Abstract
Fourier Transform Infrared (FTIR) spectroscopy coupled to chemometrics was developed to detect and quantify the adulteration in white and blue corn dough and white and blue corn tortilla, with corncob. The classification model, soft independent modeling of class analogy (SIMCA), showed 100% correct classification rate for adulterated samples from unadulterated ones. The best quantitative chemometric calibration model was developed with the partial least square (PLS) algorithm showing coefficient of determination (R2) between predicted and actual adulterant concentrations that range from 0.996 to 0.998 for all samples. Standard error of prediction (SEP) for the developed models ranged between 0.395 and 0.590 for all samples. The results showed that mid-infrared spectroscopy in conjunction with multivariate analysis can effectively be used to identify and quantify corncob in white and blue corn dough and in white and blue corn tortilla.
Se desarrollaron modelos quimiométricos acoplados a espectroscopia de infrarrojo medio por transformada Fourier (MIR–FTIR) para identificar y cuantificar la adulteración en masa y tortilla de maíz blanco y azul con olote. El modelo de clasificación, modelado suave independiente de analogía de clases (SIMCA) clasificó correctamente al 100% las muestras adulteradas con olote de las no adulteradas. El algoritmo de mínimos cuadrados parciales (PLS) desarrolló el mejor modelo quimiométrico cuantitativo con coeficientes de determinación (R2), entre concentraciones predichas por el modelo y concentraciones reales, de 0,998 para masa de maíz blanca, 0,996 para masa de maíz azul, 0,997 para tortilla de maíz blanca y 0,998 para tortilla de maíz azul. Los errores estándar de predicción (SEP) de los modelos desarrollados variaron entre 0,395 y 0,590 para todas las muestras. Los resultados mostraron que la espectroscopia de infrarrojo medio acoplada al análisis multivariable puede usarse de manera efectiva para identificar y cuantificar olote en masa de maíz blanca y azul, así como en tortilla de maíz blanca y azul.
Palabras claves: adulteración; FTIR; análisis multivariable; olote; masa de maíz; tortilla de maíz
Introduction
Corn (Zea mays L.) is probably the oldest cultivated cereal in the American continent, which is also currently grown in Europe, Africa, and Asia. This grain is the staple food for millions of people throughout the world (Serna-Saldívar, Gómez, & Rooney, Citation1990). In Mexico, Central America, and certain parts of Africa and China corn is the major source of energy for a large part of the general population. It has been estimated that in Mexico the annual consumption of corn is 253 kg per person (SAGARPA, Citation2009).
In Mexico and Central America, corn is transformed into tortillas through a process called nixtamalization (from the Náhuatl words nixtli and tamalli, which mean ashes and dough or masa, respectively). The corn is cooked in boiling water in the presence of lime (overnight steeping) and finally milled to obtain a wet dough (masa) which is used to produce tortillas, which are the principal source of calories, proteins, and calcium for low-income people. Also dough is used to make products like taco shells, tostadas, tamales, and snacks such as maize chips and tortilla chips or other snacks and Mexican foods (Caballero-Briones, Iribarren, & Peña, Citation2000).
Traditionally, white corn is used for tortilla making in Mexico. Also, in the central region of México, the consumption of blue corn tortillas occurs in communities and towns during special celebrations. However, blue corn tortillas are being sold with increasing frequency in large cities, generally at a higher price than the ordinary type. Among other reasons, people like blue tortillas because their flavor is sweeter and superior to that of ordinary ones (Salinas-Moreno, Martínez-Bustos, Soto-Hernández, Ortega-Paczka, & Arellano-Vázquez, Citation2003). Blue corn tortillas are prepared from pigmented maize, which are present in the majority of the 41 maize varieties described in México (Ortega, Sánchez, Castillo, & Hernández, Citation1991). The black, purple, and red tones that can be observed in pigmented maize are due to the anthocyanins, compounds which are present in the pericarp, in the aleurone layer or in both structures of the grain (Guzmán, Citation2007).
In spite of the great economical importance of corn tortillas as the daily staple food for many people in Mexico, no studies have been reported on the adulteration or fraudulent practices on tortilla by producers. Corncob has been reported as the most widely used adulterant in dough and tortilla (Guzmán, Citation2007).
Economic adulteration of food products involves the substitution of high-cost ingredients by cheaper and lower quality ones. Even though economic adulteration rarely presents a health hazard, it is an issue because it is a fraud for the consumer and undercuts legitimate industry prices (Gayo, Hale, & Blanchard, Citation2006). For this reason, there is a need for improved detection of adulterants to address the problem of economic adulteration in the food industry, especially for “value-added” products, such as white and blue corn tortilla.
Mexican government issued a regulation in 1988 that stated that in the elaboration of corn tortilla, it is prohibited the addition of corncob (Secretaría de Salud, Citation1988) because it modifies the rheological properties of tortilla, reduces its already low level of protein (3.9% and 4.5% for white and blue corn tortilla, respectively), and in general, it results in a poor quality dough and tortilla (Torres & Morales, Citation1997).
Although it is a suspected that corn dough and tortilla (white and blue) are adulterated by some producers with corncob (Guzmán, Citation2007), currently there is no simple and reliable methodology able to identify and quantify these adulterations, and therefore, there are only unproven claims. Rapid and reliable methods for detection of corn dough and tortilla adulteration are needed for product quality control. Ideal analytical methods for these purposes need to be specific, sensitive, rapid, economic, high throughput, and able to provide quantitative results.
In the last 10 years, the suitability of Mid Fourier Transform Infrared (MIR–FTIR) spectroscopy in conjunction with multivariate analysis for the detection of adulterants in foods has been demonstrated (Ellis et al., Citation2012; Karoui, Downey, & Blecker, Citation2010), for example, in meat (Meza-Márquez, Gallardo-Velázquez, & Osorio-Revilla, Citation2010), fish (Arvanitoyannis, Tsitsika, & Panagiotaki, Citation2005), chocolate (Che-Man, Syahariza, Mirghani, Jinap, & Bakar, Citation2005), honey (Gallardo-Velazquez, Osorio-Revilla, Zuñiga de Loa, & Rivera-Espinoza, Citation2009), and cakes (Syahariza, Che-Man, Selamat, & Bakar, Citation2005) among others applications. This method of detection possesses various advantages over the more traditional analytical methods: it is fast (tests can be carried out in 1–2 min), requires low sample volume, requires minimal or no sample preparation prior to analysis (allowing in situ or online studies), can be applied to liquids, gels, and amorphous solids and crystals, is environmental friendly because it does not require the use of reagents and solvents, and has a great potential for routine analysis (Dion & Saskia, Citation2008).
The aim of this study was to develop an analytical methodology to detect and quantify the adulteration of corn dough and tortilla (white and blue) with corncob using mid-infrared spectroscopy coupled to multivariate analysis.
Materials and methods
Chemicals: All analytical grade reagents were purchased from Sigma Chemical (St. Louis, MO), Merck (Darmstadt, Germany), and Fermont (Monterrey, México); food grade lime Ca(OH)2 was obtained from Realyt’s.
Samples: White and blue corn varieties, the most commonly types used in Mexico for dough and tortilla production, were collected from local markets in Mexico City.
Nixtamalization
The process of nixtamalization and baking of tortilla have been performed in the laboratory. The general method for tortilla preparation was as follows: 100 g of raw corn with and without the desired amount of corncob was placed in a 1 L flask and covered with a lime solution (2%). The mixture was heated to a temperature of 90°C for 90 min for white corn and 45 min for blue corn and then allowed to stand for about 12 hours (overnight). The supernatant liquor was discharged and the mixture of corn and cob was washed 4–5 times with water. The cooked mixture was then ground to fine dough in a commercial mill for 90 seconds. During grinding, 40 mL of water was added to obtain workable dough.
Preparation of adulterated samples
It has been reported that in order to develop a calibration model, apart from covering the desired range of the specific analyte, a minimum of 30–50 samples depending on the complexity of the problem must be considered (Chen, Cai, & Shao, Citation2007), to provide more information about the variability expected in unknown samples.
In this study, 25 samples of each mixture with corncob (white corn dough-cob, blue corn dough-cob, white corn tortilla-cob, and blue corn tortilla-cob), in concentration of corncob between 1% and 25% (w/w) in 2% steps, were prepared and divided into two sets: calibration and validation. The calibration set included 20 samples of each mixture and the validation set the remaining 5 samples.
Preparation of tortillas
About 30 g of corn dough either nonadulterated or adulterated with corncob were used to make a tortilla disk. The tortilla disk was heated for about 40 seconds on each side on a hot plate made of iron, in which average temperature was 230°C. During cooking, two different sides are formed: one that is a thin skin separated from the main body of the tortilla (side 1) and the other one that is the flat surface of the main body of the tortilla (side 2).
FTIR spectra acquisition
Spectra were collected at room temperature using a Perkin Elmer 1600 FTIR Spectrophotometer (Waltham, MA, USA) equipped with a deuterated triglycine sulfate detector for both nonadulterated and adulterated white and blue corn dough. The sampling station was equipped with an overhead attenuated total reflection (ATR) accessory, comprised of transfer optics within the chamber, through which infrared radiation was directed to a detachable zinc selenide (ZnSe) crystal mounted into a shallow through for sample containment. The crystal geometry was a 45° parallelogram with mirrored angled faces, with ten nominal internal reflections. The depth of penetration, which gives a measure of the intensity of the resulting spectrum, was 1.66 µm.
In the case of nonadulterated and adulterated tortilla a Perkin Elmer GX FTIR spectrophotometer (Norwalk, CT, USA) was used. This FTIR spectrophotometer is equipped with a deuterated triglycine sulfate detector and a horizontal diamond crystal ATR with one internal reflection coupled to an overhead pressure device.
The spectra were scanned at room temperature over the wave number interval of 4000–550 cm−1, averaging 64 scans at a resolution of 4 cm−1. Single beam spectra of the samples were collected against a background of air and presented in absorbance units. The acquired spectra were processed with Spectrum software version 3.01.00 (Perkin Elmer, Inc.). The mid-infrared spectra of each sample were obtained in triplicate and the results were recorded as the averages of these replicates. Samples of white corn dough, blue corn dough, and adulterated samples (white corn dough-cob and blue corn dough-cob) were applied to the ZnSe ATR accessory to give total crystal coverage. Spectra of white corn tortilla, blue corn tortilla, and adulterated samples (white corn tortilla-cob and blue corn tortilla-cob) were collected by placing the whole tortilla on side 2 (as explained in Section 2.3) onto the diamond ATR in three different zones of the surface applying pressure with the pressure applicator. A pressure applicator with a torque knob ensured the application of a reproducible pressure during all measurements.
To remove unwanted systematic variations, such as baseline shifts, scatter effects, and effects from uncontrolled external factors, prior to the statistical analysis (soft independent modeling by class analogy (SIMCA) and regression studies), data pretreatments were carried out applying ambient filters (CO2 and H2O), Savitzky–Golay filter with 9–13 smoothing points and normalization using the multiplicative scatter correction (MSC), which effectively removes baseline distortions that typically occur due to scattering in samples with differences in particle size (Beebe, Pell, & Deascholtz, Citation1998).
Discrimination between white corn dough, blue corn dough, white corn tortilla, blue corn tortilla, and adulterated samples by SIMCA
Classification methods such as SIMCA, partial least squares discriminant analysis (PLS-DA), k-nearest neighbors (KNN), and artificial neural networks (ANNs) are powerful tools for the characterization, differentiation, and classification of complex spectral data. They essentially differ in the way they achieve the classification. PLS-DA, KNN, and ANNs (discriminant techniques) have as its main drawback that samples are always classified into one on the given categories, even if they do not belong to any of them. On the other hand, SIMCA (class modeling technique) considers those objects that fit any category in the model, as part of the model, and classify as nonmembers those that do not. SIMCA model is a supervised pattern recognition classification technique and one of the most commonly used class-modeling tools in chemometrics. This technique requires a previous knowledge about the category membership of samples. Thus, the classification model is developed on a training set of samples with known categories, and the model performance is evaluated by the use of a validation set comparing predictions with true categories. SIMCA develops separate models (so-called disjointed class models or SIMCA hyperboxes) based on principal component analysis (PCA) for each training set category. The scores plot consisting of a projection of the original data onto principal component axes is used to visualize clustering among samples (sample patterns, groupings, or outliers). Class boundaries in multivariate space are assigned in a probabilistic manner, and class membership of training set samples or unknown samples may be predicted by their distances to these boundaries. Diagnostics to assess the quality of the data, included modeling power and discriminating power, that describe how well a variable helps the PCs to model variation and to classify the samples in the data set, respectively. (Berrueta, Alonso-Salces, & Héberger, Citation2007).
In this work, SIMCA method analysis was performed using the software Assure I.D Perkin Elmer version 3.0.0132. Eight classes were defined: (1) white corn dough, (2) blue corn dough, (3) white corn tortilla, (4) blue corn tortilla, (5) white corn dough-cob, (6) blue corn dough-cob, (7) white corn tortilla-cob, and (8) blue corn tortilla-cob. A total of 160 mid-infrared spectra (20 of each class) were imported into the SIMCA software and the class cutoff limits were set at the 1% level. Five additional spectra of each class were used to validate the SIMCA model obtained. Performance of the developed SIMCA model was evaluated through the interclass distance between the adulterated and nonadulterated samples, the three-dimensional principal component analysis scores plot, and the recognition and rejection rates of the samples.
Quantification of adulterant concentration
The relationships between each mid-infrared spectrum and reference data (percentage of adulterant) were determined by using Spectrum Quant+ software version 4.51.02 (Perkin Elmer, Inc., Waltham, MA, USA). The software includes principal component regression (PCR) and partial least square (PLS) algorithms.
PCR and PLS are the most widely used multivariate calibration methods in chemometrics. Both of these methods make use of the inverse calibration approach, where it is possible to calibrate for the desired component while implicitly modeling the other sources of variation. Estimation of the inverse calibration models involves the inversion of a data matrix. PCR and PLS solve this inversion matrix by replacing the original variables with linear combinations of the variables named factors or principal components (PCs) which are ordered in such a way that the first few contain most of the information that was spread all across of the original data. This reduction in complexity is helpful for exploration of large and complex data sets. Using too few factors can leave important information unmodeled, whereas using too many draws too much measurement noise from the data into the calibration model (Beebe et al., Citation1998). The difference between PCR and PLS is in how the factors (latent variables) or PCs are calculated (Beebe et al., Citation1998).
There are two steps in the PCR and PLS procedure, the first is the calibration and the second is the prediction that tests the calibration validity. Samples with known concentrations of the adulterant (in this case, corncob) were used as calibration sets; then, the concentrations of the adulterant of the validation set samples were directly calculated using the resulting models under the same conditions.
The evaluation of the calibration performance was estimated by computing both the standard error of calibration (SEC) after comparing the real percentage of corncob with the computed one, and the standard error of prediction (SEP), which gives an estimation of the prediction performance of the model obtained during the step of validation (Beebe et al., Citation1998).
The best model was chosen based on the smallest SEC and the highest coefficient of determination (R2) that indicates the strength and direction of the linear relationship between predicted values and reference values (Beebe et al., Citation1998). The prediction ability of the model was assessed using the smallest SEP of the validation set. In this work, an external validation was used to assess the calibration accuracy. The external validation set included 20 samples, 5 of each adulterated sample, ranging from 2–25% of corncob. This kind of validation is used when there are enough samples available to create separate (independent) training, calibration, and test sets. So, the test set is completely independent from the model building process (variable selection, parameter estimation, and determination of factors). This procedure has been applied successfully previously (Meza-Márquez et al., Citation2010).
Results and discussion
Interpretation of the MIR–FTIR spectra
shows an example of the MIR–FTIR spectra of white corn dough and white corn tortilla in the region of 4000–1000 cm−1. The assignments of the bands are included using previous reported studies (Kos, Lohninger, & Krska, Citation2003). The first peak (3400–3200 cm−1, maximum at 3296 cm−1) is assigned to O–H stretching vibration of water, and the two peaks at 2925–2854 cm−1 corresponds to C–H stretching bound of methyl and methylene groups. The slight shoulder at 1744 cm−1 can be attributed to C = O stretching vibration. The band at 1650 cm−1 is assigned to O–H stretching. The characteristics amide I bands of proteins at 1542 cm−1 (N–H bending mixed with C–N stretching) can be observed, and finally, the band at 1200–950 cm−1 is attributed to C–O stretching vibration of carbohydrates.
Figure 1. Mid-infrared spectra of white corn dough (dotted) and white corn tortilla (solid).
Espectro de infrarrojo medio de masa de maíz blanco (línea punteada) y tortilla de maíz blanco (línea sólida).
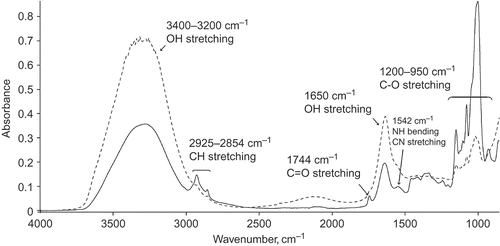
Spectrum of white corn tortilla () shows a decrease absorption at 3400–3200 cm−1 and 1650 cm−1 (O–H, stretching) due to loss water during the cooking process. The bands at 1200–950 cm−1 show an increase in absorbance due to the change in concentration of carbohydrates (C–O stretching). For blue corn dough and tortilla, same peaks and changes between dough and tortilla were observed (not shown).
Classification of corn dough and tortilla adulterated and nonadulterated using SIMCA
shows as an example the MIR–FTIR spectra of white corn tortilla adulterated with corncob used as calibration set. The region from 3700–1000 cm−1 shows a good correlation between changes in corncob percentages and spectral response. The spectra revealed differences in absorbance across the mid-infrared regions due to the change in concentration of the principal functional groups described in Section 3.1. Similar changes in the absorbance of the same bands (3700–1000 cm−1) proportional to the degree of adulteration were obtained with white corn dough, blue corn dough, and blue corn tortilla.
Figure 2. Mid-infrared spectra of white corn tortilla with different concentrations of corncob.
Espectro de infrarrojo medio de tortilla de maíz blanco con diferentes concentraciones de olote.
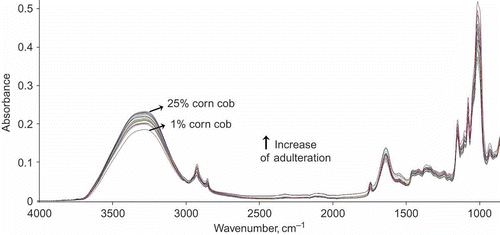
To develop the discrimination models between adulterated and nonadulterated samples, MIR–FTIR spectra were subjected to SIMCA analysis. (a–d) shows the 3D-PCA scores plot generated by the SIMCA models. This figure helps to visualize the class separation among pure samples (corn dough and tortilla) and adulterated samples. The boundary ellipse (hyperboxes) around each cluster represents the 99% confidence interval, and the points in each cluster represent the spectrum of each sample. As can be seen from (a–d), excellent cluster separation was obtained with the optimized model.
Figure 3. Three-dimensional principal component analysis scores plot of the populations derived from SIMCA: (a) white corn dough and adulterated white corn dough, (b) blue corn dough and adulterated blue corn dough, (c) white corn tortilla and adulterated white corn tortilla, and (d) blue corn tortilla and adulterated blue corn tortilla.
Análisis de componentes principales de tres dimensiones de las poblaciones derivadas de SIMCA: (a) masa de maíz blanco y masa de maíz blanco adulterado, (b) masa de maíz azul y masa de maíz azul adulterado, (c) tortilla de maíz blanco y tortilla de maíz blanco adulterado y (d) tortilla de maíz azul y tortilla de maíz azul adulterado.
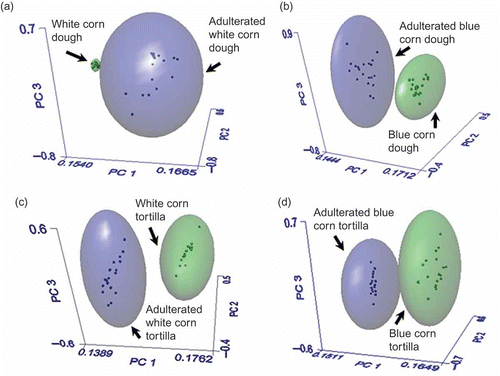
The cluster separation is a result of the interclass distances between the groups. SIMCA models provide interclass distances between clusters, the larger interclass distance between the two groups, the better separation. The interclass distance is calculated as the geometric distance from the clusters which represents 99% confidence that the particular object belongs to a class. As a rule of thumb, a distance of >3 indicates that the samples are well separated and hence different (He, Rodríguez-Soana, & Giusti, Citation2007). Interclass distances between the clusters of adulterated and nonadulterated samples are shown in . All interclass distances between the clusters were higher than 3.0, showing the capability of the developed SIMCA model to discriminate the spectral signal of nonadulterated samples with respect to adulterated ones.
Table 1. Interclass distance and percentages of recognition and rejection of the populations derived from SIMCA.
Distancia interclase y porcentajes de reconocimiento y rechazo de las poblaciones derivadas de SIMCA.
As mentioned before, an important characteristic of SIMCA is the ability not only to determine whether a sample belongs to any of the predefined categories, but also to determine if it does not belong to any class. shows the results of the classification performance of the optimized SIMCA model based on the percentage of recognition and rejection. As can be seen in , 100% correct classification rate for the adulterated and nonadulterated samples was observed. For example, for the white corn dough class, from the total of 40 adulterated and nonadulterated samples, 20 out of 20 white corn dough samples were correctly classified, and 20 out of 20 adulterated samples were rejected from that class, giving 100% recognition and rejection, respectively. The same rate of recognition and rejection was obtained for the other defined classes.
The predictive performance of the SIMCA model developed was evaluated by applying it to 40 additional spectra, 5 of each class, with different adulterant concentration ranging from 2% to 25% that served as the external validation set. All the samples were recognized and classified correctly as can be seen in . Total distance ratio for each validation sample was less than 1.0, indicating that sample was correctly classified by the model. Residual distance showed a maximum of 1.54, which is low enough for a correct classification. Residual ratio must be as low as possible; since a large residual distance indicates that the sample contains a source of variation which has not been previously encountered. The results on demonstrate the capability of the SIMCA model developed to correctly classify the eight groups studied independently on the adulterant concentration with a 99% confidence limit.
Table 2. External validation data for the SIMCA model developed.
Datos de validación externa del modelo SIMCA.
Quantification of the adulterant concentration of dough and tortilla (white and blue)
The spectral data for each calibration set were subjected to PCR and PLS multivariate analysis to obtain quantitative models for each adulterated samples (white corn dough-cob, white corn tortilla-cob, blue corn dough-cob, and blue corn tortilla-cob). shows the optimal number of factors for PLS and principal components for PCR, the coefficient of determination (R2), and the standard error of calibration (SEC) of the optimized calibration models obtained. Best models were selected based on the highest R2 and the lowest SEC shown in italics and bold in .
Table 3. Calibration and validation data of the quantitative models developed with PCR and PLS algorithms to predict corncob concentration in white dough, blue dough, white corn tortilla, and blue corn tortilla.
Datos de calibración y validación de los modelos cuantitativos desarrollados con los algoritmos PCR y PLS para predecir la concentración de olote en masa de maíz blanco y azul, tortilla de maíz blanco y tortilla de maíz azul.
As shown in , the statistical parameters used to select the best predictive models (R2 and SEC), indicated that the best models were obtained with PLS algorithm, since R2 were higher for all adulterated samples while SEC values were lower than those obtained for the PCR algorithm.
Once it was established that the best calibration models were obtained with the PLS algorithm for each adulterated sample, they were applied to the validation set in order to provide an estimate of the accuracy of predictions. shows that the values of R2 between predicted and actual adulterant concentration of the external samples was higher than 0.99. Based on Shenk and Westerhaus (Citation1996), a value of R2 between 0.82 and 0.90 indicates good prediction, and a value for R2 above 0.91 is associated with an excellent prediction. SEP values obtained for the external samples () were very low (0.395–0.590), indicating the predicting ability of the model.
(a–d) shows the correlation between prediction and actual percentages of corncob in the validation samples. As can be seen in independently of their adulterant concentrations, which range between 2% and 25% for all samples, the predicted concentrations fall very close to the equal concentration line, demonstrating the good prediction capability of the models.
Figure 4. Partial least squares regression (PLSR) validation plots for corncob percentages in (a) white corn dough, (b) blue corn dough, (c) white corn tortilla, and (d) blue corn tortilla.
Valores predichos contra valores actuales de porcentaje de olote in (a) masa de maíz blanco, (b) masa de maíz azul, (c) tortilla de maíz blanco y (d) tortilla de maíz azul para las muestras de validación determinadas por la regresión de mínimos cuadrados parciales (PLSR).
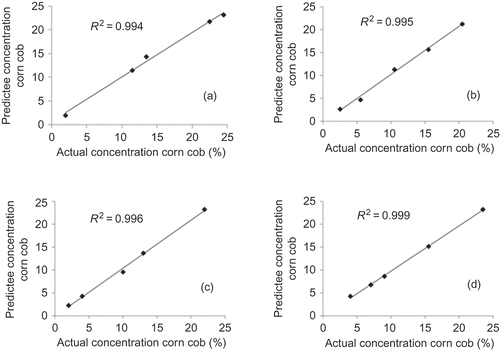
Based on these results, the PLS models developed in this work were able to predict the percentage of adulterant (corncob) in external in samples different from those used for the development of the calibration models.
Conclusion
Mid-infrared spectroscopy in combination with multivariate analysis is a fast, efficient, accurate, low-cost, and environmentally friendly tool for both the discrimination and quantification of corncob as adulterant in corn dough and corn tortilla (white and blue). Both analyses (qualitative and quantitative) can be performed in less than 5 min and only require less than 5 g of sample.
This study could be the basis for carrying out research related to the establishment of a fast and accurate analytical method to ascertain corn dough and tortilla quality. Further work must be carried out in commercial samples from tortilla selling premises, in order to confirm the applicability of the models developed on a wider variety of samples of corn dough and tortilla.
Acknowledgements
Financial support from the Consejo Nacional de Ciencia y Tecnología (CONACyT) and the Secretaría de Estudios de Posgrado e Investigación del Instituto Politécnico Nacional de México (SIP-IPN) is greatly appreciated.
References
- Arvanitoyannis, I. S., Tsitsika, E. V., & Panagiotaki, P. (2005). Implementation of quality control methods (physico-chemical, microbiological and sensory) in conjunction with multivariate analysis towards fish authenticity. International Journal of Food Science and Technology, 40, 237–263.
- Beebe, K. R., Pell, R. J., & Deascholtz, M. B. (1998). Chemometrics: A practical guide. New York, NY: John Wiley & Sons Inc.
- Berrueta, L. A., Alonso-Salces, R. M., & Héberger, K. (2007). Supervised pattern recognition in food analysis. Journal of Chromatography. A, 1158, 196–214.
- Caballero-Briones, F., Iribarren, A., & Peña, J. L. (2000). Recent advances on the understanding of the nixtamalization process. Superficies Y Vacío, 10, 20–24.
- Che-Man, Y. B., Syahariza, Z. A., Mirghani, M. E. S., Jinap, S., & Bakar, J. (2005). Analysis of potential lard adulteration in chocolate and chocolate products using Fourier Transform Infrared Spectroscopy. Food Chemistry, 90, 815–819.
- Chen, C., Cai, W., & Shao, X. (2007). An Adaptive for selecting representative calibration samples in the continuous wavelet domain for near-infrared spectral analysis. Analytical and Bioanalytical Chemistry, 387, 1041–1048.
- Dion, M. A. M., & Saskia, M. (2008). An overview of analytical methods for determining the geographical origin of food products. Food Chemistry, 107, 897–911.
- Ellis, D. I., Brewster, V. L., Dunn, W. B., Allwood, J. W., Golovanov, A. P., & Goodacre, R. (2012). Fingerprinting food: Current technologies for the detection of food adulteration and contamination. Chemical Society Reviews, 41, 5706–5727.
- Gallardo-Velázquez, T., Osorio-Revilla, G., Zuñiga de Loa, M., & Rivera-Espinoza, Y. (2009). Application of FTIR-HATR spectroscopy and multivariate analysis to the quantification of adulterants in Mexican honeys. Food Research International, 42, 313–318.
- Gayo, J., Hale, S. A., & Blanchard, M. S. (2006). Quantitative analysis and detection of adulteration in crab meat using visible and near-infrared spectroscopy. Journal of Agricultural and Food Chemistry, 54, 1130–1136.
- Guzmán, R. G. (2007). Incremento en los precios del maíz y la tortilla en México. Revista Latinoamericana De Economía, 38(151), 103–126.
- He, H., Rodríguez-Soana, L. E., & Giusti, M. M. (2007). Midinfrared spectroscopy for juice authentication-rapid differentiation of commercial juices. Journal of Agricultural and Food Chemistry, 55, 443–452.
- Karoui, R., Downey, G., & Blecker, C. (2010). Mid-infrared spectroscopy coupled with chemometrics: A tool for the analysis of intact food systems and the exploration of their molecular structure-quality relationships-a review. Chemical Reviews, 110, 6144–6168.
- Kos, G., Lohninger, H., & Krska, R. (2003). Development of a method for the determination of Fusarium Fungi on corn using mid-infrared spectroscopy with attenuated total reflection and chemometrics. Analytical Chemistry, 75(5), 1211–1217.
- Meza-Márquez, O., Gallardo-Velázquez, T., & Osorio-Revilla, G. (2010). Application of mid-infrared spectroscopy with multivariate analysis and soft independent modeling of class analogies (SIMCA) for the detection of adulterants in minced beef. Meat Science, 86, 511–519.
- Ortega, P., Sánchez, R. A., Castillo, G., & Hernández, J. M. (1991). Estado actual de los estudios sobre maíces nativos de México. In P. R. Ortega, H. G. Palomino, G. F. Castillo, H. V. A. González, & M. M. Livera (Eds.), Avances en el estudio de los recursos fitogenéticos de México (pp. 161–196). A. C. México: SOMEFI.
- SAGARPA. (2009). Situación actual y perspectivas del maíz en México 1996–2010. México, D.F: SAGARPA.
- Salinas-Moreno, Y., Martínez-Bustos, F., Soto-Hernández, M., Ortega-Paczka, R., & Arellano-Vázquez, J. L. (2003). Effect of alkaline cooking process on anthocyanins in pigmented maize grain. Agrociencia, 37, 617–628.
- Secretaría de Salud. (1988). Reglamento de la Ley General de Salud en Materia de Control Sanitario de Actividades, Establecimientos, Productos y Servicios. México, D.F: Diario Oficial de la Federación.
- Serna-Saldívar, S. O., Gómez, M. H., & Rooney, L. W. (1990) Technology, chemistry and nutritional value of alkaline-cooked corn products. In Y. Pomeranz (Ed.), Advances in cereal science and technology (Vol. X) (p. 243). St. Paul, MN: American Association of Cereal Chemists.
- Shenk, J. S., & Westerhaus, M. O. (1996). Calibration the ISI way. In A. M. C. Davies & P. C. Williams (Eds.), Near infrared spectroscopy: The future waves. Chichester, UK: NIR Publications.
- Syahariza, Z. A., Che-Man, Y. B., Selamat, J., & Bakar, J. (2005). Detection of lard adulteration in cake formulation by Fourier Transform Infrared (FTIR) Spectroscopy. Food Chemistry, 92, 365–371.
- Torres, G., & Morales, M. (1997). Maíz-Tortilla Políticas y Alternativas. Editorial. México: UNAM.