases (highlighted in blue). Bases in lowercase indicate those bases that, among the different samples tested in one species, are not always present.

Figure 4. Alignment of sequences amplified by using primer pair 7226-aad.
The alignment of sequences amplified by using primer pair 7226-aad is shown. Each sequence represents one of the following species: Gadus chalcogrammus (Gcha), G. macrocephalus (Gmac), G. morhua (Gmor), Merluccius merluccius (Mmer), M. paradoxus (Mpar), M. Merlangus (Mmel), P. virens (Pvir) and P. pollachius (Ppol). Primer pair 7226-aad produces genera-specific amplicons in terms of length. Moreover, as highlighted by colours, there are different single base positions on this locus that, taken all together into account, can be used to distinguish Merluccius genus (in red) from the others (in green); in particular, Gadus morhua has two peculiar variations (highlighted in blue).
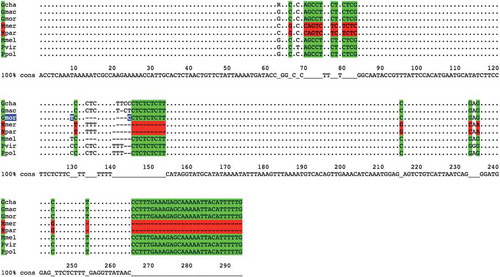
Mixture analyses
Once the pure samples were analysed, the system was challenged for its detection capability on mixtures containing different species in complex samples. A commercially available sample containing mixtures of processed white fish labelled as ‘Smoked salmon surimi’ was tested (). The label listed the ingredients as ‘fish flesh 43% (of which smoked salmon 8%)’. Amplification of the COI region, coupled with Sanger sequencing, produced a clean sequence that was analysed in the BOLD systems identification portal (http://www.boldsystems.org/) and identified the product as ‘Gadus chalcogrammus’, i.e. Alaska Pollock or Pacific Pollock (, upper part) a species often found in surimi products (Pepe et al. Citation2007; Ferrito et al. Citation2016). The surimi sample was tested in NGS with the nuclear barcode primers designed for this study. A total of 72,108 reads were obtained for the tested barcodes, and the results indicated the presence of different components: 21% of the reads were assigned to Gadus genus or to closely related species (6.3% of the reads assigned to G. chalcogrammus, 1.4% to G. morhua), 19% were assigned to Euteleosteomorpha (a cohort comprehending the genus Gadus), 16% of the reads were assigned to the salmon (Salmo salar) component of the fish flesh which was mentioned on the label, and the rest of the reads (32%) were unassigned (, lower part).
Figure 5. Analysis of complex, processed fish samplesA ‘Smoked salmon surimi’ product from the supermarket was analysed. Upper part: the traditional COI barcode, coupled to Sanger sequencing, produced a single sequence that was classified as ‘Gadus chalcogrammus’ by the BOLD systems identification portal (Ratnasingham and Hebert Citation2007). Lower part: the NGS analysis, in contrast, also detected the minority salmon fraction of the fish flesh. The picture represents a pool of the results obtained for all the nuclear barcode primers analysed (2034-aac, 4049-aab, 3726-aad, 3726-aab, 7226-aad, 7226-aaf, 10,029-aab, 10,029-aac).
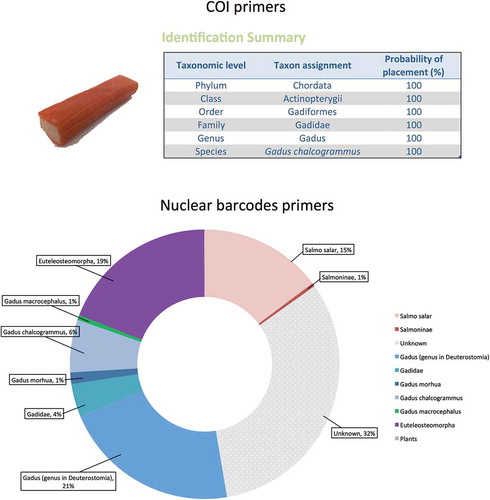
Conclusions
The method suggested in this study is potentially able to detect and identify correctly the species even in difficult matrices like mildly treated or processed seafood specimens thanks to the high resilience of DNA as a marker. Moreover, the use of nuclear barcode targets coupled with NGS could be applied to mixtures, allowing the identification of different species even in complex samples. NGS is an extremely sensitive technique, so its performance criteria in application to regulatory purposes (specificity, sensitivity and reproducibility) require severe quality controls and cut-off values.
NGS, in particular when coupled with PCR, is a powerful approach to detect different sources of DNA even in trace quantities, but it cannot be considered as a quantitative analysis. This is because of the influence of the PCR step preceding the NGS, which may cause incorrect estimation of the relative species composition and misleading results. This could be an issue when trying to distinguish a fraud from an event of accidental cross contamination during production. Nevertheless, because of the fact that cross contamination is accidental, an appropriate sampling strategy, in the number of samples to be analysed, and replicates to be included in the experiment, should provide a more appropriate way to address this point; statistical estimation of the adequate sampling strategy would be crucial in this case.
Quantification was not the objective of the current article. In this case, whole genome sequencing (WGS) could provide the appropriate answers. Instead, for qualitative questions where detection (presence or absence) of the analyte is crucial, the methodology described here seems promising, even when tested on mixtures. Moreover, the fact that amplicons are short enough allows for the applicability of the method to processed or mildly treated samples, where DNA is usually degraded and standard mtDNA barcoding methods are inappropriate.
An important issue to be taken into account is the need for harmonisation both in terms of analytical strategy and data analysis, i.e. benchmark in bioinformatics pipelines. To be able to properly analyse the results, the need of publicly available, curated databases are extremely important. The method proposed could complement existing fish identification strategies in establishing an efficient framework to detect and prevent frauds along the food chain, as well as in managing fisheries and conservation strategies.
Ethical approval
This article does not contain any studies with human participants or animals performed by any of the authors. Informed consent: Not applicable.
Supplemental Material
Download MS Word (6 MB)Acknowledgments
We are grateful to Dr Alain Maquet and Dr. Franz Ulberth from the JRC Geel for the critical review of the manuscript.
Disclosure statement
No potential conflict of interest was reported by the authors.
Additional information
Funding
References
- Asensio Gil L. 2007. PCR-based methods for fish and fishery products authentication. Trends Food Sci Technol. 18(11):558–566.
- Cawthorn DM, Steinman HA, Witthuhn RC. 2011. Establishment of a mitochondrial DNA sequence database for the identification of fish species commercially available in South Africa. Mol Ecol Resour. 11(6):979–991.
- Clark LF. 2015. The current status of DNA barcoding technology for species identification in fish value chains. Food Policy. 54(SupplementC):85–94.
- Cline SD. 2012. Mitochondrial DNA damage and its consequences for mitochondrial gene expression. Biochim Biophys Acta. 1819(9–10):979–991.
- Cutarelli A, Amoroso MG, De Roma A, Girardi S, Galiero G, Guarino A, Corrado F. 2014. Italian market fish species identification and commercial frauds revealing by DNA sequencing. Food Control. 37(SupplementC):46–50.
- Dalmasso A, Chiesa F, Civera T, Bottero MT. 2013. A novel minisequencing test for species identification of salted and dried products derived from species belonging to Gadiformes. Food Control. 34(2):296–299.
- Di Pinto A, Di Pinto P, Terio V, Bozzo G, Bonerba E, Ceci E, Tantillo G. 2013. DNA barcoding for detecting market substitution in salted cod fillets and battered cod chunks. Food Chem. 141(3):1757–1762.
- Everstine K, Spink J, Kennedy S. 2013. Economically motivated adulteration (EMA) of food: common characteristics of EMA incidents. J Food Prot. 76(4):723–735.
- [FAO] Food and Agriculture Organization. 2014. The state of world fisheries and aquaculture 2014. http://www.fao.org/3/a-i3720e.pdf.
- Fernandes TJR, Costa J, Oliveira MBPP, Mafra I. 2018. COI barcode-HRM as a novel approach for the discrimination of hake species. Fisheries Research. 197:50–59.
- Fernandes TJR, Silva CR, Costa J, Oliveira MBPP, Mafra I. 2017. High resolution melting analysis of a COI mini-barcode as a new approach for Penaeidae shrimp species discrimination. Food Control. 82(SupplementC):8–17.
- Ferrito V, Bertolino V, Pappalardo AM. 2016. White fish authentication by COIBar-RFLP: toward a common strategy for the rapid identification of species in convenience seafood. Food Control. 70(SupplementC):130–137.
- Filonzi L, Chiesa S, Vaghi M, Nonnis Marzano F. 2010. Molecular barcoding reveals mislabelling of commercial fish products in Italy. Food Res Int. 43(5):1383–1388.
- Garcia-Vazquez E, Perez J, Martinez JL, Pardinas AF, Lopez B, Karaiskou N, Casa MF, Machado-Schiaffino G, Triantafyllidis A. 2011. High level of mislabeling in Spanish and Greek hake markets suggests the fraudulent introduction of African species. J Agric Food Chem. 59(2):475–480.
- Gordon A, Hannon GJ Forthcoming 2010. Fastx-toolkit. FASTQ/A Short-Reads Preprocessing Tools (unpublished) http://hannonlab.Cshl.Edu/fastx_toolkit.
- Griffiths AM, Sotelo CG, Mendes R, Pérez-Martín RI, Schröder U, Shorten M, Silva HA, Verrez-Bagnis V, Mariani S. 2014. Current methods for seafood authenticity testing in Europe: is there a need for harmonisation? Food Control. 45:95–100.
- Hebert PD, Cywinska A, Ball SL, deWaard JR. 2003. Biological identifications through DNA barcodes. Proc Biol Sci. 270(1512):313–321.
- Hellberg RS, Kawalek MD, Van KT, Shen Y, Williams-Hill DM. 2014. Comparison of DNA extraction and PCR setup methods for use in high-throughput DNA Barcoding of fish species [journal article]. Food Anal Methods. 7(10):1950–1959.
- Helyar SJ, HaD L, de Bruyn M, Leake J, Bennett N, Carvalho GR. 2014. Fish product mislabelling: Failings of traceability in the production chain and implications for illegal, unreported and unregulated (IUU) Fishing. PLoS ONE. 9(6):e98691.
- Ivanova NV, Zemlak TS, Hanner RH, Hebert PDN. 2007. Universal primer cocktails for fish DNA barcoding. Mol Ecol Notes. 7(4):544–548.
- Jacquet J, Pauly D. 2008. Funding priorities: big barriers to small-scale fisheries. Conserv Biol. 22(4):832–835.
- Khaksar R, Carlson T, Schaffner DW, Ghorashi M, Best D, Jandhyala S, Traverso J, Amini S. 2015. Unmasking seafood mislabeling in U.S. markets: DNA barcoding as a unique technology for food authentication and quality control. Food Control. 56:71–76.
- Martin M. 2011 May. Cutadapt removes adapter sequences from high-throughput sequencing reads EMBnet. journal, [S.l.], 17(1):10–12. ISSN 2226–6089. Accessed: 2018 Dec 11. doi:https://doi.org/10.14806/ej.17.1.200.
- Mazzeo MF, Siciliano RA. 2016. Proteomics for the authentication of fish species. J Proteomics. 147:119–124.
- Miller D, Jessel A, Mariani S. 2012. Seafood mislabelling: comparisons of two western European case studies assist in defining influencing factors, mechanisms and motives. Fish and Fisheries. 13(3):345–358.
- Miller DD, Mariani S. 2010. Smoke, mirrors, and mislabeled cod: poor transparency in the European seafood industry. Front Ecol Environ. 8(10):517–521.
- Mendes R, Silva H. 2015. Control of seafood labelling in Portugal. Relatório Científico e Técnico do IPMA. p. 1–17.
- Mueller S, Handy SM, Deeds JR, George GO, Broadhead WJ, Pugh SE, Garrett SD. 2015. Development of a COX1 based PCR-RFLP method for fish species identification. Food Control. 55:39–42.
- Nedunoori A, Turanov SV, Kartavtsev YP. 2017. Fish product mislabeling identified in the Russian far east using DNA barcoding. Gene Reports. 8(SupplementC):144–149.
- Paracchini V, Petrillo M, Lievens A, Puertas Gallardo A, Martinsohn JT, Hofherr J, Maquet A, Silva APB, Kagkli DM, Querci M, et al. 2017. Novel nuclear barcode regions for the identification of flatfish species. Food Control. 79:297–308.
- Pepe T, Trotta M, Di Marco I, Anastasio A, Bautista JM, Cortesi ML. 2007. Fish species identification in surimi-based products. J Agric Food Chem. 55(9):3681–3685.
- Rasmussen RS, Morrissey MT. 2008. DNA-based methods for the identification of commercial fish and seafood species. Compr Rev Food Sci Food Saf. 7(3):280–295.
- Ratnasingham S, Hebert PDN. 2007. The Barcode of Life Data System (http://www.barcodinglife.org). Molecular Ecology Notes. 7(3):355–364.
- European Union. 2011. Regulation (EU). No 1169/2011. http://eur-lex.europa.eu/LexUriServ/LexUriServ.do?uri=OJ:L:2011:304:0018:0063:en:PDF
- European Union. 2013. Regulation (EU). No 1379/2013. http://eur-lex.europa.eu/LexUriServ/LexUriServ.do?uri=OJ:L:2013:354:0001:0021:EN:PDF
- Rehbein H. 2013. Differentiation of fish species by PCR-based DNA analysis of nuclear genes. Eur Food Res Technol. 236(6):979–990.
- Saull J, Duggan C, Hobbs G, Edwards T. 2016. The detection of Atlantic cod (Gadus morhua) using loop mediated isothermal amplification in conjunction with a simplified DNA extraction process. Food Control. 59(SupplementC):306–313.
- Strauss RE, Bond CE. 1990. Taxonomic methods: Morphology. In: Schreck CB, Moyle, editors. Methods for fish biology. American Fisheries Society; Bethesda, Maryland, p. 109–140.
- Tomás C, Ferreira IMPLVO, Faria MA. 2017. Codfish authentication by a fast Short Amplicon High Resolution Melting Analysis (SA-HRMA) method. Food Control. 71(SupplementC):255–263.
- Triantafyllidis A, Karaiskou N, Perez J, Martinez JL, Roca A, Lopez B, Garcia-Vazquez E. 2010. Fish allergy risk derived from ambiguous vernacular fish names: forensic DNA-based detection in Greek markets. Food Res Int. 43(8):2214–2216.
- [US FDA] US Food and Drug Administration. 2014. Seafood species substitution and economic fraud. http://www.fda.gov/Food/FoodScienceResearch/RFE/ucm071528.htm.
- Ward RD, Zemlak TS, Innes BH, Last PR, Hebert PDN. 2005. DNA barcoding Australia’s fish species. Philos Trans R Soc Lond B Biol Sci. 360(1462):1847–1857.
- Watanabe ME. 2013. Assembling an online tree of life of two million species. Bioscience. 63(1):64.
- Xiong X, D’Amico P, Guardone L, Castigliego L, Guidi A, Gianfaldoni D, Armani A. 2016. The uncertainty of seafood labeling in China: A case study on Cod, Salmon and Tuna. Marine Policy. 68(SupplementC):123–135.