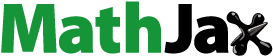
ABSTRACT
Chemometric tools and GC-MS were employed to detect the adulteration of camellia seed oil. In the training set, 65 samples were formulated by blending pure camellia seed oil with varying concentrations of soybean, peanut and rapeseed oil and their fatty acid contents were used in chemometric analysis. Principal Component analysis revealed the distribution patterns of samples that showed a clear stratiform agglomeration according to varying amount of camellia seed oil. Fisher Discriminant Analysis (FDA) was combined to establish the classification models and the correct classification rate of cross-validation model and original model reached 92.5% and 82.5%, respectively. To test the quality of the model, a new test set was prepared and the results showed a high accuracy of 88%. This was indicated that the developed discrimination model established by PCA plus FDA was efficient in predicting the presence of other low-price edible oils in camellia seed oils.
Resumen
El presente estudio empleó herramientas quimiométricas y GC-MS para detectar la adulteración del aceite de semilla de camelia. Con este objetivo, en el conjunto de entrenamiento se formularon 65 muestras, en las que se mezcló aceite de semilla de camelia puro con concentraciones variables de aceite de soya [soja], cacahuate [maní] y colza, utilizándose sus contenidos de ácidos grasos para realizar el análisis quimiométrico. El análisis de componentes principales permitió identificar los patrones de distribución de las muestras. Estas mostraron una clara aglomeración estratiforme de acuerdo con la cantidad variable de aceite de semilla de camelia que poseían. Asimismo, para establecer los modelos de clasificación, se realizó el análisis discriminante de Fisher (FDA), mediante el cual se constató que la tasa de clasificación correcta del modelo de validación cruzada y del modelo original alcanzó 92.5% y 82.5%, respectivamente. A fin de comprobar la calidad del modelo se preparó un nuevo conjunto de pruebas, constatándose una alta precisión en los resultados, de 88%. El conjunto de resultados hizo posible comprobar que el modelo de discriminación desarrollado, establecido por PCA más FDA, es eficiente para determinar la presencia de aceites comestibles de bajo precio en los aceites de semilla de camelia.
1. Introduction
In China, especially the southern provinces, the “Eastern Olive Oil,” camellia seed oil, is commonly regarded as popular edible vegetable oil with high nutritional and medicinal value, high storage stability, and high price (Su et al., Citation2014). Ninety percent of the fatty acids content of camellia seed oil are unsaturated fatty acids (UFAs) and Oleic acid (C18:1) is the major MUFA that having the highest percentages of 68%-87% (GB/T11765-Citation2018). Besides, camellia seed oil also contains various lipid accompaniments, such as tocopherols, phytosterols, squalene, etc. (Li et al., Citation2011). Previous study has summarized the effects of camellia seed oil for preventing cardiovascular diseases, arteriosclerosis, strengthening the immune system, and even treating a burn (Dou et al., Citation2018; M. T. Zhu et al., Citation2019). All those properties plus the low yield of the camellia seed oil mean that it is sold at a much higher price than other vegetable oils. In Chinese oil market, the price of pure camellia seed oil are at least 80 yuan per liter, which is much higher than pure soybean oil (6–18 yuan per liter), peanut oil (13–34 yuan per liter) and rapeseed oil (10–24 yuan per liter) (Li & Wang, Citation2019). Adulteration of camellia seed oil is a common and special phenomenon in oil market. It is barely detectable by the human eyes or nose that only a small amount (<10%) of other oils was added because of the strong flavor of camellia seed oil. Some unscrupulous businesses were toward seeking more profit by adding as much other oil as possible. According to the data statistics (Mingjun Li et al., Citation2019), the consumption amount of edible oil in 2018 reached 3440 million tons, where 43.1% was soybean oil, 22.1% was rapeseed oil and 7.7% was peanut oil, meaning the three oils have high market share and can be easily obtained. Thus, they were chosen as the adulteration oils. On the other hand, the Chinese government has developed and implemented a standard (GB 2716–Citation2018). It is clearly defined that edible blend oil was consist of two or more kinds of edible vegetable oils, and the oil type and proportion instructions it contained required to appear in the oil product’s label. But it is also a very difficult job to analyze the presence of the other oils in camellia seed oil. Therefore, the development of analytical methodologies to identify the camellia seed oil has been the subject of academic research.
As we all know, fatty acid is the basic unit of edible oil, and it is listed as a necessary testing item in the quality standards for edible oils. Edible oil is a highly complex system containing several types of fatty acids. The composition of different types of edible oil may show significant differences while somehow maintaining some degree of similarity (Hajimahmoodi et al., Citation2005). Palmitic acid (C16:0), stearic acid (C18:0), oleic acid (C18:1), linoleic acid (C18:2) are the common fatty acids existing in almost edible oils, but in some edible oils may contain characteristic fatty acids not found elsewhere (Z. Wang, Citation1999). As described in many literatures, using characteristic fatty acid to analyze whether the edible oil is adulterated or not is considered to be effective for the single targeted adulteration. However, it is difficult to identify multiple or untargeted adulterants. Thus, it is necessary to collect information on the composition of fatty acids as thoroughly as possible. GC-MS has been obtained the universal approval to evaluate the fatty acid profile of vegetable oils due to the following character: simple operation, high sensitivity, excellent separation, wide application, good reproducibility (H. Zhu et al., Citation2010).
Currently, chemometrics are considered as the usefulness application tools to analyze the richness of information obtained by GC-MS. In many literatures, the common chemometric methods, including One-way analysis of variance (Tfouni et al., Citation2017), Partial Least Square-Regression (Jiménez-Carvelo et al., Citation2017), Principal Component analysis (PCA) (Shi et al., Citation2018), Fisher Discriminant Analysis (FDA) (Casale et al., Citation2010), Genetic algorithm (Ruiz-Samblás et al., Citation2012) and so on, have been proven feasible with reasonable results for each technique. Meanwhile, combination of more than one chemometric tools has already been employed to detect the adulteration in blends. Wang et al. (Citation2019) used PCA partial least squares (PLS) and principal component regression (PCR) methods to detecting sesame oil adulteration based on low-field nuclear magnetic resonance data. Application of the PCA and discriminant analysis using chromatographic data has already been employed to detect adulteration and classification of cheeses. Romdhane Karoui et al. (Citation2007) have used PCA and factorial discriminant analysis to create classification models to identify the geographical origin of the investigated cheeses. Herman-lara et al. (Citation2019) also used variance analyses and discriminant function analysis to determine the geographic origin of the goat cheeses.
In this paper, samples of pure camellia seed oil and their mixtures with three different low-price oils (soybean oil, peanut oil, and rapeseed oil) in various concentrations were analyzed by GC–MS, and the data obtained were analyzed by chemometrics. The classification of samples was established as described in and it was using the camellia seed oil content level as evaluation index. The combination of PCA and FDA is commonly applied to fault detection. In this work, PCA was used to reduce the dimensionality of complex chromatographic data while preserving the significant information and eliminating the effects caused by information superposition (Li bo., Citation2020). But it does not consider the information between different classes of oil samples. This problem is incorporated by the FDA. It was combined to establish the classification models of samples according to their content level of camellia seed oil. The combination of the two methods can improve the precision of classification and reduce the response time. To ensure the PCs obtained from PCA can reduce the dimensionality of the original data to simplify models without losing important information, stepwise discriminant analysis, and reduced classification model were used as checking tools. Meanwhile, the new test set was also used to evaluate the quality of the classification models.
Figure 1. Classification of camellia seed oil. All samples can be divided into six categories (A to F) according to the content of camellia seed oil.
Figura 1. Clasificación del aceite de semilla de camelia. Todas las muestras pueden dividirse en seis categorías (A a F) según su contenido de aceite de semilla de camelia
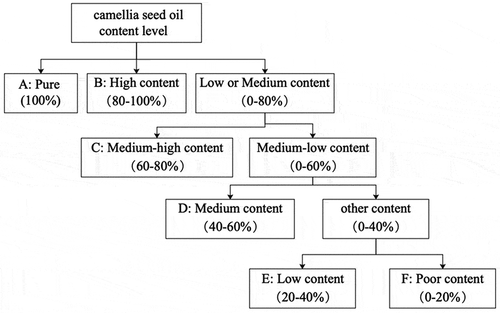
The objective of this study was to create statistical models to confirm the content level of camellia seed oil in samples based on chromatographic data. For one hand, as long as the model showed that the camellia seed oil content of the sample was less than 100%, it is certainly not a pure camellia seed oil. Rapid and accurate identification of camellia seed oil content is the key to control the adulteration of pure camellia seed oil products. For the other hand, it is able to investigate the honesty of the manufacturer and played the supervision role in the quality control of edible blend oil by compared the consistency of camellia seed oil content level showed in the label or that determined from the statistical model. In general, the proposed models herein try to offer some elicitation to solve the problem that it is difficult to establish uniform examination standards for edible blend oil and it also provides powerful technical support for quality inspection department to standardize the oil market, and earnestly protect consumers’ rights and interests.
2. Experimental section
2.1. Sample preparation and classification
Using the Soxhlet method, all pure oil samples were extracted from the camellia seed, soya bean, peanut, rapeseed, which were provided by Experiment Base of Hunan Agricultural University (Hunan, China), except for Conyda camellia seed oil, which was commercially available. A total of 11 samples of pure camellia seed oil and one each sample of pure soybean oil, peanut oil, rapeseed oil were collected, respectively.
Our previous studies have established the Fatty acids standard fingerprints of camellia seed oil which may represent the common characteristics of seed oil (Wu et al., Citation2013). By calculating the similarity degree using the vector included-angle cosine method, we found that the similarity among all the 11 pure camellia seed oil samples in our study and the Fatty acids standard fingerprints of camellia seed oil were higher than 0.9993. Finally, the Conyda camellia seed oil, which was relatively easily acquired was used to for further experiment.
To simulate as many content conditions as possible, camellia seed oil samples with different content level were prepared by adding the soybean oil, peanut oil and rapeseed oil to Conyda camellia seed oil at the ratio of 5%, 15%, 25%, and 50% v/v and were labeled according to . The setting of the proportion is mainly based on the price cost and the proportion of blended oil on the market. In total 65 samples were prepared, including 54 adulterated oil samples and 11 pure camellia seed oil samples. All of these samples constituted a training set required for the classification model. Meanwhile, another group called the test set as shown in were created with the same raw material to test the quality of the statistic model created.
Table 1. Preparation of the training set.
Tabla 1. Preparación del conjunto de entrenamiento
Table 2. Preparation of the test set.
Tabla 2. Preparación del conjunto de pruebas
2.2. Gas chromatographic-mass spectrum (GC–MS) analysis
Oil samples (approximately 100 mg) were methyl esterification by using the acid-alkali combination method (Wu et al., Citation2013). General profiles of all samples were obtained using electron impact (EI)/MS. Analyses (Tang, Citation2011) were conducted on an automated GC–MS Shimadzu equipment model GCMS-QP2010 using a capillary column (100 m × 0.25 mm i.d. × 0.2 μm, HP-88), with helium as the carrier gas, at a constant flow rate of 1.04 ml/min. Sample aliquots of 0.5 μL were injected in the split mode (20:1). Analyses were performed under the following conditions: the column was kept at 120°C for 1 min and then heated to 175°C at 10°C/min for 10 min. After that, the temperature was increased at a rate of 5°C/min up to 210°C for 5 min and finally to 230°C at 5°C/min. The final temperature was kept constant for 15 min. The mass spectrometer working in electron ionization mode at 70 eV was operated in full-scan mode (m/z 50–600).
2.3. Fatty acids analysis
The samples were identified by comparing the retention times of the sample peaks with those of a mixture of FAME standards (Sigma–Aldrich, PA, USA) (Quintanilla-Casas et al., Citation2020). The relative percentages of the constituents were determined by area normalization. Two criteria were used to select fatty acids peaks for statistical analysis: (a) Base on the current national standard GB/T 11765–2018 & 1535–2017 & 1534–2017 & 1536–2004 for camellia seed oil & soya bean oil & peanut oil & rapeseed oil, only the kinds of fatty acids coexisted in all the 4 edible oils were considered. (b) Only fatty acids with the similarity to the reference spectra larger than 90% were considered.
3. Data analysis
3.1. Principal component analysis (PCA)
Dimension reduction is an important technique in data mining and exploratory data analysis. It is an unsupervised learning process like people learning something just by observing the world around them and understanding how things work. It separates pattern and characteristic from data which need no prior knowledge and it is used to guide the selection of suitable learning strategies. Feature Extraction is the critical step in the process.
As the most widely adopted method for feature extraction, PCA can effectively reduce computational complexity (Holland, Citation2008; Liu et al., Citation2012). It also offers a better expressiveness for helping the samples be dispersed as much as possible in the lower dimensional space, while maintaining the difference among samples shown in the raw space (Croux et al., Citation2013). It is easier to understand the distribution under the visual inspection and is benefit for the subsequent analysis. In our work, standardized samples data were adopted for PCA using SPSS 19.0 and origin 2018 software.
3.2. Fisher discriminant analysis (FDA)
The fundamental idea of FDA is to project high dimensional data to the best plane, which promotes better separability of the sampled data. It can maximize the separation between inter-class distance while minimizing the within- class scatter. Then the location of each sample point and the distance from each sample to the centroids of each class can be calculated, discovering and learning the intrinsic laws and describing them using mathematical models (C. Q. Wang, Citation2016; Xu et al., Citation2018). For unknown samples, data are taken into the mathematical model and then identified according to the function value. FDA were examined with using SPSS 19.0 software.
Meanwhile, the use of FDA required an ideal number of variables to produce the best classification without overfitting (Yu Citation2011). Stepwise discriminant analysis can use the partial F-test values to select the variables with the ability to reduce the within class variance and increase the low between variance among different classes (Siddiqi et al., Citation2015). Reducing training set can be used to verified accuracy of the model. So stepwise discriminant analysis and reduced classification model were used to validate before FDA in our work.
4. Results and discussion
4.1. GC-MS
presents the chromatograms of pure camellia seed oil and a part of mixture used in this study, which illustrates the complexity of fatty acids composition. As we notice, almost all the fatty acids peaks flowed out between 20 and 46 min of the chromatographic run. Many of the compounds, which were confirmed by the similar retention time and identification in mass spectra, presented in camellia seed oil and also appeared in mixtures. So it is not reliable enough to identify camellia seed oil adulteration only based on one or two kinds of fatty acids composition. We must collect enough information to identify the subtle differences between pure camellia seed oil and samples containing other edible oil.
Figure 2. The chromatogram of camellia seed oil samples: (a) pure camellia seed oil, (b) pure camellia seed oil plus 25% soya bean oil and 25% rapeseed oil, (c) pure camellia seed oil plus 25% rapeseed oil and 25% peanut oil, (d) pure camellia seed oil plus 25% peanut oil and 25% soya bean oil, (e) pure camellia seed oil plus 25% rapeseed oil and 25% peanut oil and 25% soya bean oil.
Figura 2. Cromatograma de las muestras de aceite de semilla de camelia: (a) aceite de semilla de camelia puro, (b) aceite de semilla de camelia puro más 25% de aceite de soya y 25% de aceite de colza, (c) aceite de semilla de camelia puro más 25% de aceite de colza y 25% de aceite de cacahuate, (d) aceite de semilla de camelia puro más 25% de aceite de cacahuate y 25% de aceite de soya, (e) aceite de semilla de camelia puro más 25% de aceite de colza y 25% de aceite de cacahuate y 25% de aceite de soya
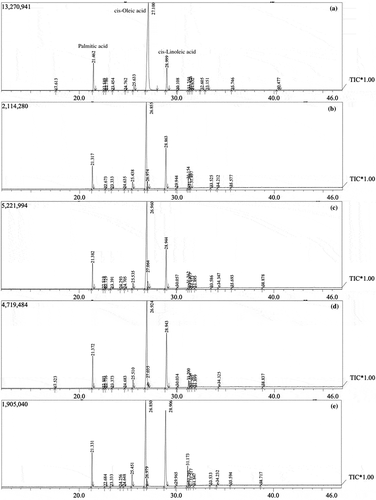
From ), camellia seed oil contained high content of cis-oleic acid, cis-linoleic acid, and palmitic acid. Similar trend was also found in all the mixture from b) to e). Although samples b) to e) were different from each other, visual inspection of the chromatograms of each sample showed non-significantly difference. Because of the complex feature of fatty acids composition and some invisible change between pure camellia seed oil and mixture, any attempt to identify the presence of other edible oil in pure camellia seed oil must consider enough effective peaks. Thus, nine kinds of fatty acids (myristic acid, palmitic acid, palmitoleic acid, stearic acid, cis-oleic acid, linoleic acid, arachidic acid, α-linolenic acid, arachidonic acid) from the samples were selected for the statistical calculations according to the criteria described in the part of “Fatty acids analysis.” Detailed information were all presented in the supplementary material (Table S1) of this manuscript.
4.2. PCA
presents the scree plot of the nine PCs calculated from the covariance matrix. It explained about 98.72% of the whole data variance captured by the first five principal components, while the fifth principal components still contributed 4.295% of the variance. The curve became flat from the sixth PC, indicating that the succeeding components had no significant variability and contribute less to the model. As shown in the , the first PC (the highest point) accounted for most of the variability in the data. A distinct bend occurred in the second PC. It is indicated that the further restriction was appropriate for the first two PCs (Constantin, Citation2014).
Figure 3. Scree plot of the eigenvalues after PCA. The first five components explained about 98.72% of the whole data variance.
Figura 3. Gráfico de los valores propios tras el PCA. Los cinco primeros componentes explicaron alrededor de 98.72% de la varianza total de los datos
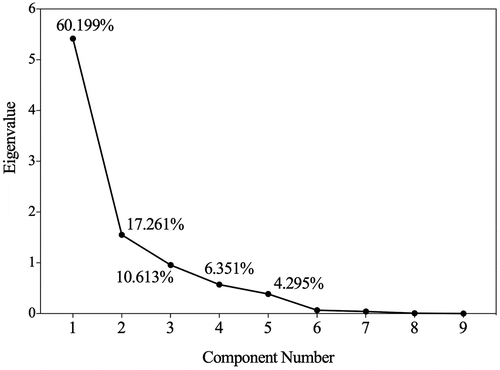
We checked the visual clustering of all 65 samples performed in the score plot of PC1 versus PC2 (). Samples grouped together in the same circle were identified according to the letters given in and the pure camellia seed oil were labeled as “A.” The pure camellia seed oil samples (A) were located relatively far from other adulteration samples, meaning using PC1 alone was enough to separate it. It was easy to distinguish between adulteration and non-adulteration using PCA. This method was also used in the detection of cheese (Kim et al., Citation2014) and cow ghee (Ayari et al., Citation2014) and so on. As for adulteration samples although they lined up much clustered, the groups could still be characterized by different circles (B to F). On the one hand, the samples tended to cluster items according to the camellia seed oil content level, where samples with the higher content level clustered more towards group A. On the other hand, these groups became more scattered with decreasing concentration of camellia seed oil in sample because they needed more space to contain the distributed samples which belongs to the same content level. Moreover, the group B, C, D, and E could be separated clearly. However, there is a relatively large overlap between group E and F.
Figure 4. Score plot of PC1 and PC 2 after analysis on fatty acids of all 65 samples. (A, 100% camellia seed oil; B, 80–100% camellia seed oil; C, 60–80% camellia seed oil; D, 40–60% camellia seed oil; E, 20–40% camellia seed oil; and F, 0–20% camellia seed oil).
Figura 4. Gráfico de puntuación de PC1 y PC2 tras el análisis de los ácidos grasos presentes en las 65 muestras. (A, 100% de aceite de semilla de camelia; B, 80–100% de aceite de semilla de camelia; C, 60–80% de aceite de semilla de camelia; D, 40–60% de aceite de semilla de camelia; E, 20–40% de aceite de semilla de camelia; y F, 0–20% de aceite de semilla de camelia)
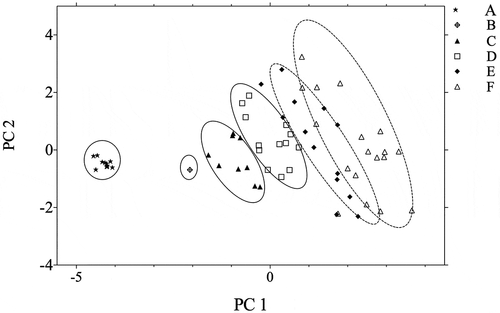
In this part, we can know that samples may cluster into different groups in the PC1 ~ PC2 space and the differentiations between groups were clear. shows enough information to distinguish the samples according to the content level of camellia seed oil. However, for samples with camellia seed oil concentration less than 40% (v/v), the results were not very desirable due to their similar fatty acid compositions.
4.3. PCA and FDA
The ideal number of PCs is the smallest number of PCs which produces the best classification without overfitting. To do this, all six PCs in the training set were filtered with a stepwise discriminant analysis method in terms of their content level classification discriminant ability. Through the statistical test of stepwise regression analysis, the PC1 and PC2 were selected due to the p-value<0.05, and with high regression coefficient (adjusted R2 = 0.955). Meanwhile, the variance proportion for the two discriminant functions established by PC1 and PC2 was 99.9% and 0.1%, respectively (Table S2). This means that the two discriminant functions may explain 100% of the data information in the original data. Moreover, the percentage of the training samples correctly classified reached 87.7%, with the accuracy of the cross-validation prediction results also reached 83.1% (Table S4). All these indicated that PC1 and PC2 were suitable for the subsequent analysis.
Furthermore, we created the reduced training set and the reduced test set by using the method of “leave-p-out.” The data set is divided equally, and P samples can be left as a reduced test set and the rest as a reduced training set. The “reduced classification model” to evaluated the ideal number of PCs (Wang et al., Citation2019; Quintanilla-Casas et al., Citation2020). Among 65 samples, 25 samples were chosen as reduced test set and 40 samples were chosen as reduced training set. Detailed information was all presented in the supplementary material (Table S3& Table S4) of this manuscript. presents the correctly classified percentage of samples by the reduced training set and reduced test set for various numbers of PCs. We can see that the percentage of samples correctly classified grew largely as the number of PCs increased from 1 to 2. Then, the percentage tended to stabilize or even decrease a bit with increase of the number of PCs. In this test, the percentage showed a fine goodness of model fit for the original part, but not for the cross-validation part. This finding is consistent with the literatures (Sun et al., Citation2018; Vinicius et al., Citation2007). Overfitting might be the reason (Nansen et al., Citation2013). Accordingly, the ideal number of PCs was 2, which, was consistent with the result of the previous analysis.
Table 3. Percentage of samples correctly classified by PC plus FDA.
Tabla 3. Porcentaje de muestras correctamente clasificadas por PC más FDA
EquationEquations (1(1)
(1) –Equation6
(6)
(6) ) were the Canonical Discriminant Functions calculated with PC1 and PC2. When the score of PC about an unknown sample was fed into the six equations, the classification corresponding to the maximum F value was the quality grade of the sample. We applied the six equations to the test set () to verify the accuracy of the discriminant model, and the results were shown in . As we expected, this method was successful with a discrimination accuracy of 88% for the test set. Samples belonging to Group A, B, D, and F can be identified correctly (100%). Although a few samples in group C were misclassified as group B, what we should be concerned about is the group E which had the highest misclassification error ratio. Moreover, all the misclassified samples became part of group D. One explanation for this was because the camellia seed oil concentration in group E seemed to be closer to that in group D. In general, our method was robust and efficient, and applicable for the quality analysis of camellia seed oil.
Table 4. Test set classification by PCA plus FDA.
Tabla 4. Clasificación del conjunto de pruebas mediante PCA más FDA
4.4. Conclusions
This study used GC-MS combined with PCA and FDA to discover the variation rules of fatty acids in various samples. It established mathematical models in the prediction of camellia seed oils content level and the accuracy of the models reached 92.5%. Meanwhile, the prediction results for the new test set also reached 88%, which confirmed that the discriminant model was robust and efficient. As shown in this paper, we used a more complex simulation where three types of edible oils were added into camellia seed oil at various percents to better recreate the conditions in the real market. This was different from many previous studies that focused on camellia seed oil adulterated with a single type of edible oil.
As a universally accepted method, GC-MS obtained the fatty acid information of oil samples and caught the subtle change between pure camellia seed oil and mixture. It sets the foundation for the chemometric analysis. In the part of PCA, the differences in the distribution of the samples with different content of camellia seed oil could be clearly found in the score plot of PC1 and PC2. It succeeded in reducing the dimensionality of original data and made it easier for subsequent analysis. Based on this, FDA was used to create the classification models which predicted the content level of camellia seed oil without identifying the nature or the number of different types of the edible oils used as adulterants. Conclusively, this model proposed in this study focused on the camellia seed oil itself, which could be used effectively for the detection of untargeted and multivariate adulteration of pure camellia seed oil. It also had some practicability for the quality supervision and control of the diversity of edible camellia seed blend oil in the market.
However, it should be noted that in this research only soybean oils, peanut oils, and rapeseed oils were involved as adulterants. There were a few misjudgments that existed because of the lack of the number of training samples. In subsequent research, massive datasets for experimentation should be collected to establish the comprehensive database of camellia seed oils to increase the prediction accuracy of the identification model. Furthermore, more diverse adulteration oil types and complex adulteration processes also be tried to applied to the models to improve the practical possibilities of the model.
Supplemental Material
Download MS Word (119.3 KB)Acknowledgments
The authors gratefully acknowledge the support from the Scientific Research Innovation Program of Hunan (CX20190507), Natural Science Foundation of Hunan Province (2019JJ50262), the Hunan Engineering Technology Research Center for Rapeseed Oil Nutrition Health and Deep Development.
Disclosure statement
No potential conflict of interest was reported by the author(s).
Supplementary material
Supplemental data for this article can be accessed on the publisher’s website.
References
- Ayari, F., Mirzaee-Ghaleh, E., Rabbani, H., & Heidarbeigi, K. (2018). Detection of the adulteration in pure cow ghee by electronic nose method (case study: Sunflower oil and cow body fat). International Journal of Food Properties, 21(1), 1670–1679. https://doi.org/https://doi.org/10.1080/10942912.2018.1505755
- Casale, M., Sinelli, N., Oliveri, P., Di Egidio, V., & Lanteri, S. (2010). Chemometrical strategies for feature selection and data compression applied to NIR and MIR spectra of extra virgin olive oils for cultivar identification. Talanta, 80(5), 1832–1837. https://doi.org/https://doi.org/10.1016/j.talanta.2009.10.030
- Constantin, C. (2014). Principal component analysis-a powerful tool in computing marketing information. Bulletin of the Transilvania University of Brasov. Economic Sciences. Series V, 7(2), 25.
- Croux, C., Filzmoser, P., & Fritz, H. (2013). Robust sparse principal component analysis. Technometrics, 55(2), 202–214. https://doi.org/https://doi.org/10.1080/00401706.2012.727746
- Dou, X. J., Mao, J., Zhang, L. X., Xie, H. L., Chen, L., Yu, L., Ma, F., Wang, X. P., Zhang, Q., & Li, P. W. (2018). Multispecies adulteration detection of camellia oil by chemical markers. Molecules, 23(2), 241–251. https://doi.org/https://doi.org/10.3390/molecules23020241
- GB/T 11765-2018, oil tea camellia seed oil. Beijing: Standards Press of China, 2018.
- GB/T 2716-2018, edible vegetable oil. Beijing: Standards Press of China, 2018.
- Hajimahmoodi, M., Vander Heyden, Y., Sadeghi, N., Jannat, B., Oveisi, M. R., & Shahbazian, S. (2005). Gas-chromatographic fatty-acid fingerprints and partial least squares modeling as a basis for the simultaneous determination of edible oil mixtures. Talanta, 66(5), 1108–1116. https://doi.org/https://doi.org/10.1016/j.talanta.2005.01.011
- Herman-lara, E., Bolivar-Moreno, D., Toledo-lopez, V. M., Cuevas-gldry, L. F., Ldpe-navarrete, M. C., Barrdn-zambrand, J. A., Díaz-rovera, P., & Ramírez-rovera, E. D. (2019, May 9). Minerals multi-element analysis and its relationship with geographical origin of artisanal Mexican goat cheeses. Food Science and Technology, 39(Suppl. 2), 517–525. https://doi.org/https://doi.org/10.1590/fst.23918
- Holland, S. M. 2008. Principal components analysis (PCA). Department of Geology, University of Georgia. 30602-2501.
- Jiménez-Carvelo, A. M., González-Casado, A., & Cuadros-Rodríguez, L. (2017). A new analytical method for quantification of olive and palm oil in blends with other vegetable edible oils based on the chromatographic fingerprints from the methyl-transesterified fraction. Talanta, 164, 540–547. https://doi.org/https://doi.org/10.1016/j.talanta.2016.12.024
- Karoui, R., Mazerolles, G., Bosset, J. O., Baerdemaeker, J. D., & Dufour, E. (2007). Utilisation of mid-infrared spectroscopy for determination of the geographic origin of Gruyère PDO and L’Etivaz PDO Swiss cheeses. Food Chemistry, 105(2), 847–854. https://doi.org/https://doi.org/10.1016/j.foodchem.2007.01.051
- Kim, N. S., Lee, J. H., Han, K. M., Kim, J. W., Cho, S., & Kim, J. (2014). Discrimination of commercial cheeses from fatty acid profiles and phytosterol contents obtained by GC and PCA. Food Chemistry, 143, 40–47. https://doi.org/https://doi.org/10.1016/j.foodchem.2013.07.083
- Li, B., Wu, Q., & Liu, Z. (2020). Identification of mine water inrush source based on PCA-FDA: Xiandewang coal mine case. Geofluids, 2020. https://doi.org/https://doi.org/10.1155/2020/2584094
- Li, C., Yao, Y., Zhao, G., Cheng, W., Liu, H., Liu, C., & Wang, S. (2011). Comparison and analysis of fatty acids, sterols, and tocopherols in eight vegetable oils. Journal of Agricultural and Food Chemistry, 59(23), 12493–12498. https://doi.org/https://doi.org/10.1021/jf203760k
- Li, M. J. (2019, September 20). Analysis of the Chinese competitive landscape in edible oil market in 2019. https://www.qianzhan.com
- Li, T. P., & Wang, T. Y. (2019). A research of the relationship between food brand awareness and quality grade –with cooking oil as an example. Journal of Southwest University (Natural Science Edition), 41(4), 57–63. https://doi.org/https://doi.org/10.13718/j.cnki.xdzk.2019.04.008
- Liu, L., Yan, J. M., Zhao, H. R., Li, D. D., & Tian, Y. M. (2012). Application method of Principle component analysis and Cluster analysis in comprehensive evaluation of students’ accomplishment. Journal of Liaoning University of Technology (Natural Science Edition), 32(3), 200–204. https://doi.org/https://doi.org/10.15916/j.issn1674-3261.2012.03.001
- Nansen, C., Geremias, L. D., Xue, Y., Huang, F., & Parra, J. R. (2013). Agricultural case studies of classification accuracy, spectral resolution, and model over-fitting. Applied Spectroscopy, 67(11), 1332–1338. https://doi.org/https://doi.org/10.1366/12-06933
- Quintanilla-Casas, B., Bertin, S., Leik, K., Bustamante, J., Guardiola, F., Valli, E., Bendini, A., Toschi, T. G., Tres, A., & Vichi, S. (2020). Profiling versus fingerprinting analysis of sesquiterpene hydrocarbons for the geographical authentication of extra virgin olive oils. Food Chemistry, 307, 125556. https://doi.org/https://doi.org/10.1016/j.foodchem.2019.125556
- Quintanilla-Casas, B., Marin, M., Guardiola, F., García-González, D. L., Barbieri, S., Bendini, A., Gallina Toschi, T., Vichi, S., & Tres, A. (2020). Supporting the sensory panel to grade virgin olive oils: An in-house-validated screening tool by volatile fingerprinting and chemometrics. Foods, 9(10), 1509. https://doi.org/https://doi.org/10.3390/foods9101509
- Ruiz-Samblás, C., Marini, F., Cuadros-Rodríguez, L., & González-Casado, A. (2012). Quantification of blending of olive oils and edible vegetable oils by triacylglycerol fingerprint gas chromatography and chemometric tools. Journal of Chromatography B, 910, 71–77. https://doi.org/https://doi.org/10.1016/j.jchromb.2012.01.026
- Shi, T., Zhu, M., Chen, Y., Yan, X., Chen, Q., Wu, X., & Xie, M. (2018). 1H NMR combined with chemometrics for the rapid detection of adulteration in camellia oils. Food Chemistry, 242, 308–315. https://doi.org/https://doi.org/10.1016/j.foodchem.2017.09.061
- Siddiqi, M. H., Ali, R., Khan, A. M., Park, Y. T., & Lee, S. (2015). Human facial expression recognition using stepwise linear discriminant analysis and hidden conditional random fields. IEEE Transactions on Image Processing, 24(4), 1386–1398. https://doi.org/https://doi.org/10.1109/TIP.2015.2405346
- Su, M. H., Shih, M. C., & Lin, K. H. (2014). Chemical composition of seed oils in native Taiwanese Camellia species. Food Chemistry, 156, 369–373. https://doi.org/https://doi.org/10.1016/j.foodchem.2014.02.016
- Sun, P. Y., Bao, K. W., Li, H. S., Li, F. J., Wang, X. P., Cao, L. X., Li, G. M., Zhou, Q., Tang, H. X., & Bao, M. T. (2018). An efficient classification method for fuel and crude oil types based on m/z 256 mass chromatography by COW-PCA-LDA. Fuel, 222(1), 416–423. https://doi.org/https://doi.org/10.1016/j.fuel.2018.02.150
- Tang, F. (2011). Study on fatty acids fingerprints and identification method of adulteration in vegetable oils. Hunan agricultural University.
- Tfouni, S. A. V., Reis, R. M., Amaro, N. D. P. L., Pascoal, C. R., de Camargo, M. C. R., Baggio, S. R., & Furlani, R. P. Z. (2017). Adulteration and presence of polycyclic aromatic hydrocarbons in extra virgin olive oil sold on the Brazilian market. Journal of the American Oil Chemists’ Society, 94(11), 1351–1359. https://doi.org/https://doi.org/10.1007/s11746-017-3046-3
- Vinicius, L. S., Eustáquio, V. R., Castro, R. C. C., Pereira, V. M. D. P., & Isabel, C. P. F. (2007). Use of Principal Component Analysis (PCA) and linear discriminant analysis (LDA) in Gas Chromatographic (GC) data in the investigation of gasoline adulteration. 21(6), 3394–3400. https://doi.org/https://doi.org/10.1021/et0701337
- Wang, C. Q. (2016) Quantitative analysis of oat flour in oat-wheat mixed powders based on fatty acids [Master’s thesis]. Jiangnan University.
- Wang, R. Y., Liu, K., Wang, X., & Tan, M. (2019). Detection of sesame oil adulteration using low-field nuclear magnetic resonance and chemometrics. International Journal of Food Engineering, 15(7), 7. https://doi.org/https://doi.org/10.1515/ijfe-2018-0349
- Wang, Z. (1999). Food chemsity (1st ed.). China Light Industry Press.
- Wu, W. G., Peng, S. M., Tan, F., Liu, J. G., & Tian, M. (2013). Study on fatty acids standard fingerprint of 5 kind of vegetable oils and their similarity. Journal of Chinese Cereals and Oils Association, 28(6), 101–105. https://doi.org/https://doi.org/10.3969/j.issn.1003-0174.2013.06.021
- Xu, Z. H., Huan, X. Y., Lin, L., Wan, Q. F., & Zhan, H. F. (2018). Dendrolimus punctatus walker damage detection based on Fisher discriminant analysis and Random forest. Spectroscopy and Spectral Analysis, 38(9), 2888. https://doi.org/https://doi.org/10.3964/j.issn.1000-0593(2018)09-2888-09
- Yu, C. L. (2011) Feature selection based on feature extraction [Master’s thesis]. Nanjing Post and Communications University.
- Zhu, H., Wang, Y., Liang, H., Chen, Q., Zhao, P., & Tao, J. (2010). Identification of Portulaca oleracea L. From Different Sources Using GC–MS and FT-IR Spectroscopy. Talanta, 81(1–2), 129–135. https://doi.org/https://doi.org/10.1016/j.talanta.2009.11.047
- Zhu, M. T., Shi, T., Chen, Y., Luo, S. H., Leng, T., Wang, Y. L., Guo, G., & Xie, M. Y. (2019). Prediction of fatty acid composition in camellia oil by 1H NMR combined T with PLS regression. Food Chemistry, 279, 339–346. https://doi.org/https://doi.org/10.1016/j.foodchem.2018.12.025